ABSTRACT
Our study draws from a natural experiment created by the school lockdowns in Finland during the 2020 coronavirus disease (Covid-19) pandemic to compare at-school and home-based responses to an online youth crime survey. Using our quasi-experimental design, we examine how at-home responses during the Covid-19 lockdown affected the sample composition and reported prevalence of offences in the nationally representative Finnish Self-Report Delinquency Study 2020 (FSRD-2020) survey (N = 5503). We compare these within-year changes in 2020 to the earlier FSRD-2016 survey (N = 5955) that did not involve a transition to at-home response. According to our analysis, the share of males decreased in remote schooling. We also detected a decrease in reported offences during lockdown (remote school response) in several types of offences, net of observed compositional changes. The findings suggest that at-school data collection helps secure more inclusive samples and encourages students to self-report their offending behaviours.
Since the beginning of the 20th century, crime surveys have been part of a more general drive to develop sensitive topic surveys, such as those focusing on sex or drug research (Kivivuori, Citation2011). Today, most developed countries supplement administrative crime data with victim and offender surveys. The survey methodology also provides a unique instrument for international comparisons (Enzmann et al., Citation2018). Yet, at the same time, methodological research has suggested that survey research on sensitive topics is context dependent (see e.g. Tourangeau & Yan, Citation2007). The rise of the internet and mobile technologies has also influenced the landscape in survey research. Therefore, there is an evident need to examine how the instrument and context of responses impacts the results of survey-based studies.
The purpose of this study is to utilize a natural experiment to assess the methodological role of the setting in crime surveys. Our natural experiment derives from the impact of coronavirus disease(Covid-19) restrictions on the ongoing data collection of the Finnish Self-Report Delinquency Survey (FSRD) in the spring of 2020 (FSRD-2020, N = 5503). We analyse the within-year changes (before and during the lockdown) in FSRD-2020 using FSRD-2016 (N = 5955) data, collected during the spring of 2016, as a counter-factual. With this design, we examine the effects of the response setting on survey sample composition and the reported prevalence of offences.
Our inquiry is based on the FSRD (see, e.g. Ellonen et al., Citation2021) collected in 2016 and 2020. The FSRD is an anonymous crime survey representing Finnish ninth-grade students. The survey sample is based on a random sampling of schools. The FSRD surveys are collected every 4 years. In the normal implementation of the FSRD, students respond to an online survey during a scheduled lesson using either their personal or school computers or mobile devices, but they are asked to avoid using smartphones. The lesson is supervised by a teacher.
The data collection of the ninth sweep of the FSRD started in January 2020, but in March 2020, the Finnish government ordered school lockdowns and a transfer from contact teaching to home-based schooling in order to try to prevent the spread of the Covid-19 virus. The lockdown lasted from 18 March until 14 May.
Because data could no longer be collected in schools, the remaining FSRD sample schools responded to the survey while in home-based schooling. This meant that the respondents were at home, using a computer or mobile device to respond to the standard online FSRD questionnaire. However, in the home-based data collection, we aimed to mimic as closely as possible the standard design of data collection in the school setting. The students filled in the questionnaires during their regular at-home remote-school classes. Thus, a teacher virtually supervised the classes, even though the students were physically at home.
We predict that as it might be easier to be truant in distance learning, students with a higher load on delinquency risk factors may drop out of the study when it is moved from school to home. Furthermore, responding remotely at home provides a different setting compared to normal classroom data collection with other students present, which may have an impact on the reporting of delinquent behaviour. Earlier studies suggest that the home-setting is associated with a lower self-reported prevalence of offending behaviour when compared to at-school responding (Gomes et al., Citation2019). However, in previous methodological studies on criminological self-report surveys, the differences between home and school responses have been confounded by other differences in survey implementation (e.g. personal vs. group administration).
The findings of this study are relevant for the continued examination of the validity of sensitive topic surveys. Due to the increasing difficulties of gaining school access (see, e.g. Marshall, Citation2010), there may also be increasing pressure to move from school-based to online home-based surveys. Our findings will therefore provide valuable information regarding this type of future transition.
Prior research
There have been several studies on method effects in self-reported surveys. For example, socially disadvantaged groups may be more difficult to reach if home serves as the contact point, in contrast to schools (Naplava & Oberwittler, Citation2002). In addition, earlier studies have reported that self-administered surveys tend to report a higher prevalence of risk behaviour than interview-based surveys (Australian Bureau of Statistics, Citation2004; Gomes et al., Citation2019). However, school-based responses do not seem to differ based on whether the data collection was supervised by a teacher or by an external research staff (Bjarnason, Citation1995; Kivivuori et al., Citation2013; Walser & Killias, Citation2012).
Earlier research has also reported a so-called setting effect for youth surveys on sensitive topics. Youths who respond to surveys in a home setting report less risky behaviour (e.g. substance use or sexual behaviour) when compared to their peers responding to surveys in a school setting (N. D. Brener et al., Citation2006; N.D. Brener et al., Citation2022). The survey setting effect has been found to be stronger than effects related to administration mode (i.e. paper and pencil or computer-assisted surveys; see, N. D. Brener et al., Citation2006). Some earlier studies have suggested that the setting effect is (at least partly) a function of the varying level of perceived anonymity or privacy between school and home settings, but the empirical evidence for this is limited or even contrary (see, e.g. N. D. Brener et al., Citation2006). It is also possible that the presence of parents or siblings at home may reduce self-reported offences (for substance use, see, Aquilino et al., Citation2000).
A few studies have analysed the effect of the survey setting on criminological self-reporting surveys (Gomes et al., Citation2019). In general, students who answer surveys in a school setting report a higher prevalence of offending behaviour when compared to responses given at home. In these studies, however, the home setting has been operationalized as mail surveys (Cops et al., Citation2016) or as a private situation where the survey has been collected at home via a personally scheduled meeting with a survey collector (N. D. Brener et al., Citation2006). Thus, the setting effect has been confounded by other differences between school-based and home-based responses such as group and personal mode of administration (N. D. Brener et al., Citation2006).
Answering the survey at school takes place in a supervised group situation (supervision is not targeted directly at the individual respondent but at the group) where the presence of peers, for example, can have an impact on responding as well (N. D. Brener et al., Citation2006). Mail surveys or privately arranged home responses do not involve a group situation. In addition, in mail surveys, responding is unsupervised, while the privately arranged survey setting at home is even more controlled than a school setting. Supervision is targeted directly at the individual respondent instead of a group. Thus, to analyse the effect of mere setting differences on the reported offending behaviour, the school-based response should be compared to situations where home responding is also a supervised group situation. We can do this by comparing school-based survey responses to remote school-based responses.
Methods
Data
We base this study on two representative large-scale FSRD surveys collected in 2016 and 2020. The FSRDs are anonymous online crime surveys that cover a wide range of offences, victimization experiences and theory-based risk factors (Ellonen et al., Citation2021; Saukkonen et al., Citation2016). Data are collected at schools during regular school lessons and are completed under the supervision of teachers (see, e.g. Ellonen et al., Citation2021). The representative surveys target the last grade of comprehensive school in the Finnish school system (ninth grade), during which the respondents are 15 or 16 years old. Data are collected during the spring term, and the window for data collection spreads from late January to May, largely depending on school access and the decision of individual schools on when to organize the data collection.
The 2016 FSDR survey consists of 6061 observations (51% females), with the overall response rate being 79%. FSRD-2020 consists of 5674 observations (50% females) with an overall response rate of 78%. For both surveys, a one-stage cluster sampling strategy considered the school area (stratified according to the level of community urbanity) and the school size (probability proportional to size). Both surveys used a forced response procedure, which means that respondents had to answer all questions to move forward in the survey. There are some missing answers for covariates in the data (e.g. 2020 max 2% for self-control) as not all respondents completed the full questionnaire.
The overall rates of suspected juvenile offences (15- to 17-year-olds) remained relatively stable between the years 2016 – when the Police of Finland registered 3237 thefts, 1325 assaults, and 979 vandalisms – and 2020, when the Police of Finland registered 2743 thefts, 1496 assaults, and 1026 vandalisms (Kolttola, Citation2021).
Measures
We used dummy variables to indicate whether the observations were collected in 2016 or 2020 (year) and before or during the lockdown period (season). The dates for pre-lockdown and lockdown periods were the same for years 2016 and 2020, even though there was no actual interruption in contact teaching in 2016. However, for the sake of consistency, we call the period ranging from the beginning of the year to March 17 a pre-lockdown period for the year 2016 as well. Similarly, the lockdown period covers the period from 18 March to 14 May for years 2016 and 2020.
We measured the possible changes in the socio-demographic composition of the sample due to response mode (school vs. home). We focused on selected individual characteristics: gender, age, mathematics grade, self-control, non-nuclear family, parental control, father’s unemployment, father’s non-native birth country status, perceived relative deprivation regarding family and personal financial situation. In addition, we measured whether the respondent’s school was in one of the 15 largest cities in Finland (urbanity of the school area). We report distributions for the composition variables within the pre-lockdown and lockdown periods in 2016 and 2020 in .
Table 1. Composition of FSRD population before and during the lockdown (2016 as a comparison year).
We measured parental control with a single item whose wording differed slightly in the FSRD-2016 and FSRD-2020. In 2016, the item was ‘When I go out, my parents ask me who I spend time with’. In 2020, it was ‘Do your parents require you to tell them where you are, with whom, and what you are about to do in the evenings?’ Since these items yielded different distributions, we applied different cut-off points to mark ‘low parental control’.
We measured self-control using the Grasmick-Tittle scale as applied in the International Self-Report Delinquency Studies and the FSRD surveys (Ellonen et al., Citation2021). The scale incorporates three items from the impulsivity, risk seeking, and self-centeredness sub-scales included in the Grasmick-Tittle scale (Grasmick et al., Citation1993). The internal consistency of this 9-item scale was 0.84/0.85 in the two data years (Cronbach’s alpha).
In our analyses regarding survey outcomes, we focused on the prevalence of 17 self-reported offences that were present in FSRD surveys in 2016 and 2020. We report the prevalence rates for the chosen offences within the pre-lockdown and lockdown periods in 2016 and 2020 in Appendix I. The used recall period covered offences committed during the prior 12 months. This highlights the fact that any possible changes in reported crime propensity within spring 2020 are likely to be the effect of methods rather than the effects of the covid-19 lockdown on criminal behaviour. However, as the lockdown period lasted 2 months for a maximum of 0–16% of the length of the recall period, some behavioural effects cannot be excluded.
In our analyses, all used variables were dichotomous, and we used them to flag the presence of studied risk factors or self-reported offending behaviour. For continuous variables, the mean value was used as a cut-off point for dichotomization. The data included some missing values (ranging from 0% to 2% for different variables). The gender option ‘other’ was only present in the 2020 data set, and thus, observations other than male or female (N = 112) were coded as missing in our analysis. For age, values above 19 were coded as missing (N = 33).
Analysis
We calculated the within-year changes in the sample socio-demographic composition with cross-tabulation for years 2016 and 2020. In addition, we calculated the difference between the within-year changes in 2020 and 2016 (the 2020 within-year change minus the 2016 within-year change) for each compositional variable (see, ). We tested this difference by conducting a logistic regression model that predicted each sample characteristic variable by year, season, and a year*season interaction term (12 tests in total). A significant interaction between year and season was interpreted as a difference in the within-year change (i.e. before and during the lockdown). This analysis tested whether there were significant differences in the sample composition between the pre-lockdown and lockdown periods in 2020 when compared to the counter-factual year 2016.
We analysed both the within-year changes in the reported prevalence of the 17 types of offence with bivariate tabulation and the corresponding chi-square test (separately for years 2016 and 2020, see Appendix I) and using logistic regression modelling. In the logistic regression models, we treated the offending behaviour as an independent variable, and we included year, season and a year*season interaction term as independent variables. For all models, we included all our sample composition variables (see, ) as covariates.
The comparison between years 2016 and 2020 enables us to tease out the lockdown effects. We technically define the lockdown effect as an interaction between the year and season variables, adjusting for social composition change and school urbanity. Because these analyses make 17 regression models in total, and the focus of this study lies solely on each model’s interaction term, we report the results for the significant lockdown effects in the text (for all estimated interaction terms, see Appendix II).
To elaborate on the found interaction effects, we plotted the adjusted offending prevalence rates for statistically significant interactions (). We based the adjusted prevalence rates on logistic regression models and predicted offending prevalence for pre-lockdown and lockdown periods in 2016 and 2020, while keeping the control variables fixed in their mean values. We calculated adjusted prevalence rates as population-average marginal effects using the margins command in the statistical software Stata (Williams, Citation2012). For all logistic regression models, we adjusted the standard errors for the clustering of observations within schools. In this research, we did not use the standard FSRD weights because the lockdown division did not conform to the sample formation and stratification. This applies to both 2016 and 2020 datasets.
Figure 1. Predicted prevalence of offending by response period and year, net of compositional changes. Note the different scales of vertical axes.
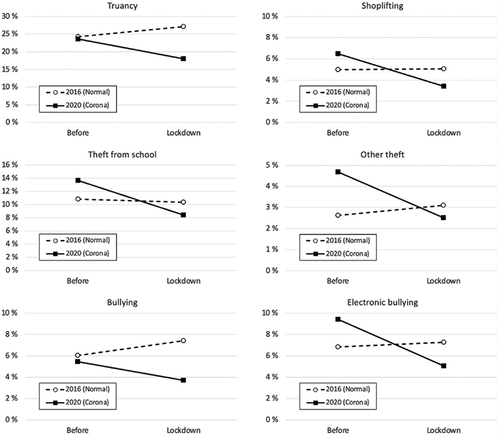
As our analysis concerns the sample compositions and reported prevalence of offences both before and during the Covid-19 lockdown, we excluded the few responses given after the lockdown from this study (N = 277). We used the conventional 95% confidence level for significance in all conducted statistical tests.
Findings
indicates the compositional changes in the samples, comparing the ‘seasons’ defined by the 2020 lockdown dates. We see that the only statistically significant difference in the within-year change between years 2020 and 2016 concerned respondents’ gender (p = .007). In 2020, there were fewer males in the sample response during the lockdown compared to respondents within the pre-lockdown period. Males were dropping out in the distance-learning mode, possibly using the new schooling mode to play truant from the distance classes. In 2016, the proportion of males slightly increased during the same period (1.7%). We observed no other significant differences in the within-year changes.
In the analysis within 2020, lockdown was associated with decreased crime prevalence rates in most offence types, suggesting that the change could be linked to a situational effect of at-home distance classes (see Appendix I). The exceptions were robbery/extortion, use of drugs other than cannabis, the carrying of weapons, and drunk driving. These offences did not show a significant difference in prevalence before and during the lockdown.
According to our logistic regression models, six offences manifested a significant lockdown effect net of measured compositional effects. These are truancy (p = .001), shoplifting (p = .021), theft from school (p = .019), other theft (p = .006), bullying (p = .015), and electronic bullying (p = .004). The adjusted prevalence rates of these six offences are reported separately for different response periods in . As noted, all six of these offences manifested clear decreases in prevalence during the 2020 lockdown. In the corresponding time slot in 2016, no such reduction in prevalence can be seen.
To assess the robustness of our non-linear interaction models, we conducted all our interaction models predicting self-reported offences with linear probability modelling as well. The same interaction effects were found using linear probability models: truancy (p = .001), shoplifting (p = .026), theft from school (p = .027), other theft (p = .001), bullying (p = .012), and cyberbullying (p = .001). Moreover, none of the non-significant interaction effects from the logistical models were significant in our linear probability models.
We are confident in linking the decreases in distance responses in 2020 because the counter-factual year 2016 did not manifest similar decreases in a simulated before-and-during lockdown comparison. In 2016, prevalence rates were stable before and during simulated lockdown. Indeed, truancy increased in prevalence from early to late spring respondents in 2016 (p = .003). In other offences, there was a non-significant increase during the spring season as defined by simulated 2020 lockdown dates.
Sensitivity analyses
We conducted several sensitivity analyses to assess the robustness of our findings. As these additional analyses comprise an extensive number of models, we report only statistically significant findings in the text.
We first explored the 2020 data collection by dividing the pre-lockdown period into two sub-periods. We kept the lockdown period as a single unity due to the small N in sub-timeslots, especially in the period closest to summer. The rationale for comparing the responses in the earliest stage and in the immediate pre-lockdown weeks is that we can indirectly probe the existence of a pure Covid-19 effect. In the weeks preceding the lockdown, there was a strong awareness of the approaching pandemic, and infections were also reported in Finland. If this awareness affected the way students responded, we expected to see a Covid-19 effect before the lockdown. However, we could not observe any changes in reporting within the pre-lockdown period. This suggests that the descriptive changes shown in reflect the situational effects of responding during home-based schooling rather than anxiety triggered by the Covid-19 pandemic as such.
We also tested whether the self-reported offences changed in a linear manner during spring 2020 instead of binary before and during the lockdown change. For this analysis, we conducted logistic regression models that predicted each type of offence with linearly modelled time. The linear time variable indicated the response time according to the half-month periods (i.e. beginning of February, end of February, beginning of March, etc.). To solely capture the timely trend of self-reported offence, we did not include any covariates in these models.
Linear time was a significant predictor only for electronic bullying (OR = 0.91, p = .008) and theft other than shoplifting or theft from school (OR = 0.90, p = .014). Moreover, even in these cases, the binary modelled time was a slightly better predictor of other thefts (for linear time: Cragg & Uhler’s R2 = .003, AIC = .376, for binary time: Cragg & Uhler’s R2 = .005, AIC = .375) and electronic bullying (for linear time: Cragg & Uhler’s R2 = .003, AIC = .615, for binary time: Cragg & Uhler’s R2 = .006, AIC = .613).
Finally, we conducted a placebo test by treating February 15 (2016 N = 1595; 2020 N = 1799) and February 28 (2016 N = 3168; 2020 N = 2995) as alternative cut-off dates to test whether the same ‘lockdown effect’ could be found with arbitrary dates. We found none of the studied lockdown effects to be significant using these alternative cut-off dates.
Discussion
In this study, we utilized a natural experiment arising from the Covid-19 pandemic impacted data collection of the FSRD in the spring of 2020. We set out to explore two types of method effects: compositional changes in the sample and setting effects on crime reporting.
According to our findings, compositional effects did not appear to be very large or extensive. The percentage of male respondents decreased during the lockdown. This can reflect opportunity and control factors: males might have used the shift to home-based schooling to engage in other activities than those assigned by the teacher. However, earlier methodological studies have reported larger differences in sample compositions between school-based surveys and mail surveys (see, e.g. Cops et al., Citation2016). Thus, it is possible that increasing the supervision of home-based response helps to decrease the bias related to nonresponse. This finding is in line with earlier studies on privately arranged at-home response where no compositional differences have been found (see, N. D. Brener et al., Citation2006).
On the other hand, we observed consistent situational effects on the self-reporting of crime during the past 12 months. On a bivariate descriptive level, the lockdown was linked to decreased prevalence of almost all offences. No similar decrease took place in the simulated 2016 ‘lockdown’ period. We consider these method impacts to be pragmatically relevant irrespective of why they happened (composition or situation).
Yet, our multivariate analysis indicated that for the six offences, there was a significant difference between the within-year changes in 2020 and the counter-factual year 2016. These effects were independent of measured compositional changes. These were truancy, shoplifting, theft from school, other thefts, bullying, and electronic bullying. This is in line with earlier criminological studies observing higher levels of self-reported offending behaviour in school-based surveys when compared to home surveys (Gomes et al., Citation2019).
However, to our knowledge, no existing study has utilized similar designs concerning criminological measurement in school-based and home-based responses when both survey settings are based on a group administration (school class) supervised by a teacher. This helps to minimize the additional differences between the situation of responses in school and at home that has been noted in earlier studies (N. D. Brener et al., Citation2006).
Our results provide strong support for the argument made in previous studies that the data collected at school during the schoolday are valuable in reducing response biases in self-reported crime surveys (see, e.g. Marshall, Citation2010). Home-based responding likely leads to biased prevalence estimates, even when the home responses seeks to mimic the school survey setting as much as possible (i.e. remote school class supervised by a teacher).
Overall, there seem to be both (limited) compositional and situational (or setting) effects of at-home responses to surveys. Compositional effects can reflect opportunity and control factors: students who do not like academic activities may use the shift to home-based schooling to engage in other activities. These found situational effects mean that students are still in (remote) class, but do not answer as openly on questions regarding their offences. According to our findings, this effect remains even when both school and home survey situations are supervised and based on group administration. There are multiple possible interpretations as to why this may be (see, e.g. Aquilino et al., Citation2000).
When youths respond from home, they may feel less comfortable in reporting their offences. There can be other people present as they respond (parents and siblings). Our analyses controlling for sibling number and parental control suggest that this is not the only interpretation. Even if there are no other persons present, the respondent may consider it a risk that the responses are somehow retrievable from the computer they are using at home. On the other hand, the presence of peers in school surveys may increase the reporting of offences, for example, by function as a memory cue for offences committed with peers (N. D. Brener et al., Citation2006).
Limitations and research needs
The most important limitation is that the lockdown did not divide the schools randomly for experimental conditions. The groups emerged naturally because of the scheduling of the survey in the schools. Related to this, it emerged that urban schools had timed their data collection differently across spring in the two study years, reducing the number of urban schools during the 2020 lockdown. Schools participating in late spring may have other unobserved properties connected to the student population. However, in our analyses we controlled for several compositional changes, including the ageing effect during spring and the urban location of the school. Furthermore, our natural experiment was highly confounded by the Covid-19 pandemic itself. However, we observed no pre-lockdown pure Covid-19 effects, possibly reflecting the increasing awareness of the pandemic.
Future research may corroborate current findings by using a randomized controlled trial in assessing how distance-class mode differs from school-class mode in surveys on sensitive topics. While a randomized controlled trial would be valuable, our current study has the compensating benefit of being a large-scale field study with probably high external validity and generalizability. The applicability of current findings to other sensitive topics, such as drugs or sex surveys, could be addressed in the future research as well.
Unless there is a future global pandemic that results in major lockdowns, it is unlikely that similar large-scale data collection will be administered during remote teaching. However, such a study design might focus on very specific and targeted groups of students, whether they are home schooled or in juvenile detention, hospital schools, and so forth. In addition, our findings imply that remote school-based responses might help in reducing compositional bias when compared to mail surveys, but the bias associated with self-reported offences remains. Future studies should elaborate on the exact characteristics of the home setting and the results of such a bias (for such studies, see, N. D. Brener et al., Citation2006).
Ethics approval
This study was performed in line with the principles of the Declaration of Helsinki. Approval was granted by the University of Helsinki Ethical Review Board in Humanities and Social and Behavioral Sciences (33/2019)
Consent
Informed consent was obtained from all individual participants included in the study.
Materials and/or Code availability
All data used in Study 1 and Study 2 will be made fully available via the Finnish Social Science Data Archive in 2021–2022.
Disclosure statement
No potential conflict of interest was reported by the author(s).
Additional information
Funding
References
- Aquilino, W. S., Wright, D. L., & Supple, A. J. (2000). Response effects due to bystander presence in CASI and paper-and-pencil surveys of drug use and alcohol use. Substance Use and Misuse, 35(6–8), 845–867+1101–1105. https://doi.org/10.3109/10826080009148424
- Australian Bureau of Statistics. (2004). Measuring crime victimisation: The impact of different collection methodologies. https://www.abs.gov.au/statistics/research/measuring-crime-victimisation-impact-different-collection-methodologies (Accessed 14 March, 2022)
- Bjarnason, T. (1995). Administration mode bias in school survey on alcohol, tobacco and illicit drug use. Addiction, 90(4), 555–559. https://doi.org/10.1111/j.1360-0443.1995.tb02190.x
- Brener, N. D., Eaton, D. K., Kann, L., Grunbaum, J. A., Gross, L. A., Kyle, T. M., & Ross, J. G. (2006). The association of survey setting and mode with self-reported health risk behaviors among high school students. Public Opinion Quarterly, 70(3), 354–374. https://doi.org/10.1093/poq/nfl003
- Brener, N. D., Bohm, M. K., Jones, C. M., Puvanesarajah, S., Robin, L., Suarez, N., Deng, X., Harding, R. L., & Moyse, D. (2022). Use of tobacco products, alcohol, and other substances among high school students during the covid-19 pandemic - adolescent behaviors and experiences survey, United States, January-June 2021. MMWR Supplements, 71(3), 8–15. https://doi.org/10.15585/mmwr.su7103a2
- Cops, D., De Boeck, A., & Pleysier, S. (2016). School vs. mail surveys: Disentangling selection and measurement effects in self-reported juvenile delinquency. European Journal of Criminology, 13(1), 92–110. https://doi.org/10.1177/1477370815608883
- Ellonen, N., Minkkinen, J., Kaakinen, M., Suonpää, K., Miller, B. L., & Oksanen, A. (2021). Does parental control moderate the effect of low self-control on adolescent offline and online delinquency? Justice Quarterly, 38(5), 827–848. https://doi.org/10.1080/07418825.2020.1738526
- Enzmann, D., Kivivuori, J., Haen Marshall, I., Steketee, M., Hough, M., & Killias, M. (2018). A Global Perspective on Young People as Offenders and Victims. First Results from the ISRD3 Study. Springer Briefs in Criminology.
- Gomes, H. S., Farrington, D. P., Maia, Â., & Krohn, M. D. (2019). Measurement bias in self-reports of offending: A systematic review of experiments. Journal of Experimental Criminology, 15(3), 313–339. https://doi.org/10.1007/s11292-019-09379-w
- Grasmick, H. G., Tittle, C. R., Bursik, R. J., & Arneklev, B. J. (1993). Testing the core empirical implications of Gottfreson and Hirschi’s general theory of crime. Journal of Research in Crime and Delinquency, 30(1), 5–29. https://doi.org/10.1177/0022427893030001002
- Kivivuori, J. (2011). Discovery of hidden crime: Self-report surveys in criminal policy context. Oxford University Press.
- Kivivuori, J., Salmi, V., & Walser, S. (2013). Supervision mode effects in computerized delinquency surveys at school: Finnish replication of a Swiss experiment. Journal of Experimental Criminology, 9(1), 91–107. https://doi.org/10.1007/s11292-012-9162-z
- Kolttola, I. (2021). Rikollisuustilanne 2020: Rikollisuuskehitys tilastojen ja tutkimusten valossa. Institute of Criminology and Legal Policy, Katsauksia. https://helda.helsinki.fi/bitstream/handle/10138/337259/Katsauksia_49_Rikollisuustilanne_2020_2021.pdf?sequence=1&isAllowed=y
- Marshall, I. H. (2010). “Pourquoi pas?” Versus “Absolutely not!” Cross-national differences in access to schools and pupils for survey research. European Journal on Criminal Policy and Research, 16(2), 89–109. https://doi.org/10.1007/s10610-010-9125-8
- Naplava, T., & Oberwittler, D. (2002). Methodeneffekte bei der Messung selbstberichteter Delinquenz von männlichen Jugendlichen. Monatsschrift für Kriminologie und Strafrechtsreform, 85(6), 401–423. https://doi.org/10.1515/mks-2002-00062
- Saukkonen, S., Laajasalo, T., Jokela, M., Kivivuori, J., Salmi, V., & Aronen, E. T. (2016). Weapon carrying and psychopathic-like features in a population-based sample of Finnish adolescents. European Child and Adolescent Psychiatry, 25(2), 183–191. https://doi.org/10.1007/s00787-015-0724-2
- Tourangeau, R., & Yan, T. (2007). Sensitive questions in surveys. Psychological Bulletin, 133(5), 859–883. https://doi.org/10.1037/0033-2909.133.5.859
- Walser, S., & Killias, M. (2012). Who should supervise students during self-report interviews? A controlled experiment on response behaviour in online questionnaires. Journal of Experimental Criminology, 8(1), 17–28. https://doi.org/10.1007/s11292-011-9129-5
- Williams, R. (2012). Using the margins command to estimate and interpret adjusted predictions and marginal effects. The Stata Journal, 12(2), 308–331. https://doi.org/10.1177/1536867X1201200209