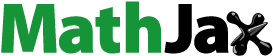
Abstract
Artificial intelligence (AI) can be used to support intelligent and sustainable mobility solutions. For AI to be functional, it must be supplied with reliable data. With the continuous expansion of the data base for AI, it can fill data gaps based on learning effects and thus increase data quality. The electric Highway (eHighway) system as a sustainable mobility solution for long-distance road freight transport is a megaproject where the use of AI can be helpful. As a case study for this paper, the research project ELISA (‘ELektrifizierter, Innovativer Schwerverkehr auf Autobahnen’ = ‘electrified, innovative road freight transport on motorways’) was chosen in which the eHighway has been tested on a 10 km test track in Hesse (Germany) for about 2.5 years. The data on overhead line hybrid trucks and overhead line infrastructure obtained from the project was analysed in terms of their availability and combined to the overall availability of the eHighway system. These results provided the basis for a subsequent SWOT analysis to evaluate the integrability of AI in the eHighway system. The findings from the SWOT analysis show that with the continuous improvement of data availability and quality, the use of AI in the eHighway system is feasible. Energy, cost, and operational improvements in the eHighway system are expected through the use of AI.
Introduction
Intelligent mobility has been proposed as a key approach towards sustainability under the assumption that the more data we have and the better we analyse them, the better decisions we will be able to make. Thus, a key to enabling artificial intelligence (AI) systems is to supply them with big data, that is consistently available, reliably produced with little to no interruptions.
One of the key intelligent mobility solutions is an electric Highway (eHighway) system, that significantly reduces greenhouse gas emissions. The eHighway system combines an ‘overhead line infrastructure’ (OL infrastructure) and an ‘overhead line hybrid truck’ (OH truck). The OH truck receives energy through a pantograph on the driver’s cabin, connecting the overhead line with an onboard battery. The truck uses the battery’s energy once it leaves the section of the OL infrastructure and, if the battery ever empties, a conventional combustion engine (Boltze et al. Citation2020; Schöpp et al. Citation2021). Aspirational goals intend to achieve an eHighway network across the globe that with the help of AI solutions minimizes or erases the need for fossil fuels. This paper analyses a German pilot project of an eHighway called ELISA (‘ELektrifizierter, Innovativer Schwerverkehr auf Autobahnen’ = ‘electrified, innovative road freight transport on motorways’) funded by the German Federal Ministry for Economic Affairs and Climate Action (until December 2021: German Federal Ministry for the Environment, Nature Conservation and Nuclear Safety). We assess ELISA’s ability to produce the availability and reliability of data needed for a sustainable artificially intelligent megaproject.
We contribute to the discussion on data-driven solutions and the decision-making ability of humans with novel technology to get us towards a sustainable future. We find that ELISA possesses the potential to become a sustainable, resilient and artificially-intelligent megaproject if data availability and reliability can be ensured and humans remain in decision-making power when major changes are made to the technology.
We further argue that the new mobility concepts will evolve in the process of its technological development, which must be considered in the data-driven assessment by enabling AI solutions of the future.
Literature review
Data-driven solutions evolved from total quality management, in which decisions were made by data and not by reasoning, experience, or intuition. Data-driven solutions can handle, control, process and analyse existing data to figure out different humankind problems. In engineering fields, numerous researches used data-driven techniques like Kirchdoerfer and Ortiz (Citation2016) or Abduljabbar et al. (Citation2019), who contributed to improving efficiency and reliability of systems, like Vinuesa et al. (Citation2020) or Iyer (Citation2021), who contributed to sustainability, like Ritchie (Citation1990), who contributed to real-time management systems, like Kassens-Noor et al. (Citation2021), who contributed to autonomous systems, or like Kişi and Öztürk (Citation2007), who contributed to forecasting.
Data-driven systems evolved parallel to improvements in AI, from the preliminary application of data systems through the most recent deep learning techniques. First time AI terms came up with a project proposal by McCarthy et al. in 1955 (John McCarthy et al. Citation2006). McCarthy and Hayes stated philosophical AI questions regarding observations, representation of the physical world, and knowledge (McCarthy and Hayes Citation1981). In the late 1960s, Herbert Simon and Marvin Minsky emphasized separately that the high probability of representing human ability by artificial intelligence exists after a generation (Simon Citation1965; Minsky Citation1967). In the early 1970s, Chamberlin and Boyce developed Structured Query Language to access and handle data efficiently (Chamberlin and Boyce Citation1976; Chamberlin Citation2012). In the 1980s, the development of ‘expert systems’, a computer system with a capability of imitation in decision making, was an important milestone since it is ‘the first truly successful’ application of AI technology (Jackson Citation1986; Russell and Norvig Citation1995). Data-driven solution studies couldn’t keep a high interest between the 1970s and the 2000s. AI researchers used the term ‘AI Winter’ for a pessimistic period in the 1970s with low funds and low interest. Disappointment and criticism related to high expectations resulted in funding cuts. Failure of a machine translation project, the collapse of the LISP machine market or the unsuccessful ending of the Fifth Generation Project had an adverse impact on AI studies. Against negative myths about AI, Rodney Brooks (Kurzweil Citation2005) stated the importance of AI as “AI is around you every second of the day.” AI term is known in the world by AI-based solutions in different areas. Since AI technology is data-dependent, a high amount of data collection started from different sources. Big data was mentioned first time in the literature in 2001 and an asymptotic interest in research was observed by improvement in technology (Tang et al. Citation2022). To handle and analyse such an amount of data, supercomputers and new techniques were developed.
One of the main concerns for data-driven solutions is that reliable, sustainable decisions may not come out from the data themselves, even if we use a robust data-driven process (Kahneman and Tversky Citation2012). Data-driven solutions by using AI require a comprehensive understanding of human decision-making. If a task is programmed for each possible scenario, then it is not a decision anymore but a certain action. However, decisions for uncertain cases are not stable when using human decision-making mechanisms. Correspondingly, difficulties for AI-based decisions are defined as “uncertainty of the future” and “granularity of the decision” (Pomerol Citation1997; Pomerol and Adam Citation2008). Three challenges in decision making are uncertainty, complexity, and equivocality (Jarrahi Citation2018). Decisions from AI-based solutions are not certain for unexpected cases or scenarios not defined by the designer. In addition, ethics in data-driven solutions is an issue for objective decision making since the decision maker may be biased when designing a data-driven system.
AI based solutions appear frequently in numerous subsections of the transportation systems. Traffic incident detection (Dia and Rose Citation1997; Wang, Fan, and Work Citation2016), predictive traffic models (Ledoux Citation1997) are AI application samples for planning, designing and controlling of transportation systems. Moreover, AI based approaches are used in aviation, shared mobility, public transit, urban mobility and autonomous technologies (Abduljabbar et al. Citation2019). However, the enormous progress observed recently in AI based application techniques in transportation area, some concerns are not figured out totally yet. Human-based information dependency, safety, precise forecasting for unexpected conditions are such limitations (Karlaftis et al. Citation2012; Vlahogianni, Karlaftis, and Golias Citation2014).
Transportation systems rely on data acquisition from both mechanical and human sources. Data propagation from varied sources brings about a management problem for a huge cloud by using human-skill. In this regard, data-driven solutions may help the evaluation of a proposed system by the capability of handling big data produced from varied sources. Real-time management of the data and analysis results from different aspects ensure with a minimum risk and error. Moreover, data issues like availability, quality management, calibration, validation, or data loss are possible by the power of data-driven solutions. Data provided by mechanical and human sources in transportation systems should be accurate and reliable. Disruptions in data collection and data quality issues carry out inefficiency for operations. Therefore, AI based solutions in transportation system is essential to perform data collection, data mining, decision making, and optimization. In this manner, implementation of AI-based data-driven solutions may provide better management of megaprojects like ELISA.
Methods
eHighway case study
As our case study, we chose ELISA. The pilot test ELISA is located in Germany’s Federal State of Hesse. The eHighway section is a 5 km long North and South stretch on highway A5 between Frankfurt am Main and Darmstadt (Boltze Citation2020). The highway stretch is equipped with an OL infrastructure. The pilot test phase started on 7 May 2019 and the accompanying research project will at least last until the end of 2022. In 2019, two OH trucks started operation. Another three OH trucks are testing the tracks since mid-2020. Similar OH trucks were tested in smaller field trials (Siemens Citation2012; Siemens AG, Technische Universität Dresden, and Deutsches Zentrum für Luft- und Raumfahrt e. V. Citation2016) before the German government chose stretches on public roads to test the eHighway system. The OH truck and associated eHighway are in their pilot testing phase and still under development. As a German field trial, the technology is rated at readiness level 7 (Boltze et al. Citation2021). Thus, the Hessian field trial is the first eHighway field trial under real-world conditions (Boltze Citation2020).
Analysis structure and data sources
We determined the availability of the eHighway system ELISA based on the definition of availability by Eberlin and Hock (Citation2014, 69) as: ‘The availability is the ratio of the time in which the system is functional to the total time’. We have divided it into three components: the OL infrastructure, the OH truck, and the data sources. For each of these components, we have independently calculated the availability using different methods. For the final determination of the availability of the eHighway system, we merged the results, see .
For the two components OL infrastructure and OH truck, data is collected automatically through different data logger systems. However, as in any system of automated data collection, unplanned data collection failures are possible. Therefore, we established further documentation strategies to ensure the quality of the OH truck data and the OL infrastructure data.
Method to determine the availability of the OL infrastructure
Because the ELISA OL infrastructure is the first of its kind in real-world operation, we defined two operation periods based on our documentation to analyse the availability of the OL infrastructure data and the availability of the OL infrastructure:
Introduction phase of the OL infrastructure (May 7, 2019 – December 31, 2019)
Regular operation of the OL infrastructure (January 1, 2020 – June 30, 2021)
During the introduction phase, ELISA was available to users on weekdays from 8:00 a.m. to 4:00 p.m. Specially trained engineers of the OL infrastructure operator managed the shutdowns and all incident management-related tasks during the introductory phase. The introduction phase began with the start of operations. During regular operations, the system became available to users 24/7, i.e. operation 24 hours a day, seven days a week. In February 2020, an expansion of the OL infrastructure took place in order to be able to switch the respective directions of travel (south direction to Darmstadt and north direction to Frankfurt) on and off separately.
Interruptions of operations can be planned and unplanned. A system operator can expect planned interruptions and can prepare for the shutdown of the system. Examples of planned interruptions are inspections, maintenance, and other works complying with the system’s German standards. Unplanned interruptions are short notice, such as crashes, and can be requested by hazard prevention authorities, such as the fire brigades or the police. Other emergency events can also cause the OL infrastructure operator to interrupt operations. For example, a disconnect between the system and its control center requires the reestablishment of safety-relevant processes.
The OL infrastructure operator documented all planned and unplanned interruptions. Moreover, data was collected within the substations. Both data sources combined in an OL infrastructure diary served as the basis for determining the availability of the OL infrastructure. The OL infrastructure diary noted the operating times (switching on and off) and the reasons for doing so. The availability of the OL infrastructure AOL is determined by the observed operating time tO compared to the expected operating time tE. The expected operating time depends on the evaluation period. Separate availability values are determined for the respective directions of travel.
Method to determine the availability of the OH truck
The OH trucks drove on the eHighway daily with a total of 362.754 kilometres (Status: March 2021) (Schöpp et al. Citation2021). As a research partner, we continuously accompanied and documented the OH truck operation. We found that during the operation of the OH trucks both planned or unplanned vehicle failures occurred. Planned breakdowns include days on which the vehicle had to go to the workshop for regular maintenance or inspection. Unplanned breakdowns include days on which the vehicle had to be taken to the workshop at short notice, e.g. for a few hours or even for several days, due to unexpected defects. In addition to the unplanned and planned downtime, there is also the option of special operation of the OH truck, such as press runs or research runs, due to the research project.
Each OH truck is equipped with a data logger. Whenever the truck is operated the logger generates data which is automatically uploaded to a data server. However, the dataloggers were not working reliably, thus we came up with additional documentation strategies for the OH truck operation. First, based on the information provided to the project by the vehicle manufacturer and the evaluations of the vehicle data, a vehicle operation diary was kept for each OH truck. Second, we interviewed the OH truck drivers weekly focusing on conspicuous incidents with the OH truck. Starting in March 2020, a weekly phone interview with the regular drivers of the OH trucks explored abnormalities that might have led to interruptions. The key questions posed were:
What peculiarities or technical incidents did you have during the OH truck operation this week?
Were there major events along the route?
Are there any other topics you would like to discuss?
The experiences of the drivers were documented by means of meeting minutes. Important events and feedback from the drivers were summarized in a structured manner and then analysed. The evaluation was based on the following criteria:
Long-term, cross-vehicle failures in-vehicle operation
Temporary errors in connection with the vehicle drive
Temporary errors in connection with the pantograph operation
The evaluation of these internal documents forms the basis of our evaluation. We preliminary measured the number of planned and unplanned interruptions and the subsequent availability of the system over time for the five OH-trucks currently in use.
Based on the definition by Eberlin and Hock (Citation2014, 69), we deducted the following equation for the availability of an OH truck
.
.
Method to determine the availability of the eHighway system
The availability of the overall eHighway system combines the availability of the OL infrastructure, the OH trucks, and the associated data. To account for the operational phases of the system as well as the successive delivery of the OH trucks in 2019 and 2020, we defined different phases for the analysis.
To determine the availability of the overall eHighway system, we combined the availabilities of the individual components on a daily basis in several steps to identify the impact of the three components on the overall availability of the eHighway. First, we checked how often all five OH trucks were available at the same time. In the next step, we combined this intermediate result for the five OH trucks with the availability of the OL infrastructure. Then, we determined the combination of the availability of the five OH trucks, the OL infrastructure, and the vehicle data to get the total availability of the eHighway system.
The following equations exemplify the calculation of the overall availability of the eHighway system considering the availability of the OL infrastructure, the five OH trucks, and the associated data.
Results of the analysis of data-driven ELISA
To foreshadow our finding, the data availability of the OL infrastructure as well as the OL infrastructure availability is very high over the entire considered period. Currently, vehicle availability and vehicle data availability lead to limitations in the deployment options of an AI.
Availability of the overhead line infrastructure
The availability of the OL infrastructure was determined on a monthly basis using the available data source. Verification of OL infrastructure data availability showed that data was reliably recorded over the entire considered period.
shows the availability over the evaluation periods as well as separated by direction of travel. During the introduction phase, the largest share of interruptions is caused by limited operating capabilities of the OL infrastructure, e.g. on public holidays and bridge days. Additionally, the OL infrastructure was temporarily shut down for about two weeks due to storm damage in August 2019 and for about one week due to planned remaining work in June 2019. Unplanned interruptions (n = 28) in the introductory phase occurred twice as often as planned ones (n = 12). In the spring of 2020, there was a prolonged shut down of the OL infrastructure in the southbound direction due to crash-based catenary damage and aggravated conditions caused by the COVID pandemic. The responsibilities for operating the OL infrastructure were redefined in January 2021, which results in a downtime of approximately two weeks. Damage to the overhead line isolators led to a shutdown in March and April 2021.
Figure 2. Availability over time of the OL infrastructure separated by direction of travel. (IVV 2022).
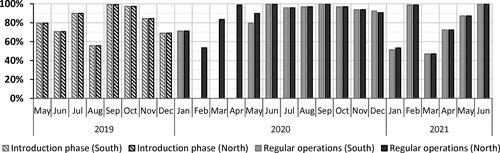
During regular operations, 26 unplanned and 17 planned interruptions occurred. But we observed a learning curve for the security authorities in charge of shutting down the system: while in 2020, 1.17 shutdowns per month occurred, in 2021 only 0.5 shutdowns per month occurred for the same reasons. Whether this effect is coincidental (fewer accidents and incidents) or indeed a learning curve by hazard prevention authorities with the system (operational interruptions are no longer requested for all incidents) will be further monitored and assessed.
Availability of the overhead line hybrid truck
Overall, the evaluations show that on average all OH trucks were operated regularly without major interruptions between 49% to 81% of the time. The reasons for the reduction in regular operating time were, planned interruptions, including regular maintenance, inspections, and routine repairs and unplanned interruptions including vehicle failures. provides an overview of the averaged availability values of each OH truck.
Table 1. Average availability of OH trucks during their observation periods (IVV 2021).
OH truck El Salto (the latest addition to the ELISA eHighway system) has the highest availability with 81% over the entire operating period (June 2020 to March 2021). This might suggest that the vehicle manufacturer improved its performance during real operations, increasing El Salto’s availability. In comparison, the availability of El Fondo ranks the lowest with only 49%, due to more frequent unplanned vehicle breakdowns. The availability of the other three OH trucks ranges between the availability values of El Fondo and El Salto. In addition, we have observed that the availability of OH trucks varies greatly, and the reasons for vehicle failures are often very different because they are prototypes.
Availability of the eHighway system
When analysing the availability of the eHighway system we found that the OL infrastructure and its associated data shows the highest availability because data collection of energy quantities and system conditions is common practice among energy suppliers. Concurrently, the vehicle data is the greatest limitation of overall availability. Due to the field test character of the project, failures of the vehicle data loggers prevented data recording. For the overall availability of the eHighway system, we defined the assumption that all five OH trucks, the OL infrastructure, and the data must be available 100% of the time so that AI can optimize the eHighway operation. The results show that the continuous recording of vehicle data is currently the weak point of the system. Reducing the number of OH trucks for the estimation of the OH truck data availability has a positive effect on the overall data availability.
It is important to note that the overall system availability as described in this study does not represent the availability of a future rolled out eHighway system for Germany, but is an evaluation that determines an idealized data basis to evaluate to what extent AI could be applied in the ELISA project. The results of the SWOT analysis presented below highlight this context.
Results on a SWOT analysis for a sustainable and artificially-intelligent
ELISA is a sustainable and resilient megaproject of the future that once built out, will require smart and data-driven solutions to optimize its operation and management. Based on a preliminary study of pilot data, the potential to introduce AI is great. Although we caution against too early implementation while humans are better capable of detecting irregularities and interpreting outliers in the system to minimize unplanned interruptions. As the project evolves, data availability and reliability of the eHighway system are likely to improve, and implementing AI solutions should be considered. This SWOT analysis aids decision-makers on when, how, and why an AI should be introduced once a rolled-out eHighway system for Germany is functional.
Strengths
The frequency, duration, and impact of planned interruptions can be reduced with AI. The measured availability of the prototype OH trucks is not representative of future OH truck generations. As the prototypes turn mainstream, the availability of OH trucks will significantly improve reducing the scheduled maintenance and inspection; vehicle operation is expected to stabilize, and unplanned outages will similarly occur less frequently.
OL infrastructure operators are continuously learning thereby building a database for AI to determine necessary shutdowns, no matter whether they are planned or unplanned. As these incidences are documented, weather data is integrated, and the eHighway system is connected to its surroundings, AI’s ability to predict unplanned events increases.
Given AI’s ability to scan its environment via sensors installed in the ELISA eHighway system, cost, and energy savings are expected as it is able to automatically shut down the OH infrastructure when it is not expected to be used.
As data availability grows with additional trucks operating on the system, other modes, such as electric buses joining, and various road users starting to use connected vehicles, data availability increases to a point in which real-time management and information sharing will exceed human capabilities.
Weaknesses
AI’s weakness to detect outliers will remain as their data sources are largely based on history. Given unplanned interruptions were twice as common as planned ones with ELISA, humans remain a key to steering the pilot project and enabling safe operations.
To enable AI on ELISA. Further sensors and hardware will need to be installed which is not only costly but also opens up the system to additional fallacies due to sensor or hardware fallacies. Non-operational hardware will result in a permanent loss of data.
It will be increasingly difficult to validate AI data, given the many data-driven solutions needed for AI.
Opportunities
An artificially intelligent ELISA opens up the holistic integration of further data sources like weather data, traffic volumes, and the availability of charging stations. Combined, life cycle management will enable improved energy management and battery usage, battery charging capabilities, and battery lifetime.
Given the wealth of data useful to optimize operations, data-driven solutions should come to the forefront of decision-making for the ELISA system to enable holistic integration with its surroundings. Mistakes humans made will be likely minimized.
Threats
Given AI uses historic data, the system will be slow in changing its course and detect new trends. AI's weakness in filling data gaps for rare circumstances and exceptions will remain.
The more sources are added over time, it might create shocks to the future AI system until more unreliable and incorrectly validated data continuously enter the data stream for AI to use.
As AI makes the system more efficient, additional road users will drive the eHighway and constitute unknowns in the AI system, unless they are also connected vehicles that are capable of avoiding crashes that could pose a vulnerability to the operating system.
Especially in Germany, data privacy and cybersecurity might put hurdles in the deployment of an artificially intelligent ELISA.
Conclusion
Data-driven solutions can be used to analyse a system that produces and processes large amounts of data. However, data-driven solutions require humans to integrate changing conditions, since the system itself cannot learn. An AI works differently. The prerequisite of using an AI is high data availability and data reliability.
In the Hessian field trial ELISA, we are currently working with large amounts of data to which we apply data-based solutions that are influenced by human decisions. However, the data evaluation within ELISA can be replaced by AI. As part of the SWOT analysis, we found that a particular strength of AI in ELISA is that it will be able to predict unplannable events in the future due to its learning processes, as well as enable high energy and cost savings through its use.
At present, humans cannot yet be replaced by AI in the eHighway system due to its prototype status. The results on different component availabilities show that new unpredictable incidents occur frequently especially to OH trucks and the corresponding data, which would be unknown to AI. In addition, currently, not all necessary data sources are available in an appropriate form for the use of AI. However, with the possible expansion of the eHighway system and thus the extension of the OL infrastructure and the increase in the number of OH trucks, the data availability will be increased. Moreover, from that point on, humans will no longer be able to process the data and automated data processing that also learns from the data will inevitably become necessary.
This paper provides the first glimpse into the possibilities of applying AI in the eHighway system. As the field test expands and new insights are gained, the SWOT analysis can also be expanded. By continuously monitoring the quality of the data, future research can estimate when and how AI can be integrated into the eHighway system.
Authors contributions
The authors confirm contribution to the paper as follows:
Introduction: R. Linke, J. K. Wilke, Ö. Öztürk, F. Schöpp, E. Kassens-Noor;
Literature review: Ö. Öztürk;
Methods: R. Linke, J. K. Wilke;
Results: R. Linke, J. K. Wilke, F. Schöpp, Ö. Öztürk, E. Kassens-Noor;
Conclusion: R. Linke, J. K. Wilke, F. Schöpp, Ö. Öztürk.
Paper revision: R. Linke, Ö. Öztürk, E. Kassens-Noor
All authors reviewed the results and approved the final version of the manuscript.
Disclosure statement
No potential conflict of interest was reported by the author(s).
Additional information
Funding
References
- Abduljabbar, Rusul, Hussein Dia, Sohani Liyanage, and Saeed Asadi Bagloee. 2019. “Applications of Artificial Intelligence in Transport: An Overview.” Sustainability 11 (1): 189. https://doi.org/10.3390/su11010189.
- Boltze, Manfred. 2020. “EHighway – an Infrastructure for Sustainable Road Freight Transport.” In CIGOS 2019, Innovation for Sustainable Infrastructure, edited by Cuong Ha-Minh, Dong Van Dao, Farid Benboudjema, Sybil Derrible, Dat Vu Khoa Huynh, and Anh Minh Tang, 35–44. Lecture Notes in Civil Engineering. Singapore: Springer. https://doi.org/10.1007/978-981-15-0802-8_4.
- Boltze, Manfred, Michael Lehmann, Gerd Riegelhuth, Holger Sommer, and Danny Wauri, eds. 2021. Elektrifizierung Von Autobahnen Für Den Schwerverkehr: Umsetzung Des System eHighway. Bonn: Kirschbaum Verlag.
- Boltze, Manfred, Regina Linke, Ferdinand Schöpp, Jürgen. K. Wilke, Özgür Öztürk, and Danny Wauri. 2020. “Insights into the Operation of Overhead Line Hybrid Trucks on the ELISA Test Track.” Presented at 4th Electric Raod Systems Conference, 2020. In ERSC2020 Abstract Book 6. p. 20. Accessed 9 July 2020. https://www.electricroads.org/wp-content/uploads/2020/05/ERSC2020-Abstract-Book-Publ.pdf
- Chamberlin, Donald. D. 2012. “Early History of SQL.” IEEE Annals of the History of Computing 34 (4): 78–82. https://doi.org/10.1109/MAHC.2012.61.
- Chamberlin, Donald. D, and Raymond. F. Boyce. 1976. “SEQUEL: A Structured English Query Language.” In Proceedings of the 1976 ACM SIGFIDET (Now SIGMOD) Workshop on Data Description, Access and Control - FIDET ’76, 249–64. ACM Press. https://doi.org/10.1145/800296.811515.
- Dia, Hussein, and Geoff Rose. 1997. “Development and Evaluation of Neural Network Freeway Incident Detection Models Using Field Data.” Transportation Research Part C: Emerging Technologies 5 (5): 313–331. https://doi.org/10.1016/S0968-090X(97)00016-8.
- Eberlin, Stefan, and Barbara Hock. 2014. Zuverlässigkeit Und Verfügbarkeit Technischer Systeme. Wiesbaden: Springer Fachmedien Wiesbaden. https://doi.org/10.1007/978-3-658-03573-0.
- Iyer, Lakshmi Shankar. 2021. “AI Enabled Applications towards Intelligent Transportation.” Transportation Engineering 5 (September): 100083. https://doi.org/10.1016/j.treng.2021.100083.
- Jackson, P. 1986. ‘Introduction to Expert Systems’, January. https://www.osti.gov/biblio/5675197.
- Jarrahi, Mohammad Hossein. 2018. “Artificial Intelligence and the Future of Work: Human-AI Symbiosis in Organizational Decision Making.” Business Horizons 61 (4): 577–586. https://doi.org/10.1016/j.bushor.2018.03.007.
- Kahneman, Daniel, and Amos Tversky. 2012. “Prospect Theory: An Analysis of Decision under Risk.” In Handbook of the Fundamentals of Financial Decision Making, Volume 4:99–127. World Scientific Handbook in Financial Economics Series, Volume 4. Singapore: WORLD SCIENTIFIC. https://doi.org/10.1142/9789814417358_0006.
- Karlaftis, M., Said Easa, Manoj Jha, and Eleni Vlahogianni. 2012. ‘Design and Construction of Transportation Infrastructure. http://Onlinepubs.Trb.Org/Onlinepubs/Circulars/Ec168.Pdf’. In, 121–33.
- Kassens-Noor, Eva., Mark Wilson, Zeenat Kotval-Karamchandani, Meng Cai, and Travis Decaminada. 2021. “Living with Autonomy: Public Perceptions of an AI-Mediated Future.” Journal of Planning Education and Research January: 0739456X2098452. https://doi.org/10.1177/0739456X20984529.
- Kirchdoerfer, T, and M. Ortiz. 2016. “Data-Driven Computational Mechanics.” Computer Methods in Applied Mechanics and Engineering 304 (June): 81–101. https://doi.org/10.1016/j.cma.2016.02.001.
- Kişi, Özgür, and Özgür Öztürk. 2007. “Adaptive Neurofuzzy Computing Technique for Evapotranspiration Estimation.” Journal of Irrigation and Drainage Engineering 133 (4): 368–379. https://doi.org/10.1061/(ASCE)0733-9437(2007)133:4(368).
- Kurzweil, Ray. 2005. The Singularity is near: When Humans Transcend Biology. New York: Viking Penguin.
- Ledoux, Corinne. 1997. “An Urban Traffic Flow Model Integrating Neural Networks.” Transportation Research Part C: Emerging Technologies 5 (5): 287–300. https://doi.org/10.1016/S0968-090X(97)00015-6.
- McCarthy, J., and P. J. Hayes. 1981. “Some Philosophical Problems from the Standpoint of Artificial Intelligence.” In Readings in Artificial Intelligence, edited by Bonnie Lynn Webber and Nils J. Nilsson, 431–450. Los Altos, CA: Morgan Kaufmann. https://doi.org/10.1016/B978-0-934613-03-3.50033-7.
- McCarthy, John, Marvin L. Minsky, Nathaniel Rochester, and Claude E. Shannon. 2006. “A Proposal for the Dartmouth Summer Research Project on Artificial Intelligence, August 31, 1955.” AI Magazine 27 (4): 12–12. https://doi.org/10.1609/aimag.v27i4.1904.
- Minsky, Marvin Lee. 1967. Computation: Finite and Infinite Machines. Englewood Cliffs, N.J. : Prentice-Hall. http://www.cba.mit.edu/events/03.11.ASE/docs/Minsky.pdf.
- Pomerol, Jean-Charles. 1997. “Artificial Intelligence and Human Decision Making.” European Journal of Operational Research 99 (1): 3–25. https://doi.org/10.1016/S0377-2217(96)00378-5.
- Pomerol, Jean-Charles, and Frederic Adam. 2008. “Understanding Human Decision Making – a Fundamental Step towards Effective Intelligent Decision Support.” In Intelligent Decision Making: An AI-Based Approach, edited by Gloria Phillips-Wren, Nikhil Ichalkaranje, and Lakhmi C. Jain, 97:3–40. Studies in Computational IntelligenceBerlin, Heidelberg: Springer Berlin Heidelberg. https://doi.org/10.1007/978-3-540-76829-6_1.
- Ritchie, Stephen. G. 1990. “A Knowledge-Based Decision Support Architecture for Advanced Traffic Management.” Transportation Research Part A: General 24 (1): 27–37. https://doi.org/10.1016/0191-2607(90)90068-H.
- Russell, Stuart, and Peter Norvig. 1995. “A Modern, Agent-Oriented Approach to Introductory Artificial Intelligence.” ACM SIGART Bulletin 6 (2): 24–26. https://doi.org/10.1145/201977.201989.
- Schöpp, Ferdinand, Özgür Öztürk, Regina Linke, Jürgen. K. Wilke, and Manfred Boltze. 2021. ‘Electrification of Road Freight Transport – Energy Consumption Analysis of Overhead Line Hybrid Trucks’. In. Washington DC (United States). https://trid.trb.org/view/1759512.
- Siemens, A. G. 2012. ‘ENUBA. Elektromobilität Bei Schweren Nutzfahrzeugen Zur Umweltentlastung von Ballungsräumen. Schlussbericht Der Siemens AG.’ without location.
- Siemens, A. G, Technische Universität Dresden, and Deutsches Zentrum für Luft- und Raumfahrt e. V. 2016. ‘ENUBA 2. Elektromobilität Bei Schweren Nutzfahrzeugen Zur Umweltentlastung von Ballungsräumen. Schlussbericht Der Verbundforschungspartner’. München, Dresden, Braunschweig (Germany). https://www.erneuerbar-mobil.de/sites/default/files/2016-09/ENUBA2_Abschlussbericht_V3_TIB_31-08-2016.pdf.
- Simon, Herbert. A. 1965. (Herbert Alexander) The Shape of Automation for Men and Management. New York, Harper & Row. http://archive.org/details/shapeofautomatio00simo.
- Tang, Ling, Jieyi Li, Hongchuan Du, Ling Li, Jun Wu, and Shouyang Wang. 2022. “Big Data in Forecasting Research: A Literature Review.” Big Data Research 27 (February): 100289. https://doi.org/10.1016/j.bdr.2021.100289.
- Vinuesa, Ricardo, Hossein Azizpour, Iolanda Leite, Madeline Balaam, Virginia Dignum, Sami Domisch, Anna Felländer, Simone Daniela Langhans, Max Tegmark, and Francesco Fuso Nerini. 2020. “The Role of Artificial Intelligence in Achieving the Sustainable Development Goals.” Nature Communications 11 (1): 233. https://doi.org/10.1038/s41467-019-14108-y.
- Vlahogianni, Eleni. I., Matthew. G. Karlaftis, and John. C. Golias. 2014. “Short-Term Traffic Forecasting: Where We Are and Where We’re Going.” Transportation Research Part C: Emerging Technologies, Special Issue on Short-Term Traffic Flow Forecasting 43 (June): 3–19. https://doi.org/10.1016/j.trc.2014.01.005.
- Wang, Ren., Shimao Fan, and Daniel. B. Work. 2016. “Efficient Multiple Model Particle Filtering for Joint Traffic State Estimation and Incident Detection.” Transportation Research Part C: Emerging Technologies 71 (October): 521–537. https://doi.org/10.1016/j.trc.2016.08.003.