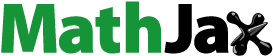
Abstract
A proof of the continuous parameter martingale convergence theorem is provided. It relies on a classical martingale inequality and the almost sure convergence of a uniformly bounded non-negative super-martingale. A probabilistic proof of Liouville’s theorem is also presented applying the continuous parameter martingale convergence theorem.
1 Introduction
Unless otherwise stated, in this article all stochastic processes are defined on a filtered probability space and “almost surely” is abbreviated “a.s.”
The continuous parameter martingale convergence theorem states the following:
Theorem 1.1.
Let be a right-continuous integrable sub-martingale.
If
then there exists an integrable random variable X such that
a.s.
The limit
exists a.s. in
Over the years, the martingale convergence theorem has received much interest in the probability literature.
Doob (Citation1953) gave an original proof of the continuous parameter martingale convergence theorem applying the up-crossing lemma (see also Dellacherie and Meyer Citation1982 and Karatzas and Shreve Citation1998 for more details). Helms and Loeb (Citation1982) presented another proof of Theorem 1.1 (1) employing non-standard analysis. Kruglov (Citation2009) deduced Theorem 1.1 from convergence theorems of separable sub-martingales.
Various interesting proofs of the discrete parameter martingale convergence theorem are also discovered periodically. Doob (Citation1940) first presented a proof without up-crossings. Isaac (Citation1965) provided a proof using classical martingale inequalities and ingenious reductions. Garsia (Citation1970) gave a proof due to E. Bishop applying technical Jensen-like inequalities. Dinges (Citation1970) obtained a proof from a special point-wise criterion of convergence. Meyer (Citation1972) offered a proof due to S. D. Chatterji using convergence theorems of closed martingales. Lamb (Citation1973) gave a functional analytic proof. Austin, Edgar, and Tulcea (Citation1974) provided a proof applying an approximation lemma. Chacon (Citation1974) and Chen (Citation1976) offered proofs using a version of Fatou’s lemma. Chen (Citation1981) presented a proof employing elementary martingale theorems. Al-Hussaini (Citation1981) obtained a proof from a projective limit and Banach’s principle. Petersen (Citation1983) provided a proof due to J. Horowitz using a simple decomposition and an elementary optional sampling theorem. Malliavin (Citation1995) offered a proof applying a sample function property of martingales. Kruglov (Citation2001) derived a proof by approximating random variables. Garling (Citation2007) gave a proof applying Banach-Alaoglu theorem.
Motivated by the historical interest in this problem, the purpose of this article is to present a new elementary proof of Theorem 1.1, avoiding the usual up-crossing inequality and using the classical martingale inequality:(1)
(1) where
and
is an integrable super-martingale.
The discrete parameter martingale convergence theorem is also needed along with the following decomposition theorem.
Theorem 1.2
(Krickerberg decomposition). Let be an integrable sub-martingale for which
. Then, there exist a non-negative martingale
and a non-negative super-martingale
such that
and for all
The reader is referred to Dellacherie and Meyer (Citation1982), Karatzas and Shreve (Citation1998), Krickeberg (Citation1965), and Revuz and Yor (Citation1999) for a proof of inequality (1) and Theorem 1.2.
Lastly, we note that our approach gives a new proof of the theorem on the convergence of an integrable reversed discrete parameter sub-martingale (see Chow and Teicher Citation1997 and Neveu Citation1975 for a general statement and a proof using the up-crossing lemma).
The article is organized as follows. In Section 2 we prove convergence theorems for non-negative super-martingales. In Section 3 we prove Theorem 1.1. Finally, in Section 4 we apply Theorem 1.1 to give a probabilistic proof of Liouville’s theorem.
2 Preliminary tools
In this section we present the necessary tools for our proof of Theorem 1.1.
The following lemma is crucial for the proof of Theorem 2.2 below.
Lemma 2.1.
If Y is an integrable random variable, then converges to
in
Proof.
For every let
We fix
We consider first the case where We have
We note that the sequence is non-increasing and bounded below, hence it converges in
Consequently, there exists such that
and H is
-measurable.
Sinceit follows that
a.s.
Turning to the general case, we have for all
Considering and from the special case which we already proved, we deduce that for every
Applying the dominated convergence theorem, we conclude the proof. □
We provide next a theorem on the almost sure convergence of a uniformly bounded non-negative super-martingale.
Theorem 2.2.
If is an integrable super-martingale such that
then there exist integrable random variables Y and
such that
a.s. and
a.s.
Proof.
First, we will prove the almost sure convergence at
Since
it follows from the discrete parameter martingale convergence theorem that
converges a.s. to a random variable Y such that
a.s.
To prove the result in its generality, we begin by fixing
and
Noticing that
is a super-martingale relative to
and applying inequality (1), we obtain that for all
We note that
from the dominated convergence theorem.
Hence for any
and all
Taking
and applying the dominated convergence theorem, we conclude that for all
in other words
a.s.
The proof of the almost sure convergence at
is essentially the same as before, therefore it’s sufficient to show that the sequence
converges a.s.
We will provide an elegant proof that doesn’t use the up-crossing inequality.
For every
let
and
We note that the sequence
is non-decreasing and bounded above, hence it has a finite limit denoted by l.
Also for every
a.s., therefore by monotone convergence theorem we have
So
is a sequence of integrable random variable such that
Next, we check that
is an integrable martingale relative to
We fix
.
is
-measurable and integrable. We also have
Consequently,
is a martingale and hence for all
a.s.
It follows from Lemma 2.1 that
converges to
in
Lastly, noticing that
is a super-martingale relative to
and using the discrete version of inequality (1), we have for every
and every
Letting
we obtain that for any
and all
So for every
concluding the proof. □
Remark 2.3.
The proof in Theorem 2.2 of the almost sure convergence at relies on Doob decomposition of an integrable reversed discrete parameter sub-martingale (we refer to Dudley Citation2002 for a general statement and a proof).
We end this section by stating and proving a general version of Theorem 2.2.
Theorem 2.4.
Let is a non-negative super-martingale. Then, the limits
and
exist a.s. in
Further, if the sample paths of
are right-continuous, then
and
exist a.s. in
Proof.
We will only prove the almost sure existence of the limit at the proof is analogous at
.
The idea is to truncate properly so that the super-martingale property is preserved.
We fix
is a non-negative super-martingale uniformly bounded by q. We deduce from Theorem 2.2 that
(q,
) a.s.
We also have the following relations:
So for every
a.s. Hence
a.s., yielding that
exists a.s. in
If is right-continuous, then
a.s. □
Remark 2.5.
Theorem 2.4 holds true if is replaced by a countable dense subset D of
3 Proof of the continuous parameter martingale convergence theorem
Finally, we are ready to prove our theorem.
Proof of Theorem 1.1.
Since the sample paths of are right-continuous, it’s sufficient to prove that the limits of
at
and
exist almost surely.
Applying Theorem 1.2, there exist a non-negative martingale
and a non-negative super-martingale
such that
and for every
Theorem 2.4 yields that
and
Wr exist a.s. in
we denote these almost sure limits by U and W, respectively.
It follows by Fatou’s lemma that U and W are integrable, in particular they are finite a.s. and hence
a.s.
To verify the result, we need to write Xr suitably.
We note that
is an integrable martingale such that for all
a.s., so
is a non-negative super-martingale.
Applying again Theorem 2.4 and since
the limits
and
exist a.s. in
and
respectively.
By writing for every
we conclude that
exists a.s. in
□
Remark 3.1.
Applying the procedure used in proving Theorem 1.1 we can show that if D is a countable dense subset of and
is an integrable sub-martingale, then there exist integrable stochastic processes
and
such that for every
a.s.,
a.s.,
a.s., and
a.s. by the following sequence of arguments:
For all
and
is a non-negative integrable super-martingale.
For every
a.s. and
a.s.
There exist integrable stochastic processes
and
such that for all
a.s.,
a.s.,
a.s., and
a.s.
We refer to Dellacherie and Meyer (Citation1982) for another proof using the up-crossing inequality.
4 Application to Liouville’s theorem
In this section we apply Theorem 1.1 to prove the following classical theorem from harmonic analysis.
Theorem 4.1
(Liouville’s theorem). Let If
is a harmonic function such that
then
Proof.
Let be the d-dimensional Euclidean space,
be the d-dimensional Brownian motion starting from
be the Lebesgue measure on
and
For all let
Since we obtain by integration by parts that
It follows by Fubini’s theorem that
Taking and applying the dominated convergence theorem, we find that
Next, we check that is an integrable martingale relative to
For every is
-measurable and integrable. We also have
Consequently, is a continuous martingale relative to
such that
It follows from Theorem 1.1 that there exists an integrable random variable ζ such that a.s.
Noticing that for all
we get that
Considering and using the dominated convergence theorem, we obtain that
and hence
Therefore
Applying the dominated convergence theorem, we conclude that
□
Remark 4.2.
An alternative approach to Theorem 4.1 is to use Itô’s formula, Theorem 1.1, and Blumenthal’s 0-1 law (see Chung and Williams Citation2014 for more details).
Disclosure Statement
No potential conflict of interest was reported by the author(s).
References
- Al-Hussaini AN. 1981. A projective limit view of L1-bounded martingales. Rev Roumaine Math Pures Appl. 26:51–54.
- Austin DG, Edgar GA, Tulcea AI. 1974. Pointwise convergence in terms of expectations. Z. Wahrscheinlichkeitstheorie verw. Gebiete 30:17–26.
- Chacon RV. 1974. A “Stopped” proof of convergence. Adv Math. 14:365–368.
- Chen R. 1976. A simple proof of a theorem of Chacon. Proc Amer Math Soc. 60:273–275.
- Chen LHY. 1981. Martingale convergence via the square function. Proc Amer Math Soc. 83:125–127.
- Chow YS, Teicher H. 1997. Probability theory: independence, interchangeability, martingales. 3rd ed. New York: Springer-Verlag.
- Chung KL, Williams RJ. 2014. Introduction to stochastic integration. 2nd ed. New York: Springer.
- Dellacherie C, Meyer PA. 1982. Probabilities and potential B: theory of martingales. Amsterdam: North-Holland.
- Dinges H. 1970. Inequalities leading to a proof of the classical martingale-convergence theorem. In: Martingales. Vol. 190, Lecture Note in Mathematics. Berlin: Springer. pp. 9–12.
- Doob JL. 1940. Regularity properties of certain families of chance variables. Trans Amer Math Soc. 47:455–486.
- Doob JL. 1953. Stochastic processes. New York: Wiley.
- Dudley RM. 2002. Real analysis and probability. Cambridge: Cambridge University Press.
- Garling DJH. 2007. Inequalities: a journey into linear analysis. Cambridge: Cambridge University Press.
- Garsia AM. 1970. Topics in almost everywhere convergence. Chicago: Markham.
- Helms LL, Loeb PA. 1982. A nonstandard proof of the martingale convergence theorem. Rocky Mt J Math. 12:165–170.
- Isaac R. 1965. A proof of the martingale convergence theorem. Proc Amer Math Soc. 16:842–844.
- Karatzas I, Shreve SE. 1998. Brownian motion and stochastic calculus. 2nd ed. New York: Springer.
- Krickeberg K. 1965. Probability theory. Reading, MA: Addison-Wesley.
- Kruglov VM. 2001. On the Kolmogorov theorem. J Math Sci (NY). 106:2830–2834.
- Kruglov VM. 2009. On convergence of submartingales. Theory Probab Appl. 53:321–329.
- Lamb CW. 1973. A short proof of the martingale convergence theorem. Proc Amer Math Soc. 38:215–217.
- Malliavin P. 1995. Integration and probability. New York: Springer-Verlag.
- Meyer PA. 1972. Martingales and stochastic integrals I. Vol. 284, Lecture notes in mathematics. Berlin: Springer-Verlag.
- Neveu J. 1975. Discrete-parameter martingales. Amsterdam: North-Holland.
- Petersen KE. 1983. Ergodic theory. Cambridge: Cambridge University Press.
- Revuz D, Yor M. 1999. Continuous martingales and Brownian motion. 3rd ed. Berlin: Springer-Verlag.