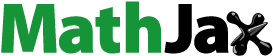
Abstract
This study delves into dynamics and determinants of agricultural exports from India. India’s agricultural export basket is heavily reliant on a limited range of commodities, including basmati rice, buffalo meat, spices, tea, coffee, and marine products. Such concentration poses risks, making the sector vulnerable to price fluctuations, changes in global demand, and challenges in accessing specific markets. Furthermore, the declining ratio of export value to import value in recent years indicates an unfavourable trade imbalance. To address these challenges and foster sustainable growth in the agricultural export sector, policymakers must gain a comprehensive understanding about the determinants for agricultural exports. So, this study utilizes panel data encompassing 40 agricultural export items over an 11-year period. The researchers employ the system Generalized Method of Moments (GMM) estimation and the findings showed positive and significant impact of past export performance on current export decisions. Moreover, the study highlights the positive and significant influences of gross output of agriculture, value-added activities in agricultural sector, gross domestic product, trade openness, foreign direct investment, water use efficiency, corruption index and exchange rate dynamics on quantum of agricultural exports. However, higher consumer prices have a negative effect on export quantities, emphasizing the importance of price competitiveness in international markets. The findings of this study provide valuable insights for diversifying the export basket, enhancing productivity, value addition, and sustainability. Addressing challenges related to trade imbalances and price competitiveness is crucial for driving growth in India’s agricultural sector, benefiting farmers, the economy, and the nation as a whole.
Impact Statement
This study sheds light on the dynamics and determinants of agricultural exports from India, offering valuable insights for policymakers and stakeholders. By analyzing a panel dataset of 40 agricultural export items using the system Generalized Method of Moments (GMM) estimation, the study uncovers key factors influencing export performance. The findings underscore the importance of past export momentum, agricultural output, economic factors, water use efficiency, corruption levels, population size, and consumer prices in shaping agricultural exports. Importantly, the study highlights the need for diversifying India's agricultural export portfolio to mitigate risks associated with concentration on a few commodities, address trade imbalances, and enhance export competitiveness. The recommendations provided, including focusing on sustainable growth, enhancing productivity, value addition, trade policy reforms, attracting foreign investment, and investing in skill development, are crucial for fostering long-term growth and stability in India's agricultural export sector. Despite certain limitations, this study lays a solid foundation for future research and policy interventions aimed at ensuring sustainable development and resilience in India's agricultural exports.
REVIEWING EDITOR:
Subjects:
1. Introduction
In today’s globalized world, countries possess varying resource endowments and technological advancements in the production of goods and services. The advent of liberalization, privatization, and globalization has aimed to facilitate the enhancement of agricultural exports. These processes primarily focus on reducing tariffs and non-tariff barriers within a liberalized trade regime. India, in particular, stands to gain numerous advantages by leveraging these reforms to boost the exports of agricultural commodities through promoting comparative advantage in the global market (Navjit, Citation2011; Dani, 2012).
Liberalization is crucial in expanding agricultural exports by reducing tariffs. Lower import duties on agricultural products allow India to improve its competitiveness in global markets. This reduction enables Indian exporters to access foreign markets more easily, thereby expanding export opportunities for agricultural commodities. Additionally, liberalization efforts aim to address non-tariff barriers such as quotas, licenses, and sanitary and phytosanitary measures (Martin, Citation2017; Michele et al., Citation2009).
Privatization in the agricultural sector promotes efficiency and productivity. Increased competition, innovation, and management expertise from private entities lead to improved production, processing, and marketing capabilities. It also encourages investment in value addition and processing facilities for agricultural commodities. This enables India to export processed and value-added products, such as packaged foods, beverages, and processed ingredients. By moving up the value chain, India can capture a larger share in global market and command higher prices for its agricultural exports (Abhishek et al., Citation2019; Rehman, Citation2012).
Globalization enhances agricultural exports by facilitating the exchange of technology and knowledge between countries. India can benefit from advancements in agricultural practices, machinery, and research through collaborations, partnerships, and foreign direct investment. Adoption of modern technologies enhances agricultural productivity, efficiency, and sustainability, positioning India as a reliable supplier of high-quality agricultural products in the global market. Globalization promotes Foreign Direct Investment (FDI) inflows that can contribute to the modernization of Indian agriculture, including the adoption of advanced farming techniques, irrigation systems, and machinery. Increased investment promotes agricultural growth, strengthens supply chains, and improves India’s overall competitiveness in agricultural exports.
All these measures facilitate smoother trade flows and provide Indian farmers and agribusinesses with access to larger consumer bases, increasing export volumes and revenues (FAO, Citation2017). This also leads to increased exports, technological upgrading, and knowledge spill-overs, creating a positive feedback loop for India’s agricultural sector. Besides above, the differences in resource endowments and production methods between countries lead to variations in factor prices. These variations in factor prices, in turn, result in differences in product prices. India, with its diverse climate, fertile land, and abundant labour force, possesses a natural advantage in production of certain agricultural commodities. These resources, combined with modern agricultural practices and knowledge, enable India to produce agricultural commodities more efficiently and at lower costs compared to many other countries. Thus, Indian agricultural products become attractive to international buyers, leading to increased exports.
Specializing in highly productive agricultural products at the initial stage of economic development has been observed in several Asian countries, including India. This specialization allows these countries to leverage their comparative advantage and gain a foothold in the global market. As these countries continue to develop, they gradually transition from exporting labour-intensive products to capital-intensive export products. This process of structural transformation is essential for enhancing competitiveness. For India, it means progressing from primary agricultural exports to value-added and processed agricultural products. By adding value through processing and developing capabilities in the production of higher-value agricultural goods, India can further boost its agricultural exports and capture a larger share in global market.
The agricultural export basket of India is primarily composed of commodities such as basmati rice, buffalo meat, spices, tea, coffee, and marine products. These commodities have traditionally been the main drivers of India’s agricultural exports. The concentration of exports on a few commodities indicates lack of diversification in India’s agricultural export portfolio. This can pose risks in terms of severe price fluctuations, changes in global demand, and potential challenges in market access for specific commodities. Furthermore, the ratio of export value to import value of India has decreased recently (). This declining ratio signals an unfavourable trade imbalance leading to potential economic implications. So, understanding the reasons behind this and the factors influencing agricultural exports is crucial, as it is a critical source of foreign exchange earnings in India (Kumareswaran et al., Citation2019). Moreover, agricultural sector plays a pivotal role in the Indian economy, employing 60% of population and contribute 18.6% in Gross Domestic Product (GDP) in 2021–2022. So, strengthening agricultural exports can drive growth in this sector, benefiting rural livelihoods and overall economic development. Additionally, agricultural exports generate income and employment opportunities for farmers and related industries. This study represents a significant departure from previous research paradigms, which have primarily focused on broad trends in total agricultural export values or on analyzing the determinants of individual agricultural commodities using gravity models. By honing in on the nuanced intricacies of Indian agricultural exports, it provides policymakers with a comprehensive understanding of the sector’s underlying strengths and weaknesses. This deeper analysis empowers policymakers to craft targeted trade policies and strategies aimed at not only boosting exports but also enhancing overall competitiveness and reducing import dependency. Utilizing a dynamic panel data approach, this study delves into the multifaceted factors influencing the dynamics of agricultural exports from India. By adopting this sophisticated analytical framework, the study effectively unravels the complex web of variables shaping the trajectory of agricultural exports, offering invaluable insights to policymakers, researchers, and stakeholders alike. As India’s agricultural sector assumes an increasingly central role in the global economy, the findings of this study are poised to be instrumental in informing evidence-based policy formulation and driving strategic decision-making processes. Moreover, given the ever-evolving nature of the global agricultural trade landscape, the insights generated by this study are expected to retain their relevance over time. They are anticipated to serve as a vital compass for navigating future challenges, harnessing emerging opportunities, and steering India towards greater prosperity in the realm of agricultural exports.
2. Review of literature
Theory of international trade has undergone various interpretations and advancements over time. While the absolute advantage theory provided a framework for understanding trade, it failed to explain why nations with an absolute advantage in the production of all goods engage in international trade (Carbaugh, Citation2011). This led to the development of comparative advantage theory. According to Suranovic (Citation2006), comparative advantage theory states that even if a nation has no absolute advantage in the production of goods, it can still benefit from international trade by specializing on a particular good in which its relative advantage is higher.
Samuelson (Citation2001) introduced the term ‘Sraffa bonus’ to denote the gains from trading inputs. However, it took considerable time until Shiozawa (Citation2017) successfully remedied this deficiency, leading to the development of new theory of international values, which now stands as the sole theory capable of comprehensively handling input trade in a general context. Drawing on ideas from Fujimoto, an expert in the automobile industry and a philosopher of international competitiveness, Fujimoto (Citation2001) and Shiozawa (Citation2017) delved into discussions on how factories of the same multinational firms compete across borders. This notion of international intra-firm competition represents a novel aspect of international competition in the era of so-called global competition.
The emergence of revolutionary changes in communication and information techniques, coupled with substantial reductions in transport costs, has enabled a historic fragmentation of the production process, leading to the formation of networks of fragmented production across countries known as global value chains (Escaith & Miroudot, Citation2016; WTO, Citation2013). This development has transformed our understanding of trade and international economy. However, traditional theories have remained dominated by assumptions of complete goods trade, prompting the need for a new paradigm to better comprehend the implications of these trends (Escaith & Miroudot, Citation2016; Grossman & Rossi-Hansberg, Citation2006). The Extended Ricardian trade model accounted for trade of input goods and the emergence of global value chains. Based on this novel theory, which Shiozawa terms the ‘theory of international values,’ he explains why and how global value chains have rapidly spread world-wide by the end of twentieth century (Shiozawa, Citation2020). Unemployment is closely linked to international trade (David et al., Citation2014). Shiozawa’s (Citation2020) discovery of a new definition of regular international value has enabled the construction of a new theory that accommodates unemployment within international trade scenarios.
Previous studies have empirically investigated the relationship between various factors and volume of agricultural exports in different countries. Argaie (Citation2021) and Bereket (Citation2020), reported a positive and significant correlation between exchange rate and export volume. Conversely, Joshi and Ghosh (Citation2021), Lilik et al. (Citation2020), Sertoglu and Dogan (Citation2016) and Abebemarkos (Citation2016) found negative and statistically significant relationship. For instance, Xu et al. (Citation2023) discovered a negative and significant link between the exchange rate and export volume in Vietnam. Chi and Cheng (Citation2016) highlighted about impact of exchange rate volatility on Australia’s maritime exports. On the other hand, Huchet-Bourdon and Korinek (Citation2011) opined that exchange rate fluctuations have minimally affected trade flows in China. Eshetu and Mehare (Citation2020), Cheffo (Citation2020), Geda and Seid (Citation2015) and Kebede (Citation2016) revealed a positive and significant association between GDP and agricultural exports. Fassil and Abule (2020), Sekar and Mathanraj (Citation2021), and Mengistu (Citation2014) found negative and significant association between FDI and agricultural export volume, unlike Xu et al. (Citation2023) in Vietnam. Trade openness showed positive and significant relationship with volume of agricultural exports in studies conducted by Eshetu and Mehare (Citation2020), Bereket (Citation2020), Cheffo (Citation2020), and Gebrehiwot and Gebru (Citation2015). Sekar and Mathanraj (Citation2021) and Narayan and Bhattacharya (Citation2019) found negative and significant relationship between Consumer Price Index (CPI) and agricultural exports.
Empirical studies have delved into the intricate relationship between various factors and the volume of agricultural exports in different countries. While some studies have revealed positive correlations between factors such as exchange rates, GDP, and trade openness with agricultural export volume, others have reported negative correlations. For instance, the impact of exchange rate fluctuations on export volume varies across countries, as evidenced by studies conducted in Vietnam and China. Similarly, the relationship between factors like FDI and CPI with agricultural exports also exhibits variations. Given the diverse findings from empirical studies on agricultural exports in various countries, it becomes evident that there is no one-size-fits-all approach to understanding these dynamics. The complexities of factors such as exchange rates, GDP fluctuations, FDI inflows, and trade openness underscore the need for nuanced analysis. Hence, tailored approaches are necessary to unravel the specific drivers operating within India’s unique socio-economic and agricultural landscape. In the context of India, where agricultural exports play a crucial role in the economy, there is a pressing need for further research to understand the specific factors that impact agricultural export volume. Given the significant contribution of agricultural exports to the Indian economy, it is imperative to evaluate the impact of both demand-side and supply-side factors on Indian agricultural exports. While previous studies predominantly focused on analyzing the determinants of total export value through time-series econometrics or the determinants of individual agricultural commodities using the gravity model, this study takes a unique approach. It employs panel data encompassing 40 agricultural export items and utilizes the system Generalized Method of Moments (GMM) for estimation. Specifically, the study aims to evaluate the trade performance of the Indian agricultural sector and comprehensively investigate the determinants of Indian agricultural exports, leveraging the robustness and efficiency offered by the GMM estimation technique.
3. Materials and methods
This study takes a more comprehensive approach by utilizing panel data of 40 agricultural export items (N) across 11 years (T) from 2011 to 2021 comprising of 440 observations and employing the system GMM estimation.
3.1. Data sources and variables
The selected sample of top 40 agricultural export items from India () account for 66% of total quantity of agricultural and livestock exports in 2021. The selection of these agricultural export items is likely based on their significant contribution to India’s total agricultural and livestock exports, thus providing a comprehensive representation of the country’s export portfolio. These commodities are chosen based on their high export volumes, economic importance, strategic focus within India’s agricultural export strategy, diversity across various product categories, and the availability of reliable data from sources such as the FAO. While these products may not directly align with clusters identified under the agricultural export policy, they offer valuable insights into India’s export performance and potential areas for growth and improvement. The requisite secondary data were collected from international institutions and provides a description of the variables.
Table 1. Shares of selected commodities in terms of total quantity of agricultural & livestock exports (2021).
Table 2. Data description and sources (2011–2021).
The selected determinants encapsulate a comprehensive array of factors crucial for understanding the dynamics of agricultural exports from India. These variables have been chosen based on their direct and indirect impacts on export performance and their relevance to the Indian agricultural context. Factors such as TO, GDP, and EXR reflect macroeconomic conditions and global trade dynamics, influencing the competitiveness of Indian agricultural products in international markets. CPI, GOA, and VAA offer insights into domestic demand, sectoral productivity, and value addition, which are essential for shaping export volumes. Additionally, variables like FDI, DFA, and GFCF capture investment inflows critical for modernizing agricultural practices and infrastructure, thus enhancing export capacity. EMP and POP underscore the significance of labor dynamics in agricultural productivity and export competitiveness. Furthermore, the CI serves as a proxy for governance quality, which impacts trade facilitation and investor confidence, ultimately influencing export performance. Collectively, these determinants provide a robust framework for analyzing the multifaceted drivers of agricultural exports from India, considering both internal and external factors shaping export outcomes.
3.2. Tools of analysis
3.2.1. Panel unit root test for stationarity
The Levin-Lin-Chu (LLC) panel data unit root test is employed to assess the stationarity of panel data (Levin et al., Citation2002; Fassil & Abule, 2020). It takes into account both the cross-sectional (commodities) and time-series dimensions of panel data, making it a powerful tool for analyzing non-stationarity in this context. The HO of this test is: ‘panel data series has a unit root, indicating non-stationarity’ against HA: ‘data are stationary’. This test estimates a panel-specific ADF regression for each cross-sectional unit in the dataset, and then combine individual unit root test statistics to obtain a pooled test statistic.
3.2.2. Generalized method of moments (GMM)
For analyzing dynamic panel data model, the GMM outperforms Ordinary Least Squares (OLS), Random Effects, and Fixed Effects models for several reasons. OLS estimation do not address endogeneity, leading to biased estimates. Random Effects and Fixed Effects models assume uncorrelated individual-specific effects and explanatory variables, potentially leading to biased estimates in the presence of correlation. GMM allows for both individual-specific effects and time-invariant covariates as instruments, offering robust estimates (Nickell, Citation1981; Jiatao, 2021). It is a desirable estimation technique because it allows researchers to exploit both internally generated instruments (endogenous instruments) and externally supplied instruments (exogenous instruments) to address endogeneity issues. Internally generated instruments or variables can be created from within the model itself. Externally supplied instruments or variables are chosen externally to the model and their selection requires a careful consideration of the theoretical framework, empirical relevance, correlation with the endogenous variable, exogeneity, exclusion restriction, strength, data availability, heterogeneity, redundancy, and instrument exclusion tests. Even GMM also suffers from few limitations viz., sensitivity to instrument choice, a requirement for large sample sizes, potential identification issues, susceptibility to misspecification, computational intensity, and difficulties in interpretation. Despite these limitations, GMM remains a valuable tool in econometric analysis, particularly for addressing endogeneity issues in dynamic panel data models. Two widely used approaches in GMM are Difference GMM and System GMM. Difference GMM involves first-differencing the model to eliminate commodity-specific effects in panel data analysis (Wooldridge, Citation2002, Citation2010). However, it can suffer from weak instrumentation when past levels provide insufficient information for predicting future changes in transformed variables. Moreover, it may not effectively identify time-invariant or slowly changing variables (say, WUE). Additionally, the difference transformation can magnify gaps in the data. This estimator causes a loss of information (data) by subtracting the past value of a variable from its current value. These potential pitfalls warrant the use of System GMM by combining equations in both first-differences and levels (EquationEqs. 1(1)
(1) and Equation2
(2)
(2) ). According to Blundell and Bond (Citation1998), the system GMM estimator outperforms the difference GMM estimator because the instruments in the level equation remain good predictors for the endogenous variable. By utilizing both transformed and untransformed variables, System GMM takes advantage of additional moment conditions, leading to improved finite sample properties in terms of bias and root mean squared error. Thus, System GMM is more robust in dealing with weak instrumentation and provides more efficient estimates (Maurice & Frank, Citation2007).
(1)
(1)
(2)
(2)
In EquationEq. (1)(1)
(1) , ‘
’ is the export item-specific fixed effect, ‘
’ ∼ N (0,
) is the random term, ‘
’ and ‘
’ are independently and identically distributed. In system GMM estimation, the system of EquationEqs. (1)
(1)
(1) and Equation(2)
(2)
(2) uses two sets of instruments, where Zi = ZD+ZL. Here, ZD represents the instruments for the first difference model, while ZL represents the instruments for the level model. So, system GMM estimator is a weighted average of difference and level coefficients, with the first difference equation estimated using the lagged level value as an instrumental variable, and the level equation estimated using the lagged differences of the endogenous variables as instruments (Wintoki et al., Citation2012).
During estimation process, several tests are conducted to ensure validity of instruments and overall model specification in GMM analysis. The Arrellano–Bond Serial Correlation tests (Arellano & Bond, Citation1991), denoted as AR1 and AR2, assess the presence of first and second-order autocorrelation in error term of the first difference equation, respectively. Since the errors in the levels are assumed to be uncorrelated, it is expected to find significant AR1 and non-significant AR2, which confirms validity of the instruments. The Hansen and Sargan statistics are employed to examine the orthogonality condition of each over-identifying instrument. A non-significant Hansen test implies that the chosen instrumental variables meet the necessary conditions for consistency and efficiency in the GMM estimation, thereby supporting the credibility of the instruments. Similarly, a non-significant Sargan test provide further evidence for validity of instrumental variables, indicating that the GMM results are less likely to suffer from endogeneity bias (Eichenbaum et al., Citation1988). Furthermore, a non-significant result of Hansen test for 'GMM instruments for levels,' reinforces the credibility of the instrumental variables used in level form of the model. Similarly, a non-significant outcome of Hansen test for 'instrument variables,' supports the credibility of the selected instrumental variables. It suggests that the GMM results are less likely to suffer from endogeneity bias, and the instruments utilized in the analysis are appropriate for addressing potential endogeneity concerns.
GMM is particularly well-suited for analyzing short panels (T < 25 and N > 25) (Roodman, Citation2009). So, this study using 11 years data across 40 agricultural export items allowed the researchers to use a dynamic panel data model (Cremers et al., Citation2017) and GMM estimation to address potential endogeneity issues, heteroskedasticity, and measurement errors, enabling more efficient and robust parameter estimation. Though, N > T violates classic linear regression assumptions, the use of GMM offers several advantages for panel data analysis that make it well-suited for this context. It efficiently handles panel data by capturing time-series and cross-sectional variations simultaneously while exploiting moment conditions derived from economic theory. GMM's ability to address endogeneity, account for unobserved heterogeneity, and produce robust estimates despite data limitations makes it the preferred method for analyzing the determinants of agricultural exports in this study.
4. Results and discussion
4.1. Trade performance of Indian agricultural sector
4.1.1. Share of the top 10 commodities in total agricultural exports from India
This accounts for 52.42% averaged over the period 2012–2021 (). This substantial share underscores the significant role these commodities play in driving India’s agricultural export revenues and trade balances. Among these commodities, rice milled equivalent holds the highest share at 24.29%, followed by refined sugar at 5.77%, and wheat at 4.08%. These commodities are essential export drivers due to factors such as high domestic production, global demand, and competitive pricing. Additionally, commodities like maize, cotton lint, and onions also hold notable shares, indicating their importance in India’s export market. This indicates that slightly over half of India’s agricultural export volume during this period can be attributed to these top 10 commodities. Their significant contribution underscores their importance in India’s agricultural export portfolio and highlights their role in driving export revenues and trade balances (NABARD (National Bank for Agriculture & Rural Development), 2021).
4.1.2. Destination-wise agricultural exports
highlights that Bangladesh holds the largest share of total Indian agricultural products’ export (11.46%). The strong economic ties and geographical proximity between the two countries contribute to this substantial share, making Bangladesh a major destination for Indian agricultural exports (Rahul et al., Citation2015). UAE follows closely with a share of 6.48%, driven by its status as a regional trade hub and its demand for diverse agricultural products. The USA and Vietnam also hold notable shares of 5.05 and 5.01%, respectively, reflecting their sizable markets and India’s ability to cater to their specific agricultural needs. Saudi Arabia, Nepal, Malaysia, and Indonesia contribute with shares ranging from 4.54 to 4.03%, indicating India’s successful presence in various Asian markets. The "Others" category represents the cumulative share of smaller markets and is at 54.74%, indicating agricultural exports are widely distributed globally. The varying destination-wise export shares in India’s agricultural sector can be attributed to several factors (SASEC, Citation2020). Geographical proximity and regional trade agreements influence exports to neighbouring countries like Bangladesh and Nepal. Demand and consumption patterns in specific regions drive exports to countries like UAE and Saudi Arabia reflecting their preferences and climatic conditions. Strong trade relationships with USA and Vietnam facilitate market access for Indian agricultural products. Additionally, marketing efforts, trade promotions, and export incentives encourage market penetration and competitiveness. The share of "Others" highlights the need to diversify export markets and explore opportunities in emerging economies. India’s ability to cater to diverse markets, leveraging agricultural strengths, trade relations, and marketing efforts, sustains and expands the agricultural export sector.
4.1.3. Share of agricultural exports in national exports during post-WTO regime
indicates that agricultural exports had substantial growth from 6.1 billion US$ in 1995–1996 to 50.2 billion US$ in 2021–2022. This growth is reflective of India’s efforts to enhance its agricultural productivity, diversify its product range, and tap into global markets. Similarly, national exports have also witnessed a significant rise, expanding from 31.79 billion US$ in 1995–96 to 335.44 billion US$ in 2021–2022. The overall growth in India’s export sector can be attributed to various factors, including economic liberalization, globalization, trade agreements, and advancements in transportation and communication, which have facilitated access to international markets. However, share of agricultural exports in national exports has shown some fluctuations over the years (Gulati, Citation2018). After an initial increase during early 2000s, the share declined and then fluctuated between 9 and 15% until the mid-2010s. Subsequently, there was an upward trend, reaching 14.97% in 2021–2022. These trends can be attributed to several factors. Advancements in agricultural technology and research have improved productivity and met global quality standards, enhancing India’s competitiveness. Trade liberalization under the WTO has facilitated market access, promoting export growth. Government policies, export promotion schemes, and market diversification strategies have also been instrumental. Changing consumer preferences and growing demand for Indian products like spices, tea, rice, and fruits have driven exports. Additionally, improved cold chain infrastructure and agro-processing industries have increased value addition and export potential. Challenges include regulations, non-tariff barriers, price volatility, and environmental concerns. Addressing these challenges and investing in export-oriented policies will sustain and boost India’s agricultural exports (Government of India, Citation2020).
4.1.4. Trends in agricultural exports and imports at national level during post-WTO regime
depicted agricultural exports have shown a gradual upward trajectory, rising from 6.1 billion USD in 1995–1996 to 41.56 billion USD in 2020–2021. This is due to advancements in agricultural technology, improved productivity, increased market access, and supportive government policies and export promotion initiatives. Additionally, changing consumer preferences and growing international demand also contribute for this trend. Similarly, agricultural imports have also exhibited an increasing trend, growing from 1.76 billion USD in 1995–1996 to 21.47 billion USD in 2020–2021 due to rise in demand for certain agricultural products that are not sufficiently produced domestically (Kumar, Citation2021a, Citationb). Changing consumption patterns and preferences also contribute to the need for importing specific commodities. Additionally, agricultural imports are necessary to supplement domestic supply and ensure a stable market availability of certain products.
Figure 4. Trends in Agricultural Exports and Imports at National Level during post-WTO regime (1995–1996 to 2021–2022).

The share of agricultural exports in total national exports has varied from 19.18% in 1995–1996 to 14.2% in 2020–2021. Similarly, the share of agricultural imports in total national imports has fluctuated from 4.8% in 1995–1996 to 5.42% in 2020–2021. These fluctuations can be influenced by various factors, including changes in global market conditions, fluctuations in international commodity prices, and shifts in domestic demand and production patterns. Trade policies, exchange rates, and geopolitical factors also determine the trade balance and the share of agricultural trade in the overall economy. Weather conditions impact crop yields and supply levels, affecting the need for imports or export surpluses. Changing trade agreements and partnerships with countries also influence agricultural trade dynamics (Gulati et al., Citation2019; Malini, Citation2021). These trends are subject to a complex interplay of domestic and international factors. Enhancing agricultural productivity, quality, and value addition, along with strategic trade policies, are crucial for sustainable growth.
4.1.5. Ratio of agricultural exports to agricultural imports during post-WTO regime
The average ratio of agricultural exports to agricultural imports in India had shown fluctuating trends over the years (). From 1995–1996 to 2004–2005, the ratio fluctuated between 3.4 and 1.8. Subsequently, from 2004–2005 to 2013–2014, the average ratio increased to 3.2, indicating a slight improvement in the relative value of agricultural exports compared to imports. This period may have been influenced by factors like increased agricultural productivity, market diversification, and favorable global market conditions. However, from 2013 to 2014 onwards, the average ratio declined to 1.70 due to changing global trade dynamics, exchange rate fluctuations, geopolitical events, and the impact of the COVID-19 pandemic on international trade (Serpil & Mehmet, Citation2020; OECD, 2020). These factors have affected both agricultural exports and imports, contributing to the shift in the trade balance. These fluctuations reflect the dynamic nature of India’s agricultural trade (Agricultural Policies in India, Citation2018; Agriculture Export Policy, Citation2018). The ratio is affected by a combination of domestic and international factors, including agricultural productivity, trade policies, global market conditions, exchange rates, and geopolitical events. Analyzing the trends, we can observe that the ratio has experienced significant variations from year to year. In some years, the ratio has been relatively higher, signifying a surplus in agricultural trade, while in other years, the ratio has been lower, indicating a trade deficit in the agricultural sector (Kumar, Citation2021a, Citationb).
4.2. Panel unit root test
presents the outcomes of LLC test for selected variables, evaluating both their level and first difference forms, with and without intercept and trend. This critical test aims to ascertain the stationarity of variables prior to employing panel data GMM model. Remarkably, LogEQ and LogEMP are identified as integrated of order zero, I(0) at 1% level. Consequently, these variables demonstrate stationarity in their level form and can be directly utilized in GMM models without any transformation. Conversely, other variables are found to be integrated of order one, I(1), at significance level, indicating their non-stationarity in their level form, necessitating first differencing to achieve stationarity (Hu, Citation2012; Maddala & Wu, Citation1999). Therefore, it is advisable to employ their first difference forms in GMM analysis to overcome spurious regression. By taking into account the stationarity properties of the variables, panel data GMM model provide reliable and accurate estimates, ensuring robust and valid findings in the study.
Table 3. LLC panel data unit root test results for selected variables.
4.3. Determinants for agricultural exports (quantity) from India
Appendix 1 shows the smaller pair-wise correlation values between explanatory variables indicates the absence of multicollinearity in the model estimation. In , System GMM is the preferred method for interpreting findings over Difference GMM to analyze the determinants for agricultural exports from India (Blundell & Bond, Citation1998; Bond, Citation2002; Fassil & Abule, Citation2020; Sandip et al., Citation2022) The positive coefficient of 0.6627 (significant at p < 0.01) for lagged export quantity suggests that past export performance significantly influences current export decisions. This indicates that positive momentum in export quantities can lead to continued demand from existing international markets, encouraging exporters to maintain or increase their shipments. For each one percent increase in lagged export quantity, the study estimates a 0.66% increase in current export quantity.
Table 4. Regression results of difference GMM, one-step and two-step system GMM.
Similarly, ‘Gross Output of Agriculture’ demonstrates a highly significant positive effect on agricultural exports. The substantial positive coefficient of 6.7755 (significant at p < 0.01) for gross output of agriculture highlights its crucial impact on agricultural exports. A 1% increase in gross agricultural output leads to a substantial 6.78% increase in agricultural export quantity. A larger agricultural output provides a surplus of goods available for export, enabling India to meet international demand. This might be driven by technological advancements, improved farming practices, or favourable weather conditions, leading to increased agricultural production (Waseem & Aditi, Citation2017). Moreover, the positive coefficient of 3.2039 (significant at p < 0.01) for value added from agriculture suggesting that value-added activities in agriculture positively impact agricultural exports (Nugroho et al., Citation2021). Each 1% increase in value added from agriculture corresponds to approximately a 3.20% increase in agricultural export quantity, emphasizing the significance of value enhancement for export competitiveness. The positive coefficient of 2.9113 (significant at p < 0.05) for water use efficiency highlights its positive influence on agricultural exports. Efficient water usage in agriculture can lead to increased crop yields, allowing for more agricultural products to be available for both domestic consumption and export. So, a one-unit increase in water use efficiency leads to approximately a 2.91% increase in agricultural export quantity, highlighting the importance of sustainable water practices for export growth. Further, the positive coefficients for GDP (0.0705, significant at p < 0.01), TO (0.5122, significant at p < 0.05), FDI (0.5201, significant at p < 0.05), and EXR (1.9429, significant at p < 0.01) indicate that economic factors play a crucial role in influencing agricultural exports (Teng & Dic, Citation2019). These variables reflect the significance of economic growth, reduced trade barriers, foreign investments, and exchange rate dynamics in driving export quantities. Higher GDP contributes to higher agricultural exports through increased domestic demand, investments in the agricultural sector, improved productivity, enhanced infrastructure, and enhanced competitiveness in the global market. As the overall economy thrives, the agricultural sector benefits, leading to a positive impact on export of agricultural products from India. Trade openness fosters a conducive environment for agricultural exports by expanding market access, creating export opportunities, capitalizing on comparative advantage, facilitating technological transfer and innovation, attracting foreign investment, improving price competitiveness, contributing to economic growth, and enhancing risk management. Embracing trade openness allows India’s agricultural sector to thrive in the global marketplace and capitalize on international demand for its products. The result of FDI also signifies that FDI plays a crucial role in elevating the agricultural sector and, subsequently, contributing to higher agricultural exports. The influx of capital, technology, market access, distribution networks, and expertise brought by foreign investors creates an enabling environment for Indian farmers and exporters to meet international standards, expand their reach, and seize export opportunities in the global marketplace. Though the relationship between exchange rates and agricultural exports is complex, but a currency devaluation or an increase in the exchange rate can lead to a decrease in the relative price of exports, thereby contributing to a higher agricultural export (Bereket, Citation2020; Javed et al., Citation2020; Mao, Citation2019; Sekar & Mathanraj, Citation2021; Siyakiya, Citation2016).
Furthermore, the positive coefficient of 0.0445 (significant at p < 0.01) for the CI indicate that a one point increase in CI, increase the quantum of agricultural export by 0.04 units and statistically significant at 5% level. Since the corruption index lies between 1 (weak governance) and 89 (strong governance), the higher the value of the index, the lower the corruption level. Studies conducted by Eshetu and Mehare (Citation2020; Kebede, Citation2016) also found a positive and statistically significant effect of CI on the export flow of Ethiopia.
On the other hand, the negative coefficient of −7.5517 (significant at p < 0.05) for population suggests that a larger population negatively impacts the export quantity of agricultural products. That is, increased domestic demand reduce the quantities available for export, leading to a 7.55% decrease in export quantity for each 1% increase in population (Martina et al., Citation2015). So, a higher population may lead to increased domestic consumption, limiting the surplus available for export. Additionally, a larger population may require a greater share of resources, potentially reducing the resources available for export-oriented production.
The negative coefficient of −1.9210 (significant at p < 0.01) for CPI suggest that an increase in consumer prices negatively affects agricultural export quantities. So, 1.92% decrease in agricultural export quantity is estimated for each 1% increase in the consumer price index, emphasizing the importance of price competitiveness in international market. So, higher consumer prices may reduce the demand for agricultural products in international markets, leading to decreased export volumes (Nazir et al., Citation2022).
Finally, lack of statistical significance for the coefficient of employment in agriculture (−0.3161) suggests that employment in agriculture sector do not have a clear and robust impact on agricultural exports. The employment-output gap in this sector indicates low labour productivity and lower exportable surplus after catering to domestic needs. Subsistence farming practices and small landholdings prioritize self-consumption over commercial trade. Moreover, farmers’ limited engagement in export-oriented farming, infrastructural constraints, and policy barriers hinder access to international markets. Price fluctuations and competition from other agricultural-exporting countries further add to the challenges.
The findings from this study have not deviated from prior expectations or prior research primarily because they align with existing theoretical frameworks and empirical evidences from determinants for agricultural exports. A significant value of Wald-χ2 indicates that at least one of the instruments used in the GMM estimation is jointly significant in explaining the variation in the endogenous variables. A non-significant value of the Arellano-Bond test for AR(2) in first differences suggests that there is no evidence of second-order autocorrelation in the differenced errors, unlike for Arellano-Bond test for AR(1) in first differences. This suggests that there might be first-order autocorrelation in the model errors but no evidence of second-order autocorrelation. The Sargan test of overidentification restrictions is non-significant and this implies that the instruments used in the model are not jointly correlated with the error term. Similarly, as the Hansen test of overidentification restrictions is found non-significant, it indicates that the instruments used in the model are not correlated with the error term after accounting for the moment conditions specified in the model. This result suggests that the instruments are valid and that the model adequately addresses the issue of endogeneity.
5. Conclusions and suggestions
The study focuses on understanding the dynamics and determinants for agricultural exports from India. The agricultural export basket of India is heavily dominated by few commodities and this pose risks in terms of vulnerability to price fluctuations, changes in global demand, and challenges in market access for specific commodities. Additionally, the declining ratio of export value to import value in recent years indicates an unfavourable trade imbalance. To address these challenges and promote sustainable growth in the agricultural export sector, policymakers must understand the factors influencing export performance. The study utilizes panel data of 40 agricultural export items and employs the system Generalized Method of Moments (GMM) estimation to analyze the determinants of agricultural exports from India. The results revealed that past export performance has a significant positive influence on current export decisions, indicating the importance of maintaining positive momentum in export quantities to retain existing international markets. The gross output of agriculture and value-added activities in agriculture also play crucial roles in driving agricultural exports, as higher agricultural output and value addition contribute to increased export competitiveness. Economic factors, such as GDP, TO, FDI, and EXR have a significant impact on agricultural exports. The study also highlights the significance of WUE in influencing agricultural exports, as sustainable water practices lead to increased crop yields and export growth. The CI also influences agricultural exports, with lower corruption levels positively impacting export quantities. A transparent and accountable governance system fosters export growth. However, a larger population negatively impacts agricultural exports, as increased domestic demand may limit the surplus available for export. Additionally, higher consumer prices also have a negative effect on export quantities. In conclusion, the study underscores the importance of diversifying India’s agricultural export portfolio to mitigate risks associated with concentration on a few commodities.
Policymakers must address challenges related to trade imbalances and take steps to enhance export competitiveness by focusing on sustainable growth and enhancing competitiveness in India’s agricultural exports. Diversification of the export basket is crucial to reduce dependence on a few commodities and mitigate market volatility risks. Policymakers should focus on enhancing productivity, using advanced technology, and improving farming practices to meet domestic demand and bolster exports. Value addition through processing and branding can elevate the market value and competitiveness of Indian exports. Effective trade policy reforms, trade agreements, and partnerships are essential to facilitate market access for agricultural products. Attracting FDI in the agricultural sector can bring advanced technologies and global market access. Sustainable water management, anti-corruption measures, and price competitiveness are vital for export success. Investing in skill development and research will equip farmers and exporters for international markets. Implementing these strategies can strengthen India’s agricultural exports, benefitting farmers, the economy, and overall development. Policymakers must prioritize these measures to ensure a thriving agricultural export sector and foster long-term growth and stability.
While the study provides valuable insights into the determinants of India’s agricultural exports and offers actionable policy recommendations, it has certain limitations that warrant consideration. One notable limitation is the omission of market access issues faced by agricultural exports in developed countries. Ignoring these challenges may underestimate the true complexities of the export environment, potentially leading to incomplete policy prescriptions. Additionally, the study focuses primarily on macro-level determinants and may overlook micro-level factors that could significantly impact export performance. Furthermore, the analysis could benefit from a more granular examination of commodity-specific dynamics to capture the unique challenges and opportunities within individual agricultural products. Moreover, the study’s timeframe and sample size, while providing valuable insights, may limit the generalizability of findings and overlook longer-term trends or emerging patterns. Despite these limitations, the study lays a solid foundation for future research endeavors. Future studies could address these gaps by incorporating a more comprehensive analysis of market access issues, adopting a finer-grained approach to understand commodity-specific dynamics, and extending the analysis over a longer timeframe to capture evolving trends. Additionally, exploring market diversification beyond traditional destinations, assessing the adoption of advanced technologies for enhanced productivity and quality, and analyzing the effectiveness of trade policies in promoting exports can inform targeted interventions. Furthermore, research focusing on value addition, branding strategies, sustainable water management, and governance structures can contribute to fostering long-term growth and competitiveness in India’s agricultural export sector. Addressing these areas can deepen our understanding and guide policymakers toward strategies that ensure sustainable development and resilience in India’s agricultural exports.
Author’s Contributions
K.N.R.K., conceptualization, methodology, data collection, data curation, data analysis, and writing—initial draft; A.B.S., expert comments and suggestions—revisions; G. M. N data collection, data curation, expert suggestions. All the authors have read and agreed to the published version of the manuscript.
Acknowledgments
We appreciate the sharing of ideas and suggestions provided by Dr. K. Sowjanya, Assistant Professor - Department of Food Processing Technology, ANGRAU during the early stage of this investigation.
Disclosure statement
The authors declare no conflict of interest.
Data availability statement
The data presented in this study are available on request from the authors.
Additional information
Notes on contributors
K. Nirmal Ravi Kumar
Dr. K. Nirmal Ravi Kumar holding Master's and Ph.D. degrees in Agricultural Economics, has studied at Acharya N.G. Ranga Agricultural University (ANGRAU). He has a brilliant academic career with specialization in ‘Agricultural Marketing’ both in his postgraduate and doctoral programmes. Dr. Kumar is currently Professor & Head (Agril. Economics) in Agricultural College, Bapatla. He is actively involved both in agricultural research and teaching activities during the past twenty-two years in the ANGRAU. He also worked as Director (Agricultural Marketing) in National Institute of Agricultural Extension Management (MANAGE), Ministry of Agriculture & Farmers’ Welfare, Government of India. He was the recipient of ‘Sri Mocherla Dattatreyulu Gold Medal’ (2013) and ‘State Best Teacher Award’ (2016). Dr. Kumar has written extensively and has to his credit 10 books, and six (6) published articles in reputable journal of higher impact.
G. Mohan Naidu
Adinan B. Shafiwu, Ph.D. Adinan B. Shafiwu, is currently a J-Pal African Scholar who has just finished his project on Digitalization of Cocoa Value Chain in Ghana under the DigiFi initiatives. He has worked and participated in J-pal initiatives that involves Randomized Control Trial. As part of his training under J-Pal he participated in both DIWA and Development Methodologies workshops here in Ghana and Morocco-Rabat respectively. He holds a PhD and MPhil in (Agricultural Economics) and also a chartered Health Economics certificates and currently works with the University for Development Studies in the capacity as a lecturer. He is an expert in Adoption Studies, efficiency, welfare and consumption studies. He is into modeling and has deep seated knowledge in econometrics and its models. A good data manager. He has also consulted for Plan International Ghana and Oxfarm on both midline and Baseline survey. He has published over twenty (20) research articles in reputable journals with higher impact. He is Highly regarded for his good leadership, communication, proposal writing, research, and analytical skills. Able to establish, implement and monitor systems for effectiveness and efficiency at organizations.
Adinan Bahahudeen Shafiwu
Dr. G. Mohan Naidu, is currently working as Professor in the Department of Statistics and Computer Applications, in SV Agricultural College, Tirupati. Dr. Naidu’s significant contributions underscore his steadfast commitment to advancing statistical techniques for the betterment of the student community. His multifaceted endeavors epitomize a profound dedication to driving positive change within the agricultural sector through conducting numerous research studies of both national and international acclaim. With an extensive portfolio, Dr. Naidu has authored approximately 50 research articles published in esteemed national and international journals, showcasing his expertise and impact in the field.
References
- Abebemarkos, S. (2016). Determinants of export trade in econometric study with special reference to Ethiopia. International Journal of Science and Research, 5(12), 132–137.
- Abhishek, D., Lal Sharma, V., Rajgaria, S., & Aruna, P. (2019). A case study on privatization of agriculture in the states of Odisha and Chhattisgarh. JETIR, 6(3), 543–556. http://www.jetir.org/papers/JETIR1903575.pdf
- Aday, S., & Aday, M. S. (2020). Impact of COVID-19 on the food supply chain. Food Quality and Safety, 4(4), 167–180. https://doi.org/10.1093/fqsafe/fyaa024
- Agricultural Policies in India. (2018). OECD food and agricultural reviews. OECD/ICRIER. OECD Publishing. https://doi.org/10.1787/9789264302334-en
- Agriculture Export Policy. (2018). Ministry of Commerce, Government of India.
- Anand, R., Kochhar, K., & Mishra, S. (2015). Make in India: Which exports can drive the next wave of growth? (IMF Working Paper Asia and Pacific Department. WP/15/119). International Monetary Fund. ISBN/ISSN: 9781513597393/1018-5941.
- Arellano, M., & Bond, S. (1991). Some tests of specification for panel data: Monte Carlo evidence and an application to employment equations. The Review of Economic Studies, 58(2), 277–297. https://doi.org/10.2307/2297968
- Argaie, S. T. (2021). The impact of exchange rate on Ethiopia’s Coffee export. European Scientific Journal ESJ, 17(23), 27. https://doi.org/10.19044/esj.2021.v17n23p27
- Autor, D. H., Dorn, D., Hanson, G. H., & Song, J. (2014). Trade adjustment: Worker-level evidence. The Quarterly Journal of Economics, 129(4), 1799–1860. https://doi.org/10.1093/qje/qju026
- Basarac Sertic, M., Vuckovic, V., & Skrabic Peric, B. (2015). Determinants of manufacturing industry exports in European Union member states: A panel data analysis. Ekonomska Istraživanja [Economic research], 28(1), 384–397. https://doi.org/10.1080/1331677X.2015.1043781
- Bereket, I. (2020). The determinate of agricultural export in Ethiopia: An error correction modle and cointegration approach. Journal of Economics and Sustainable Development, 11(3), 51–57.
- Blundell, R., & Bond, S. (1998). Initial conditions and moment restrictions in dynamic panel data models. Journal of Econometrics, 87(1), 115–143. https://doi.org/10.1016/s0304-4076(98)00009-8
- Bond, S. R. (2002). Dynamic panel data models: A guide to micro data methods and practice. Portuguese Economic Journal, 1(2), 141–162. https://doi.org/10.1007/s10258-002-0009-9
- Bun, M. J. G., & Windmeijer, F. (2007). The weak instrument problem of the system GMM estimator in dynamic panel data models. The Econometrics Journal, 13(1), 95–126. https://doi.org/10.1920/wp.cem.2007.0807
- Carbaugh, R. J. (2011). International economics (13th ed.). South-Western Cengage Learning.
- Cheffo, R. W. (2020). Determinants of agricultural exports in Ethiopia: A gravity model approach. African Journal of Economic Review, 8(1), 95–106.
- Chi, J., & Cheng, S. (2016). Do exchange rate volatility and income affect Australia’s maritime export flows to Asia? Transport Policy, 47, 13–21. https://doi.org/10.1016/j.tranpol.2015.12.003
- Cremers, K. J. M., Litov, L. P., & Sepe, S. M. (2017). Staggered boards and long-term firm value, revisited. Journal of Financial Economics, 126(2), 422–444. https://doi.org/10.2139/ssrn.2364165
- Eichenbaum, M. S., Hansen, L. P., & Singleton, K. J. (1988). A time series analysis of representative agent models of consumption and leisure choice under uncertainty. The Quarterly Journal of Economics, 103(1), 51–78. https://doi.org/10.2307/1882642
- Escaith, H., & Miroudot, S. (2016). Industry-level competitiveness and inefficiency spillovers in global value chains. In 24th International Input-Output Conference, Seoul, Korea. https://www.iioa.org/conferences/24th/papers/files/2437_20160523071_HESMTiVAandSupplySideSeoul2016finaldraft.pdf.
- Eshetu, F., & Mehare, A. (2020). Determinants of Ethiopian agricultural exports: A dynamic panel data analysis. Review of Market Integration, 12(1–2), 70–94. https://doi.org/10.1177/0974929220969272
- FAO. (2017). The future of food and agriculture – Trends and challenges. Rome.
- Fujimoto, T. (2001). The evolution of a manufacturing system at Toyota. Productivity Press.
- Gebrehiwot, G., & Gebru, B. (2015). Ethiopia’s foreign trade potential: Inferences from a dynamic gravity approach. International Journal of Economics and Business Research, 9(4), 355–375. https://doi.org/10.1504/IJEBR.2015.069667
- Geda, A., & Seid, S. (2015). Determinants of export performance of Ethiopia: A firm-level analysis. Ethiopian Journal of Economics, 23(1), 45–76.
- Government of India. (2020). "Growing India’s Agricultural Exports through Crop Specific, State-led Plans", Submission to the XV Finance Commission, New Delhi.
- Grossman, G., & Rossi-Hansberg, E. (2006). The rise of offshoring: It’s not wine for cloth anymore. American Economic Review, 98(5), 978–997.
- Gulati, A. (2018). “Doubling Farmers’ Income: Here’s Why Boosting Agri-Exports is Important”. Financial Express, Delhi Edition.
- GulatI, A., Kapur, D., & Bouton, M. (2019). Reforming Indian Agriculture, ICRIER Working Paper Centre for the Advanced Study of India
- Hu, Y. (2012). Growth of Asian pension assets: Implications for financial and capital markets, ADBI Working Paper, No. 360, Asian Development Bank Institute (ADBI), Tokyo.
- Huchet-Bourdon, M., & Korinek, J. (2011). To what extent do exchange rates and their volatility affect trade?. OECD Trade Policy Papers, No. 119, OECD Publishing. https://doi.org/10.1787/5kg3slm7b8hg-en
- Javed, I., Rehman, A., Nabi, I., Razzaq, A., Saqib, R., Bakhsh, A., Mohibullah, M., & Luqman, M. (2020). Performance and macroeconomic determinants of basmati rice exports from Pakistan. Sarhad Journal of Agriculture, 36(2), 617–624. https://doi.org/10.17582/journal.sja/2020/36.2.617.624
- Joshi, N., & Ghosh, I. (2021). Determinants of trade in services. Mednarodno Inovativno Poslovanje = Journal of Innovative Business and Management, 13(1), 72–78. https://doi.org/10.32015/JIBM/2021.13.1.72-78
- Kebede, W. (2016). Determinants of Ethiopia’s agricultural export performance: A gravity model approach. Journal of Agricultural Sciences, 61(3), 269–282.
- Kumar, V. (2021a). Strategies to Improve Performance of Agri-exports”, Writings on Rural Economy: Charting the Way to Rural Prosperity". National Bank for Agriculture and Rural Development, pp.28–31
- Kumar, V. (2021b). Trends and performance of India’s Agricultural Trade in the midst of COVID-19 pandemic. Indian Journal of Agricultural Economics, 76(3), 352–370.
- Kumareswaran, T., Jolia, P., Maurya, M., Maurya, A., Abbasmandri, S., & Kamalvanshi, V. (2019). Export scenario of Indian agriculture: A review. Journal of Pharmacognosy and Phytochemistry, 8(1), 2653–2656.
- Levin, A., Lin, C. F., & Chu, C. S. J. (2002). Unit root tests in panel data: Asymptotic and nite-sample properties. Journal of Econometrics, 108(1), 1–24. https://doi.org/10.1016/S0304-4076(01)00098-7
- Maddala, G. S., & Wu, S. (1999). A comparative study of unit root tests with panel data and a new simple test. Oxford Bulletin of Economics and Statistics, 61(S1), 631–652. https://doi.org/10.1111/1468-0084.0610s1631
- Malini, L. T. (2021). Policy and Performance of Agricultural Exports in India, Institute for Social and Economic Change, Working Paper No. 509.
- Mao, R. (2019). Exchange rate effects on agricultural exports. China Agricultural Economic Review, 11(4), 600–621. https://doi.org/10.1108/CAER-12-2017-0232
- Martin, W. (2017). Agricultural trade and food security (No. 664). ADBI Working Paper. https://www.adb.org/publications/agriculturaltrade-and-food-security.
- Mengistu, A. A. (2014). Ethiopia’s export performance with major trade partners: A gravity model approach. Journal of Natural Sciences Research, 4(20), 21–28.
- Michele, A., Fattouh, B., Ferrarini, B., & Scaramozzino, P. (2009). Tariff Liberalization and Trade Specialization in India, ADB Economics Working Paper Series No. 177.
- Naik, G. (2021). Agricultural trade with special reference to plantation crops and international trade agreements. Indian Journal of Agricultural Economics, 76(1), 109–143.
- Narayan, S., & Bhattacharya, P. (2019). Determinants of India’s agricultural exports: An empirical analysis. International Journal of Scientific Research and Modern Education, 4(2), 1062–1071. https://doi.org/10.1016/j.worlddev.2018.12.013
- Nazir, S., Javed, I., Luqman, M., & Azra, A. (2022). Mandarin exports from Pakistan and its macroeconomic determinants. Sarhad Journal of Agriculture, 38(2), 563–571. https://doi.org/10.17582/journal.sja/2022/38.2.563.571
- Nickell, S. (1981). Biases in dynamic models with fixed effects. Econometrica, 49(6), 1417–1426. https://doi.org/10.2307/1911408
- Nugroho, A. D., Bhagat, P. R., Magda, R., & Lakner, Z. (2021). The impacts of economic globalization on agricultural value added in developing countries. PLoS One, 16(11), e0260043. https://doi.org/10.1371/journal.pone.0260043
- Rehman, S. (2012). Privatization in Indian Agriculture - An Overview. Available at SSRN: https://ssrn.com/abstract=2387278 or https://doi.org/10.2139/ssrn.2387278
- Roodman, D. (2009). How to do xtabond2: An introduction to difference and system GMM in Stata. The Stata Journal: Promoting Communications on Statistics and Stata, 9(1), 86–136. https://doi.org/10.1177/1536867X0900900106
- Samuelson, P. A. (2001). A Ricardo-Sraffa paradigm comparing gains from trade in inputs and finished goods. Journal of Economic Literature, 39(4), 1204–1214. 10.1257/jel.39.4.1204
- SASEC. (2020). Potential Exports and Nontariff Barriers to Trade, India National Study, ADB. DOI https://doi.org/10.22617/TCS190172-2
- Sekar, P., & Mathanraj, T. (2021). An economic analysis of India’s Spices exports. Palarch’s Journal of Archaeology of Egypt/Egyptology, 18(8), 3706–3718.
- Sertoglu, K., & Dogan, E. (2016). Determinants of Turkish agricultural exports: A gravity model approach. Journal of Economics and Sustainable Development, 7(12), 159–167.
- Shiozawa, Y. (2017). The new theory of international values: An overview. A new construction of Ricardian theory of international values: Analytical and historical approach, 3–73.
- Shiozawa, Y. (2020). A new framework for analyzing technological change. Journal of Evolutionary Economics, 30(4), 989–1034. https://doi.org/10.1007/s00191-020-00704-5
- Singh, N. (2011). Indian agriculture: Before and after economic reforms. European Journal of Business and Management, 3(4), 292–298.
- Siyakiya, P. (2016). An econometric analysis of Zimbabwe’s export competitiveness. Maghreb Review of Economics and Management, 3, 1–14.
- Solanki, S., Singh, S., & Murthy, I. K. (2022). Agricultural exports and performance of agricultural firms in India: An empirical analysis using system GMM. Asian Economic and Financial Review, 12(2), 121–131. https://doi.org/10.55493/5002.v12i2.4431
- Sugiharti, L., Purwono, R., & Esquivias, M. A. (2020). Analysis of determinants of Indonesian agricultural exports. Entrepreneurship and Sustainability Issues, 7(4), 2676–2695. https://doi.org/10.9770/jesi.2020.7.4(8)
- Suranovic, S. M. (2006). International trade theory and policy. In S. M. Suranovic (Ed.), International trade theory and policy analysis. Flat World Knowledge.
- Waseem, A. K., & Aditi, A. (2017). A causal relationship between agricultural production and exports: An impact on Indian economy. International Journal of Business Management & Research, 7(2), 91.
- Wintoki, M. B., Linck, J. S., & Netter, J. M. (2012). Endogeneity and the dynamics of internal corporate governance. Journal of Financial Economics, 105(3), 581–606. https://doi.org/10.1016/j.jfineco.2012.03.005
- Wooldridge, J. M. (2002). Econometric analysis of cross section and panel data. The MIT Press.
- Wooldridge, J. M. (2010). Econometric analysis of cross section and panel data. The MIT Press.
- WTO. (2013). Global value chains in a changing world. Edited by Deborah K. Elms and Patrick Low. WTO Publications.
- Xu, H., Nghia, D. T., & Nam, N. H. (2023). Determinants of Vietnam’s potential for agricultural export trade to Asia-Pacific economic cooperation (APEC) members. Heliyon, 9(2), e13105. https://doi.org/10.1016/j.heliyon.2023.e13105
- Yue, T., & Lo, D. (2019). Determinants of developing countries’ export upgrading: The role of china and productive investment (SOAS Department of Economics Working Paper No. 227) (p. 227). SOAS University of London.