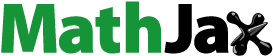
ABSTRACT
The current registered report investigated the effects of source credibility in relation to one’s own worldviews (i.e. supernatural beliefs and belief in science) in a spiritual and scientific context. We asked people to rate the truthfulness of ambiguous auditory statements about the cosmos attributed to a scientist or a spiritual guru and analyzed this using hierarchical Bayesian modeling. In line with our hypotheses, we found that the scientist was seen as more credible than the spiritual guru. The overall credibility of the statements was positively related to supernatural beliefs. These beliefs also interacted with the source of the statement, which was reflected in a tendency for supernatural believers to rate statements from both the scientist and the guru as credible. In contrast, with increasing belief in science, the credibility of the sources diverged with higher ratings for the scientist compared to the guru. The study involved a conceptual replication of previous research and increased the confidence in the robustness of source credibility effects and their interaction with people’s worldviews.
Introduction
Abstract entities like the space-time continuum, gravitational fields, and quantum energy are fascinating topics but incomprehensible to most people. An expert’s story on these invisible forces can make us feel overwhelmed and yet often we tend to trust the experts. Some experts tend to draw on scientific evidence to interpret the invisible world for us, while other experts rely on different sources. Spiritual gurus, for instance, can make strikingly similar statements to those of astrophysicists about the universe, but their conclusions rest mainly on experiential insight and intuition. Statements like “cosmic entities absorb energy at all frequencies” could be found in both scientific and spiritual discourse. Such statements appear meaningful, but they also are difficult to verify by the non-expert. Non-experts are therefore more likely to rely on their trust in the expert source to infer if the statement makes sense. The trust in an expert, in turn, depends on one’s personal beliefs and attitudes. Therefore, source credibility might interact with one’s own worldview and thereby affect the interpretation of statements, which is the focus of the present research.
Below, we first describe research on source credibility. We further elaborate on this topic in relation to leaders in a religious context. Scientists also enjoy a highly credible status in our society and their statements are often trusted and respected. However, one often overlooked aspect in research on this topic is the role of individual differences, such as one’s beliefs and attitudes. The current study investigated how people with different beliefs and worldviews (i.e. supernatural beliefs and beliefs in science) rate ambiguous statements from a spiritual and scientific source. If the statement is ambiguous, people tend to rely more strongly on contextual information to make inferences and derive meaning from the statement. Note that one can distinguish the ambiguity of the statement (i.e. content) from the ambiguity of the source (i.e. the context). Accordingly, in the present study, we used ambiguous statements (in content) that could be attributed to either a spiritual or a scientific authority (ambiguous in context), as this allowed us to study the top-down effects of source on the processing of statements. In short, content ambiguity is achieved by using meaningless, though not obviously false, profound-sounding statements, while context ambiguity is accomplished by selecting statements that could be encountered in both a spiritual and a scientific context (see the sections “Current research” below and “Pre-test” in the Online Supplemental Material).
We were also interested in elaborating how people’s supernatural worldviews interact with source credibility effects. Whereas religion typically refers to the more institutionalized aspects of supernatural beliefs, spirituality is more related to individual experiences. Both religion and spirituality typically involve the belief in supernatural invisible agents (Lindeman & Svedholm, Citation2012) that are characterized by minimally counterintuitive properties (i.e. they violate basic principles of the natural world and are therefore considered supernatural). Despite the different definitions associated with both concepts (for more extensive discussion, see: Maij et al. Citation2017) throughout this article we use the umbrella term “supernatural beliefs,” to refer to both people’s religious and spiritual beliefs, but when discussing the literature we adhere to the terms used in the respective articles.
Source credibility
We often ascribe meaning and significance to messages from presumed authorities on a specific topic. Source credibility can be defined as the effect of the credibility of the source on the perception of messages and stories from that source (Chaiken & Maheswaran, Citation1994; Pornpitakpan, Citation2004; Umeogu, Citation2012) and it involves the interplay of the message, the source and characteristics of the receiver (e.g. beliefs and worldviews; Roberts, Citation2010). Research on source credibility has demonstrated that in general people are more likely to perceive messages as credible if they believe in the trustworthiness and expertise of the source (Pornpitakpan, Citation2004). Next to the effects of source credibility, individual characteristics of the perceiver (e.g. initial disposition and personality) have been shown to affect people’s tendency to accept messages (Pornpitakpan, Citation2004). Research on the effects of source credibility has been framed in terms of the elaboration likelihood model (ELM), which proposes a dual-process model of attitude and belief formation (Petty & Cacioppo, Citation1986). On this account, information from sources can be processed through an intuitive and superficial route or through an analytical and reflective evaluation of the statements. When using the intuitive route, listeners are more likely to be affected by peripheral cues associated with the message, such as the attractiveness and the credibility of the source. Indeed, when reading ambiguous statements, the higher the credibility of the source, the more participants seemed to use heuristics and the more strongly they developed a positive attitude towards the described product (Chaiken & Maheswaran, Citation1994). In contrast, when using the analytical route, listeners engage in a more critical and reflective evaluation of the message, and they will be more likely to detect potential ambiguities or errors in the message.
Beyond general source credibility effects
Most research on source credibility has focused on persuasion, such as in advertising or health improvement. However, in the fields of science and religion, source credibility effects may be even more pronounced. For example, charismatic religious and political leaders often tend to use somewhat opaque language (e.g. jargon, non-falsifiable prophecies), which further adds to their credibility as an authoritative source (Pornpitakpan, Citation2004).
A similar process may be at play when people listen to scientific authorities. For example, scientific experts are typically consulted in the media as an important source of information. People often refer to scientific evidence because it takes discussions beyond subjective opinions (Faircloth, Citation2010). In general, the US population places a high trust in scientists (Funk et al., Citation2019) and statements containing irrelevant scientific jargon were judged to be more sound when trust in science was high (Weisberg et al., Citation2008, Citation2015). In a recent cross-cultural study, we found that overall people tend to be less skeptical towards meaningless statements attributed to a scientist compared to a spiritual guru, further evidencing the widespread strong trust that people have in scientific authorities (Hoogeveen et al., Citation2022).
Individual differences
The effects of source credibility on message acceptance are likely affected by individual differences in beliefs, and could possibly be related to thinking style and receptivity to pseudo-profound bullshit. The role of individual differences in source credibility is exemplified, for instance, in the domain of policy making, where people’s political worldviews affected expert credibility (Lachapelle et al., Citation2014). Supernatural beliefs and belief in science may be especially prominent in affecting source credibility effects. The relationship between supernatural beliefs and belief in science, commonly described as in conflict, is multifaceted and complicated (McPhetres & Nguyen, Citation2018). Religious beliefs were shown to be negatively correlated with scientific knowledge, and this effect was partially mediated by the attitude towards science (McPhetres & Zuckerman, Citation2018). Attitude towards science was less positive for religious people, but only in some cultures (McPhetres et al., Citation2020). Other research suggests that the overall belief in science correlates negatively with spirituality (Farias et al., Citation2013; Rutjens & van der Lee, Citation2020). We previously showed that religiosity is more strongly predictive of credibility ratings for meaningless statements from a guru, than of credibility ratings for meaningless statements from a scientist (Hoogeveen et al., Citation2022). Furthermore, research has suggested that religious people in general might be less skeptical in evaluating evidence in support of both religious and scientific statements (McPhetres & Zuckerman, Citation2017). In another study, it was reported that supernatural beliefs increased the likelihood of finding supernatural stories credible and scientific (Garrett & Cutting, Citation2017).
Individuals tend to differ in their faith in science and in its superior status as a source of knowledge; as such belief in science can even be considered a worldview, akin to religious worldviews (A. Evans et al., Citation2020; Farias et al., Citation2013; Taves et al., Citation2018). For example, high faith in science could lead to less scientific skepticism, thereby influencing message acceptance (Mayo, Citation2019). Previous research has also shown that scientific statements that conflict with one’s worldview are difficult to accept and people often interpret these statements in favor of their own worldview (Kahan et al., Citation2011, Citation2012). Indeed, in a recent study, participants’ belief in scientific statements was not influenced by their perception of the credibility of the scientist but merely by their own views (Kobayashi, Citation2018). Still, physical appearances of scientists (i.e. perception of the individual source) may influence their rated quality as Gheorghiu et al. (Citation2017) found that more competent looking scientists are perceived as more credible. Thus, the general perception of the credibility of scientists seems to be different from the individual perception of a scientist. The research by Kobayashi (Citation2018) also suggests that strong beliefs about a specific scientific topic (e.g. vaccination, threat of COVID-19) might be less influenced by source credibility effects, because these beliefs are already deeply ingrained (e.g. through the media and experiences) and different from general attitudes towards science. The inconsistent results of the relationship between supernatural beliefs and belief in science might be dependent on the topic (e.g. a highly contested one like creationism vs. an uncontested one like “electrons are smaller than atoms”; J. H. Evans, Citation2011; McPhetres & Nguyen, Citation2018).
Next to supernatural beliefs and belief in science, thinking style might affect source-credibility effects. Science and atheism have often been associated to an analytical thinking style (Pennycook et al., Citation2012; Pennycook, Fugelsang et al., Citation2015), whereas supernatural beliefs have been associated to an intuitive thinking style (Pennycook, Cheyne et al., Citation2015). On the other hand, using latent class analysis to identify both skeptic and religious groups, Lindeman and Lipsanen (Citation2016) found that there were both low and high analytical thinkers within each of these groups. Another article reported that across three different studies there was no relationship between thinking style (i.e. analytical or intuitive thinking) and supernatural beliefs (Farias et al., Citation2017). The proposed relationship has been further contested based on cross-cultural research, showing that there is a large cultural variability in the relation between religion and intuitive thinking (Gervais et al., Citation2018). In sum, the relationship between a preference for an intuitive compared to an analytical processing style and supernatural beliefs is strongly dependent on the cultural context and might be less straightforward than previously thought. But so far, the effects of thinking style on source credibility have not been investigated, even though the elaboration likelihood model predicts that an intuitive thinking style should render individuals more vulnerable to be affected by source credibility effects.
A general gullibility and receptivity to pseudo-profound bullshit (i.e. meaningless jargon that contains references to scientific entities, such as quantum theory or photons) might also be a factor influencing the perceived profoundness of statements (Pennycook, Cheyne et al., Citation2015). Belief in science was found to be slightly positively correlated to receptivity to pseudo-profound bullshit and both were also correlated with receptivity to scientific bullshit (A. Evans et al., Citation2020). Faith in intuition was also correlated to receptivity to bullshit. However, susceptibility to bullshit might reflect a general individual trait that is not influencing the effects that different sources have on message processing.
Current research
In sum, individual differences exert a strong effect on how we evaluate statements from a scientific or spiritual authority. It remains unclear how the source (irrespective of the content) influences the credibility of statements and the relationship with supernatural beliefs and belief in science. Therefore, in the present study, we asked people to rate ambiguous statements that are attributed to a spiritual guru or a scientist. We did not use media-covered topics (because of pre-existing opinions, as discussed above) but a topic that could be discussed by both scientists and spiritual leaders alike. The statements were ambiguous in terms of both their content and their inferred source, i.e. the statement could be made both by a scientist or a guru. We manipulated ambiguity by creating meaningless though profound-sounding statements on topics related to quantum mechanics and the universe. We used pseudo-scientific jargon to make the statements sound profound, to make it more difficult for participants to infer the meaning of the statement and to directly verify whether the statement is true. In a pre-test, the statements were matched in terms of their perceived spirituality and scientificity. We also selected statements that were perceived to be somewhat truthful, and we excluded statements that were clearly completely true or false. This way, we assessed to what extent people would rely on source credibility cues and their own background beliefs to infer the credibility of the statement. We included a question about the participant’s astrophysics knowledge to account for personal background knowledge of the topic that could play a role in processing the statements. We also included thinking style and susceptibility to pseudo-profound bullshit in our exploratory analyses, as they may play a role in source credibility effects.
This registered report study extends our previous cross-cultural work (Hoogeveen et al., Citation2022) in three ways. First, we assessed the effects of supernatural beliefs on source credibility, in contrast to solely religious beliefs, and we included a well-validated measure of belief in science to investigate its effect on source credibility as well. Second, instead of using statements presented on screen, here we used auditory stimuli to ensure participants have the same amount of time to process a stimulus (as opposed to reading). Using auditory stimuli also opens the path for future functional Magnetic Resonance Imaging (fMRI) experiments to investigate the neural mechanisms underlying source credibility effects. A key advantage of using auditory stimulus presentation is that it allows a passive task design, thereby avoiding potential confounds related to overt eye movements. Thus, our registered report study also provided a proof-of-concept by developing and making available a new experimental paradigm that can be used in future studies. Third, by repeatedly presenting participants with statements from different sources (i.e. instead of only presenting a statement once) we aimed to investigate the dynamics of source credibility effects over time. This also allows future studies to focus on the neurocognitive correlates of source credibility, e.g. by using a repeated-measurements electroencephalography or fMRI designs, and to assess how source credibility effects might enhance or decline over time (i.e. learning effects).
Hypotheses
The hypotheses listed below represent our general confirmatory hypotheses, which were complemented with additional exploratory analyses, highlighted in the next subsection.
First, we expected that a scientist is in general seen as more credible than a spiritual guru (A. Evans et al., Citation2020; Funk et al., Citation2019):
: Ambiguous messages are rated as more credible when pronounced by a scientist than by a spiritual guru (i.e. we expect a main effect of source)
It has been found that individuals with increased pseudo-profound bullshit receptivity were more likely to believe in the supernatural (Pennycook, Cheyne et al., Citation2015) and belief in the supernatural increased the likelihood of finding a statement credible (Garrett & Cutting, Citation2017). Accordingly our second hypothesis was that:
: Supernatural beliefs are positively related to credibility ratings; participants that score higher on supernatural beliefs will rate ambiguous statements as more credible (i.e. we expect a main effect of supernatural beliefs)
Together, and
indicate that people with higher supernatural beliefs would rate statements from both the spiritual authority and scientific authority as high in credibility, because scientists are generally considered credible, while supernatural believers may also put much trust in the guru. On the other end, people with lower supernatural beliefs would be more skeptical towards an authority with different worldviews than their own, but at the same time rate a science authority as relatively high in credibility because of the source credibility effect of science (
) (cf. Hoogeveen et al., Citation2022). This idea is further supported by the observation that religious people require a similar amount of evidence for a scientific claim as non-religious people, whereas religious people were faster to accept evidence for a religious claim than non-religious people (McPhetres & Zuckerman, Citation2017). These observations resulted in the following interaction hypothesis:
: Crucially, the effect of source on statement credibility will be stronger for participants scoring low compared to high on supernatural beliefs (i.e. there is a negative interaction effect between source and supernatural beliefs)
Belief in science has been proposed as an alternative worldview (Farias et al., Citation2013; Taves et al., Citation2018). People scoring high on belief in science will particularly rate the scientist as more credible than the guru, while for people with lower belief in science, the difference in credibility ratings between the scientist and the guru will be less pronounced:
: The effect of source on statement credibility will be stronger for participants scoring high compared to low on belief in science (i.e. there is a positive interaction effect between source and belief in science).
Variables for exploratory analyses
Thinking style, bullshit receptivity and knowledge of astrophysics have been introduced as measures that relate to our topic of interest. We have not included these measures in the main hypotheses above to keep a clear focus. Nonetheless, they could be related to our dependent measure (the ratings) and therefore were analyzed subsequently in an exploratory way.
Non-registered studies
We have performed a pre-test to select computerized-voices and ambiguous statements that we describe in the Online Supplemental Material, followed by a pilot study that provides the groundwork for the preregistered study. Data and scripts can be found on https://osf.io/v92gy.
Methods
Participants and sampling plan
Participants were recruited through online advertisements. Due to the COVID-19 pandemic, advertising at venues with scientific and spiritual events, which was part of the preregistered recruitment method, was not possible. To reach our planned sample size, we also collected data through Prolific.com. Participants received 5 euro as compensation. Individuals were included if they were between 18 and 70 years old.
The online survey was spread among participants without a fixed predetermined number of observations. We adopted a Bayesian inference framework, which allowed us to monitor evidence while data accumulated, without running into statistical problems associated with optional stopping (i.e. inflated Type I error rates; Rouder, Citation2014; Schönbrodt et al., Citation2017; Wagenmakers et al., Citation2018). Initially, 60 participants were recruited (that did not meet the exclusion criteria). Subsequently, we continued collecting data until the Bayes factor for each of the critical tests (i.e. : the source
supernatural beliefs interaction and
: the source
belief in science interaction) passed the threshold for sufficient evidence, i.e. BF
or BF
, which means that the data are at least 6 times more likely under the alternative model than under the null model, or vice versa.Footnote1 The online format and advertising did not permit to check the evidence after each participant. Therefore, we checked the evidence approximately every 10 participants offline. We preregistered to stop data collection when the criteria for evidence were met or a maximum of
(due to monetary reasons) was reached. Importantly, we checked whether our sample had a sufficient variability of supernatural beliefs scores, i.e. including people with low scores and high scores. Specifically, we expected more difficulties when recruiting people with higher scores, as this is a minority group in the general population in the Netherlands. We aimed to achieve an approximately equal division between mean supernatural beliefs scores above and below the scale midpoint of 3.
Design
Stimuli. The selection of ambiguous stimuli and computerized-voices is described in the section “Pre-test” in the Online Supplemental Material. In total, we included 24 ambiguous auditory stimuli in our paradigm on the topic of astrophysics. The full list of statements, including a link to the recording, can be found on: https://osf.io/8gcrp/. Statements were ordered based on ambiguity and split into two subsets of 12 stimuli. We compared both sets on ambiguity (i.e. difference between applicability to science and spirituality; BF). We also checked that truthfulness did not differ between the two subsets (BF
).
To increase voice naturalness, 500 ms silences were inserted between sentences. This was also done at the stimulus end to prevent an abrupt ending. Compensation for the speed difference between both voices on the same stimuli was achieved by slowing down the fast voice and increasing the speed of the slow voice to their average speed on that stimulus. The final stimuli lasted on average 18.82 seconds (std = 1.24 s, min = 16.81 s, max = 20.68 s). The spoken text was preceded by a silence of 300 ms.
Task. The task consisted of a short introduction, the rating of auditory stimuli and a few questions. First, participants were introduced to the goals and objectives of the study. Specifically, participants were instructed that the study was aimed at uncovering their worldviews. Second, a spiritual guru and scientist were introduced using a photo and a descriptive text. Additionally, participants listened to a (fictional) quote of each source. We presented the sources as an authority in their own field. The description was fictional and photos were found online with re-use permission. However, participants were made to believe that these were real people and that their statements were recorded from documentaries, translated and again recorded with computerized-voices for the purpose of this experiment. As a first step in this field, we chose to maximize our manipulation, by manipulating both the cover story, the picture and the background. Thus, our manipulation can be considered a package deal, while leaving it to follow-up studies to single out which specific factors might be more crucial in driving source credibility effects. Then, the participants were presented with the 24 statements. After each statement, they were asked to what extent they think the statement is true of the world (the dependent variable). This was evaluated on a 6-point Likert scale (1 = completely false, 6 = completely true; see .
Randomization was achieved by making four sets of stimuli. Each participant was randomly assigned to a set during the experiment. In two sets (A and B), voice one belonged to the spiritual guru and voice two belonged to the scientist, in the other two (C and D) this was reversed. An overview of the randomization can be seen in Online Supplemental Table 4. During the experiment, statements of the spiritual guru and the scientist were presented in random order. Following the 24 statements, participants were asked to link the photo to the name of the corresponding authority in the experiment, as an attention check. Next, the participants were asked to rate six questions on a 5-point Likert scale about their trust in the spiritual guru and scientist, the perceived competence of the spiritual guru and scientist, and their understanding of the statements from each source. These six questions were used as descriptive measures. In addition, participants had the opportunity to comment about the task in an open question.
Table 1. Descriptives per source.
Table 2. Bayes factors of the different models.
Questionnaires
After the task, participants filled in several questionnaires. A 10-item supernatural beliefs questionnaire was administered (first independent variable), assessing belief in supernatural phenomena (e.g. spiritual healing, angels) on a 5-point Likert scale (as used in previous studies; Van Elk & Snoek, Citation2020). Other studies asked mainly whether participants viewed themselves as spiritual (e.g. Rutjens & van der Lee, Citation2020). Spirituality is a broad concept and many people identify with (different) aspects of spirituality (Rutjens & van der Lee, Citation2020); therefore, we used a supernatural beliefs questionnaire to address different aspects and investigate a person’s worldview more directly. In addition, an attention check was embedded in this questionnaire where participants were asked to select the instructed pre-defined answer. A 5-item belief in science questionnaire was administered to investigate this as an alternative worldview (second independent variable). This was an adapted version based on Farias et al. (Citation2013) and Rutjens et al. (Citation2018) from which we deleted items related to religiosity to solely assess belief in science and not their conflicting worldviews. The order of the supernatural beliefs and the belief in science questionnaires was randomized.
For a first exploratory analysis, we also added the Cognitive Reflection Test (CRT), to address thinking style, with a previously used selection of six out of seven original items (Pennycook et al., Citation2020; Thomson & Oppenheimer, Citation2016). Questions similar to these items are often used, which can harm the validity of the measure (see however Bialek & Pennycook, Citation2018). This was circumvented by asking participants “Have you seen this questionnaire before?” and removing all confirmatory responses from further analysis. For a second exploratory analysis, we added the Bullshit Receptivity (BSR) scale to measure the construct validity of our statements (Pennycook, Cheyne et al., Citation2015). The order of these exploratory measures was not randomized. Subjects’ objective knowledge of astrophysics was assessed using five knowledge questions after the task. This was used for the third exploratory analysis.
Procedure
Participants got access to the survey on Qualtrics. They provided online informed consent and were asked to fill in demographics (age, gender, religiosity, spirituality) and a question about their subjective knowledge of the topic on a 10-point Likert scale, i.e. “How much knowledge do you have about astrophysics/space/quantum mechanics? Topics include for example, the origin of the universe, black holes and the behavior of particles.” Participants were then asked to listen to a test fragment after which they were prompted by a question what the fragment was about. This was done in order to check whether their sound worked and they were listening. Participants could listen multiple times and only when they provided the correct answer (i.e. dog), they could proceed with the experiment. Participants were randomly assigned to one of four sets and presented with the task. Afterwards, they were asked to rate the sources on competence and trustworthiness, complete the supernatural beliefs questionnaire, the belief in science scale, the astrophysics knowledge questionnaire, the CRT and the BSR scale. Participants were rewarded with 5 euro.
Descriptive statistics
We present descriptive statistics of our sample including age, gender, religiosity, spirituality, scores on the belief in science, supernatural beliefs and astrophysics knowledge questionnaire, the performance on the CRT, average BSR score, average trust and competence of each of the sources and average understanding of the statements. Additionally, we report correlations between the different measures that we included in our study, e.g. the CRT with the BSR and the BSR with belief in science scale.
Data analysis
We applied hierarchical Bayesian modeling to account for the nested structure of the data (observations within participants), using the BayesFactor package (Morey & Rouder, Citation2018). This method is based on the work by Haaf and Rouder (Citation2017); Rouder et al. (Citation2019). First, we conducted preliminary analyses to check if there were any effects of voice, stimulus subset, and trial sequence. If the Bayes factor for the presence of an effect was larger than 3 (i.e. BF), we included the respective factor in the main analyses. If not, we left it out – as is done for the analysis of the pilot data (see Online Supplemental Material). Second, we tested to what extent the data provided evidence for the source effect on statement credibility ratings (
), using the models (i) to (iv) described below. Third, we extended the hierarchical models by including the covariates gender and age (Legare et al., Citation2012; Randall & Desrosiers, Citation1980), as well as the second-level predictor supernatural beliefs to test
. For this analysis, the parameter for the main effect of supernatural beliefs was restricted to be positive, as we expected higher supernatural beliefs to be predictive of higher overall credibility ratings. As preregistered, we first checked the correlation between supernatural beliefs and belief in science scores and used a cut-off of
to determine multicollinearity of the predictors. We would then proceed with two models with their respective cross-level interactions with source, to test
(source
supernatural beliefs) and
(source
belief in science). If the predictors were not strongly correlated, we would test both interactions in the same model. As we expected the source effect to become weaker with increased supernatural beliefs and stronger with increased belief in science, we restricted the source-by-supernatural beliefs interaction parameter to be negative and the source-by belief in science interaction parameter to be positive in this analysis. Analyses were carried out in R.Footnote2
Statistical models
The multilevel Bayesian modeling approach allows us to systematically evaluate the evidence in the data under different models: (i) for all participants the effect is truly null; (ii) all participants share a common nonzero effect; (iii) participants differ, but all effects are in the same direction; and (iv) for some participants the effect is positive whereas for others the effect is negative. The models differ in the extent to which they constrain their predictions, from the most constrained (i) to completely unconstrained (iv). We refer to these models as the null model, the common effect model, the positive/negative effects model, and the unconstrained model, respectively. Note that models (iii) and (iv) solely apply to the first-level effect of source; for the second-level predictor supernatural beliefs and the cross-level interaction between source and supernatural beliefs, only models (i) and (ii) are relevant.
Prior Settings. The BayesFactor package applies the default priors for ANOVA and regression designs described in Rouder et al. (Citation2012), in which the researcher can determine the scale settings for each individual predictor in the model. We used the settings for the critical priors in the multilevel models as proposed by Rouder et al. (Citation2019), concerning the scale settings on and
. The scale on
reflects the expected size of the overall source effect and is set to
. The scale of
reflects the expected amount of variability in effect size across participants. This scale is set to
of the overall effect, resulting in a value of
. For the effects that are not relevant for the specific hypotheses of interest, uninformative priors were used. Specifically, we set the prior scale for the overall between-subjects variance to 1. When testing the main effect of supernatural beliefs in
, we additionally used a prior scale of
Footnote3 for the source predictor. When testing the interaction in
, the prior scale of 0.707 was used for the parameters of source and supernatural beliefs. In addition, we assessed the robustness of the results under different priors. Specifically, we varied the priors for the effect of interest using the following
scale settings, in decreasing order of informativeness:
,
, and
(corresponding to the “medium,” “wide” and “ultrawide” prior scale settings provided in the BayesFactor package (Morey & Rouder, Citation2018; Rouder et al., Citation2012).
Inference criteria
We used model selection by means of Bayes factors to draw inferences. That is, we calculated Bayes factors that reflect the relative evidence in the data for various constructed models, including models that correspond to the null hypotheses. A Bayes factor of 6 in favor of the respective alternative models vs. the null model is required to consider the hypothesis supported. We applied the sequential sampling design to our critical tests (i.e. : the source
supernatural beliefs interaction and
: the source
belief in science interaction) and continued collecting data until we reached sufficient evidence BF
or BF
or until a maximum of
was reached.
Data exclusions
We excluded data from participants who failed any of the following criteria:
1. Participants should have completed the experiment in less than 1.5 hours (estimated completion time is 30 minutes). Participants who spent more than 1.5 hours on the task would have had too much time between listening to the statements and responding to the questions, which compromises the validity of the responses.
2. Participants should have selected the instructed pre-defined answer for the attention check item embedded in the supernatural beliefs questionnaire.
We preregistered to exclude participants who did not correctly link the name and photo to the corresponding authority in the experiment, i.e. the person who appeared as the scientist should have been identified as the scientist and the person who appeared as the guru should have been identified as the guru. However, since various participants indicated that they misunderstood this item or thought it was a trick question, we decided to leave the 14 who failed this item in the analytic sample and report the results excluding them as part of the robustness analysis.
Exploratory analyses
To investigate the underlying mechanism of source credibility effects, we used scores on the CRT as a predictor for the ratings. Participants with a confirmatory response to the question “Have you seen this questionnaire before?” were removed from this analysis. In addition, we correlated scores on the BSR to overall credibility ratings, as a measure of construct validity of our statements. Bullshit receptivity was not included as a predictor in our model, because we are interested in effects of beliefs on the source effects and not the general credibility ratings (i.e. intercept, which would reflect bullshit receptivity). Finally, we added astrophysics knowledge as a predictor to the main model and tested whether knowledge is predictive of overall credibility ratings and whether it interacts with the source effect.
Results
Following the sequential sampling plan, we recruited participants until we obtained strong evidence (BF > 6 or BF < 1/6) for or against the crucial interaction effects between source and supernatural beliefs () and between source and belief in science (
), after exclusions. This resulted in an analytic sample of 176 participants, from which 76 via direct advertisement (email lists, online groups, snowballing) and 100 through Prolific. In total, 203 participants started the survey and 181 participants finished the survey. Of those, 4 participants failed one or two of the explicit attention checks and
participant reported being older than 70, which was preregistered as an exclusion criterion. This resulted in 176 participants (Mean age = 37.2, SD = 14.8, range: [18, 69]; 48.3% females) in the analytic sample.
A Bayesian reliability analysis using the Bayesrel package (Pfadt et al., Citation2021) indicated good internal consistency of the supernatural beliefs scale, McDonald’s omega = 0.908 [0.889, 0.928], with no indication that any item should be removed. Similarly, we found good reliability for the belief in science scale (McDonald’s omega = 0.863 [0.835, 0.897]) and for the bullshit receptivity scale (McDonald’s omega = 0.840 [0.805, 0.874]. Removing any of the items would not improve the internal consistency of either scales.
Descriptives
The descriptive statistics of the ratings per source are given in . The correlations between the different variables are presented in and .
Table 3. Correlation table measured variables.
Table 4. Correlation table credibility per source.
Confirmatory analyses
Preliminary analyses
As preregistered, we first investigated to what extent the experimental effect could be influenced by the specific voice and subset of stimuli that were used or the pairing of the sources with the voice and the subset of stimuli. We also assessed the effect of time, i.e. the evolution of the ratings over the sequence of trials. As can be seen in Online Supplemental Table 5, there is (some) evidence for the absence of a main effect of voice, a voice-by-source interaction, a stimulus set-by-source interaction, and a trial sequence effect. For the main effect of stimulus set, the evidence in favor of an effect is moderate (i.e. BF 9.93), suggesting that the overall ratings for stimulus set 1 are slightly higher than for stimulus set 2. Note that this main effect might thus influence the intercepts, but not the crucial effects of source and the interactions between source and supernatural beliefs or belief in science. Nevertheless, as preregistered, we added stimulus set as a covariate in the main analyses (see also the robustness analyses for results without covariates). Online Supplemental Figure 4 further illustrates the absence of these effects.
Effect of source
For the main effect of source, we compared between the model without an effect of condition (i.e. the scientist and spiritual guru are judged equally credible), the model with a common positive effect of condition across participants (i.e. the scientist is judged as more credible than the spiritual guru, to an equal degree by everyone), the model with a varying positive effect of source (i.e. the scientist is judged more credible than the spiritual guru, but to varying degrees by different participants), and the model that allows the source effect to be varying from positive to negative (i.e. some people consider the scientist more credible than the spiritual guru, others consider the spiritual guru more credible than the scientist).
The Bayes factor model comparison summarized in the top rows of shows that the data provide most evidence for the unconstrained model, which assumes variability between people such that some people consider the scientist more credible than the guru, whereas others consider the guru more credible than the scientist. This effect is visualized in . At the same time, we do find strong evidence for the source effect over the null-model: BF
and for the varying positive effect: BF
. This qualifies as strong evidence for a common source effect and strong evidence for a varying source effect, respectively. These results indicate that, on average, people consider the scientist more credible than the guru. The mean of the unstandardized size of the source effect (i.e. the regression coefficient) is 0.37,
credible interval [0.26, 0.49] and the standard deviation between participants is 0.46. additionally shows the intercepts for the credibility ratings per subject (irrespective of the source).
Effect of supernatural beliefs
As preregistered, we first checked the correlation between supernatural beliefs and belief in science. Since the observed correlation Pearson's () is smaller (i.e. stronger) than the criterion of
, we assessed the effects of supernatural beliefs and belief in science separately.
First, we assessed the main effect of supernatural beliefs on overall credibility ratings, i.e. are supernatural beliefs associated with higher credibility ratings for pseudo-profound statements? As shown in the middle rows of , the Bayes factor model comparison provided most evidence for the full model that included both a main effect of supernatural beliefs and an interaction between source and supernatural beliefs. Specifically, we find a Bayes factor of BF 32,895.49 (see ), which qualifies as strong evidence for the main effect of supernatural beliefs, indicating that higher supernatural beliefs are associated with higher overall credibility ratings. The mean of the unstandardized size of the effect of supernatural beliefs is 0.23,
credible interval [0.14, 0.32].
Table 5. Summary of Bayes factor model comparisons.
To assess the evidence for the interaction, we compared the null model to the model that additionally included a common interaction term (i.e. model 3). The interaction term was constrained to be negative, in the sense that the difference in credibility between sources was hypothesized to become smaller with increased supernatural beliefs. The critical Bayes factor for the source-by-supernatural beliefs interaction effect vs. the null model is BF 21.96 (see ). This qualifies as strong evidence for a source-by-supernatural beliefs interaction. Assuming a main effect of supernatural beliefs, we get a Bayes factor of BF
22.64 for the additional inclusion of the interaction term; strong evidence for the interaction. As hypothesized, the interaction entails that the relatively higher credibility for statements from the scientist vs. the spiritual guru decreases with higher supernatural beliefs. The mean of the unstandardized size of the source-by-supernatural beliefs effect is −0.18,
credible interval [−0.29, −0.07]. See also that visualizes the interaction based on the predicted effect of supernatural beliefs on credibility per source, derived from the posterior distributions of the parameters.
Effect of belief in science
As shown in the bottom rows of , the Bayes factor model comparison again provided most evidence for the full model that included a main effect of belief in science and an interaction between source and belief in science. The investigation of the main effect of belief in science is exploratory and has not been preregistered. The evidence for the main effect of belief in science on truth ratings is BF 75.37 (), which qualifies as strong evidence for a main effect of belief in science. This main effect indicates that higher belief in science are associated with lower overall credibility ratings. The mean of the unstandardized size of the effect of belief in science is −0.16,
credible interval [−0.26, −0.07].
The critical Bayes factor for the source-by-belief in science interaction effect vs. the null model is BF 283.13 (), which qualifies as strong evidence for a source-by-belief in science interaction. Assuming the main effect of belief in science, the evidence for additionally including the interaction term is BF
292.53; again strong evidence for the interaction. As hypothesized, the interaction effect entails that the relatively higher credibility for statements from the scientist vs. the spiritual guru increases with higher belief in science. The mean of the unstandardized size of the source-by-belief in science effect is 0.22,
credible interval [0.11, 0.33]. See also for the predicted effect of belief in science on credibility for both sources.
Figure 2. Hierarchical model estimates for the source effect (). The filled points are hierarchical estimates (unconstrained model) with 95% credible intervals, ordered from largest to smallest and +’s are the observed sample means. Red points and +’s denote negatively valued effects. The shaded bands give the 95% credible interval for the estimated effects. The horizontal line indicates zero. A. Individual variability in overall credibility ratings (individual intercepts) B. Credibility by source. Positive values for the source effect indicate scientist > guru and negative values indicate guru > scientist. C. Predicted credibility by source and supernatural beliefs (interaction effect). D. Predicted credibility by source and belief in science (interaction effect).

Exploratory analyses
Cognitive reflection task
In an exploratory fashion, we re-ran the models for and
with CRT scores as the predictor of interest. Specifically, we assessed whether there is a negative relation between CRT scores and overall credibility ratings (i.e. a main effect), and whether there is an interaction between source and CRT scores. As preregistered, we only included participants who indicated that they were not familiar with the CRT items used in the survey (
133). The evidence for the main effect of CRT on credibility ratings is BF
0.58; BF
1.71, which qualifies as anecdotal evidence against a main effect of CRT. The Bayes factor for the source-by-CRT interaction effect vs. the null model: BF
0.11; BF
9.17; moderate evidence against a source-by-CRT interaction.
Bullshit receptivity
To validate our statements, we correlated the scores on the 10-item Bullshit Receptivity scale (BSR) to credibility ratings in our task. As expected, we found that the BSR score and the credibility ratings were positively correlated for the guru: Pearson’s 0.38, 95% credible interval [0.25, 0.50], BF
361,799.83 and for the scientist: Pearson’s
0.30, 95% credible interval [0.15, 0.50], BF
1,443.05. shows this association in a scatterplot, reflecting that higher bullshit receptivity is associated with higher overall credibility ratings.
Astrophysics knowledge
Similar to the effect of CRT, we also assessed astrophysics knowledge as predictor in the models. The evidence for the main effect of astrophysics knowledge on credibility ratings is BF 0.07; BF
14.81, which qualifies as strong evidence against a main effect of astrophysics knowledge. The Bayes factor for the source-by-astrophysics knowledge interaction effect vs. the null model: BF
1.66; BF
0.60; anecdotal evidence for a source-by-astrophysics knowledge interaction.
Robustness checks
We assessed the robustness of our results by conducting three additional analyses. First, we removed the covariates age, gender, and stimulus set from the models for ,
, and
(note that
only assessed a within-subjects effect, so no between-subjects covariates were added in the first place). Second, we excluded participants who failed the photo-source matching item, but this emerged as unclear based on participant reports and is only reported here as part of the robustness analyses. Third, we used a different, less informed prior setting for r scale; r = √(2)/2 ≈ 0.707 corresponding to the default wide prior setting in the BayesFactor package (Morey & Rouder, Citation2018). As shown in Online Supplemental Table 6, the results for the interaction effects are robust against these three different analysis paths; the BFio is consistently above 10 and the preferred model is always the full model that includes the main effect as well as the interaction term.
Evolution of credibility over time
We estimated the effect of trial number in the experiment (i.e. time) per source per subject to get insight into the evolution of the ratings over the course of the experiment (Online Supplemental Figure 5). As already became apparent in the raw data visualized in Online Supplemental Figure 4, there does not seem to be a trend upwards or downwards in the evaluation of the statements. This is corroborated by the posterior samples for the slopes of time, for which we estimated a slope per subject per source. Specifically, for 100% of the subjects the 95% credible interval of the slope of time for the guru included 0 and for 97.73% of the subjects the 95% credible interval of the slope of time for the scientist included 0 (for 1 subject the slope for the scientist was reliably negative, and for 3 subjects it was reliably positive). Apparently, people determined an initial level of credibility for each source (range guru: [2.44, 5.21]; range scientist: [2.35, 5.33]) and did not substantially revise this assessment during the experiment.
Discussion
The current study aimed to shed light on the influence of worldviews on source credibility effects. In line with , our results showed that the scientist was on average seen as more credible compared to the guru, which was also confirmed in ratings of trust and competence for both sources. The hypothesis stating a positive main effect of supernatural beliefs on credibility ratings (
), was also supported in our sample. Furthermore, there was strong evidence for the interaction between supernatural beliefs and source (
) such that increasing supernatural beliefs were associated with a reduced difference between the credibility of the scientist and guru. There was also a negative main effect of belief in science on credibility ratings, i.e. higher belief in science was associated with lower credibility ratings (note that this effect was not preregistered). More importantly, we found strong evidence for a source-by-belief in science interaction effect (
) reflecting that the credibility ratings of the scientist and guru diverged with increasing belief in science. Based on these findings, we conclude that scientific and supernatural worldviews influence source credibility effects in predictable but opposing ways.
In line with previous studies, we found that the credibility of a message is affected by the source delivering it (Chaiken & Maheswaran, Citation1994; Pornpitakpan, Citation2004; Umeogu, Citation2012), and that individual differences in worldviews have a moderating effect on source credibility (Roberts, Citation2010). In addition, our supported hypotheses are in agreement with previous findings, i.e. an increase in supernatural beliefs is generally associated with an increase in perceived credibility, but more so for the guru than for the scientist (Hoogeveen et al., Citation2022). This effect is reversed for belief in science (Garrett & Cutting, Citation2017; Kahan et al., Citation2011, Citation2012; Kobayashi, Citation2018).
Our data indicate that the credibility of the scientist is less affected by people’s worldview, while the credibility of the spiritual guru differs strongly between people from different backgrounds. From this observation, we hypothesize that worldviews mainly affect the credibility of the guru, while the high trust in and credibility of a scientist is more robust (Hoogeveen et al., Citation2022) and is less susceptible to one’s prior worldview. This is in line with earlier research also indicating that people in general have a high trust in scientists (Funk et al., Citation2019) and the observation that scientific evidence adds to the importance of arguments in subjective discussions (Faircloth, Citation2010). On the other hand, a guru is generally less visible in Western societies (e.g. in the news or media) which might have reduced the familiarity and perceived trustworthiness of the guru compared to the scientist. However, even in countries such as India and China, where a spiritual leader might be more prominent in daily life, a scientist was seen as more credible overall (Hoogeveen et al., Citation2022).
Our exploratory analyses demonstrated that the above results are robust to the inclusion or exclusion of covariates, participants failing a sub-optimally designed attention check and to the use of a less informative prior. Moreover, in our exploratory analyses we investigated several mechanisms possibly of influence on the hypothesized source effects. We found moderate evidence against an interaction between thinking style (as measured using the CRT) and source. It should be noted that the CRT may not be the optimal tool for establishing intuitive vs. analytical thinking, as it relies solely on reflective capabilities (Pennycook et al., Citation2016) or possibly other hidden skills such as insight and numeracy (Patel, Citation2017). Our study indicated that source credibility is affected by worldview but not by thinking style as measured by the CRT, although this leaves open the possibility that other measures of intuitive thinking might have an effect on source credibility. Interestingly, recent research showed that general trust in science makes people vulnerable to belief in pseudoscience, but that methodological literacy could protect against this (O’Brien et al., Citation2021). The ambiguous statements that were used in our study were seldom rejected with the lowest credibility score, possibly indicative of a general acceptance of pseudo-scientific statements. However, we did not find a significant correlation between belief in science and the credibility ratings of the scientist. In contrast, we found strong evidence against a main effect of astrophysics knowledge on credibility ratings. Although this is different from methodological literacy, it shows that general topical knowledge might not protect against accepting ambiguous statements with scientific jargon. Our additional investigation of bullshit receptivity showed positive correlations with the perceived credibility of the guru and the scientist, which may reflect a general gullibility effect (Pennycook, Cheyne et al., Citation2015). In support of this idea, both belief in science and faith in intuition were positively correlated to receptivity to bullshit (A. Evans et al., Citation2020), while this correlation only replicates in our sample between BSR and supernatural beliefs. The potential discrepancy between our and previous studies could well be related to the sample characteristics and our study may have been underpowered to detect these relatively small to moderate correlations between the different variables that were included. Previous research has also shown that high trust in science may lead to wrongful attribution of relevance to scientific jargon and vice versa that irrelevant scientific content can lead to increased belief in science (O’Brien et al., Citation2021; Weisberg et al., Citation2008, Citation2015). While our stimuli were not specifically designed around scientific terms, the topic of astrophysics and quantum mechanics that was featured in our statements, resulted in a lot of jargon being used. This observation together with our results hint that although jargon may increase credibility, to whom this jargon is attributed is more important in boosting perceived credibility.
The strong negative correlation between supernatural beliefs and belief in science was expected based on previous research (Farias et al., Citation2013; McPhetres et al., Citation2020; McPhetres & Zuckerman, Citation2018; Rutjens & van der Lee, Citation2020), but made it impossible to directly compare these within the same model. Their relationship therefore remains difficult to disentangle, and probably complex and multifaceted (McPhetres and Nguyen, Citation2018), besides often being perceived as purely conflicting.
In the present research, we used a successful implementation of an experimental paradigm evaluating the effect of scientific and supernatural beliefs on source credibility. We also exposed several factors influencing source credibility, which are important for current societal discussions (e.g. COVID-19, vaccines) and may improve our understanding of the evaluation of statements.
Nevertheless, a limitation of the use of these ambiguous statements was that participants sometimes indicated that they felt unintelligent or unable to ground their rating in relation to more contemporary theories and ideas. This might explain why participants mostly used the middle part of the scale and why there was little variability in the ratings. In addition, the lack of incentives (i.e. participants could freely rate without consequences) could have contributed to the scores being centered around the middle of the scale, as there was clearly nothing at stake with agreement or disagreement with the presented statements. Further research could adapt the paradigm with the use of incentives to investigate whether the source credibility effect on the processing of statements holds up when there is more at stake. Higher motivation and engagement generally lead to more balanced decisions and less confirmation bias, which could prompt participants to evaluate the statements more critically (Dawson et al., Citation2002). However, research on climate change and confirmation bias has shown that people tend to stick to their prior beliefs when processing new information (Myers et al., Citation2013; Sambrook et al., Citation2021). Therefore, it is unsure whether adaptations to increase motivation will have any effect. When these are implemented to improve upon our current design, it should be noted that participants may have to be unfamiliar with the topic (as was the case here), otherwise people’s reasoning will mainly depend on their prior beliefs and this might not lead to more critical evaluation (Myers et al., Citation2013). In our context, the astrophysics topic of the ambiguous statements fitted in nicely with the interests of scientific and spiritual-minded people without drawing upon great familiarity with the topic. Because of this and their ambiguous nature, the statements were acceptable and seemingly plausible to people from both a spiritual and a scientific background. Therefore, in combination with the stimuli being incomprehensible and not containing truly factually incorrect information, they likely did not challenge people’s prior worldview. Hence, we could show here the effect of worldviews on credibility ratings.
The current design, using repeated measures in combination with auditory stimuli, is both feasible and valid to test our hypotheses. We found that the source credibility effects were stable over time, indicating that people did not change their mind or became more sceptical over the course of the experiment. Consistent with previous research, we did not notice any issues using auditory stimuli (Stern et al., Citation2006). Furthermore, the use of auditory stimuli allows setting up future research using fMRI. The main goal of such a follow-up study would be to investigate whether the assignment of credibility to a source is processed differently in participants with different worldviews and how different brain areas might contribute to the evaluation of statements and credibility ratings. For example, in an fMRI study, Schjoedt et al. (Citation2011) used auditory stimuli to explore the neural effects of source credibility on believers and non-believers. They found that believers downregulate several prefrontal brain areas in response to statements (prayers) by a trusted source (a charismatic healer). Inspired by predictive processing accounts, they propose that trusted sources may reduce efforts to monitor incoming statements for errors (Schjoedt et al., Citation2013). The fMRI study, however, was exploratory and their interpretations rely heavily on reverse inference. A follow-up study should be carefully designed to both ensure enough power to detect these effects and to avoid reverse inference problems (Poldrack, Citation2011).
Although the current research shows that worldviews influence credibility ratings, it is likely that other factors also drive people to trust a source, which could limit generalization to different sources and situations. Previous research already mentioned for instance, attractiveness (although here both sources were equally attractive; Gheorghiu et al., Citation2017; Patzer, Citation1983) and religiosity (Hoogeveen et al., Citation2022) as factors that could affect source credibility. In the current study, we checked whether astrophysics knowledge, bullshit receptivity and CRT scores affected the credibility ratings, but other factors could come into play as well. Advances towards generalization could be made by including other sources, both with esteemed status in society as well as sources with lower status (e.g. the president vs. a teacher or construction worker), and by using different sources within each group varying on other aspects such as attractiveness. The attribution of credibility to a source may also be affected by the confidence in that attribution (e.g. in Western societies people may be more confident judging the credibility of a scientist than that of a guru). Therefore, the addition of confidence ratings on the rated credibility may also be useful in its generalization. These additions could indicate whether the contribution of worldviews is specific for the currently investigated sources or related to a more general tendency to take source credibility into account. A final step in generalizing to daily life situations would naturally involve also real statements instead of ambiguous statements. However, if people already have prior ideas about daily topics, it complicates the study and design because it is hard to separate the effect of source on the statement from a lifetime of experiences with a specific topic such as climate change (J. H. Evans, Citation2011; Kobayashi, Citation2018; McPhetres & Nguyen, Citation2018).
In conclusion, our findings replicate the Einstein-effect, by showing that information from scientific sources is deemed more credible than from spiritual sources. Furthermore, supernatural and scientific worldviews affect source-credibility effects, highlighting how our prior beliefs shape the processing of information and our understanding of the world.
Contributions
MMvdM designed the experiment, collected the pilot and main data, wrote the manuscript. GJMvdL designed the experiment, collected the pilot and main data, wrote the manuscript. SH designed the experiment, collected main data, analyzed pilot and main data, wrote the manuscript. US designed the experiment. MvE designed the experiment, revised the manuscript.
Supplemental Material
Download Zip (85.2 KB)Disclosure statement
No potential conflict of interest was reported by the author(s).
Supplementary material
Supplemental data for this article can be accessed here
Additional information
Funding
Notes
1. The subscripts on the Bayes factor refer to the hypotheses or models being compared, with the first and second subscript referring to the alternative hypothesis/model of interest and the null hypothesis/model, respectively.
2. For all analyses, we used R (Version 4.0.2; R Core Team, Citation2013) and the R-packages BayesFactor (Version 0.9.12.4.2; Morey and Rouder Citation2018), Bayesrel (Version 0.7.0.3; Pfadt et al. Citation2021), beeswarm (Version 0.2.3; Eklund Citation2016), coda (Version 0.19.4; Plummer et al. Citation2006), dplyr (Version 1.0.5; Wickham et al. Citation2021), Matrix (Version 1.3.2; Bates and Maechler Citation2010), papaja (Version 0.1.0.9997; Aust and Barth Citation2018), qualtRics (Version 3.1.4; Ginn and Silge Citation2021), report (Version 0.3.0; Makowski et al. Citation2021), scales (Version 1.1.1; Wickham and Seidel Citation2020), tinylabels (Version 0.1.0 Barth Citation2020), and wesanderson (Version 0.3.6; Ram and Wickham Citation2018).
3. This is the default “wide” prior scale in the BayesFactor package (Morey & Rouder, Citation2018).
References
- Aust, F., & Barth, M. (2018). papaja: Prepare reproducible apa journal articles with r markdown. (version 0.1.0.9997) https://github.com/crsh/papaja
- Barth, M. (2020). Tinylabels: Lightweight variable labels. (version 0.1.0) https://CRAN.R-project.org/package=tinylabels
- Bates, D., & Maechler, M. (2010). Matrix: Sparse and dense matrix classes and methods. (version 1.3.2) http://cran.r-project.org/package=Matrix
- Bialek, M., & Pennycook, G. (2018). The cognitive reflection test is robust to multiple exposures. Behavior Research Methods, 50(5), 1953–1959. https://doi.org/10.3758/s13428-017-0963-x
- Chaiken, S., & Maheswaran, D. (1994). Heuristic processing can bias systematic processing: Effects of source credibility, argument ambiguity, and task importance on attitude judgment. Journal of Personality and Social Psychology, 66(3), 460–473. https://doi.org/10.1037/0022-3514.66.3.460
- Dawson, E., Gilovich, T., & Regan, D. T. (2002). Motivated reasoning and performance on the was on selection task. Personality & Social Psychology Bulletin, 28(10), 1379–1387. https://doi.org/10.1177/014616702236869
- Eklund, A. (2016). beeswarm: The bee swarm plot, an alternative to stripchart. (version 0.2.3) h ttps.//CRAN.R-project.o rg/package=beeswarm
- Evans, A., Sleegers, W., & Mlakar, Ž. (2020). Individual differences in receptivity to scientific bullshit. Judgment and Decision Making, 15(3), 401–412 http://journal.sjdm.org/20/200221/jdm200221.pdf
- Evans, J. H. (2011). Epistemological and moral conflict between religion and science. Journal for the Scientific Study of Religion, 50(4), 707–727. https://doi.org/10.1111/j.1468-5906.2011.01603.x
- Faircloth, C. (2010). ‘what science says is best’: Parenting practices, scientific authority and maternal identity. Sociological Research Online, 15(4), 85–98. https://doi.org/10.5153/sro.2175
- Farias, M., Newheiser, A.-K., Kahane, G., & de Toledo, Z. (2013). Scientific faith: Belief in science increases in the face of stress and existential anxiety. Journal of Experimental Social Psychology, 49(6), 1210–1213. https://doi.org/10.1016/j.jesp.2013.05.008
- Farias, M., van Mulukom, V., Kahane, G., Kreplin, U., Joyce, A., Soares, P., Oviedo, L., Hernu, M., Rokita, K., Savulescu, J., & Möttönen, R. (2017). Supernatural belief is not modulated by intuitive thinking style or cognitive inhibition. Scientific Reports, 7(1), 1–8. https://doi.org/10.1038/s41598-017-14090-9
- Funk, C., Hefferon, M., Kennedy, B., & Johnson, C. (2019). Trust and mistrust in americans’ views of scientific experts. Pew Research Center. https://www.pewresearch.org/science/2019/08/02/trust-and-mistrust-inamericans-views-of-scientific-experts
- Garrett, B. M., & Cutting, R. L. (2017). Magical beliefs and discriminating science from pseudoscience in undergraduate professional students. Heliyon, 3(11), e00433. https://doi.org/10.1016/j.heliyon.2017.e00433
- Gervais, W. M., van Elk, M., Xygalatas, D., McKay, R. T., Aveyard, M., and Buchtel, E. E., Dar-Nimrod, I., Klocová, E. K., Ramsey, J. E., Riekki, T., Svedholm-Häkkinen, A. M., & Bulbulia, J. A. (2018). Analytic atheism: A cross-culturally weak and fickle phenomenon? Judgment and Decision Making, 13(3), 268-274. http://journal.sjdm.org/18/18228/jdm18228.pdf.
- Gheorghiu, A. I., Callan, M. J., & Skylark, W. J. (2017). Facial appearance affects science communication. Proceedings of the National Academy of Sciences, 114(23), 5970–5975. https://doi.org/10.1073/pnas.1620542114
- Ginn, J., & Silge, J. (2021). Qualtrics: Download ‘qualtrics’ survey data. (version 3.1.4) https://CRAN.R-project.org/package=qualtRics
- Haaf, J. M., & Rouder, J. N. (2017). Developing constraint in Bayesian mixed models. Psychological Methods, 22(4), 779–798. https://doi.org/10.31234/osf.io/ktjnq
- Hoogeveen, S., Haaf, J. M., Bulbulia, J. A., Ross, R. M., McKay, R., Altay, S., and van Elk, M. (2022). The Einstein effect provides global evidence for scientific source credibility effects and the influence of religiosity. Nature Human Behaviour. https://doi.org/10.1038/s41562-021-01273-8
- Kahan, D. M., Jenkins-Smith, H., & Braman, D. (2011). Cultural cognition of scientific consensus. Journal of Risk Research, 14(2), 147–174. https://doi.org/10.1080/13669877.2010.511246
- Kahan, D. M., Peters, E., Wittlin, M., Slovic, P., Ouellette, L. L., Braman, D., & Mandel, G. (2012). The polarizing impact of science literacy and numeracy on perceived climate change risks. Nature Climate Change, 2(10), 732–735. https://doi.org/10.1038/nclimate1547
- Kobayashi, K. (2018). The impact of perceived scientific and social consensus on scientific beliefs. Science Communication, 40(1), 63–88. https://doi.org/10.1177/1075547017748948
- Lachapelle, E., Montpetit, É., & Gauvin, J.-P. (2014). Public perceptions of expert credibility on policy issues: The role of expert framing and political worldviews. Policy Studies Journal, 42(4), 674–697. https://doi.org/10.1111/psj.12073
- Legare, C. H., Evans, E. M., Rosengren, K. S., & Harris, P. L. (2012). The coexistence of natural and supernatural explanations across cultures and development. Child Development, 83(3), 779–793. https://doi.org/10.1111/j.1467-8624.2012.01743.x
- Lindeman, M., & Lipsanen, J. (2016). Diverse cognitive profiles of religious believers and nonbelievers. The International Journal for the Psychology of Religion, 26(3), 185–192. https://doi.org/10.1080/10508619.2015.1091695
- Lindeman, M., & Svedholm, A. M. (2012). What’s in a term? Paranormal, superstitious, magical and supernatural beliefs by any other name would mean the same. Review of General Psychology, 16(3), 241–255. https://doi.org/10.1037/a0027158
- Maij, D. L., van Harreveld, F., Gervais, W., Schrag, Y., Mohr, C., & van Elk, M. (2017). Mentalizing skills do not differentiate believers from non-believers, but credibility enhancing displays do. PloS one, 12(8), e0182764. https://doi.org/10.1371/journal.pone.0182764
- Makowski, D., Ben-Shachar, M. S., Patil, I., & Lüdecke, D. (2021). Automated results reporting as a practical tool to improve reproducibility and methodological best practices adoption. (version 0.3.0).https://github.com/easystats/report
- Mayo, R. (2019). The skeptical (ungullible) mindset. In J. P. Forgas & R. F. Baumeister (Eds.), The Social Psychology of Gullibility: Conspiracy Theories, Fake News and Irrational Beliefs (pp.140-158). Routledge, New York. https://doi.org/10.4324/2F9780429203787-8
- McPhetres, J., Jong, J., & Zuckerman, M. (2020). Religious americans have less positive attitudes toward science, but this does not extend to other cultures. Social Psychological and Personality Science, 12(4), 528-536. https://doi.org/10.1177/1948550620923239.
- McPhetres, J., & Nguyen, T.-V. T. (2018). Using findings from the cognitive science of religion to understand current conflicts between religious and scientific ideologies. Religion, Brain & Behavior, 8(4), 394–405. https://doi.org/10.1080/2153599X.2017.1326399
- McPhetres, J., & Zuckerman, M. (2017). Religious people endorse different standards of evidence when evaluating religious versus scientific claims. Social Psychological and Behavior Personality Science, 8(7), 836–842. https://doi.org/10.1177/1948550617691098
- McPhetres, J., & Zuckerman, M. (2018). Religiosity predicts negative attitudes towards science and lower levels of science literacy. PloS one, 13(11), e0207125. https://doi.org/10.1371/journal.pone.0207125
- Morey, R. D., & Rouder, J. N. (2018). Bayesfactor: Computation of Bayes factors for common designs. (version 0.9.12.4.2) https://CRAN.R-project.org/package=BayesFactor
- Myers, T. A., Maibach, E. W., Roser-Renouf, C., Akerlof, K., & Leiserowitz, A. A. (2013). The relationship between personal experience and belief in the reality of global warming. Nature Climate Change, 3(4), 343–347. https://doi.org/10.1038/nclimate1754
- O’Brien, T. C., Palmer, R., & Albarracin, D. (2021). Misplaced trust: When trust in science fosters belief in pseudoscience and the benefits of critical evaluation. Journal of Experimental Social Psychology, 96, 104184. https://doi.org/10.1016/j.jesp.2021.104184
- Patel, N. (2017). The cognitive reflection test: A measure of intuition/reflection, numeracy, and insight problem solving, and the implications for understanding real-world judgments and beliefs. University of Missouri-Columbia.
- Patzer, G. L. (1983). Source credibility as a function of communicator physical attractiveness. Journal of Business Research, 11(2), 229–241. https://doi.org/10.1016/0148-2963(83)90030-9
- Pennycook, G., Cheyne, J. A., Barr, N., Koehler, D. J., & Fugelsang, J. A. (2015). On the reception and detection of pseudo-profound bullshit. Judgment and Decision Making, 10(6), 549–563. http://journal.sjdm.org/15/15923a/jdm15923a.pdf
- Pennycook, G., Cheyne, J. A., Koehler, D. J., & Fugelsang, J. A. (2016). Is the cognitive reflection test a measure of both reflection and intuition? Behavior Research Methods, 48(1), 341–348. https://doi.org/10.3758/s13428-015-0576-1
- Pennycook, G., Cheyne, J. A., Seli, P., Koehler, D. J., & Fugelsang, J. A. (2012). Analytic cognitive style predicts religious and paranormal belief. Cognition, 123(3), 335–346. https://doi.org/10.1016/j.cognition.2012.03.003
- Pennycook, G., Fugelsang, J. A., & Koehler, D. J. (2015). Everyday consequences of analytic thinking. Current Directions in Psychological Science, 24(6), 425–432. https://doi.org/10.1177/0963721415604610
- Pennycook, G., McPhetres, J., Zhang, Y., Lu, J. G., & Rand, D. G. (2020). Fighting covid-19 misinformation on social media: Experimental evidence for a scalable accuracy-nudge intervention. Psychological Science, 31(7), 770–780. https://doi.org/10.1177/0956797620939054
- Petty, R. E., & Cacioppo, J. T. (1986). The elaboration likelihood model of persuasion. In R. E. Petty & J. T. Cacioppo (Eds.), Communication and persuasion (pp. 1–24). Springer, New York.
- Pfadt, J. M., van den Bergh, D., & Goosen, J. (2021). Bayesrel: Bayesian reliability estimation. (version0.7.0.3) https://CRAN.R-project.org/package=Bayesrel
- Plummer, M., Best, N., Cowles, K., & Vines, K. (2006). Coda: Convergence diagnosis893and output analysis for mcmc. (version0.19.4) https://journal.r-project.org/archive/
- Poldrack, R. A. (2011). Inferring mental states from neuroimaging data: From reverse inference to large-scale decoding. Neuron, 72(5), 692–697. https://doi.org/10.1016/j.neuron.2011.11.001
- Pornpitakpan, C. (2004). The persuasiveness of source credibility: A critical review of five decades’ evidence. Journal of Applied Social Psychology, 34(2), 243–281. https://doi.org/10.1111/j.1559-1816.2004.tb02547.x
- R Core Team (2013). R: A language and environment for statistical computing. (version 4.0.2) https://www.R-project.org/
- Ram, K., & Wickham, H. (2018). Wesanderson: A wes anderson palette generator. (version 0.3.6) https://journal.r-project.org/archive/
- Randall, T. M., & Desrosiers, M. (1980). Measurement of supernatural belief: Sex differences and locus of control. Journal of Personality Assessment, 44(5), 493–498. https://doi.org/10.1207/s15327752jpa4405_9
- Roberts, C. (2010). Correlations among variables in message and messenger credibility scales. American Behavioral Scientist, 54(1), 43–56. https://doi.org/10.1177/0002764210376310
- Rouder, J. N. (2014). Optional stopping: No problem for Bayesians. Psychonomic Bulletin & Review, 21(2), 301–308. https://doi.org/10.3758/s13423-014-0595-4
- Rouder, J. N., Haaf, J. M., Davis-Stober, C. P., & Hilgard, J. (2019). Beyond overall effects: A Bayesian approach to finding constraints in meta-analysis. Psychological Methods, 24(5), 606–621. https://doi.org/10.1037/met0000216
- Rouder, J. N., Morey, R. D., Speckman, P. L., & Province, J. M. (2012). Default Bayes factors for ANOVA designs. Journal of Mathematical Psychology, 56(5), 356–374. https://doi.org/10.1016/J.JMP.2012.08.001
- Rutjens, B. T., Sutton, R. M., & van der Lee, R. (2018). Not all skepticism is equal: Exploring the ideological antecedents of science acceptance and rejection. Personality & Social Psychology Bulletin, 44(3), 384–405. https://doi.org/10.1177/0146167217741314
- Rutjens, B. T., & van der Lee, R. (2020). Spiritual skepticism? Heterogeneous science skepticism in the Netherlands. Public Understanding of Science, 29(3), 335–352. https://doi.org/10.1177/0963662520908534
- Sambrook, K., Konstantinidis, E., Russell, S., & Okan, Y. (2021). The role of personal experience and prior beliefs in shaping climate change perceptions: A narrative review. Frontiers in Psychology, 12, 2679. https://doi.org/10.3389/fpsyg.2021.669911
- Schjoedt, U., Sørensen, J., Nielbo, K. L., Xygalatas, D., Mitkidis, P., & Bulbulia, J. (2013). Cognitive resource depletion in religious interactions. Religion, Brain & Behavior, 3(1), 39–55. https://doi.org/10.1080/2153599X.2012.736714
- Schjoedt, U., Stødkilde-Jørgensen, H., Geertz, A. W., Lund, T. E., & Roepstorff, A. (2011). The power of charisma—perceived charisma inhibits the frontal executive network of believers in intercessory prayer. Social Cognitive and Affective Neuroscience, 6(1), 119–127. https://doi.org/10.1093/scan/nsq023
- Schönbrodt, F. D., Wagenmakers, E.-J., Zehetleitner, M., & Perugini, M. (2017). Sequential hypothesis testing with Bayes factors: Efficiently testing mean differences. Psychological Methods, 22(2), 322–339. https://doi.org/10.1037/met0000061
- Stern, S. E., Mullennix, J. W., & Yaroslavsky, I. (2006). Persuasion and social perception of human vs. synthetic voice across person as source and computer as source conditions. International Journal of Human-Computer Studies, 64(1), 43–52. https://doi.org/10.1016/j.ijhcs.2005.07.002
- Taves, A., Asprem, E., & Ihm, E. (2018). Psychology, meaning making, and the study of worldviews: Beyond religion and non-religion. Psychology of Religion and Spirituality, 10(3), 207. https://doi.org/10.1037/rel0000201
- Thomson, K. S., & Oppenheimer, D. M. (2016). Investigating an alternate form of the cognitive reflection test. Judgment and Decision Making, 11(1), 99. http://journal.sjdm.org/15/151029/jdm151029.pdf
- Umeogu, B. (2012). Source credibility: A philosophical analysis. Open Journal of Philosophy, 2(2), 112. https://doi.org/10.4236/ojpp.2012.22017
- van Elk, M., & Snoek, L. (2020). The relationship between individual differences in gray matter volume and religiosity and mystical experiences: A preregistered voxel-based morphometry study. European Journal of Neuroscience, 51(3), 850–865. https://doi.org/10.1111/ejn.14563
- Wagenmakers, E.-J., Marsman, M., Jamil, T., Ly, A., Verhagen, J., Love, J., Selker, R., Gronau, Q. F., Šmíra, M., Epskamp, S., Matzke, D., Rouder, J. N., & Morey, R. D. (2018). Bayesian inference for psychology. Part I: Theoretical advantages and practical ramifications. Psychonomic Bulletin & Review, 25(1), 35–57. https://doi.org/10.3758/s13423-017-1343-3
- Weisberg, D. S., Keil, F. C., Goodstein, J., Rawson, E., & Gray, J. R. (2008). The seductive allure of neuroscience explanations. Journal of Cognitive Neuroscience, 20(3), 470–477. https://doi.org/10.1162/jocn.2008.20040
- Weisberg, D. S., Taylor, J. C., & Hopkins, E. J. (2015). Deconstructing the seductive allure of neuroscience explanations. Judgment and Decision Making, 10(5), 429. http://journal.sjdm.org/15/15731a/jdm15731a.pdf
- Wickham, H., François, R., Henry, L., & Müller, K. (2021). Dplyr: A grammar of data manipulation. (version 1.0.5) https://CRAN.R-project.org/package=dplyr
- Wickham, H., & Seidel, D. (2020). Scales: Scale functions for visualization. (version 1.1.1) https://CRAN.R-project.org/package=scales