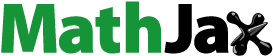
Abstract
The purpose of the study is to determine the most relevant method of a time series analysis to identify the cyclicality in the development of regional economies. In pursuit of the goal, a comparative approach was employed, focusing on the utilization of Gross Regional Product (GRP) indicators from the regions within the Central Federal District of the Russian Federation. Statistical and econometric methods were also employed to analyze the changes in these indicators over time (from 1998 to 2019). The relevance of this study manifests in its capacity to elucidate the cyclic processes within the development of regional economies, thereby enabling the identification of strategies to influence them toward the attainment of sustainable and balanced development goals. The study revealed that the majority of the examined regions remain in the lowest quartile of Gross Regional Product (GRP), while two regions consistently feature in the highest GRP quartile. The research findings hold practical implications for policy decisions and regional development strategies, aiding in understanding economic dynamics and supporting the implementation of effective management measures to bolster regional development. Based on the research findings, it is recommended to intensify support for regions with low GDP by providing additional investments and infrastructural development, as well as adapting successful development strategies. Political decisions should be grounded in cyclical trends, taking into account the needs and opportunities of each region. Future research efforts may focus on refining the spectral analysis method for a more precise and reliable analysis of GRP dynamics at the regional level.
Impact statement
This research has shown that the distribution of growth in the gross regional product is not statistically normal and therefore it is necessary to complement traditional narrative statistics with other methods. The results of our spectral analysis confirmed our hypothesis about the usefulness of this method. An introduced model for spectral analysis of GRP treats it as a specific case of the regression model using discrete Fourier transformation. Considering the persistent trend of low levels of regional product in certain regions juxtaposed with consistently high levels in others, we recommend the development of a national strategy encompassing specialized programs to support and foster the regions in the lowest quartile of regional product. Policymakers should actively incentivize investments in regions with low levels of regional product, directing financial and other resources to bolster economic growth and infrastructural development.
Reviewing Editor:
1. Introduction
The specific objective of this study was to perform a spectral analysis of the Gross Domestic Product of the regions of the Central Federal District of Russia to detect the similarity or disparities in the regional development. We suppose this method to be a useful addition to traditional statistics analytic instruments to determine the existence or absence of cyclical dynamics in economic activity in the named District. We also intended to define the limitations of the study. The implementation of the method proposed can solve the problem of designing an “evidence-based” macroeconomic policy for regional development.
In the global context of economic and social sciences, the issue revolves around the instability and unpredictability of cyclical processes at various levels of administrative and territorial organization. The primary challenge lies in the lack of clarity in understanding the interaction between national and regional levels of these cycles. Thus far, there has been inadequate comprehension of how events and processes at the national level influence regional economic and social dynamics, and vice versa.
National and regional governments, as well as business structures, often base decisions on economic and social forecasts. Inaccurate forecasts can lead to failures and stress for citizens and households. Therefore, the importance of understanding these cyclical processes for the development and implementation of policies in the realms of economics, social development, and regional planning is essential for stability and effective governance. Hence, the problem that this research seeks to address involves unraveling the nature and interplay of cyclical regularities at the national and regional levels, utilizing the "Prism" methodology for the meso-level. The study of regional dynamics is an important task for the formulation of state macroeconomic policies aimed at minimizing the possible negative impact of the cyclical development of the economy. The uneven development of federal entities is well known (Economist, Citation2018), but most of the work on this topic is limited to citing statistical data, comparing growth/decline rates, etc.
The objective is to use a proven statistical tool, which, as we say below, provides an incomplete information base for further analysis. The main challenge faced by many states is the differences in regional development. Strategic spatial planning should be based on an analysis of the dynamics of regional dynamics and the identification of the causes of differences. This issue has been studied by many researchers analyzing trends of gross regional product and similar indexes (Sturn & Epstein, Citation2021).
Hence, it is essential to examine developmental imbalances within the framework of economic growth theory, such as the Robert Solow theory developed by Mankiw et al. (Citation1992), as was done in the work of Burakov et al. (Citation2019); their paper also ‘takes Robert Solow seriously’. This approach has many proponents and opponents. For instance, Nelson and Winter (Citation1990) contend that Solow’s theory offers limited explanatory power for the observed productivity growth. We did not (and do not) aim to criticize theories of economic growth, especially as our study showed the relative closeness of these rates in the regions studied during the period under investigation. The regions analyzed have a similar infrastructure for economic growth in terms of Robert Solow’s theory: every region has higher and secondary vocational education institutions, occupied by what is called the ‘production of knowledge’.
These educational institutions (primarily higher education institutions) are concentrated in regional centers, which corresponds to the signs of an effective geographical concentration of intellectual resources. The research states that ‘cognitive centers’ (Rossi-Hansberg et al., Citation2019) have formed, but there is no productive return from them. Some regions of the Central Federal District managed to overcome a peculiar ‘middle-income trap’ and show a higher rate of growth of gross regional product than others at the same time. The specific objective of this study was to complement traditional macroeconomic research methods with a spectral time series analysis of the gross regional product. This is even more important, as the COVID-19 pandemic not only has confirmed regional differences in development but has also added differences in the impact of the pandemic and the resulting restrictive measures observed in individual regions.
Considering a theory, we can state an existing conceptual issue. Correia et al. (Citation2020) state that ‘non-pharmaceutical interventions’ (NPI) minimized the ‘Spanish flu’ consequences of the epidemic of ‘Spanish flu’ in 1918 in cities of the United States; Lilley et al. (Citation2020) argue that NPI did not have a positive impact on post-pandemic recovery in US cities. It is assumed that the implementation of a spectral time series analysis of the gross regional product can become a conceptual and empirical response to the actual call for ‘evidence-based policymaking’ that is currently increasing (Bukharbayeva et al., Citation2020; Umirzakov et al., Citation2019). According to Weible et al. (Citation2020), ‘evidence-based policymaking’ replaces normative orientations and political expediency.
Considering the provided information, the research gap lies in the insufficient analysis of the interaction between national and regional cycles, complicating evidence-based policy formulation in the realms of economics, social development, and regional planning. The motivation of the study rests in the necessity to unveil the nature of cyclic processes within the development of regional economies, aiming to enhance the effectiveness of regional development strategies and facilitate informed decision-making in economic and social development spheres. The identified issue stems from an inadequate understanding of the interaction between national and regional levels of these cycles, thereby complicating the development of effective development strategies and evidence-based decision-making.
The relevance and significance of this study lie in addressing the critical issue of inadequate understanding of cyclic processes in the development of regional economies. Unveiling these cycles enables the development of more effective regional development strategies and the formulation of informed decisions in the realms of economics, social development, and regional planning. The application of spectral analysis in this research represents an innovative approach that aids in the identification and analysis of these cyclic processes with greater precision. Consequently, the outcomes of this study will have a considerable impact on the formulation of regional development policies and will contribute to stable and balanced economic growth.
The contribution of this article lies in the application of spectral analysis to investigate cyclic processes in the development of regional economies, particularly within the framework of the Central Federal District of Russia. This method enables the identification of similarities or disparities in the dynamics of regional economic development. Thus, the article proposes a novel approach to analyzing these processes, which is significant in the context of developing effective strategies for regional development and policy formulation.
Hence, this study will contribute to an enhanced comprehension of cyclical regularities and their interplay between the national and regional levels of the economy. This will aid in better understanding how events at the national level impact regional dynamics and vice versa.
Additionally, this research has the potential to address some of the existing gaps through:
Expanding the volume of data: Increasing access to regional-level data and conducting more detailed analyses can help unravel complex dependencies.
Employing new methods of analysis: Implementing state-of-the-art statistical and econometric analysis methods may lead to better modeling and understanding of cyclical regularities.
Comparing different regions: By comparing diverse regions and scrutinizing their interconnections, a more comprehensive understanding of how cyclical regularities impact various parts of the country can be achieved.
The research findings can have practical significance for policymakers, economists, and decisions related to regional development and national strategy. They can be utilized for making management decisions that contribute to the effective development of regions.
The limitations of previous research in this area include insufficient data and methodological challenges. However, this study aims to overcome these limitations by employing new analytical methods and expanding the scope of available data. Through the comparative analysis of diverse regions and the examination of their interrelations, our objective is to advance comprehension of cyclical phenomena at both national and regional scales. This endeavor seeks to provide a noteworthy contribution to the exploration of this pivotal facet of socio-economic dynamics.
The primary aim of this research is to determine the most effective method for time series analysis in detecting cyclical variations within the development of regional economies. Additionally, the principal objective involves comprehending the interdependencies between national and regional levels of economic and social processes using the "Prism" methodology for meso-level analysis. The research objectives are as follows:
Analysis of cyclical phenomena at the national level.
Analysis of cyclical phenomena at the regional level.
Determination of the interrelation between national and regional levels.
Utilization of the "Prism" methodology for meso-level analysis.
Research hypotheses:
Hypothesis 1:
There is a cyclical dynamics at the national level that influences regional dynamics and vice versa.
Hypothesis 2:
The “Prism” methodology for the meso-level enables better detection and analysis of cyclical patterns than traditional analysis methods.
Hypothesis 3:
Regional peculiarities and characteristics impact the degree of coincidence of cyclical patterns between regions and the national level.
The Section 2 contains an overview of the recent studies published in 2019–2021. In the Section 3 we describe the data on the gross regional product of the regions of the Central Federal District of Russia collected by the Russian Federation Federal State Statistics Service. The Section 4 contains exploratory results of the data analysis and the spectral analysis including fast Fourier transformation of the time series of the Central Federal District’s gross regional product for the period 1998–2019 obtained by us. The research findings and limitations are compared with similar studies in the Section 5. Our policy implication and recommendation of the proposed method can be found under Section 6.
2. Literature review
The first applications of spectral analysis to economic research date back to the mid-1960s, most notably works by Nerlove (Citation1964) and Granger (Citation1966, Citation1969) and Granger and Hatanak (Citation1964) who won the 2003 Nobel Prize in Economics for developing time series analysis techniques. The Clive Granger approach found its continuation in a considerable amount of literature, including Mao Takongmo and Lebihan (Citation2021), dedicated to the so-called ‘Granger causality’ in the correlation between government spending and Gross External Product (the GDP) of the United States, as well as in Ye and Zhang (Citation2018) on the ‘Granger causality’ between health care spending and economic growth in OECD countries.
Atesoglu and Vilasuso (Citation1999) used spectral analysis to study exports and economic growth in the US. Harvey and Jaeger (Citation1993) showed that the application of statistical filters (such as the Hodrick-Prescott filter) to time series can lead to artificial cycles. Extensive research has shown cyclical patterns of economic development (Owens & Sarte, Citation2005; Pollock, Citation2008). Hughes-Hallett and Richter (Citation2004, Citation2007) in their studies used a spectral analysis for European business cycle dynamics. Batorova (Citation2012), Kapounek and Pomenkova (Citation2010), and Maršálek and Poměnková (Citation2010a, Citation2010b, Citation2011) conducted research on Eastern European economies as part of a spectral analysis. Croux et al. (Citation1999, Citation2005, Citation2008) used spectral analysis to test the hypothesis that the European Union can be considered as a single market. We did not find papers by Russian researchers on the GDP dynamics and GRP in spectral analysis except for papers by Korotayev and Tsirel (Citation2010), and Monakhov and Chechurin (Citation2012).
In the last few years, the application of spectral analysis outside physics and chemistry has gained popularity in such sciences as epidemiology, demography, and economics. In addition, the widespread use of computer languages for statistical data analysis has also contributed to the wider use of the named method in data analysis. Leaving aside the strictly econometric literature and resorting to a broader point of view, we can name the following works as introductory to the methodology: Harrington and Rubold (Citation2018), Jones (Citation2018), and Amazonaws (Citation2023).
Part of the work used in the preparation of the present research is indirectly related to the application of spectral analysis to the study of the evolution of gross regional product and gross domestic product. This is a business cycle study on a variety of country data (Altman et al., Citation2019; Hara et al., Citation2012). The relevance of this approach to our study lies in the fact that recurring cyclic patterns in regional development can be identified through spectral analysis.
There is a growing body of literature that recognizes the cyclical development. Fernández-Villaverde and Guerrón-Quintana (Citation2020) contain an extensive review of the literature on studies of uncertainty shocks and business cycles. We can mention a few papers beginning with a study of dynamic correlation between monetary policy cycles, the financial cycle, and the business cycle in China (Li et al., Citation2021; Liu et al., Citation2020), in India (Saini et al., Citation2021); a research on decomposition of stochastic trends in business cycles (Yang, Citation2020); a study of American business cycles in 1889–1913 (Jiang & Weder, Citation2021); a Bayesian analysis of Taiwanese business cycle fluctuations as part of the dynamic stochastic general equilibrium model (DSGE model) (Lin, Citation2021), as well as for the business cycles of developing countries (Notz & Rosenkranz, Citation2021). Adarov (Citation2021) applied the Bayesian approach with more data from 24 countries for the 1998–2012 period. Another example of the application of Bayesian statistics revealed both a global business cycle and four regional ones. European, Asian, North American, and Latin American (Berger et al., Citation2021).
An interesting development of the DSGE model in countercyclicality studies is the model, the key element of which is the ‘home information bias’ (HIB) as opposed to the ‘full information rational expectations’ (FIRE) (Iliopulos et al., Citation2021). The attempt to revise the business cycle model based on the topological entropy approach and the ‘cubic nonlinear investment function’ undertaken by Muñoz-Guillermo (Citation2021) deserves close attention. Research on cyclical development has gained prominence in the last two years since the outbreak of the COVID-19 pandemic. Considering the hypothesis of the downward trend of the actual economic cycle, it is appropriate to review the consequences of the coronavirus pandemic and the resulting cyclical constraints. Empirical research into the health effects of economic cycles has been carried out by Chai et al. (Citation2021), Liu and Huang (Citation2020), and Pu et al. (Citation2021); Cavicchioli and Pistoresi (Citation2020) have investigated the correlation between economic fluctuations and mortality in Italy.
Previously we tested the hypothesis of the presence of cyclical development (Kitchin cycles, Juglar cycles) in the regional economy of the Bryansk region (Dubovik & Dmitriev, Citation2022). For this purpose, we conducted a spectral analysis of the gross regional product and the output of the regional economy branches, as well as a trend analysis of the regional economy branches that have a statistically significant correlation with the gross regional product. Promising and worthy of further development is the methodology used by Rostan and Rostan (Citation2019), who applied the capabilities of spectral analysis to forecast the dynamics of nominal and real gross domestic product of Spain and the Eurozone countries until 2026, which allowed the named authors to present a less optimistic vision compared to the official point of view.
The hypothesis is that the use of spectral analysis to study gross regional product is more effective than conventional statistical methods. This will allow identifying periodic fluctuations of this indicator, and therefore the underlying stable patterns of development of regional economies, to conduct a more meaningful counter-cyclical policy and synchronize business cycles inherent in regional, and federal development. In addition, the planned study is the first attempt to apply spectral analysis to study the dynamics of indicators characterizing the economic development of Russia at the meso-level. Spectral analysis of the likely cyclical development of regions is useful since it can make it possible to synchronize business cycles of regions, and, therefore, to develop a more effective economic policy, for example, in price and tax aspects.
3. Methods and materials
3.1. Data
Data for this study were collected using the Russian Federation Federal State Statistics Service (FSSS) website (Federal State Statistics Service of Russian Federation, Citation2021). We used a comparison method (indicators of the gross regional product of the constituent entities of the Russian Federation that are part of the Central Federal District) over time annually (from 1998 to 2019), statistics methods, and econometrics. GRP, as opposed to the GDP, is calculated in basic prices, i.e., it includes subsidies for products and does not include taxes on products; does not take into account the activities of financial intermediaries; partially takes into account the added value created by the public administration.
The Central Federal District of Russia is an administrative entity established in 2000 on the territory of the historical center of the country. Its area is 650,205 km2 (3.8% of the territory of Russia), and the population of the district is 40,334,532 (27.4% of the total population of Russia). The CFD has the highest population density in the country (62.03 inhabitants/km2) and the urban population is 80.46 per cent (Kim, Citation2021). The district consists of small but densely populated regions. These indicators, the absence in the district of significant quasi-private latifundia and enterprises that parasitize the seeking of natural resource rent from hydrocarbon extraction, as well as the homogeneity of the development of its constituent regions, excluding significant statistical outliers, make the district a suitable subject for analysis of regional development of Russia.
3.2. Method of data analysis
We calculated and interpreted four central standardized moments, as well as other indicators (SE (Standard Error), Median (median is a measure of central tendency), MAD (Mean Absolute Deviation). GRP graphs, diagrams, and histograms have been constructed to show the indicators distribution of the analyzed indicators among the subjects (CFD regions) from 1998 to 2019. The model of spectral analysis of gross regional product proposed by the authors is applied to the dynamics of the above-mentioned indicator in 14 regions of the Central Federal District of Russia for 1998–2019.
Spectral analysis can be presented as a multiple regression problem, where the dependent variable observed in the series is the gross regional product, and the independent variables are the discrete frequency cosine function. The analysis of spectral density was conducted using Global Radiation Perturbation (GRP) data, with a particular focus on spectral graphs. These graphs visually represent the distribution of spectral power across different frequency components in the GRP data. The GRP data used in this analysis is sourced from the Center for Hydrometeorology (CFD) in Russia.
The spectral power density of each frequency component in the GRP data is displayed on the vertical axis (Y-axis) in the spectral graphs, while the corresponding frequencies are represented on the horizontal axis (X-axis). These graphs are generated using the Fast Fourier Transform (FFT) method, which decomposes the GRP data into its constituent frequency components. Higher values on the vertical axis (Y-axis) indicate greater spectral power at the corresponding frequencies. This spectral analysis provides valuable information about the distribution of energy across different frequency components in the GRP data.
The frequency (f) of a sine or cosine function is the number of cycles per unit of time (T) that is defined as the duration of the time interval required for a single complete cycle.
Suppose that we have a time series of GRP xt of length n (let n be an even number). We can represent a time series as a regression model xt, where n – 1 is the predicted variable (EquationEq. 1(1)
(1) ).
(1)
(1)
π (pi) is a mathematical constant, equal to 3.14,
t is the time variable.
We represent the coefficients of the regression that are determined a1, b1, … an/2-1, bn/2-1, an/2, respectively, we can write xt as (EquationEq. 2(2)
(2) ).
(2)
(2)
The variable "k" represents an integer index used in summation to incorporate terms with various frequencies and their corresponding coefficients into the model for x_t.
The values of cosine (ak) and sine (bk) represent the level at which the corresponding functions are correlated with the data of the time series, in our case the gross regional product. The represented regression model is a discrete Fourier transform (‘Discrete Fourier transform’) for time series. The Fourier transformation for time series yt for cycle frequency p in observations of n can be represented as (EquationEq. 3(3)
(3) ).
(3)
(3)
where p = 0, … n – 1,
zp is the Fourier coefficient associated with the frequency p.
We tested the time series for stationarity using the Augmented Dickey-Fuller test, checking the value of coefficient α in the first-order autoregressive Equationequation (4)(4)
(4) :
(4)
(4)
where yt is a time series, a εt is an error term. If α = 1, then the process has a unit root, so yt is not stationary. If |α| < 1, then yt is stationary.
Since the process can be a higher-order autoregression add lags of the first differences and obtain the following test model Equationequation (5)(5)
(5) :
(5)
(5)
If the resulting time series has a one-unit root, then the first differences are stationary by definition.
demonstrates the refinement of equations and the inclusion of citations to justify the selection of variables.
Table 1. Justifications for variable selection.
These variables and analysis methods were chosen to study the time series of Gross Regional Product (GRP) in the Central Federal District of Russia to assess the variability, central tendency, and periodic fluctuations in GRP data. The selection of these variables and methods is based on statistical and econometric approaches, as well as an analysis of long-term changes in economic activity. Before performing the Augmented Dickey-Fuller test, we condense our data by removing the trend from it and then re-center the time series by giving it a mean of zero. The test outputs a p-value: if p < 0.05, the time series probably returns to the mean (i.e., it is stationary), whereas p > 0.05 provides no such evidence because we cannot reject the null hypothesis. When a time series returns to the mean, it tends to return to its long-run mean. If the time series is not returning to the mean, it may shift without ever returning to the mean.
In addition, a correlation analysis was conducted among the annual GDP means.
Analyzes were conducted using the R Statistical language (version 4.2.2; R Core Team, Citation2022) on Windows 10 x64 (build 18363) (Appendix 1).
4. Results
It was not our intention to provide a detailed analysis of the differences in the gross regional product of the CFD, so they are omitted from our study. The results of the exploratory data analysis of the gross regional product of 14 regions of the Central Federal District are shown in and .
Table 2. The descriptive statistics of the gross domestic product of the Central Federal District, current prices, mln $ USA.
Table 3. The results of an exploratory data analysis of the gross regional product of the Central Federal District (current prices, million dollars USA).
All values of a symmetrical distribution are positive, showing that the mean is shifted to the right compared to the median. The angle of inclination of the GRP supports this conclusion. However, in 2009, the skewness coefficient took a negative value (−0.05), indicating a slight leftward skew, but it is not a strong deviation. After 2009, the skewness coefficient values remained negative, also suggesting a leftward skew in the distribution of the annual GDP. This means that the mean GDP is typically less than the median value. It implies that the average annual GDP becomes less than the median value after 2009.
All kurtosis values are negative, which means the ‘flat’ distribution of the GRP values of the selected regions in the analyzed period (thin tails and lower peak).
According to the data in , the CFD regions can be divided as
4 quartile (25% with the highest GRP): Tula, Yaroslavl (1998–2019), Tver (1998–2009), and Lipetsk (1999–2019).
2–3 quartiles: Vladimir (1998–2009, 2014, 2017–2019), Kursk (1998–2011, 2014–2019).
1 quartile (25% with the lowest GRP): Ivanovo, Kostroma, Orel, Bryansk (1998–2019), Kaluga (1998–2001, 2003–2007), Ryazan (1999–2000, 2008–2011, 2015–2019), Smolensk (1998, 2001–2005), 2008, 2010–2011, 2013), Tambov (1999, 2001–2004, 2006–2007, 2009, 2021–2013, 2015–2017, 2019).
Throughout the observed period, the gross regional product of the Bryansk region is below the median value.
We deliberately did not calculate the growth rate of the GRP of these areas, since the visual analysis of the graphs shown in indicates fairly close values of this indicator.
Figure 1. Gross regional product of the regions of the Central Federal District, current prices, mln $ USA.
Source: Own representation based on data from the Federal State Statistics Service of the Russian Federation (2021).

Figure 2. Gross regional product of the Central Federal District without Moscow and the Moscow region, current prices, mln $ USA.
Source: Own representation based on data from the Federal State Statistics Service of the Russian Federation (Citation2021).

Figure 3. Gross regional product of the Central Federal District without the Voronezh and Belgorod regions, current prices, mln $ USA.
Source: Own representation based on data from the Federal State Statistics Service of the Russian Federation (Citation2021).

We have analyzed the dynamics of the gross regional product of the constituent entities of the Russian Federation comprising the Central Federal District ().
illustrates significant differences in the rates of GDP growth among the subjects of the Central Federal District. Particularly, Moscow and the Moscow region exhibit substantially higher growth rates compared to other regions within the district. This disparity signifies distinct economic dynamics and performance between these subjects and other regions of the Central Federal District. This trend also underscores the economic significance and resilience of Moscow and its surrounding region compared to their counterparts.
Considering the pronounced disparity in growth rates and the potential distorting impact of Moscow and the Moscow region on the overall analysis of the Central Federal District (CFD), it is recommended to exclude these entities from further investigation. This would allow focus on a more detailed examination of the dynamics of the remaining regions within the district and provide a clearer understanding of the overall trends in regional development in the CFD.
After excluding Moscow and the Moscow region from the analysis, presents the adjusted dynamics of GDP in the CFD. This corrected depiction offers insights into the economic activities of the remaining regions within the district, offering a more balanced perspective on the general trends in regional development in the CFD.
By the next iteration, we will exclude two other federated entities that are members of the CFD, whose growth rate differs significantly from the others, the Voronezh and Belgorod regions. It should be noted that differences in the growth rates of these regions and the rest of the CFD areas began immediately after the Great Recession of 2008–2009. Increasing over the following periods ().
Further analysis was performed for the 14 regions of the Central Federal District, although the box plot () provides an idea of the existence of some variation in the gross regional product of the study areas.
Figure 4. A box plot of the GRP of regions of the Central Federal District.
Source: Own representation based on data from the Federal State Statistics Service of the Russian Federation (Citation2021).

Despite the generally positive trend in GRP, most regions remain within the same quartile (with minor variations). Considering that this period (1998–2019) covers almost the entire period of the establishment of the market economy of Russia, we consider it possible to establish both the existence of the ‘path dependence’ and the ‘middle-income trap’. In the latter case, it is more the ‘low-income trap’ that should be mentioned. Analysis of the difference between ‘path dependence’ and ‘path determinacy’ (North, Citation1990; Tool, Citation1994) is beyond the scope of this research. We have referred to this problem in our earlier studies.
We represented the frequency of the gross regional product as a histogram ().
The data in the histogram, the preliminary descriptive analysis, as well as the Shapiro-Wilk test, lead us to the conclusion that the distribution of growth in the gross regional product of the regions of the Central Federal District cannot be regarded with the necessary degree of confidence as normal; the usual statistical methods do not fit efficiently enough.
In this regard, we believe that a study of the evolution of the gross regional product should be carried out as part of a spectral analysis that reveals the existence/absence of cyclical trends in this indicator. This requires a decomposition of the time series characterizing changes in GRP on the functions of sine and cosine of different frequencies to determine this frequency as well as its influence on the dynamics of GRP.
Graphs (‘periodograms’) representing the preliminary results of the spectral analysis of the GRP of 14 regions of the Central Federal District are shown in .
and provide the results of a spectral analysis of the GRP of 14 regions of the Central Federal District and the GDP of Russia over the year: there is a certain cyclicality present but not sufficiently expressed.
Figure 7. Periodogram of the GRP of the CFD in 1998–2019 according to annual data.
Source: Own representation.

Figure 8. Periodogram of the GDP of Russia in 1998–2019 according to annual data.
Source: Own representation.

The obtained results for the 14 regions of the Central Federal District indicate a certain degree of similarity in the dynamics of gross regional product across the investigated territories. Specifically, there is a tendency towards an increase in GDP, which may indicate a generally positive trend in economic development across the regions of the Central Federal District.
presents the results of the spectral analysis of the GDP of Russia for 1998–2019, according to quarter data. Cyclical dynamics are more visible. These graphs illustrate the utilization of spectral analysis for studying the cyclical dynamics of economic indicators. They enable the identification of cycles and their frequencies, which aids in understanding the nature of economic processes and in developing corresponding management strategies.
Figure 9. Periodogram of Russian GDP in 1998–2019 according to quarter data.
Source: Own representation.

Spectrum plots (“Periodograms”, ) refer to a graphical representation of the frequency spectrum of a gross regional product of regions of the Central Federal District, Russia. They visualize the distribution of frequencies present in data.
Spectrum plots () display the power spectral density of each frequency component of the GRP on the y-axis, while the corresponding frequencies are shown on the x-axis. The plots are generated using Fast Fourier Transform (FFT), which decomposes the GRP in the CFD, Russia into its constituent frequency components. Higher values on the y-axis indicate stronger spectral power at corresponding frequencies. The spectrum plots help in identifying the dominant frequencies and their relative strengths in the GRP in the CFD, Russia. It can provide insights into the periodicity, harmonics, and overall frequency content of the GRP, which can be useful in the detection of the cyclical patterns of the gross regional product.
The conducted correlation analysis of GRP over the years is presented in .
Therefore, the Pearson correlation coefficient is approximately 0.9982, indicating a positive linear relationship, meaning that the Gross Regional Product (GRP) increases over the years.
In the present study, we found that the analyzed time series, reflecting the dynamics of the gross regional product of the Central Federal District of Russia are non-stationary.
We suggest that it is appropriate to use data for shorter periods, such as quarters.
The results of this study can be utilized in the following aspects:
Formulation of an effective national strategy: Understanding and analyzing the cyclical patterns in regional development at micro and macro levels can aid in the formulation of national development strategies. Policymakers can use this data to design programs aimed at enhancing the stability and growth of regional economies.
Optimization of investment decisions: The research findings can be employed by economists and financial analysts for the rational allocation of investment resources. They can determine the optimal timing and regions for investment to maximize returns and minimize risks.
Development of regional growth strategies: Local authorities and regional governments can utilize this data to formulate specific development strategies for their regions. This may involve measures to stimulate entrepreneurship, and infrastructure development, and ensure sustainable growth.
Risk management: Understanding the cyclical processes in regional development allows for the development of risk management strategies. Policymakers and business leaders can make informed decisions, preempting potential downturns in regional economies.
Modeling future scenarios: Analysis of cyclical patterns can be employed to develop models for future development scenarios.
The findings of the study make a significant novel contribution to the field of regional economic development, particularly in the application of spectral analysis to identify cyclical patterns in the Gross Regional Product (GRP) within the Central Federal District of Russia. These results offer new opportunities for understanding the periodicity, coherence, and overall frequency content of GRP, which can be valuable in identifying cyclical regularities in regional economies.
Compared to previous studies, which typically confine themselves to the analysis of statistical data alone, this work employs spectral analysis for a deeper exploration of cyclical processes. The utilization of spectral analysis in this context represents an innovative approach, allowing for additional insights into the structure and dynamics of GRP in the regions.
The conclusions drawn carry significant implications for various policy and practical aspects, particularly for the development of national development strategies, optimization of investment decisions, formulation of regional growth strategies, risk management, and forecasting of future development scenarios. These results may prove beneficial for economists, policymakers, and governmental bodies in addressing issues of regional development and formulating national strategies.
Hence, this study can be considered a valuable instrument for policymakers, economists, and governmental bodies when dealing with matters about regional development and the formulation of national strategies. Jawadi et al. (Citation2022) used quarter data for two indicators measuring financial and business cycles; Iyetomi et al. (Citation2020) also used quarter data from the USA. We have encountered a limitation in the present research in the statistical base for analysis. However, we believe that the proposed model of spectral time series analysis of the gross regional product could be further developed and refined in our next work. Further research is needed to compare the GDP and GRP of the Russian regions according to quarter data. Due to the lack of the quarter data provided by the Federal State Statistics Service of the Russian Federation (2021), this study cannot provide a comprehensive review of such a comparison, which is a limitation of the study.
5. Discussions
In complex social systems, macro-level structures and patterns are stable; but they are not explicit at the micro level. Accordingly, the micro-macro dichotomy “skips” the meso level, which connects the activities and interactions of various actors. Such structures and patterns include, for example, the cyclical development of the economy, which finds itself at the level of the country’s economy, but the presence of cycles at the meso level is a debatable phenomenon, and, therefore, its diagnosis is among the topical and promising research tasks. Our view is that it is necessary to supplement the traditional methods of studying economic dynamics with other, inter alia, spectral analysis of the gross regional product, i.e., meso-level analysis. The graphs in do not provide an exhaustive representation of the spectral variations within our model.
Our previous study did not find a significant correlation between innovation activity in one region and growth in the corresponding GRP (Chomen, Citation2022), while in OECD countries cyclical dynamics in RandD activity, innovation, and patent activity correlation with the GDP dynamics (Ahmad, Citation2021; Zhang & Ye, Citation2022). Attempts to find a correlation between global GDP growth and patent activity through spectral analysis (Monakhov & Chechurin, Citation2012; Zhang & Ye, Citation2022) have not been successful; on the other hand, Korotayev and Tsirel (Citation2010) have been able to detect ‘Kondratieff waves’ using spectral analysis of the world GDP (Leal & Marques, Citation2022).
Spectral analysis of the likely cyclical development of the regions is useful because it may make possible the synchronization of business cycles (and the graphs above indicate their discontinuity) of the regions, and hence the formulation of more efficient economic policies, for example, in price and tax terms (Angeletos et al., Citation2020). Price mechanisms have limited potential for stabilizing economies through countercyclical policies; the interaction between price and investment mechanisms for adjusting to cyclicality can generate fluctuations and cycles (Gao & Gong, Citation2020). A similar problem is faced by the economic authorities of a united Europe (Belke et al., Citation2017), especially as monetary policy instruments increase cyclicality but have asymmetric effects that are more visible in developing countries (Hang & Xue, Citation2020). By contrast, cyclical side effects are distributed symmetrically, and business cycles in European countries have become more synchronized since the Great Recession (Arčabić & Škrinjarić, Citation2021).
Equally important in the pursuit of present research is the construction of a predictive model of the time series of the gross regional product. Typical forecasting methods (ARIMA models as an example) are built on assumptions such as the stationarity of time series and the normality of their residuals. As we mentioned above distributional assumptions of the GRP are beyond normality. Spectral analysis that does not depend on stationary and non-linear assumptions could be very useful for modeling and forecasting economic data.
For Keynes-based economic theory, it is traditional to use macro- and micro-levels as the basic categories of the hierarchical structure of economics, reflecting the hierarchical nature of the evolutionary process. The designation of the meso-level as a stand-alone study remains controversial in economic science. We believe that the difference between the cyclical development of the state and the individual region is a convincing argument in favor of the need to abandon the binary structure of the economy and the transition to the trinary logic of research: macro–meso–micro levels. The discrepancy between economic dynamics (gross domestic product and gross regional product) allows us to assert that the meso-level is the key epistemic construct linking macro- and micro-level research; for the Russian economy, it is the level of the region.
The discontinuity we have observed in the cyclical development of the analyzed regions is also interesting in that the establishment of the common European currency has led to a significant increase in the synchronization of European countries, even in countries where the euro was not immediately introduced (Campos et al., Citation2019), and Russian regions, even in the single economic space, do not display such synchrony. Blinova et al. (Citation2021) revealed a cyclical reaction in unemployment rates in the Russian regions. Almeida et al. (Citation2020) performed a similar study on regional unemployment and the sensitivity of the economy to cyclic dynamics in Spain; Hutter (Citation2021) identified cyclical seasonal patterns in seeking employment in Germany. Thus, the identification of cyclic dynamics in Russian data is an important scientific task.
Unfortunately, we are not aware of other studies of the dynamics of the gross regional product of the Central Federal District using the spectral analysis and Fast Fourier transformation method. In this connection, we were obliged to make a comparison with other studies performed on other data, which makes it impossible to draw conclusions about the adequacy of the method chosen and the correctness of the results obtained. We posit that the discrepancy in the cyclical dynamics of the Russian economy as a whole and the regions of the Central Federal District, in particular, can be attributed to the peculiarities of Russia’s development in the early pre-industrial era compared to Europe in the same period.
The authors argue that the spread of agriculture in Russia is explained by diffusion theory, within which it was not the idea of agriculture that was spread, but rather the farmers themselves. Considering the relatively homogeneous farming environment, as well as the limited variety of crops and significant distances between settlements, the economic space of the country was sufficiently disjointed. This resulted in weak specialization of regions and, consequently, slow development of craft and domestic trade, as well as delayed urban growth compared to Europe. The significant distances between settlements in the country also had a negative effect. These factors are what is referred to as “path-dependence” in the framework of the new institutional economic theory, which determined the topological disjointedness of the country’s territory, confirmed by the “Global Connectedness Index” (Altman et al., Citation2019). In these conditions, the “spatial competition” for consumers, which led to the Industrial Revolution and economic growth in Britain but not in China (Desmet et al., Citation2017, pp. 7–16), as well as the “market for ideas”, which enables the emergence and implementation of innovations (Mokyr, Citation2007), the spread of knowledge and trade (Tisdell & Svizzero, Citation2015, pp. 18–19), the formation of human capital, and subsequently, endogenous economic development (Romer, Citation1990, Citation2015), are extremely challenging. Modern satellite images of nighttime illumination of the surface of Russia (Garner, Citation2017) compared to similar images of other countries (Adhikari & Dhital, Citation2021; Mendez & Santos‐Marquez, Citation2021; Qiao-Li et al., Citation2021).
In general, the vast majority of the regions studied did not change their development trajectory during the entire period under review. We assume that there is clear evidence of the existence of a ‘track effect’ resulting from the previous historical experience of the regions. This confirms our original hypothesis about ‘the low-income trap. According to Taymaz (Citation2022) a region in “Convergence Club 1” had, on average, 8 times higher GDP per capita than a region in “Convergence Club 4” in 2017. Income differences across Russian regions are quite persistent, and low-income regions are not able to improve their relative positions (Taymaz, Citation2022, p. 481; Windle et al., Citation2023).
We found that among all the industries only the hotel and restaurant business have the same trend break point with GRP in 2013. In the named industry there is one more breakpoint of the trend in 2015; the trend of the gross regional product in the mentioned period does not have a breakpoint. We believe that the results of the analysis do not confirm the initial hypothesis about the existence of Kitchin cycles, and Juglar cycles in the economy of the Bryansk region in the observed period.
A high correlation of business cycles is usually seen as a key criterion for an optimal currency area, for example, the European Union. The results of the spectral analysis obtained by the authors do not give a clear answer about the existence of sustainable cycles in meso-level development; nevertheless, they (results) can serve as a basis for further in-depth study of the subject of the study, and improve the proposed model of spectral analysis of the gross regional product. We find it promising to apply the theory of the economic cycle of meso-level in the interpretation of the causes of the disproportional regional development in Russia.
6. Conclusions
It has been established that the vast majority (10 out of 14) of these regions remain in the lowest quartile of GRP throughout the analysis period; 2 regions are invariably in the quartile with the highest GRP. It is possible to state with confidence the existence of a ‘path dependence’ predetermined by the historically established positions (and specialization) of regions in the Russian value chain, as well as the existence of a ‘low-income trap’ that the regions of the first quartile have not been able to overcome, despite the constant upward trend in their gross regional product.
Our analysis has shown that the distribution of growth in the gross regional product is not statistically normal and therefore it is necessary to complement traditional narrative statistics with other methods. The results of our spectral analysis confirmed our hypothesis about the usefulness of this method. An introduced model for spectral analysis of GRP treats it as a specific case of the regression model using discrete Fourier transformation.
Based on the conducted research, recommendations for policymakers can be delineated. Considering the persistent trend of low levels of regional product in certain regions juxtaposed with consistently high levels in others, we recommend the development of a national strategy encompassing specialized programs to support and foster the regions in the lowest quartile of regional product. Policymakers should actively incentivize investments in regions with low levels of regional product, directing financial and other resources to bolster economic growth and infrastructural development. It is crucial to consider measures supporting entrepreneurship and creating favorable business conditions in regions with low levels of regional product, which will aid in stimulating economic development. There is a necessity to develop programs and projects aimed at modernizing and diversifying the economies of regions, thereby reducing their dependence on specific sectors and markets. Policymakers should actively develop and implement risk management strategies to prevent potential downturns in regional economies and ensure stable development.
One of the primary constraints of this study is the absence of quarterly data for comparing the GDP and GRP of Russian regions. Having access to these data is crucial, as they can provide a more detailed and dynamic representation of economic processes at the regional level. The prospect for future research lies in refining the spectral analysis method for a more precise and reliable analysis of GRP dynamics at the regional level.
Disclosure statement
The authors report there are no competing interests to declare.
Data availability statement
Data will be available on request.
Additional information
Notes on contributors
Mayya Dubovik
Mayya Dubovik Ph.D. in Economics, Associate Professor, Professor of the Department of Economic Theory, Plekhanov Russian University of Economics, Moscow, Russia. Her research interests include economic theory, economic methodology, property and cash inequality of states and regions, sustainable development, technological progress and innovation, modern economic growth. She published books on Macroeconomics, Digital economics etc. Her works have been published in such journals as Creative Economics, Vestnik of Plekhanov Russian University of Economics, Financial Management, Drucker’s Bulletin and others.
Sergey Dmitriev
Sergey Dmitriev Ph.D. in Economics, Researcher, Associate Professor at the Department of Economics, Customs, Information Technology and Natural Science Disciplines of the Bryansk Branch of the Plekhanov Russian University of Economics, Russia, Bryansk. His research interests include convergent and divergent processes of regional economic development, inequality between countries and regions, spectral analysis in economics, problems of methodology of economic science. He has published a book on the theory of innovative development from a Schumpeterian economic growth perspective. His works have been published in such journals as Creative Economics, Issues of Innovative Economics, Vestnik of Plekhanov Russian University of Economics and others.
Natalya Obidovskaya
Natalya Obidovskaya Ph.D. in Economics, Head of the Department of Civil, Criminal Law and Procedure and Financial and Legal Disciplines, Russia, Bryansk. Her research interests include tax and customs regulation, legal regulation of entrepreneurial activity and digital economy. The results of her research have been published in scientific journals such as Kant, Innovation and Investment, Financial Management and others.
Gulchexra Khalmatjanova
Gulchexra Khalmatjanova Ph.D. in Economics, Head of the Department of World and Regional Economics, Ferghana State University, Uzbekistan, Ferghana. Her research interests include regional economics, agrarian sector development, investment policy, and economic growth. Her works have been published in such journals as Academicia: an international multidisciplinary research journal, Scientific progress, Problems of Economics, Education and Science in Russia and abroad and others.
References
- Adarov, A. (2021). Dynamic interactions between financial cycles, business cycles and macroeconomic imbalances: A panel VAR analysis. International Review of Economics & Finance, 74, 434–451. https://doi.org/10.1016/j.iref.2021.03.021
- Adhikari, B., & Dhital, S. (2021). Decentralization and regional convergence: Evidence from night-time lights data. Economic Inquiry, 59(3), 1066–1088. https://doi.org/10.1111/ecin.12967
- Ahmad, M. (2021). Non-linear dynamics of innovation activities over the business cycles: Empirical evidence from OECD economies. Technology in Society, 67, 101721. https://doi.org/10.1016/j.techsoc.2021.101721
- Almeida, A., Galiano, A., Golpe, A. A., & Martín, J. M. (2020). Regional unemployment and cyclical sensitivity in Spain. Letters in Spatial and Resource Sciences, 13(2), 187–199. https://doi.org/10.1007/s12076-020-00252-3
- Altman, S. A., Ghemawat, P., & Bastian, P. (2019). DHL Global Connectedness Index 2018. The state of globalization in a fragile world. Dhl.com. https://www.dhl.com/content/dam/dhl/global/core/documents/pdf/glo-core-gci-2018-full-study.pdf
- Amazonaws. (2023). Spectral analysis of time series. https://rstudio-pubs-static.s3.amazonaws.com/9428_1197bd003ebd43c49b429f22ea4f36e5.html
- Angeletos, G. M., Iovino, L., & La’O, J. (2020). Learning over the business cycle: Policy implications. Journal of Economic Theory, 190, 105115. https://doi.org/10.1016/j.jet.2020.105115
- Angerer, P., Kluyver, T., & Schulz, J. (2023). Repr: Serializable representations. R package version 1.1.6. https://CRAN.R-project.org/package=repr
- Arčabić, V., & Škrinjarić, T. (2021). Sharing is caring: Spillovers and synchronization of business cycles in the European Union. Economic Modelling, 96, 25–39. https://doi.org/10.1016/j.econmod.2020.12.023
- Atesoglu, H. S., & Vilasuso, J. (1999). A band spectral analysis of exports and economic growth in the United States. Review of International Economics, 7(1), 140–152. https://doi.org/10.1111/1467-9396.00152
- Azzalini, A., & Genz, A. (2022). The R package\texttt{mnormt}: The multivariate normal and $t$ distributions (version 2.1.1). http://azzalini.stat.unipd.it/SW/Pkg-mnormt/
- Bache, S., & Wickham, H. (2022). Magrittr: A forward-pipe operator for R. R package version 2.0.3. https://CRAN.R-project.org/package=magrittr
- Batorova, I. (2012). Spectral techniques for economic time series. Unpublish dissertation thesis, Comenius University.
- Belke, A., Domnick, C., & Gros, D. (2017). Business cycle desynchronisation. VOX, CEPR Policy Portal. https://voxeu.org/article/business-cycle-desynchronisation
- Ben-Shachar, M. S., Lüdecke, D., & Makowski, D. (2020). Effectsize: Estimation of effect size indices and standardized parameters. Journal of Open Source Software, 5(56), 2815. https://doi.org/10.21105/joss.02815
- Berger, T., Everaert, G., & Pozzi, L. (2021). Testing for international business cycles: A multilevel factor model with stochastic factor selection. Journal of Economic Dynamics and Control, 128, 104134. https://doi.org/10.1016/j.jedc.2021.104134
- Blinova, T. V., Rusanovskii, V. A., & Markov, V. A. (2021). Estimating the impact of economic fluctuations on unemployment in Russian regions based on the Okun model. Studies on Russian Economic Development, 32(1), 103–110. https://doi.org/10.1134/S1075700721010032
- Bukharbayeva, A. Z., Nauryzbayev, A. Z., Jrauova, K. S., Oralbayeva, K., & Aimagambetova, A. D. (2020). Formation of the transport and logistics system as the basis for rice production development in the Kazakhstan Aral Sea region in the context of the EAEU economic integration. Academy of Strategic Management Journal, 19(3), 1–7.
- Burakov, N. A., Bukhvald, E. M., Kolchugina, A. V., Rubinstein, A. Y., Slavinskaya, O. A., & Slutskin, L. N. (2019). Regional Index of economic development and ranking of the subjects of the Russian Federation. Institute of Economics.
- Campos, N. F., Fidrmuc, J., & Korhonen, I. (2019). Business cycle synchronisation and currency unions: A review of the econometric evidence using meta-analysis. International Review of Financial Analysis, 61, 274–283. https://doi.org/10.1016/j.irfa.2018.11.012
- Cavicchioli, M., & Pistoresi, B. (2020). Unfolding the relationship between mortality, economic fluctuations, and health in Italy. The European Journal of Health Economics: HEPAC: health Economics in Prevention and Care, 21(3), 351–362. https://doi.org/10.1007/s10198-019-01135-1
- Chai, K. C., Li, Q., Bao, X. L., Zhu, J., & He, X. X. (2021). An empirical study of economic cycle, air quality, and national health since reform and opening up. Frontiers in Public Health, 9, 706955. https://doi.org/10.3389/fpubh.2021.706955
- Chomen, M T. (2022). Institutions–economic growth nexus in Sub-Saharan Africa. Heliyon, 8(12), e12251. https://doi.org/10.1016/j.heliyon.2022.e12251
- Correia, S., Luck, S., & Verner, E. (2020). Pandemics depress the economy, public health interventions do not: Evidence from the 1918 flu. SSRN Electronic Journal, https://papers.ssrn.com/sol3/papers.cfm?abstract_id=3561560 https://doi.org/10.2139/ssrn.3561560
- Croux, C., Dekimpe, M. G., & Lemmens, A. (2005). The European consumer: United in diversity? ERIM Report Series Reference No. ERS-2005-022-MKT.
- Croux, C., Dekimpe, M. G., & Lemmens, A. (2008). Measuring and testing granger causality over the spectrum: An application to European production expectation surveys. International Journal of Forecasting, 24(3), 414–431. https://doi.org/10.1016/j.ijforecast.2008.03.004
- Croux, C., Forni, M., & Reichlin, L. (1999). A measure of comovement for economic variables: Theory and empirics. CEPR Discussion paper No. 2339.
- Csárdi, G. (2019). Pkgconfig: Private configuration for 'R' packages. R package version 2.0.3. https://CRAN.R-project.org/package=pkgconfig
- Desmet, K., Greif, A., & Parente, S. L. (2017). Spatial competition, innovation and institutions: The industrial revolution and the great divergence. SSSN. https://papers.ssrn.com/sol3/papers.cfm?abstract_id=2927147
- Dowle, M., & Srinivasan, A. (2023). Data.table: Extension of ′data.framè. R package version 1.14.8. https://CRAN.R-project.org/package=data.table
- Dubovik, M. V., & Dmitriev, S. G. (2022). Application of spectral analysis to diagnose cyclical economic development. Drukerovskij Vestnik, 2(2), 229–242. https://doi.org/10.17213/2312-6469-2022-2-229-242
- Economist. (2018). Economists still lack a proper understanding of business cycles. https://www.economist.com/finance-and-economics/2018/04/19/economists-still-lack-a-proper-understanding-of-business-cycles
- Federal State Statistics Service of Russian Federation. (2021). National accounts. Gross Regional Product. https://eng.rosstat.gov.ru/folder/13913
- Fernández-Villaverde, J., & Guerrón-Quintana, P A. (2020). Uncertainty shocks and business cycle research. Review of Economic Dynamics, 37(S1), S118–S146. https://doi.org/10.1016/j.red.2020.06.005
- Gao, Y., & Gong, G. (2020). Stabilizing and destabilizing mechanisms: A new perspective to understand business cycles. Economic Modelling, 93, 51–68. https://doi.org/10.1016/j.econmod.2020.07.002
- Garner, R. (2017). New night lights maps open up possible real-time applications. NASA. https://www.nasa.gov/feature/goddard/2017/new-night-lights-maps-open-up-possible-real-time-applications
- Gaslam, B. (2023). Fansi: ANSI control sequence aware string functions. R package version 1.0.4. https://CRAN.R-project.org/package=fansi
- Granger, C. W. I. (1966). The typical spectral shape of an economic variable. Econometrica, 34(1), 150–161. https://doi.org/10.2307/1909859
- Granger, C. W. I. (1969). Investigating casual relations by econometric models and cross-spectral methods. Econometrica, 37(3), 424–438. https://doi.org/10.2307/1912791
- Granger, C. W. I., & Hatanak, M. (1964). Spectral analysis of economic time series. Princeton University Press.
- Grolemund, G., & Wickham, H. (2011). Dates and times made easy with lubridate. Journal of Statistical Software, 40(3), 1–25. https://doi.org/10.18637/jss.v040.i03
- Hang, Y., & Xue, W. (2020). The asymmetric effects of monetary policy on the business cycle: Evidence from the panel smoothed quantile regression model. Economics Letters, 195, 109450. https://doi.org/10.1016/j.econlet.2020.109450
- Hara, K., Uwasu, M., Kobayashi, H., Kurimoto, S., Yamanaka, S., Shimoda, Y., & Umeda, Y. (2012). Enhancing meso level research in sustainability science—challenges and research needs. Sustainability, 4(8), 1833–1847. https://doi.org/10.3390/su4081833
- Harrington, J., & Rubold, U. (2018). Spectral analysis. https://www.phonetik.uni-muenchen.de/∼jmh/lehre/sem/ws1819/emuR/LESSON5/SpectralAnalysis.html
- Harvey, A. C., & Jaeger, A. (1993). Detrending, stylized facts and the business cycle. Journal of Applied Econometrics, 8(3), 231–247. https://doi.org/10.1002/jae.3950080302
- Hughes-Hallett, A., & Richter, C. R. (2004). A time-frequency analysis of the coherences of the US business cycle and the European business cycle. Center for Economic Policy Research Discussion Paper No. 4751.
- Hughes-Hallett, A., & Richter, C. R. (2007). Are the new member states converging on the Eurozone? A business cycle analysis for economies in transition. OECD Journal: Journal of Business Cycle Measurement and Analysis, 2, 49–68. https://doi.org/10.1787/19952899
- Huntington-Klein, N. (2023). Vtable: Variable table for variable documentation. R package version 1.4.4. https://CRAN.R-project.org/package=vtable
- Hutter, C. (2021). Cyclicality of labour market search: A new big data approach. Journal for Labour Market Research, 55(1), 1–16. https://doi.org/10.1186/s12651-020-00283-9
- Iliopulos, E., Perego, E., & Sopraseuth, T. (2021). International business cycles: Information matters. Journal of Monetary Economics, 123, 19–34. https://doi.org/10.1016/j.jmoneco.2021.06.001
- Iyetomi, H., Aoyama, H., Fujiwara, Y., Souma, W., Vodenska, I., & Yoshikawa, H. (2020). Relationship between macroeconomic indicators and economic cycles in US. Scientific Reports, 10(1), 8420. https://doi.org/10.1038/s41598-020-65002-3
- Jacob, M. (2023). Kit: Data manipulation functions implemented in C. R package version 0.0.13. https://CRAN.R-project.org/package=kit
- Jawadi, F., Ameur, H. B., Bigou, S., & Flageollet, A. (2022). Does the real business cycle help forecast the financial cycle? Computational Economics, 60(4), 1529–1546. https://doi.org/10.1007/s10614-021-10193-8
- Jiang, D., & Weder, M. (2021). American business cycles 1889-1913: An accounting approach. Journal of Macroeconomics, 67, 103285. https://doi.org/10.1016/j.jmacro.2020.103285
- Jones, J. H. (2018). Time series and spectral analysis. http://web.stanford.edu/class/earthsys214/notes/series.html
- Kapounek, S., & Pomenkova, J. (2010). Business cycle development in Czech and Slovak economies. Bulletin of the Transilvania University of Brasov, 3(52), 155–166.
- Kassambara, A. (2023). Ggpubr: 'ggplot2' based publication ready plots. R package version 0.6.0. https://CRAN.R-project.org/package=ggpubr
- Kim, D. (2021). Economies of scale and international business cycles. Journal of International Economics, 131, 103459. https://doi.org/10.1016/j.jinteco.2021.103459
- Korotayev, A. V., & Tsirel, S. V. (2010). A spectral analysis of world GDP dynamics: Kondratieff Waves, Kuznets Swings, Juglar and Kitchin Cycles in global economic development, and the 2008-2009 economic crisis. Structural Dynamics, 4(1), 3–32. https://doi.org/10.5070/SD941003306
- Krantz, S. (2023a). Collapse: Advanced and fast data transformation. R package version 196. https://CRAN.R-project.org/package=collapse
- Krantz, S. (2023b). Fastverse: A suite of high-performance packages for statistics and data manipulation. R package version 0.3.1. https://CRAN.R-roject.org/package=fastverse
- Leal, P. H., & Marques, A C. (2022). The evolution of the environmental Kuznets curve hypothesis assessment: A literature review under a critical analysis perspective. Heliyon, 8(11), e11521. https://doi.org/10.1016/j.heliyon.2022.e11521
- Li, X. L., Yan, J., & Wei, X. (2021). Dynamic connectedness among monetary policy cycle, financial cycle and business cycle in China. Economic Analysis and Policy, 69, 640–652. https://doi.org/10.1016/j.eap.2021.01.014
- Lilley, A., Lilley, M., & Rinaldi, G. (2020). Public health interventions and economic growth: Revisiting the Spanish flu evidence. SSRN Electronic Journal, https://papers.ssrn.com/sol3/papers.cfm?abstract_id=3590008 https://doi.org/10.2139/ssrn.3590008
- Lin, Y. C. (2021). Business cycle fluctuations in Taiwan – A Bayesian DSGE analysis. Journal of Macroeconomics, 70, 103349. https://doi.org/10.1016/j.jmacro.2021.103349
- Liu, D., Wang, Q., & Song, Y. (2020). China’s business cycles at the provincial level: National synchronization, interregional coordination and provincial idiosyncrasy. International Review of Economics & Finance, 69, 629–650. https://doi.org/10.1016/j.iref.2020.06.006
- Liu, Y. H., & Huang, W. H. (2020). Asymmetric effect of business cycles on population health: Evidence from the ASEAN Countries. Frontiers in Public Health, 8, 32. https://doi.org/10.3389/fpubh.2020.00032
- Lüdecke, D., Ben-Shachar, M. S., Patil, I., & Makowski, D. (2020). Extracting, computing and exploring the parameters of statistical models using R. Journal of Open Source Software, 5(53), 2445. https://doi.org/10.21105/joss.02445
- Lüdecke, D., Ben-Shachar, M. S., Patil, I., Waggoner, P., & Makowski, D. (2021). Performance: An R Package for assessment, comparison and testing of statistical models. Journal of Open Source Software, 6(60), 3139. https://doi.org/10.21105/joss.03139
- Lüdecke, D., Makowski, D., Ben-Shachar, M. S., Patil, I., Wiernik, B. M., Bacher, E., & Thériault, R. (2022). Easystats: Framework for easy statistical modeling, visualization, and reporting. CRAN. R package. https://easystats.github.io/easystats/
- Lüdecke, D., Patil, I., Ben-Shachar, M. S., Wiernik, B. M., Waggoner, P., & Makowski, D. (2021). See: An R Package for visualizing statistical models. Journal of Open Source Software, 6(64), 3393. https://doi.org/10.21105/joss.03393
- Lüdecke, D., Waggoner, P., & Makowski, D. (2019). Insight: A unified interface to access information from model objects in R. Journal of Open Source Software, 4(38), 1412. https://doi.org/10.21105/joss.01412
- Makowski, D., Ben-Shachar, M. S., & Lüdecke, D. (2019). BayestestR: Describing effects and their uncertainty, existence and significance within the Bayesian Framework. Journal of Open Source Software, 4(40), 1541. https://doi.org/10.21105/joss.01541
- Makowski, D., Ben-Shachar, M. S., Patil, I., & Lüdecke, D. (2020a). Estimation of model-based predictions, contrasts and means. CRAN. https://github.com/easystats/modelbased
- Makowski, D., Ben-Shachar, M. S., Patil, I., & Lüdecke, D. (2020b). Methods and algorithms for correlation analysis in R. Journal of Open Source Software, 5(51), 2306. https://doi.org/10.21105/joss.02306
- Makowski, D., Lüdecke, D., Patil, I., Thériault, R., Ben-Shachar, M. S., & Wiernik, B. M. (2023). Automated results reporting as a practical tool to improve reproducibility and methodological best practices adoption. CRAN. https://easystats.github.io/report/
- Makowski, D., Wiernik, B. M., Patil, I., Lüdecke, D., & Ben-Shachar, M. S. (2022). Correlation: Methods for correlation analysis. Version 0.8.3. https://CRAN.R-roject.org/package=correlation.
- Mankiw, G. N., Romer, D., & Weil, D. N. (1992). A contribution to the empirics of economic growth. The Quarterly Journal of Economics, 107(2), 407–437. https://doi.org/10.2307/2118477
- Maršálek, R., & Poměnková, J. (2010a). Spectral analysis of the cyclical behaving of the Czech Republic industrial production. In Forum Statisticum Slovacum 2/2010. Aula EU.
- Maršálek, R., & Poměnková, J. (2010b). Industrial production periodicity testing using R.A. Fisher Test. Acta Universitatis Agriculturae et Silviculturae Mendelianae Brunensis, 58(3), 189–196. https://doi.org/10.11118/actaun201058030189
- Maršálek, R., & Poměnková, J. (2011). Time and frequency domain in the business cycle structure MENDELU Working Papers in Business and Economics 7/2011.
- Mendez, C., & Santos‐Marquez, F. (2021). Regional convergence and spatial dependence across subnational regions of ASEAN: Evidence from Satellite Nighttime Light Data. Regional Science Policy & Practice, 13(6), 1750–1777. https://doi.org/10.1111/rsp3.12335
- Mokyr, J. (2007). The market for ideas and the origins of economic growth in eighteenth century in Europe. Tijdschr. Tijdschrift voor Sociale en Economische Geschiedenis/ The Low Countries Journal of Social and Economic History, 4(1), 3–38. https://doi.org/10.18352/tseg.557
- Monakhov, G. O., & Chechurin, L. S. (2012). The spectral analysis of the world gross domestic product’s growth and the patent activity. Nauchno-Technicheskie Vedomosti SPbGPU, 3, 176–179.
- Müller, K., & Wickham, H. (2023). Tibble: Simple data frames. R package version 3.2.1. https://CRAN.R-project.org/package=tibble
- Muñoz-Guillermo, M. (2021). Revisiting the business cycle model with cubic nonlinear investment function. Chaos, Solitons and Fractals, 142, 110510. https://doi.org/10.1016/j.chaos.2020.110510
- Nelson, R. R., & Winter, S. G. (1990). An evolutionary theory of economic change. The Belknap Press of Harvard University Press.
- Nerlove, M. (1964). Spectral analysis of seasonal adjustment procedures. Econometrica, 32(3), 241–286. https://doi.org/10.2307/1913037
- North, D. C. (1990). Institutions, institutional change and economic performance. Cambridge University Press.
- Notz, S., & Rosenkranz, P. (2021). Business cycles in emerging markets: The role of liability dollarization and valuation effects. International Review of Economics & Finance, 76, 424–450. https://doi.org/10.1016/j.iref.2021.04.010
- Owens, R. E., & Sarte, P D G. (2005). How well do Diffusion indexes capture business cycles? A spectral analysis. Federal Reserve Bank of Richmond Economic Quarterly, 91(4), 23–42.
- Patil, I., Makowski, D., Ben-Shachar, M. S., Wiernik, B. M., Bacher, E., & Lüdecke, D. (2022). Datawizard: An R Package for easy data preparation and statistical transformations. Journal of Open Source Software, 7(78), 4684. https://doi.org/10.21105/joss.04684
- Perry, P. (2023). Utf8: Unicode text processing. R package version 1.2.3. https://CRAN.R-project.org/package=utf8
- Pollock, D. S. G. (2008). The frequency analysis of the business cycle. Working Paper No. 08/12. University of Leicester.
- Pu, X., Zeng, M., & Luo, Y. (2021). The effect of business cycles on health expenditure: A story of income inequality in China. Frontiers in Public Health, 9, 653480. https://doi.org/10.3389/fpubh.2021.653480
- Qiao-Li, X., Wang, Y., & Zhou, W. X. (2021). Regional economic convergence in China: A comparative study of Nighttime Light and GDP. Frontiers in Physics, 9, 525162. https://doi.org/10.3389/fphy.2021.525162
- R Core Team. (2022). R: A language and environment for statistical computing. R Foundation for Statistical Computing, Vienna, Austria. https://www.R-project.org/
- Revelle, W. R. (2023). Psych: Procedures for psychological, psychometric, and personality research. Northwestern University. R package version 2.3.6. https://CRAN.R-project.org/package=psych
- Romer, P. (1990). Endogenous technological change. Journal of Political Economy, 98(5, Part 2), S71–S102. https://doi.org/10.1086/261725
- Romer, P. (2015). Speeding-up: Theory. https://paulromer.net/speeding-up-theory/
- Rossi-Hansberg, E., Sarte, P. D., & Schwartzman, F. (2019). Cognitive hubs and spatial redistribution, working paper series. WP 19-16. Cognitive Hubs and Spatial Redistribution. https://doi.org/10.21144/wp19-16
- Rostan, P., & Rostan, A. (2019). Forecasting Spanish GDPs with spectral analysis. Studies of Applied Economics, 36(1), 217–234. https://doi.org/10.25115/eea.v36i1.2526
- Saini, S., Ahmad, W., & Bekiros, S. (2021). Understanding the credit cycle and business cycle dynamics in India. International Review of Economics & Finance, 76, 988–1006. https://doi.org/10.1016/j.iref.2021.08.006
- Sturn, S., & Epstein, G. (2021). How much should we trust five-year averaging to purge business cycle effects? A reassessment of the finance-growth and capital accumulation-unemployment nexus. Economic Modelling, 96, 242–256. https://doi.org/10.1016/j.econmod.2020.12.028
- Takongmo, C. O. M., & Lebihan, L. (2021). Government spending, GDP and exchange rate in zero lower bound: Measuring causality at multiple horizons. Journal of Quantitative Economics: journal of the Indian Econometric Society, 19(1), 139–160. https://doi.org/10.1007/s40953-020-00213-z
- Taymaz, E. (2022). Regional convergence or polarization: The case of the Russian Federation. Regional Research of Russia, 12(4), 469–482. https://doi.org/10.1134/S2079970522700198
- Tisdell, C., & Svizzero, S. (2015). The Malthusian Trap and development in pre-industrial societies: A view differing from the standard one. ISSN: 1442-8563. Working Paper on Social, Economics, Policy and Development The University of Queensland.
- Tool, M. R. (1994). Institutional adjustment and instrumental value. Review of International Political Economy, 1(3), 405–443. https://doi.org/10.1080/09692299408434293
- Umirzakov, S. Y., Nauryzbayev, A. Z., Bukharbayeva, A. Z., Bekesheva, D. A., & Oralbayeva, A. K. (2019). Assessment of the supply chain management and problems of agricultural production development and marketing in Kazakhstan. International Journal of Supply Chain Management, 8(3), 256–265.
- Waring, E., Quinn, M., McNamara, A., de la Rubia, E. A., Zhu, H., Lowndes, J., Ellis, S., McLeod, H., Wickham, H., Müller, K., Kirkpatrick, C., Brenstuhl, S., Schrat, P., Mikko Korpela, I., Thompson, J., McGehee, H., Roepke, M., Kennedy, P., Possenriede, D., … Stewart, H. M. (2022). Skimr: Compact and flexible summaries of data. R package version 2.1.5. https://CRAN.R-project.org/package=skimr
- Weible, C. M., Nohrstedt, D., Cairney, P., Carter, D. P., Crow, D. A., Durnová, A. P., Heikkila, T., Ingold, K., McConnell, A., & Stone, D. (2020). COVID-19 and the policy sciences: Initial reactions and perspectives. Policy Sciences, 53(2), 225–241. https://doi.org/10.1007/s11077-020-09381-4
- Wickham, H. (2022). Stringr: Simple, consistent wrappers for common string operations. R package version 1.5.0. https://CRAN.R-project.org/package=stringr
- Wickham, H. (2023). Forcats: Tools for working with categorical variables (factors). R package version 1.0.0. https://CRAN.R-project.org/package=forcats
- Wickham, H., & Bryan, J. (2023). Readxl: Read Excel Files. R package version 1.4.2. https://CRAN.R-project.org/package=readxl
- Wickham, H., & Henry, L. (2023). Purrr: Functional programming tools. R package version 1.0.1. https://CRAN.R-project.org/package=purrr
- Wickham, H., Averick, M., Bryan, J., Chang, W., McGowan, L. D., François, R., Grolemund, G., Hayes, A., Henry, L., Hester, J., Kuhn, M., Pedersen, T. L., Miller, E., Bache, S. M., Müller, K., Ooms, J., Robinson, D., Seidel, D. P., Spinu, V., … Yutani, H. (2019). Welcome to the Tidyverse. Journal of Open Source Software, 4(43), 1686. https://doi.org/10.21105/joss.01686
- Wickham, H., Bryan, J., Barrett, M., & Teucher, A. (2023). Usethis: Automate package and project setup. R package version 2.2.1. https://CRAN.R-project.org/package=usethis
- Wickham, H., Chang, W., Henry, L., Pedersen, T. L., Takahashi, K., Wilke, C., Woo, K., Yutani, H., & Dunnington, D. (2016). Ggplot2: Elegant graphics for data analysis. Springer-Verlag. https://ggplot2.tidyverse.org
- Wickham, H., Francois, J. H. R., Bryan, J., & Bearrows, S. (2023). Readr: Read rectangular text data. R package version 2.1.4. https://CRAN.R-project.org/package=readr
- Wickham, H., François, R., Henry, L., Müller, K., & Vaughan, D. (2023). Dplyr: A grammar of data manipulation. R package version 1.1.2. https://CRAN.R-project.org/package=dplyr
- Wickham, H., Hester, J., Chang, W., & Bryan, J. (2022). Devtools: Tools to make developing R packages easier. R package version 2.4.5. https://CRAN.R-project.org/package=devtools
- Wickham, H., Kuhn, M., & Vaughan, D. (2022). Generics: Common S3 generics not provided by base R methods related to model fitting. R package version 0.1.3. https://CRAN.R-project.org/package=generics
- Wickham, H., Vaughan, D., Girlich, M., & Ushey, K. (2023). Tidyr: Tidy messy data. R package version 1.3.0. https://CRAN.R-project.org/package=tidyr
- Windle, A., Javanparast, S., Freeman, T., & Baum, F. (2023). Factors that influence evidence-informed meso-level regional primary health care planning: A qualitative examination and conceptual framework. Health Research Policy and Systems, 21(1), 99. https://doi.org/10.1186/s12961-023-01049-8
- Yang, M. (2020). Remeasuring and decomposing stochastic trends in business cycles. Research in Economics, 74(4), 354–362. https://doi.org/10.1016/j.rie.2020.10.006
- Ye, L., & Zhang, X. (2018). Nonlinear granger causality between health care expenditure and economic growth in the OECD and major developing countries. International Journal of Environmental Research and Public Health, 15(9), 1953. https://doi.org/10.3390/ijerph15091953
- Zhang, Y., & Ye, A. (2022). Improving global gross primary productivity estimation by fusing multi-source data products. Heliyon, 8(3), e09153. https://doi.org/10.2139/ssrn.3978981
- Zhu, H. (2021). KableExtra: Construct complex table with 'kable’ and pipe syntax. R package version 1.3.4. https://CRAN.R-project.org/package=kableExtra
Appendix 1
R packages for statistical calculations
The packages repr (version 1.1.6; Angerer et al., Citation2023), mnormt (version 2.1.1; Azzalini & Genz, Citation2022), magrittr (version 2.0.3; Bache & Wickham, Citation2022), effectsize (version 0.8.3.6; Ben-Shachar et al., Citation2020), pkgconfig (version 2.0.3; Csárdi, Citation2019), data.table (version 1.14.8; Dowle & Srinivasan, Citation2023), fansi (version 1.0.4; Gaslam, Citation2023), lubridate (version 1.9.2; Grolemund & Wickham, Citation2011), vtable (version 1.4.4; Huntington-Klein, Citation2023), kit (version 0.0.13; Jacob, Citation2023), ggpubr (version 0.6.0; Kassambara, Citation2023), collapse (version 1.9.6; Krantz, Citation2023a), fastverse (version 0.3.1; Krantz, Citation2023b), parameters (version 0.21.1; Lüdecke et al., Citation2020), performance (version 0.10.4; Lüdecke, Ben-Shachar, et al., Citation2021), easystats (version 0.6.0.8; Lüdecke et al., Citation2022), see (version 0.8.0; Lüdecke, Patil, et al., Citation2021), insight (version 0.19.3; Lüdecke et al., Citation2019), bayestestR (version 0.13.0.10; Makowski et al., Citation2019), modelbased (version 0.8.6.3; Makowski et al., Citation2020a, Citation2020b), report (version 0.5.7; Makowski et al., Citation2023), correlation (version 0.8.4; Makowski et al., Citation2022), tibble (version 3.2.1; Müller & Wickham, Citation2023), datawizard (version 0.8.0; Patil et al., Citation2022), utf8 (version 1.2.3; Perry, Citation2023), skimr (version 2.1.5; Waring et al., Citation2022), ggplot2 (version 3.4.2; Wickham et al., Citation2016), stringr (version 1.5.0; Wickham, Citation2022), forcats (version 1.0.0; Wickham, Citation2023), tidyverse (version 2.0.0; Wickham et al., Citation2019), readxl (version 1.4.2; Wickham & Bryan, Citation2023), usethis (version 2.2.1; Wickham, Bryan, et al., Citation2023), dplyr (version 1.1.2; Wickham, R. François, et al., 2023), purr (version 1.0.1; Wickham & Henry, Citation2023), readr (version 2.1.4; Wickham, J. H. R. Francois, et al., 2023), devtools (version 2.4.5; Wickham, Hester, et al., Citation2022), generics (version 0.1.3; Wickham, Kuhn, et al., Citation2022), tidyr (version 1.3.0; Wickham, Vaughan, et al., Citation2023), psych (version 2.3.6; Revelle, Citation2023) and kableExtra (version 1.3.4; Zhu, Citation2021).