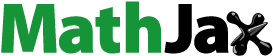
Abstract
Since the anthrax attacks in the USA in 2001, there is increasing concern about biosurveillance. Moreover, the coronavirus pandemic showed up, even more, the significance of continual systematic collection, analysis, interpretation, and dissemination of health data for early detection of disease outbreaks. To timely and efficiently detect infectious or noninfectious disease outbreaks we should consider both spatial and temporal dimensions. Of interest are global changes in the number of new disease events on time in a specific broader area and/or hotspots of disease events in smaller areas which may evolve into outbreaks or even into pandemics, such as the coronavirus pandemic. In this paper, we propose a practitioner-friendly monitoring procedure for monitoring simultaneously the number of disease events and the spatial distribution of disease events. The proposed method exploits a flexible and efficient mathematical tool, the convex hull, in conjunction with control charting procedures. As the numerical illustration showed, the proposed method has excellent performance under different outbreak scenarios.
1 Introduction
Since the anthrax attacks on USA (2001), there is increasing concern about the impact of potential bioterrorism attacks. Biosurveillance refers to the continual systematic collection, analysis, interpretation, and dissemination of health data (Kman and Bachmann Citation2012) for early detection of abnormal changes in the global or local prevalence of a communicable or noncommunicable disease. Recently, the COVID-19 pandemic showed up, even more, the significance of biosurveillance techniques and systems. According to World Health Organization (WHO) data, on February 3, 2023, more than 0.75 billion cases and 6.8 million deaths had been confirmed. Given the seriousness of the virus and its consequences worldwide, it immediately emerges that there is a need to develop new methods for the effective monitoring of the number of coronavirus cases or of any other infectious disease events and their spatial distribution to detect in time outbreaks that will have a severe impact on the public health.
A systematic review of almost 30 biosurveillance systems concluded that there is insufficient evidence to determine which of these systems is best (Bravata et al. Citation2004). Modern biosurveillance is related to the monitoring of a wide range of prediagnostic and diagnostic data for the purpose of enhancing, mainly the ability of the public health infrastructure to detect, investigate, and respond to disease outbreaks. In the last decades, a lot of statistical techniques have been used in the framework of biosurveillance with the main ones being Statistical Process Monitoring (SPM) and Scan Statistics. Here we should note that biosurveillance is not referred only to communicable diseases but also to non-communicable diseases in the sense that any increase or/and a high consecration in a specific area may denote the presence of an important health risk factor (e.g. pollution).
Several researchers have dealt with the application of SPM in health surveillance (biosurveillance). Woodall (Citation2006) published a review paper on the use of control charts in healthcare monitoring and public health surveillance. Tsui et al. (Citation2008) also presented a review of methods involved in healthcare, public health, and syndromic surveillance. Furthermore, Unkel et al. (Citation2011) presented an expanded review of general statistical methods for the prospective detection of infectious disease outbreaks.
A simple tool coming from the SPM toolbox and used for biosurveillance is the well-known c-chart, which, is used to monitor the number of defects (in manufacture) or events in a specified area in a biosurveillance context. The number of events is usually assumed to follow a specific distribution, such as the Poisson distribution. The use of control charts in the field of biosurveillance has been expanded beyond Shewhart-type control charts, e.g. cumulative sum (CUSUM) procedures are proposed by Rossi et al. (Citation1999), which are based on the normal approximation to a Poisson process. A lot of notable problems arise when applying SPM techniques for monitoring health-related variables. In view of that, Bersimis and Economou (Citation2017) proposed a methodology to bridge the gap of a change in the sampling scheme. This is one of the cases that naturally arise in public health monitoring and syndromic surveillance.
The more recent methodologies of Multivariate Statistical Process Monitoring (MSPM) have been also used in the framework of biosurveillance. Bersimis et al. (Citation2016) presented an extensive literature review on the applications of MSPM in health and especially in the field of public health monitoring. Moreover, Rogerson and Yamada (Citation2004) compared the univariate and multivariate cumulative sum (CUSUM) methods for disease surveillance. Rogerson (Citation2005) discussed the use of the CUSUM method on spatial surveillance. Rolka et al. (Citation2007) discussed MSPM techniques (i.e. T2, MEWMA, MCUSUM) in the field of public health surveillance for emerging threats.
Furthermore, Woodall and Montgomery (Citation2014) provided an overview of research and applications of SPM in areas, such as health-related monitoring, spatiotemporal surveillance, etc. while Schiöler and Frisén (Citation2012) presented a generalized-likelihood ratio surveillance method by adapting a robust method for detecting outbreaks. Correia et al. (Citation2011) used both univariate and multivariate control charts to monitor patients suffering from chronic respiratory disease. Shmueli and Burkom (Citation2010) discussed the monitoring of time series to have on-time alarms of abnormalities to start an investigation of potential outbreaks, while Frisén et al. (Citation2010) discussed the challenges of evaluating multivariate surveillance methods. Zhang et al. (Citation2010) proposed a new MEWMA Phase II control chart for simultaneously monitoring the mean and variability that is based on the generalized likelihood ratio test. Bersimis and Sachlas (Citation2022) proposed a unified framework for surveilling public health through statistical process monitoring.
Apart from monitoring the number of events in a specified area, it is of special interest to monitor their spatial distribution as well. Spatial surveillance systems are used to detect changes in public health data, and alert to possible outbreaks of disease. Statistical methods play a key role in spatial surveillance, as they are used to identify changes in data and build models of that data in order to make predictions about future activity. For example, Fricker and Chang (Citation2008) proposed a spatiotemporal methodology for real-time biosurveillance. Upon a signal of a possible outbreak, the methodology suggests a way to graphically indicate the likely outbreak location, and the output can subsequently be used to track the spread of an outbreak.
Additionally, during the last 20 years, many researchers have dealt with local spatial clustering procedures as the spatial dimension arises naturally in a vast number of cases. Indicatively we mention the work of Caldwell (Citation1989), Kulldorff et al. (Citation1997), and Bhowmick et al. (Citation2008) from the health sector or Bersimis et al. (Citation2014), which aims to detect possible clusters of economic activity.
The methods for determining spatial clustering are categorized into two major groups: point-based methods and area-based methods. The difference between the two categories is that the first one uses exact locations of events under investigation, while the latter uses aggregated values in regions. A review of methods for the statistical analysis of spatial patterns of disease was presented by Marshall (Citation1991).
Among the methods that appeared in the literature, the scan statistic is mainly used for detecting spatial clusters. The two-dimensional scan-type statistics were introduced by Naus (Citation1965). Since then they have been extensively used for the detection of spatial clustering (among others see Darling and Waterman Citation1985; Anderson and Titterington Citation1997; Hjalmars et al. Citation1996; Haiman Citation2000; Kulldorff Citation2001; Glaz et al. Citation2001; Boutsikas and Koutras Citation2003).
Among all the proposed methods the most well-known is Kulldorff’s method (Kulldorff Citation1997). The application of the Kuldorff scan statistics has been popularized by the provision of the SaTScan software and by the simplicity of the original idea. This method exploits a circle-based spatial scan statistic and tests the hypothesis that the events are distributed uniformly in a geographical region. The assumption of the uniformly distributed disease events on the plane is a plausible one since this means that there is no disease either disease becomes extinct (Moitra and Sinha Citation2019). The spatial scan statistic approach places a circular window on the map and lets the center of the circle relocated continuously over the area so that at different positions the window includes different sets of neighboring census areas. If the window contains the centroid of a census area, then the whole area is included in the window. For each centroid, the radius of the circular window is varied continuously from zero to a maximum radius, in such a way, that the window never includes more than 50% of the total population of the area under study. Other shaped scan statistics have been used as well. However, Kulldorff’s method has two main limitations: The scanning window may be of any shape, including circular (Kulldorff and Nagarwalla Citation1995) and elliptic (Duczmal et al. Citation2006) but needs to be predetermined and it is “computationally expensive” as it searches across the entire study area (Fanaee-T and Gama Citation2015).
Sparks (Citation2012) combined the SCAN statistic with multiple-sized windows of Kulldorff (Citation2001) with the EWMA control chart to detect spatially clustered outbreaks. The multiple window EWMA SCAN plan first calculates the exponentially weighted moving of temporal cell counts prior to scanning spatial regions for these smoothed counts. The advantage of the multiple window SCAN plans is their ability to retain several levels of spatial memory and therefore expected to detect both large and small outbreaks early.
In this paper, a new method for monitoring the number of disease events and their spatial distribution using convex hulls and control charting is proposed. In Section 2, three motivating well-known cases are given, and in Section 3 the new monitoring method is introduced based on the assumption that under the null hypothesis (normal conditions) disease events consist of a homogeneous Poisson process in the plane. Section 4 contains a performance investigation while in Section 5 we provide a numerical illustration. In Section 6, we present an application of the proposed method to a real-data set. In Section 7, we discussed three possible extensions of the proposed method to take into account possible heterogeneity under the null hypothesis while in the last section, some concluding remarks are given.
2 Motivating examples
In this section, we present several motivating examples from three different fields: public health, terrorism attacks, and the environment.
2.1 Public health threats from Infectious diseases outbreaks
The main motivation for our research was the Coronavirus disease 2019 (COVID-19), a pandemic that has hit the whole world since the end of 2019, with more than 6.8 million deaths until today. According to WHO, COVID-19 is an infectious disease that first appeared in Wuhan, China, caused by the most recently discovered coronavirus.
People infected by COVID-19 mainly show symptoms of fever, dry cough, and tiredness. Less common symptoms are nasal congestion, headache, sore throat, diarrhea, loss of taste or smell, etc. The COVID-19 symptoms are usually mild and begin gradually. Many people become infected by the virus but the symptoms are very mild.
About 80% of patients recover from the disease without any hospital treatment. Among the groups with a higher risk to show serious symptoms are elderly people and those with underlying medical problems (i.e., high blood pressure, heart problems, lung problems, diabetes, cancer, etc.). However, anyone can catch COVID-19 and become seriously ill.
The COVID-19 case highlighted the need for real-time surveillance of emerging public health threats and disease outbreaks. Toward this aim, online health maps (like the Global Health Observatory Map Gallery by the WHO and HealthMap by the Boston Children’s Hospital) are becoming useful tools in the detection of global public health threats. Typical examples of monitored diseases that are of global interest include seasonal flu, Zika fever, and Measles. Other examples, that although not worldwide spread include Ebola hemorrhagic fever (mainly in Africa) and Japanese Encephalitis (mainly in Asia).
Early detection of an outbreak of these diseases or the identification of an area with high consecration of incidents is crucial for limiting the spread of these public health threats. Although, the huge number of data, reported and collected from all over the world for a large number of diseases, demands automatic and in most cases unsupervised procedures to identify any threat. For that reason developing proper statistical tools to quickly detect a global threat not only by noticing a disease outbreak (significant increase of events) but also by the presence of any hotspots is necessary.
Zhao et al. (Citation2011) proposed a real-time surveillance methodology, which uses a local linear estimation method that incorporates day-of-week effects to construct predicted spatiotemporal incidence rate surfaces and applies residual analysis to detect anomalies and Yan et al. (Citation2018) proposed a methodology, based on spatiotemporal smooth sparse decomposition, to monitor high-dimensional data streams.
Sowmyanarayanan et al. (Citation2008) investigated an outbreak of symptomatic viral hepatitis in children in Vellore, India, and studied the disease pattern through serological and epidemiological methods, along with Geographical Information Systems (GIS) mapping. Wand and Ramjee (Citation2010) assessed the core areas of HIV infection in KwaZulu-Natal, South Africa, using epidemiological data among sexually active women from localized communities. Kammerer et al. (Citation2013) compared the effectiveness of county-based log-likelihood ratio, cumulative sums, and a spatial scan statistic as techniques for tuberculosis outbreak detection.
Recently, Yang and Qiu (Citation2020) proposed a sequential monitoring technique, which takes into account the dynamic nature of the observed disease incidence rates, spatiotemporal data correlation, and arbitrary data distribution.
2.2 Noncommunicable disease outbreaks
Unlike communicable or infectious diseases, which are caused by microorganisms such as bacteria, viruses, parasites, and fungi that can be transmitted, directly or indirectly, from one person to another, communicable diseases are the result of a combination of genetic, physiological, environmental, and behavioral factors. The main types of noncommunicable diseases are cardiovascular diseases, cancers, chronic respiratory diseases, and diabetes. It is worth mentioning that noncommunicable diseases are the leading cause of death worldwide (Kristoffersson and Lindén Citation2020).
The importance of noncommunicable disease surveillance is highlighted by the fact that the WHO has a special unit, i.e. the Surveillance, Monitoring, and Reporting unit. Its main objective is to support the collection, analysis, and dissemination of country-level risk factor information to inform and improve public health policy.
The review of Kroll et al. (Citation2015) concluded that although there is an increasing disease burden, noncommunicable disease surveillance is limited in most low- and middle-income countries. Kristoffersson and Lindén (Citation2020) reviewed 45 articles, published between 2011 and 2020, concerning wearable sensors for the monitoring and prevention of noncommunicable diseases. Blangiardo et al. (Citation2020) presented an overview of spatiotemporal noncommunicable disease surveillance, using hierarchically specified models. Through simulation, they concluded that mixture models designed for detection perform better than standard disease mapping models.
2.3 Chemical or biological attacks or incidents
Chemical or biological attacks or even incidents involving nuclear materials affect a smaller area but the percentage of the affected people among the risk population, living in that area, maybe significantly high. In such a case a detailed record of the number and location of incidents can be useful in locating the start (or the hotspot) of the attack.
Again in this case, a formal, statistical monitoring procedure, as the one described in the following section, is necessary to confirm that an attack or the incident has occurred but also to determine the presence of any hotspot. Such information can be used by the authorities to determine where the attack or the incident took place and to limit the consequences.
A recent example, that fortunately did not have any significant effect on the population, is the ruthenium 106 that have been detected by many European monitoring stations in the atmosphere at the end of September 2017. The increasing number of reports (events) and the location of the reporting stations allowed, with some delay, to locate its source of emission in Russia’s southern Urals.
Kleinman et al. (Citation2004) proposed a method, based on generalized linear mixed models, which is used to evaluate whether observed disease incidences in relatively small areas are larger than would be expected.
2.4 Environmental monitoring
The quality of life of the population is significantly influenced by the environmental conditions of an area. For example, air pollution causes the deterioration of the population’s quality of life, principally in cities where the urbanization level seems limitless.
Park et al. (Citation2015) dealt with the relationship between air pollutant levels and standardized mortality between 2005 and 2013 in Korea. The statistical interpolation technique is adapted to solve the problem of spatial misalignment between air pollutants and administrative districts. In addition, SaTScan is used to detect the high relative risk area based on spatial and temporal characteristics.
Bersimis et al. (Citation2017b) presented an advanced technique for real-time monitoring of pollution data at the city level. This technique is based on an appropriately adjusted multivariate time series model. The proposed methodology was applied in Athens, Greece to monitor carbon monoxide levels. Bersimis and Triantafyllopoulos (Citation2020) proposed a method of dynamic monitoring of pollution data using Kalman filters and multivariate statistical process control techniques, as the measurements are the output of multivariate processes. The detection of a change in pollutant levels may be done either globally (it affects entire the city) or locally (it affects specific sub-areas). Recently, in the context of air pollution surveillance, Yang and Qiu (Citation2020) and Xie and Qiu (Citation2023) proposed new control charts that can suitably accommodate a dynamic temporal pattern and serial correlation in a sequential process.
2.5 Agricultural monitoring
An applied task in smart agriculture is weed detection. That is the timely identification of the location of weeds, usually through cameras installed on moving objects (e.g. tractors, autonomous vehicles, and drones). Rakhmatulin et al. (Citation2021) conducted a literature review of papers on the use of machine vision for weed detection in images with high accuracy. Emphasis was given to deep learning techniques. Such methods have been used in onion seed production (Pannacci et al. Citation2020), wild blueberry (Rehman et al. Citation2019), tomato (Agarwal et al. Citation2020), etc. They concluded that there is no neural network that is ideal for determining the exact location of weeds in real-time.
3 A new monitoring method based on convex hulls and control charting
In this section, we propose a new method for monitoring simultaneously the number and the spatial distribution of events , where s expresses the spatial location (e.g, latitude and longitude) and t refers to the time. This new method exploits control charts based on (a) the evolvement of the number of cases in a broader area in order to detect a global change (using a c-chart or any other appropriate control chart), as well as (b) the level of a newly proposed statistic that uses an advanced graphical technique, the convex hull technique, for detecting hot spots (i.e. concentration of events into one or more small areas). Thus, the proposed method consists of two control charting procedures applied simultaneously to the recorded disease events. The simultaneous application of these two monitoring procedures gives us the flexibility of identifying both a general increase in the number of cases as well as a probable change in the spatial distribution of the events.
3.1 Spatial distribution of the events
Regarding the spatial distribution of the events, the size of the area under investigation needs to be carefully chosen in order to preserve an appropriately, at least, homogeneous stationary Poisson point process in that area. Although the independence of events assumption has been challenged (Yang and Qiu Citation2020), a usual starting point is a null hypothesis that the observed distribution of events is consistent with the homogeneous Poisson process (Diggle et al. Citation1976). Moreover, the homogeneous Poisson process has been used to model disease events, such as the Covid-19 cases (Alawiyah et al. Citation2021). The homogeneous Poisson process is the simplest point process and its realizations exhibit complete spatial randomness (Cressie Citation1994).
A spatial point process N is considered a homogeneous Poisson process with intensity λ if the two following conditions hold (Cressie Citation1994): (i) the number of events in any bounded region E follows a Poisson distribution with mean where
denotes the d-dimensional volume of E, and (ii) given that there are n events in E, those events form an independent random sample from a uniform distribution on E.
More specifically, the homogeneous Poisson process is characterized by
where
is the number of events in the time interval
, λ is the expected number of events that occur per unit of time in a predetermined area s. The mean and variance of the Poisson process is
.
It is worth pointing out that under the assumption of a Poisson process on a plane it can be easily proven that the points in a region are uniformly distributed on A. This result will be used in the following sections to generate a random sample on the plane satisfying the assumption of the homogeneous Poisson process.
3.2 Monitoring the number of events
As a first component of the proposed method, a classical one-sided c-chart for monitoring the mean number of events can be used, under the assumption that the number of events follows a Poisson distribution. This control chart assumes that each sub-group has an equal sample size and its upper control limit is calculated by the th quantile of the Poisson(λ) distribution for a given α (Sim and Lim Citation2008). Under the Poisson(λ) distribution it holds that
.
Here we should clarify that the c-chart is adopted only for simplicity purposes and in order to be easily understood by practitioners. The c-chart can be replaced, for monitoring the number of events, by more advanced charts that may be more appropriate in some cases in which special characteristics, like overdispersion, zero inflation, or seasonality, are observed. These cases are discussed in detail in Section 7.3.
3.3 Monitoring the spatial distribution of the events
The second component of the proposed scheme consists of a new method to monitor the spatial distribution of the events and to identify any patterns that indicate that they violate the homogeneous Poisson process hypothesis on the plane. As already mentioned, we choose appropriately the size of the area under investigation to ensure the validity of the homogeneous Poisson process assumption under the null hypothesis.
The proposed method makes use of the convex hull of a set X of n points. Thus, before introducing the proposed method for monitoring the spatial distribution of the events to detect any deviation from the homogeneous Poisson process assumption is presented, it is necessary to define what a convex hull is.
3.3.1 Convex hull
The Convex hull of a set X of points in the Euclidean plane is the smallest convex polygon that contains X. Every point in X is either on the boundary of the polygon or in its interior. Applications of the convex hull concept can be found in image processing and computer visualization (Goshtasby and Stockman Citation1985; Laurentini Citation1994; Rosin Citation2006), geographic information systems (Getz and Wilmers Citation2004), verification methods (Lee Citation2009), ethology (Griesser et al. Citation2009; Mahoney and Young Citation2017), etc.
The proposed public health monitoring method utilizes the area of a convex hull removing the furthest (most remote) point, with the criterion of the largest reduction of the area by removing a point from the boundary of the convex hull. The rationale of the test is that under the null hypothesis of uniformly distributed events on the plane, the area should be reduced approximately at a constant rate when one of the points on the boundary is removed. In the present context, we proposed to remove the most remote point in order to make the process more sensitive. Removing sequentially the most remote point is also used as an ordering technique for multidimensional data, first proposed by Tukey (Citation1975) and it is referred to as convex hull peeling.
3.3.2 A spatial test statistic based on convex hull
A test statistic that arises naturally for testing the assumption that the events consist of a homogeneous Poisson process with a constant, known, intensity λ0 on a plane can be defined as
(1)
(1) by noticing that under the homogeneous Poisson process assumption, the expected value and the corresponding variance of the number of the events in the area defined by removing the most remote point (1 point) from the convex hull are both equal to
. With Hn we denote the area of the convex hull of the n data, with
the area of the convex hull after removing the first (the furthest) point,
, and with H3 the area of the convex hull with the last 3 points.
Examples of how a convex hull works after the removal of several points/events are given in and . In both examples, the number of events is 60. In the first example, the events are generated under the homogeneous Poisson process assumption (D = 52.0624) while in the second a hotspot is located in the center of the plane resulting in a violation of the aforementioned assumption (D = 3584.69). As one may observe in , the area of Hn is decreasing steadily as points are removed, while in , the respective area is decreasing quickly and large jumps are present.
Fig. 1 An example of a uniformly distributed set of events and how a convex hull works after the removal of several points/events (D = 52.0624).

Fig. 2 An example of a non-uniformly distributed set of events and how a convex hull works after the removal of several points/events. A hotspot is located in the center of the plane (D = 3584.69).

The test statistic D can be depicted in a one-sided control chart, which we call convex-chart, to monitor the spatial distribution of the events. The upper control limit can be determined by the critical values of the test statistic D.
Here we have to note that the test statistic is conditional to the number n of cases observed and to intensity λ. Since this dependency is a complicated one in the next sub-section we provide a simulation study to determine the distribution and thus the critical values of the test statistic D.
3.3.3 Distribution and critical values determination
The proposed method rejects the null hypothesis when its observed value is larger than the corresponding critical value at a designated significance level α. Since the distribution of the test statistic D under the null hypothesis is not available (to the best of our knowledge this is the first time that the test statistic D is introduced), a simulation study was conducted in order to determine (approximate) via simulations the distribution of the test statistic D. More specifically, 106 simulated samples were generated under the homogeneous Poisson process assumption by using events on the plane. For each simulated sample the value of the test statistic D was calculated and stored. Then, using the 106 observed values for each n, 22 different distributions on
were fitted using the GAMLSS package in R (Rigby and Stasinopoulos Citation2005). The pool of the used distributions includes not only some of the most frequently used distributions on
, like the lognormal and the Weibull distributions but also some very flexible ones like the generalized gamma distribution and the Box-Cox t distribution (Rigby and Stasinopoulos Citation2006). Based on this procedure the distribution with the smaller Akaike information criterion (AIC) was identified for each n. For all n, except for n = 70, the distribution with the smaller AIC was the Box-Cox t distribution. For n = 70 the smaller value of AIC was obtained by the Box-Cox Power Exponential distribution. Although, even in this case the Box-Cox t distribution was ranked 2nd among all the candidate distributions. Apart from that the Box-Cox t distribution presented an excellent fit (using a Q-Q plot) providing evidence that the null distribution of the proposed test statistics can be approximated sufficiently by the Box-Cox t distribution. Some caution should be taken whenever an extreme upper quantile, such as
—a frequently used quantile in control charting—is required.
A positive random variable Y is considered to be distributed accordingly to a Box-Cox t distribution, denoted as , if the transformed random variable Z
follows a (truncated) t distribution with τ degrees of freedom where
, is treated as a continuous parameter. The four parameters
may be interpreted as relating to location and more specifically to the median, scale, skewness, and kurtosis of the distribution, respectively. In the MLEs of the four parameters for each n are presented while in the plots of these values against n are presented. In the estimate based on the fractional polynomial (see following paragraphs) and the simulated 1-0.0027 quantile are also presented (in the last two columns). From the plot, it is clear that there is a well-defined functional relationship between the number of events n and each one of the Box-Cox t distribution parameters.
Fig. 3 The fractional polynomial models for the parameters (or the link functions of the parameters) of the along with their estimated values based on 106 simulated samples generated under the homogeneous Poisson process assumption by using
events on the plane.

Table 1 The MLEs of the four parameters for each n based on 106 simulated samples generated under the homogeneous Poisson process assumption and the estimated based on the fractional polynomial (see following paragraphs) and the simulated 1-0.0027 quantile.
To determine a functional relationship among the sample size n and the four parameters of the distribution we relied on the fractional polynomials. Fractional polynomials were introduced by Royston and Altman (Citation1994) for modeling the relationship between a continuous response variable and one or more covariates in general regression models, while a modified version for multivariate analysis was proposed by Sauerbrei and Royston (Citation1999). This method combines backward elimination with a systematic search for a proper transformation of the covariates in order to find the optimal model. In this case, the best second-degree fractional polynomial models for the parameters (or the link functions of the parameters) of the
are given by
and are embedded in the scatterplots of .
The above relationship can be used to approximate the distribution for any n in
and to estimate any quantile of this distribution that can be used to determine the required critical value for the convex-chart. The estimated 1-0.0027 quantile based on the above functional forms of the parameters are given in and are very close to the simulated ones.
Remark 1.
The critical values of the proposed statistics can also be computed directly for any n (and n smaller than 20) with extended simulated procedures as the ones presented before, i.e. by using the simulated quantiles based on the parametric bootstrap approach. While such an approach could provide, in general, extremely accurate critical values, especially when the number of simulated samples is extremely large, we have to mention that the proposed approach offers a quick, robust, and unified approach that approximates very well the critical values for .
3.4 Monitoring algorithm
The steps of the proposed monitoring procedure are described in detail in the form of the following Algorithm.
Step. 1At each time point record the number n of events and the coordinates of each event.
Step. 2Construct an one-sided c-chart for monitoring the mean number of events with Footnote1.
Step. 3Calculate the observed value Dobs of the test statistic D, determine the upper 0.0027 quartile under the null hypothesis for the observed number n of events and construct the convex-chart.
Step. 4 Using both procedures (c-chart and convex-chart) determine if the process is under control or not.
4 Performance investigation
In this section, the performance of the proposed methodology under different scenarios is presented. The first scenario represents the in-control scenario, i.e no global change in the expected number of events and no violation of the homogeneous Poisson process assumption for the events on the plane (Case 1). The second scenario represents a global change and more specifically an increase in the expected number of events (Case 2). The following three scenarios investigate the performance of the proposed methodology in the presence of a hotspot. More specifically, they investigate the influence of the position of the hotspot by considering three different locations, a corner hotspot (Case 3.1), a side hotspot (Case 3.2), an inner hotspot (Case 3.3), by holding the total expected number of events fixed. This situation describes a situation in which some events are moved (or reported) to a different location.
The next two scenarios (labeled as Cases 4.1 and 4.2) investigate the performance of the proposed methodology under an increase in the expected number of events and the presence of a hotspot. The difference between these two cases lies in the fact that in the first case, the hotspot is not created by the relocation of a number of events (as in Cases 3.1, 3.2, and 3.3) but rather by a local increase of the expected number of events in the hotspot section. Case 4.2 on the other hand describes a situation in which a global increase occurs and at the same time, a local increment takes place as well in the hotspot.
The last two scenarios (Cases 5 and 6) investigate the impact of the presence of two hotspots under two different situations, namely under the assumption of a local (Case 5) and a global (Case 6) increase in the expected number of events. Additionally, Case 5 investigates also the influence on the monitoring procedure of the relative position of the two hotspots (Cases 5.1 and 5.2).
In all cases, the number of events and the spatial distribution of the points were monitored with the proposed methodology in order to compute the Average Run Length (ARL), i.e. the number of samples (time records) before an out-of-control condition is indicated. For the number of events, as already mentioned a 3-sigma c-chart was used while for monitoring the spatial distribution of the points the proposed test statistic was implemented. The first signal from both procedures is of interest. In all the samples the number of events was generated using a Poisson distribution. That is, we simultaneously monitor the number of disease events through control charting and the spatial distribution of disease events via convex hulls choosing appropriately the size of the area under investigation in order to preserve population uniformity ensuring that way the validity of the assumption of the homogeneous Poisson process under the null hypothesis.
Case 1. (In-control case).
For the in-control case, an area, and more particularly a square unit, was initially defined and the value of the parameter λ0, of the Poisson distribution was set. Then, n simulated random, uniformly distributed, points on the square unit were generated, where n follows a Poisson(λ0), for . For each t, a c-chart for λ0 with
, and the new method to assess whether the points are uniformly distributed (
) were implemented. The whole procedure was repeated with 2,000 runs. For each run, the sample number of the first signal from both procedures was recorded.
In the first rows of the ARLs, the standard deviation of the RLs, the percent of alarms due to the two charts, and the correlation of the RL of the two methods for are presented. Additionally, the ARL and the standard deviation of the RL distribution are given until the first signal appears in at least one of the CCs, denoted as COM-chart (combined chart). The ARL of the c-chart is notably different from its target value of 370.37 due to the discrete nature of the Poisson distribution. Although the simulated ARL are close to their theoretical values of
252.681, and 353.704 for
, respectively. On the other hand, the ARL of the proposed convex-chart is relatively close to its target value for all λ0.
Table 2 The ARL, the standard deviation of the RL and, the percent of alarms for the c-chart, and the proposed test statistic for monitoring the spatial distribution of the events for all the cases.
Regarding the RL until the first signal (denoted in Table as COM-chart) it is worth noticing that it is smaller than the ARLs of the two charts if only one of the procedures were implemented. This is due to the fact that each observed RL until the first signal is the minimum of two geometric random variables with a probability of success and
, respectively. If the two procedures were independent, the expected RL for
would be 150.447, and 181.173, respectively. Although, in both cases, the simulated ARL for the first signal is smaller that the corresponding values. This indicates that the two procedures are not independent and that some small correlation is presented. This is reflected in the reported values of the correlation of the RL of the two procedures. An approximation of COM-chart ARL may also be calculated by the classical formula
where ARLc and ARLch are the ARL of the c-chart and the convex-chart (see for example Koutras et al. Citation2006). These values are also reported in and are very close to the simulated ones indicating the robustness of the proposed method. Finally, regarding the percent of alarms due to c-chart and the percent of alarms for the new method for monitoring the spatial distribution of the events, it seems that the c-chart produces earlier, as expected due to the smaller ARL, signals than the other.
Case 2. (Global change).
For the second scenario, again the same unit square area was defined but the expected number of events was set equal to , where λ0 is the expected number of events under the null hypothesis and k = 1, 2, 3. This situation describes a global increase of 1, 2, and 3 standard deviations in the expected number of events. For each k, n simulated random points uniformly distributed, were generated on the area, where n follows a Poisson(λ1), for
and the procedure was repeated again 2,000 times. The value of
was used to maintain the number of events relatively small.
The ARL, the standard deviation of the RL, the percent of alarms due to the c-chart, and the percent of alarms from each chart for the case of a global change, are shown in the second block of rows for . The proposed method identifies the change quickly; mainly through the c-chart, as was expected, due to the global change and the absence of a hotspot. As was also expected, as k increases the percentage of the signals due to the c-chart increases and the ARL decreases. It is also worth mentioning that the ARL of the convex-chart also decreases. This is due to the fact that the points are closer than expected for the given λ0 and as a result larger values of the test statistic D are observed.
Case 3. (One hotspot–no global change).
In this case, a singular hotspot of area E was considered. The area E was set equal to 0.08 representing a 8% of the total area under study. Additionally, three different positions of the hotspot were considered (corner hotspot, side hotspot, inner hotspot) and the performance of the proposed methodology was studied by running 2,000 times the following procedure for each position of the hotspot. Initially, λE and were set equal to
and
, with k = 1, 2, 3. These parameters represent the expected number in each section, i.e. hotspot E and the rest of the area E′, of the square unit. The values of λE and
were set in this way so that the expected total number in the square unit to be λ0, so that no global change in the expected number of events to occur. Again the value
was used.
Afterward, nE random points (uniformly) on the subarea E and random points on the remaining area were generated from a Poisson(λE) and Poisson(
) respectively, for
(2,000 runs). In some simulated examples for each case are presented. It is worth mentioning that in these simulated examples the expected number of events if there were not a hotspot in area E, would be
(E = 0.08). The presence of the hotspot increases this number to 1.55, 3.10, and 4.65 for k = 1, 2 and 3, respectively, indicating a relatively small, moderate, and large increase in the expected number of events in section E.
Fig. 4 Simulated examples of a corner hotspot (Case 3.1—left plot), a side hotspot (Case 3.2—-middle plot), an inner hotspot (Case 3.3—right plot), by holding the total expected number of events fixed (κ = 3).

The results in (see lines corresponding to Case 3) present the ARL, the standard deviation of the RL, and the percent of alarms from the c-chart and the proposed test statistic for monitoring the spatial distribution of the events for all three positions of the hotspot (subcase 1: corner hotspot, subcase 2: side hotspot, subcase 3: inner hotspot). For k = 2 and k = 3 the percentage of alarms from the proposed test statistic for monitoring the spatial distribution of the events is larger than the percent of alarms from the c-chart. The difference between these two percentages increases as κ increases. The largest values for this difference are observed for an inner hotspot and the smallest for a side hotspot. The position of the hotspot seems to have also an effect on the ARL. The smaller ARL is achieved for an inner hotspot and the largest for a side hotspot.
It is worth mentioning that the increase in the expected number of events in the hotspot is only 1.55, 3.10, and 4.65, respectively in a total of 30 (λ0) expected events in the area. As a consequence, it is not surprising that for κ = 1 the ARL is not that smaller than the in-control ARL (362.416) for given in the first row of . Of course as κ increases the ARL decreases as expected.
Case 4. (One hotspot–global change).
In this case, two different scenarios were studied under the presence of a hotspot. In the first one (Case 4.1) the presence of the hotspot is not created by the relocation of a number of events (as in Cases 3.1, 3.2, and 3.3) but by a local increase of the expected events which leads to a general increase in the number of events in the total area. The second scenario (Case 4.2) involves, additionally to the local increase, and a global increase in the expected number of events. A simulated example for these two scenarios is presented in . A corner hotspot was used to test the proposed monitoring procedure in a not-so-favorable scenario, as for example, would be the case with an inner hotspot.
Fig. 5 Simulated examples of a hotspot created by a local increase without any global increase (Case 4.1—left plot–κ = 3) and with a global increase of the expected number of events (Case 4.2—right plot–).

Case 4.1.
Following a similar notation to the previous cases the Poisson parameters used for Case 4.1 were ,
and
, with k = 1, 2, 3.
As can be seen in (see lines corresponding to case 4.1), the presence of the hotspot and the change in the expected number of events is identified faster than in the previous scenario. This is mainly due to the fact that the local increase leads to a total increase in the expected number of events. The bigger the change in the hotspot, the easier the detection is, as it is expected. However, the overall increase in events appears to be more easily, or better faster, detected by the c-chart.
Case 4.2.
For this scenario the parameters were used for the Poisson distributions: and
, where
with
and
and
. Thus the expected total number of events in the whole area was set equal to
.
In order to generate the simulated samples, nE random, uniformly distributed, points on the subarea E and random, uniformly distributed, points on the subarea
were generated, where nE and
were randomly generated by a Poisson distribution with parameter λE and
, respectively, for
(2,000 runs).
The ARL, the standard deviation of the RL, the percent of alarms for the c-chart, and the proposed test statistic for monitoring the spatial distribution of the events for this case are presented in (see lines corresponding to case 4.2). Additionally, the values of the Poisson parameters and the expected total number of events are also given. As expected, the out-of-control state of the underline process is quickly identified. The identification of the out-of-control state is mainly done by the c-chart.
Case 5. (Two hotspots–no global change).
The next scenario concerns the case two hotspots were used. In unit square two, no adjacent subareas were selected, both of area E. The Poisson parameters for the distribution of the number of events in each subarea , and
were defined as
and
in order for the expected total number of events in the original 1 × 1 area to be
, the initial assumed expected number of events, where
denoted the expected number of events outside the two hotspots. Additionally, two different positions of the two hotspots were used to identify the possible influence of their relative position on the monitoring procedure. More particularity, in Case 5.1 the hotspots were located at
and
and in Case 5.2 at
and
. Simulated examples of the two cases (labeled as Case 5.1 and 5.2) are presented in .
Fig. 6 Simulated examples of two hotspots (Case 5.1—left plot, Case 5.2—right plot), by holding the total expected number of events fixed ().

To simulate the samples, and
random, uniformly distributed, points on the subareas E1 and E2 respectively and
random, uniformly distributed, points on the subarea
, were generated with
and
follow a Poisson distribution with parameters
, and
, respectively, for
(2,000 runs).
The results are shown in (see lines corresponding to Case 5). It is clear that the more events are “transferred” to the hotspots, the more easily the proposed monitoring method detects the out-of-control stage of the process. The proximity of the two hotspots seems to play a small role. In particular, it appears that the closer the hotspots are, the faster the method detects the problem. This is reasonable as the two hotspots resemble a big hotspot.
Case 6. (2 hotspots–global change).
The last scenario concerns the case where a global change and two hotspots occur simultaneously. In the unit square two not adjacent subareas both of area E were selected. The parameters , and
were defined such that the expected total number of events in the original 1 × 1 area to be λ1 where
for
and
Again the least favorable scenario for the location of the hotspot was used by locating the two hotspots in the opposite corners of the area.
To simulate the samples a similar procedure to Case 5 was followed with the necessary adjustment to the parameters. The case of the smallest change of λ0 (κ = 1) (less favorable for the c-chart) and the two remote hotspots located in the two opposite corners of the area, as in Case 5.2 (less favorable for monitoring the spatial distribution of the events) was studied. The results are shown in (see lines corresponding to Case 6) and a simulated example in .
The small values of the ARL in (see lines corresponding to Case 6) indicate a very quick identification of the out-of-control stage. The identification is made again mainly by the c-chart as there is a significant increase in the expected total number of events.
5 Numerical illustration and comparison
5.1 Numerical illustration
For illustration purposes, the proposed monitoring process was applied in three simulated data sets generated under three different scenarios. More specifically, the proposed method was used to construct typical control charts firstly for the in-control case, i.e. no global change in the expected number of events and no violation of the homogeneous Poisson process assumption of the events on the plane, and secondly for the out-of-control cases: 1) increase of the expected number of events and 2) inner hotspot (Case 3.3), by holding the total expected number of events fixed. For each case, 150 observations, corresponding to 150 distinct time points, were generated. For the two out-of-control scenarios the first 30 observations were generated under an in-control process. For each time point the number n of points was generated under a Poisson(50) distribution and n points were generated on the plane according to the assumed scenario. Then, the c-chart and the new control chart (convex-chart) were constructed.
shows the proposed control charts. In each graph, the control limits are indicated with gray lines. In the upper pair of charts of , which represents the in-control case, all the points fall within the control limits in both charts, indicating that the process is indeed in control. In the following pairs of graphs, it is clear that the process is not in an in-control state. More particularly, in the charts of the second row of it is obvious that only the number of events is increased since a large number of points in the c-chart fall near the UCL and there is also a number of points above the UCL. On the other hand, there is no indication that a violation of the homogeneous Poisson process assumption for the events on the plane occurs since no significant change in the convex-chart is observed. The opposite occurs in the bottom pair of graphs in . These graphs clearly indicate a violation of the homogeneous Poisson process assumption for the events on the plane with no corresponding change in the expected number of events.
5.2 Comparisons
Our method is not directly comparable to Kulldorff’s spatiotemporal scan statistic, given that it assumes that the events represent a homogeneous Poisson process on the plane. However, given that our method is a prospective one, for comparison reasons, we conducted a prospective analysis with Kulldorff’s method to the same simulated data sets through the Satscan software.
For the in-control case, Kulldorff’s method correctly did not identify any statistically significant cluster. The same result also arose in the case we have an increase in the expected number of events, due to the fact that Kulldorff’s method monitors only the distribution of the events and not their number. In the third case, i.e. an inner hotspot with no global change on the expected number of events, Kulldorff’s method identified a statistically significant (p < 0.001) cluster located at (0.55,0.55) concerning the time period 76 to 150.
From the above results, it derives that the proposed method challenges Kulldorff’s method since the spatiotemporal scan statistic is unable to detect the increase of the expected number of events while our method detects the hotspot when the total expected number of events is held fixed much earlier than Kulldorff’s method.
Before concluding this subsection, we would like to address a method called one-class classification, which utilizes the smallest possible volume to envelop the in-control dataset (Sun and Tsung Citation2003). Although the rationale for surrounding data points with a support vector-based boundary is similar to using a convex hull, the one-class classification-based control chart varies considerably from the proposed methodology both in logic and implementation, which makes the two methods not straightforwardly comparable.
6 Application
For illustration purposes, the proposed monitoring scheme is applied in a real data set concerning the emergency calls to 911, in Montgomery Country, Pennsylvania, USA (Chirico Citation2020). 911 is a three-digit emergency telephone number for any police, fire, or medical incident. The data set covers a time period from 12/10/2015 to 4/28/2020 and provides the exact location from which the call was made (Latitude, Longitude, and Township), the Timestamp of the call, and the description of the emergency (three categories: Fire, Traffic, Emergency Medical Services (EMS), including a short description).
6.1 Data description and preprocessing
The data set contains 624,047 records of calls to 911 from the 68 Townships/Boroughs of Montgomery Country, Pennsylvania, USA. From these records, the calls made from Abington Township, Rockledge, and Jenkintown boroughs were selected for further analysis (see ). The total population of these three areas was 65,859 as of the 2020 census and they have a total area of 1,379.38 km2.
Fig. 9 Location of Abington Township (red), Rockledge (light blue), and Jenkintown (dark blue) in Montgomery County, Pennsylvania, United States. The location of Abington Township in Pennsylvania and in the United States are given in the embedded maps (Wikipedia contributors Citation2023, edit by authors).

In order to apply the proposed monitoring scheme we restricted ourselves to the EMS calls that activate a system that provides emergency medical care for the patient(s). Moreover, we considered a time interval of three days as a sampling unit, resulting in 533 total samples (the first two days were removed from the analysis in order to complete three days at the end of the study period).
Additionally, only one call was kept whenever several calls were made from the same latitude and longitude, and at the same date since they obviously concerned the same incident. These resulted in a total of 17,516 unique records/calls, 15,302 from Abington Township and 1,528 and 686 from Rockledge, and Jenkintown boroughs, respectively.
6.2 Results–monitoring procedure
To apply the proposed monitoring procedures the λ0 intensity parameter of the assumed underlined homogeneous Poisson process has first to be determined. Since on average 32.929 calls were made in a three days interval, the value 30 (10 EMS calls per day) was adopted.
shows the proposed control charts for the EMS calls for the Abington Township, Rockledge, and Jenkintown boroughs using three days intervals as sampling units. In each graph, the control limits are indicated with gray lines and were calculated using the value 30 (10 EMS calls per day) for the intensity parameter of the assumed underlined homogeneous Poisson process. From the left chart (c-chart) it is clear that 4 observations, all at the second half of the time period (date intervals 384, 439, 488, and 507), are above the UCL indicating a large number of calls in these three days intervals. On the other hand, at the same time period, there is no indication that a violation of the homogeneous Poisson process assumption for the events on the plane occurs since no observation above the control limit in the convex-chart is observed. The same does not hold for the first half of the period study. In the first half of the study period, there are five three days intervals (date intervals 31, 121, 135, 156, and 244) that the assumption of a homogeneous Poisson process seems to be violated.
Fig. 10 The control charts for the proposed monitoring procedures for the EMS calls for the Abington Township, Rockledge, and Jenkintown boroughs using three days intervals as sampling units. In each graph, the control limits are indicated with gray lines.

In maps of the study area in four different date intervals with the exact locations of the EMS calls made in that period are presented. The upper left map presents the locations of the EMS calls over the third date interval in which no violation of the underline assumptions was observed. The upper right map corresponds to the calls made in the date interval when a large number of calls was observed. The two maps in the bottom line of correspond to the calls received in date intervals 135 and 244, respectively, in which a violation of the homogeneous Poisson process assumption was identified. From these figures, it is clear that in these two date intervals, the calls were not distributed in the entire area but there were concentrated in some parts of the study area.
Fig. 11 Spatial distribution of the EMS calls made from the study area in four different three days intervals.

Applying a prospective space-time analysis through Kulldorff’s method to the data resulted in a non-significant cluster consisting of 294 cases with coordinates (40.117499 N, 75.109481 W) and a radius of 0.76 km concerning the time period 524 to 533. It is obvious that the proposed method detected hotspots and increased number of events in different time intervals while Kulldorff’s method actually failed to detect any such irregular behavior.
At this point, we should mention that apart from the determination of the intensity parameter λ0, the proposed method does not require any parameter optimization (e.g., spatial and temporal cluster size, minimum number of cases in a cluster, etc), as Kulldorff’s method requires. This allows the new methodology to be applied directly to the data easily and quickly.
7 Adjusting for heterogeneity and other key assumptions violations
In several real cases, the key assumption that, under normal conditions, disease events can be described by a homogeneous Poisson process is violated. According to the literature spatial health data monitoring must allow for three fundamental issues: (i) the heterogenity of the population at risk, (ii) the heterogeneity under the null hypothesis, and (iii) features, such as overdispersion, zero inflation, or seasonality, that are often present in spatio-temporal data. In the first two paragraphs of this section, we treat the case of homogeneous Poisson assumption violation while in the third sub-section, we discuss solutions for other fundamental issues.
7.1 The c chart adjusted for heterogeneity
Often, disease variation within small areas occurs within a population that is spatially varying. If the population is not taken into account then there will be many false positives when clustering is considered. Furthermore, the spatial distribution of disease is mostly characterized by extra heterogeneity related to various unobserved/unknown confounders. If this heterogeneity is not accounted for under the null hypothesis then the test procedure will likely be flawed. Thus, in this section, we provide guidance in order to adjust for these via the inclusion of predictors and appropriate weights.
In order to account for such characteristics an “adjusted” c-chart for counts can be used. The adjustment can be made through a Poisson regression model which is applied when the response is counted, as the number of events. For a vector of explanatory variables , the Poisson regression model has form
where μ is the mean of a Poisson distribution and α, β are the parameters of the model. A c-chart can be used to monitor the estimated by such a model fitted using in-control data. A similar methodology of adjustment was proposed by Bersimis et al. (Citation2017a) while Sachlas et al. (Citation2019) provided an extensive literature review on risk-adjusted control charts.
7.2 The convex-chart adjusted for heterogeneity
In order to monitor the spatial distribution of disease events via convex hulls, under heterogeneity, we can include weight in the test statistic D. In this case, the test statistic will be
where wj is a weight representing the possibility of a case being an event. For example, the weight can be the ratio of the area density to the mean density. The control limits of the convex-chart can be obtained through simulation.
7.3 Monitoring in the case of other key assumptions violations
Spatio-temporal data are often characterized by features, such as overdispersion, zero inflation, or seasonality. In such cases, the c-chart, adopted for simplicity purposes, can be replaced by more advanced charts that can take account of such characteristics.
For example, in the case of zero inflation, we can use the one-sided cJ-chart proposed by Sim and Lim (Citation2008), which is based on an upper Jeffreys prior interval with Poisson parameter estimated from the zero-inflated Poisson model. In the case of overdispersion, we can replace the c-chart with an appropriate CUSUM or EWMA chart for monitoring homogeneous and non-homogeneous negative binomial disease counts (Sparks et al. Citation2010, Citation2011). When excessive cross-correlation is present, we can use the Z-chart of Kalgonda and Kulkarni (Citation2004). This chart is based on the single-step finite intersection test and enables both the detection of the out-of-control status and the identification of the variable or variables responsible for the out-of-control situation. Whenever serial dependence is strong probability limits can also be used (see, for example, Weiß and Testik Citation2019). However, although there are various models for spatiotemporal counts, which account for typical features such as those mentioned above (see, for example, Held and Paul Citation2012; Bracher and Held Citation2022; Jahn et al. Citation2023), we made the hypothesis that disease events consist of a homogeneous Poisson process in the plane, which is a plausible hypothesis in epidemiology and public health (Charu et al. Citation2017; Dworkis et al. Citation2020; Jesri et al. Citation2021). Similar procedures can also be applied in the convex-chart in case of any violation as the ones presented above. For example, in the case of autocorrelation, the use of an EWMA version of the convex-chart can also be used.
8 Conclusions
In this paper, a new biosurveillance method based on control charting and convex hulls was presented. More specifically, the proposed method utilizes the c-chart (or any other appropriate CC) to identify a global change in the number of disease events and a monitoring procedure based on the change of the area of a convex hull when the furthest point (with criterion the largest reduction of the area by removing a point from the boundary of the convex hull) is removed to identify one or more hotspots of disease events which may evolve into outbreaks.
The rationale of the test is that under the null hypothesis of the homogeneous Poisson process for the events on the plane, the area should be reduced approximately at a constant rate of when the most remote point is removed. The test statistic is corrected in order for the original size (with all the points) to have size 1. The performance investigation showed excellent performance of the new monitoring procedure. The comparison shows that the new method is comparable to Kulldorff’s spatiotemporal scan statistic.
As we have already mentioned, this is the first time that the test statistic D is used. Thus, we approximated its null distribution through a simulation study. An interesting topic to investigate is the mathematical derivation of the distribution of the test statistic and compare it with other spatial scan statistics.
Somebody can argue how often one needs to collect the sample or how large the initial area of investigation needs to be. Both the sample collection frequency and the size of the initial area of investigation can be derived by the disease incidence. Run rules can also be applied to the proposed procedure to increase the sensitivity of the method. Instead of the c-chart, a more complex chart, such as a CUSUM or an EWMA, can be used to identify the global change, depending on the particular conditions (assumptions) of the problem. Using the normal approximation to Poisson, we would be able to propose a bivariate control chart. All these are topics for further research.
Another interesting topic for future research is the extension of the proposed method to handle multiple quality variables, such as monitoring the incidence rates of multiple diseases, by incorporating an appropriate multivariate control chart for attributes and appropriately adjusting the proposed convex-chart.
Disclosure statement
No potential conflict of interest was reported by the author(s).
Notes
1 The interested practitioner may replace c-chart by using a more advanced control chart (i.e. an EWMA control chart) depending on his/her familiarity with control charting procedures and the specific conditions (assumptions) of the problem
References
- Agarwal M, Gupta SKr, Biswas KK. 2020. Development of efficient CNN model for tomato crop disease identification. Sustain Comput Informatics Syst. 28:100407.
- Alawiyah M, Johar D, Ruchjana B. 2021. Homogeneous poisson process in daily case of covid-19. J Phys Conf Ser. 1722:012078.
- Anderson N, Titterington D. 1997. Some methods for investigating spatial clustering, with epidemiological applications. J R Stat Soc Series A (Stat Soc) 160(1):87–105.
- Bersimis S, Economou P. 2017. The use of length-biased distributions in statistical monitoring. Aust N Z J Stat. 59(2):155–167.
- Bersimis S, Sachlas A. 2022. Surveilling public health through statistical process monitoring: a literature review and a unified framework. Commun Stat Case Stud Data Anal Appl. 8(3):515–543.
- Bersimis S, Triantafyllopoulos K. 2020. Dynamic non-parametric monitoring of air-pollution. Methodol Comput Appl Probab. 22:1457–1479.
- Bersimis S, Chalkias C, Anthopoulou T. 2014. Detecting and interpreting clusters of economic activity in rural areas using scan statistic and lisa under a unified framework. Appl Stoch Models Bus Ind. 30(5):573–587.
- Bersimis S, Sgora A, Psarakis, S. 2016. The application of multivariate statistical process monitoring in non-industrial processes. Qual Technol Quant Manag. 15:526–549.
- Bersimis S, Sachlas A, Sparks R. 2017a. Performance monitoring and competence assessment in health services. Methodol Comput Appl Probab. 19(4):1169–1190.
- Bersimis S, Degiannakis S, Georgakellos D. 2017b. Real-time monitoring of carbon monoxide using value-at-risk measure and control charting. J Appl Stat. 44(1):89–108.
- Bhowmick T, Griffin A, Maceachen A, Kluhsman B, Lengerich EL. 2008. Informing geospatial toolset design: Understanding the process of cancer data exploration and analysis. Health Place 14(3):576–607.
- Blangiardo M, Boulieri A, Diggle P, Piel FB, Shaddick G, Elliott P. 2020. Advances in spatiotemporal models for non-communicable disease surveillance. Int J Epidemiol. 49(Supplement_1):i26–i37.
- Boutsikas M, Koutras M. 2003. Bounds for the distribution of two-dimensional binary scan statistics. Probab Eng Inf Sci. 17(4):509–525.
- Bracher J, Held L. 2022. Endemic-epidemic models with discrete-time serial interval distributions for infectious disease prediction. Int J Forecast. 38(3):1221–1233.
- Bravata D, McDonald K, Smith W, Rydzak C, Szeto H, Buckeridge DL, Haberland C, Owens DK. 2004. Systematic review: surveillance systems for early detection of bioterrorism-related diseases. Ann Internal Med. 140(11):910–922.
- Caldwell G. 1989. Time-space cancer clusters. Health Environ Digest. 3(5):1–3.
- Charu V, Zeger S, Gog J, Bjørnstad ON, Kissler S, Simonsen L, Grenfell BT, Viboud C. 2017. Human mobility and the spatial transmission of influenza in the United States. PLoS Comput Biol. 13(2):e1005382.
- Chirico M. 2020. Emergency - 911 calls. Accessed: 2013-06-24.
- Correia F, Neveda R, Oliveira P. 2011. Chronic respiratory patient control by multivariate charts. Int J Health Care Qual Assur. 24(8):621–643.
- Cressie N. 1994. Models for spatial processes. In: Stanford JL, Vardeman SB, editors. Statistical Methods for Physical Science, volume 28 of Methods in Experimental Physics. New York: Academic Press. p. 93–124.
- Darling R, Waterman M. 1985. Matching rectangles in d-dimensions: algorithms and laws of large numbers. Adv Math. 55(1):1–12.
- Diggle PJ, Besag J, Gleaves JT. 1976. Statistical analysis of spatial point patterns by means of distance methods. Biometrics 32:659–667.
- Duczmal L, Kulldorff M, Huang L. 2006. Evaluation of spatial scan statistics for irregularly shaped clusters. J Comput Graph Stat. 15(2):428–442.
- Dworkis DA, Marvel J, Sanossian N, Arora S. 2020. Neighborhood-level stroke hot spots within major united states cities. Amer J Emer Med. 38(4):794–798.
- Fanaee-T H, Gama J. 2015. Eigenspace method for spatiotemporal hotspot detection. Expert Syst. 32(3):454–464.
- Fricker R, Chang J. 2008. A spatio-temporal methodology for real-time biosurveillance. Qual Eng. 20(4):465–477.
- Frisén M, Andersson E, Schiöler L. 2010. Evaluation of multivariate surveillance. J Appl Stat. 37(12):2089–2100.
- Getz W, Wilmers C. 2004. A local nearest-neighbor convex-hull construction of home ranges and utilization distributions. Ecography 27(4):489–505.
- Glaz J, Naus J, Wallenstein S. 2001. Scan statistics. New York: Springer.
- Goshtasby A, Stockman GC. 1985. Point pattern matching using convex hull edges. IEEE Trans Syst Man Cybern SMC-15(5):631–637.
- Griesser M, Barnaby J, Schneider N, Figenschau N, Wright J, Griffith S, Kazem A, Russell A. 2009. Influence of winter ranging behaviour on the social organization of a cooperatively breeding bird species, the apostlebird. Ethology 115(9):888–896.
- Haiman G. 2000. Estimating the distributions of scan statistics with high precision. Extremes 3(4):349–361.
- Held L, Paul M. 2012. Modeling seasonality in space-time infectious disease surveillance data. Biom J. 54(6):824–843.
- Hjalmars U, Kulldorff M, Gustafsson G, Nagarwalla N. (1996). Childhood leukaemia in Sweden: using gis and a spatial scan statistic for cluster detection. Stat Med. 15(7–9):707–715.
- Jahn M, Weiß, CH, Kim H-Y. 2023. Approximately linear ingarch models for spatio-temporal counts. J R Stat Soc Series C Appl Stat. 72(2):476–497.
- Jesri N, Saghafipour A, Koohpaei A, Farzinnia B, Jooshin MK, Abolkheirian S, Sarvi M. 2021. Mapping and spatial pattern analysis of covid-19 in Central Iran using the local indicators of spatial association (lisa). BMC Public Health 21:1–10.
- Kalgonda A, Kulkarni S. 2004. Multivariate quality control chart for autocorrelated processes. J Appl Stat. 31(3):317–327.
- Kammerer S, Shang N, Althomsons S, Haddad M, Grant J, Navin T. 2013. Using statistical methods and genotyping to detect tuberculosis outbreaks. Int J Health Geograph. 12(1):15–22.
- Kleinman K, Lazarus R, Platt R. 2004. A generalized linear mixed models approach for detecting incident clusters of disease in small areas, with an application to biological terrorism. Amer J Epidemiol. 159(3):217–224.
- Kman N, Bachmann D. 2012. Biosurveillance: a review and update. Adv Prevent Med. 2012:301408.
- Koutras M, Bersimis S, Antzoulakos D. 2006. Improving the performance of the chi-square control chart via runs rules. Methodol Comput Appl Probab. 8:409–426.
- Kristoffersson A, Lindén M. 2020. Wearable sensors for monitoring and preventing noncommunicable diseases: a systematic review. Information 11(11):521.
- Kroll M, Phalkey RK, Kraas F. 2015. Challenges to the surveillance of non-communicable diseases–a review of selected approaches. BMC Public Health 15(1):1–12.
- Kulldorff M. 1997. A spatial scan statistic. Commun Stat Theory Methods 26(6):1481–1496.
- Kulldorff M. 2001. Prospective time periodic geographical disease surveillance using a scan statistic. J R Stat Soc Series A Stat Soc. 164(1):61–72.
- Kulldorff M, Nagarwalla N. 1995. Spatial disease clusters: detection and inference. Stat Med. 14(8):799–810.
- Kulldorff M, Feuer E, Miller B, Freedma L. 1997. Breast cancer clusters in the Northeast United States: a geographic analysis. Amer J Epidemiol. 146(2):161–170.
- Laurentini A. 1994. The visual hull concept for silhouette-based image understanding. IEEE Trans Pattern Anal Mach Intel. 16(2):150–162.
- Lee M. 2009. An enhanced convex-hull edge method for flatness tolerance evaluation. Comput-Aided Des. 41(12):930–941.
- Mahoney P, Young J. 2017. Uncovering behavioural states from animal activity and site fidelity patterns. Methods Ecol Evol. 8(2):174–183.
- Marshall R. 1991. A review of methods for the statistical analysis of spatial patterns of disease. J R Stat Soc Series A (Stat Soc) 154(3):421–441.
- Moitra P, Sinha S. 2019. Localized spatial distributions of disease phases yield long-term persistence of infection. Sci Rep. 9(1):20309.
- Naus J. 1965. Clustering of random points in two dimensions. Biometrika 52:263–267.
- Pannacci E, Farneselli M, Guiducci M, Tei F. 2020. Mechanical weed control in onion seed production. Crop prot. 135:105221.
- Park J, Yoon S, Na M, Song H. 2015. The effects of air pollution on mortality in South Korea. Proc Environ Sci. 26:62–65.
- Rakhmatulin I, Kamilaris A, Andreasen C. 2021. Deep neural networks to detect weeds from crops in agricultural environments in real-time: a review. Remote Sens. 13(21):4486.
- Rehman TU, Zaman QU, Chang YK, Schumann AW, Corscadden KW. 2019. Development and field evaluation of a machine vision based in-season weed detection system for wild blueberry. Comput Electron Agric. 162:1–13.
- Rigby R, Stasinopoulos D. 2006. Using the box-cox t distribution in gamlss to model skewness and kurtosis. Stat Model. 6(3):209–229.
- Rigby RA, Stasinopoulos DM. 2005. Generalized additive models for location, scale and shape, (with discussion). Appl Stat. 54:507–554.
- Rogerson P. 2005. Spatial surveillance and cumulative sum methods. Hoboken, NJ: Wiley-Blackwell. p. 95–114.
- Rogerson P, Yamada I. 2004. Monitoring change in spatial patterns of disease: comparing univariate and multivariate cumulative sum approaches. Stat Med. 23(14):2195–2214.
- Rolka H, Burkom H, Cooper G, Kulldorff M, Madigan D, Wong W. 2007. Issues in applied statistics for public health bioterrorism surveillance using multiple data streams: research needs. Stat Med. 26(8):1834–56.
- Rosin P. 2006. Training cellular automata for image processing. In: Kalviainen H, Parkkinen J, Kaarna A, editors. Image Analysis. Berlin, Heidelberg: Springer. p. 195–204.
- Rossi G, Lampugnani L, Marchi M. 1999. An approximate cusum procedure for surveillance of health events. Stat Med. 18(16):2111–2122.
- Royston P, Altman D. 1994. Regression using fractional polynomials of continuous covariates: Parsimonious parametric modelling. J R Stat Soc Series C (Appl Stat) 43(3):429–467.
- Sachlas A, Bersimis S, Psarakis S. 2019. Risk-adjusted control charts: theory, methods, and applications in health. Stat Biosci. 11:630–658.
- Sauerbrei W, Royston P. 1999. Building multivariable prognostic and diagnostic models: transformation of the predictors by using fractional polynomials. J R Stat Soc Series A (Stat Soc) 162(1):71–94.
- Schiöler L, Frisén M. 2012. Multivariate outbreak detection. J Appl Stat. 39(2):223–242.
- Shmueli G, Burkom H. 2010. Statistical challenges facing early outbreak detection in biosurveillance. Technometrics 52(1):39–51.
- Sim CH, Lim MH. 2008. Attribute charts for zero-inflated processes. Commun Stat Simul Comput. 37(7):1440–1452.
- Sowmyanarayanan T, Mukhopadhya A, Gladstone B, Sarkar R, Kang G. 2008. Investigation of a hepatitis a outbreak in children in an urban slum in Vellore, Tamil Nadu, using geographic information systems. Indian J Med Res. 128(1):32–37.
- Sparks R. 2012. Spatially clustered outbreak detection using the ewma scan statistics with multiple sized windows. Commun Stat Simul Comput. 41(9):1637–1653.
- Sparks RS, Keighley T, Muscatello D. 2010. Early warning cusum plans for surveillance of negative binomial daily disease counts. J Appl Stat. 37(11):1911–1929.
- Sparks RS, Keighley T, Muscatello D. 2011. Optimal exponentially weighted moving average (ewma) plans for detecting seasonal epidemics when faced with non-homogeneous negative binomial counts. J Appl Stat. 38(10):2165–2181.
- Sun R, Tsung F. 2003. A kernel-distance-based multivariate control chart using support vector methods. Int J Prod Res. 41(13):2975–2989.
- Tsui K, Chiu W, Gierlich P, Goldsman D, Liu X, Maschek T. 2008. A review of healthcare, public health, and syndromic surveillance. Qual Eng. 20(4):435–450.
- Tukey J. 1975. Mathematics and the picturing of data. In: Proceedings of the International Congress of Mathematicians, Vancouver, 1975. Vol. 2, p. 523–531.
- Unkel S, Farrington P, Garthwaite P, Robertson C, Andrews N. 2011. Statistical methods for the prospective detection of infectious disease outbreaks: a review. J R Stat Soc Series A (Stat Soc) 175(1):49–82.
- Wand H, Ramjee G. 2010. Targeting the hotspots: investigating spatial and demographic variations in hiv infection in small communities in South Africa. J Int AIDS Soc. 13:41.
- Weiß CH, Testik MC. 2019. Risk-based metrics for performance evaluation of control charts. Qual Reliab Eng Int. 35(1):280–291.
- Wikipedia contributors. 2023. Abington township, montgomery county, pennsylvania; [accessed 2013 June 24]. https://en.wikipedia.org/w/index.php?title=Abington_Township,_Montgomery_County,_Pennsylvania&oldid=1161175928.
- Woodall W. 2006. The use of control charts in health-care and public-health surveillance. J Qual Technol. 38(2):89–104.
- Woodall W, Montgomery D. 2014. Some current directions in the theory and application of statistical process monitoring. J Qual Technol. 46(1):78–94.
- Xie X, Qiu P. 2023. Control charts for dynamic process monitoring with an application to air pollution surveillance. Ann Appl Stat 17(1):47–66.
- Yan H, Paynabar K, Shi J. 2018. Real-time monitoring of high-dimensional functional data streams via spatio-temporal smooth sparse decomposition. Technometrics 60(2):181–197.
- Yang K, Qiu P. 2020. Online sequential monitoring of spatio-temporal disease incidence rates. IISE Trans 52(11):1218–1233.
- Zhang J, Li Z, Wang Z. 2010. A multivariate control chart for simultaneously monitoring process mean and variability. Comput Stat Data Anal 54(10):2244–2252.
- Zhao Y, Zeng D, Herring AH, Ising A, Waller A, Richardson D, Kosorok MR. 2011. Detecting disease outbreaks using local spatiotemporal methods. Biometrics 67(4):1508–1517.