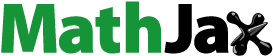
Abstract
The present study suggests an enhanced class of estimators for the population mean estimation utilizing simple random sampling (SRS). The bias, mean square errors (MSE), and minimum MSE of the suggested estimators are computed to the approximation of order one. The efficiency conditions are obtained by comparing the MSE of the proposed and existing estimators. An empirical investigation is carried out using some real and artificially generated symmetric and asymmetric populations. The empirical findings are observed to be positive, clearly demonstrating the preponderance of the suggested estimators over the existing estimators.
1 Introduction
The traditional ratio, regression, and exponential approaches have been widely utilized for population mean estimation in the recent years due to their simple structure and computational ease. With the help of various auxiliary variable-related parameters, including coefficients of variation, skewness and kurtosis, along with the population mean, the standard ratio and exponential estimators have been improved by various researchers to estimate the unknown population mean of the study variable. When applying the ratio, regression, and exponential estimation methods, the population characteristics of the auxiliary variable must also be available ahead of time. Watson (Citation1937) considered auxiliary information and proposed the conventional regression estimator of population mean of the study variable which is best linear unbiased (BLU) estimator. Cochran (Citation1940) proposed the conventional ratio estimator of population mean under SRS. Srivastava (Citation1967) utilized auxiliary information and suggested a power ratio estimator of population mean under SRS. Walsh (Citation1970) suggested a ratio type estimator for the population mean under SRS. Bahl and Tuteja (Citation1991) proposed an exponential ratio estimator of population mean under SRS. Sisodia and Dwivedi (Citation1981), Singh and Kakran (Citation1993), Upadhyaya and Singh (Citation1999), and Singh (Citation2003a) utilized known parameters of auxiliary variable and suggested some modified ratio estimators of population mean under SRS. Singh et al. (Citation2009) suggested a generalized exponential ratio type estimator of population mean which was later on enhanced by Yadav and Kadilar (Citation2013) by utilizing Searls (Citation1964) technique. Yan and Tian (Citation2010) utilized the coefficient of skewness of auxiliary variable and presented a ratio method of estimation of population mean, whereas Subramani and Kumarapandiyan (Citation2012) considered co-efficient of variation and median of an auxiliary variable and developed an estimation procedure of population mean. Jeelani et al. (Citation2013) developed a modified ratio estimators of population mean utilizing a linear combination of co-efficient of skewness and quartile deviation, while Jerajuddin and Kishun (Citation2016) suggested a modified ratio estimators for population mean utilizing size of the sample chosen from the population. Kadilar (Citation2016) suggested an improved ratio cum exponential ratio estimator of population mean. Soponviwatkul and Lawson (Citation2017) considered utilizing a coefficient of variation, correlation coefficient and a regression coefficient, and construct a new ratio estimator for estimating population mean under SRS. Ijaz and Ali (Citation2018) suggested some improved ratio estimators for estimating population mean utilizing SRS. Yadav et al. (Citation2019) adopted various auxiliary information and developed a class of population mean estimators under SRS. Yadav et al. (Citation2019) examined the efficiency of their developed estimators using primary data of production of peppermint oil obtained from the crop from Banikodar Block of Barabanki District situated in Uttar Pradesh, India.
The studies discussed in previous paragraph are either equally or less efficient than the conventional regression (BLU) estimator. In this article, we propose an enhanced class of estimators for the population mean (PM) estimation of the study variable (SV) employing the data from auxiliary variable (AV) based on SRS. The aim of this paper is to:
Propose an enhanced class of estimators for the PM estimation under SRS that competes with the existing estimators, especially regression (BLU) estimator,
Compare theoretically the efficiency of the suggested estimators with the current estimators,
Exemplify the proposed estimators using some real-life populations,
Perform a simulation study using some artificially generated symmetric and asymmetric populations.
The current paper is further designed in the succeeding sections. In Section 2, methodology and terminologies used are explained. In Section 3, some prominent estimators along with their bias, MSE, and minimum MSE expressions are discussed. In Section 4, we suggest an enhanced class of estimators for PM estimation under SRS along with the bias, MSE, and minimum MSE expressions. The efficiency conditions are obtained in Section 5. In Section 6, an empirical illustration of the efficiency of the suggested estimators is provided by using some examples based on some real populations as well as some artificially generated populations. The paper is concluded in Section 7.
2 Methodology and notations
Let a population (
,…,
be the composition of the distinguishable items with a finite size N. Choose a sample of n size based on a finite population of N length by employing simple random sampling without replacement. Let yi and xi symbolize the SV and AV for the ith (i
1, 2,…, N) unit from P. Let
and
be the sample and population mean of SV y, respectively;
and
be the sample and population mean of AV x. Let Mx and My be the median of AV and SV, respectively. Let
and
be the sample and population standard deviation of SV y. Let
and
be the sample and population standard deviation of AV x. Let C
S
and Cx = Sx/
be the population coefficient of variation of SV and AV, respectively. Let ρxy represent the population correlation coefficient between AV and SV.
The characteristics of the suggested estimators are further deduced using the notations below:
Let us consider the error terms as /
and
such that the expected values of these error terms are zero and E(e
E(e
, and E(e0e
, where
1/n.
3 Existing estimators
The current section devotes to some prominent available estimators which are discussed below along with their MSE/minimum MSE expressions.
The usual unbiased estimator is given by:
with the variance as
The usual ratio estimator was proposed by Cochran (Citation1940) which is given by:
with the bias and MSE as
The usual regression estimator was proposed by Watson (Citation1937) which is given by
with the bias and minimum MSE at the optimum value of scalar
as
Srivastava (Citation1967) suggested the power ratio estimator as follows
with the bias and minimum MSE at the optimum value of scalar
as
Walsh (Citation1970) suggested the following ratio estimator as
with the bias and minimum MSE at the optimum value of scalar
as
Sisodia and Dwivedi (Citation1981) utilized the auxiliary information and suggested the following ratio estimator
with the bias and MSE as
where
.
Bahl and Tuteja (Citation1991) proposed the exponential ratio estimator as
with the bias and MSE as
Singh and Kakran (Citation1993) used the coefficient of kurtosis and construct the following ratio estimator
with the bias and MSE as
where
.
Upadhyaya and Singh (Citation1999) utilized the transformed auxiliary information and suggested the following estimators:
with the bias and MSE as
where
and
Singh (Citation2003a) examined the following ratio estimator:
with the bias and MSE as
where
.
Singh et al. (Citation2009) considered various auxiliary information and suggested following class of exponential estimator:
with the bias and MSE as
where
.
Yadav and Kadilar (Citation2013) suggested an improved class of exponential ratio estimator:
with the bias and minimum MSE at optimum value of
as
where
and
.
Here, u and v are either real amounts or some available population parametric values of AV x.
Kadilar (Citation2016) developed an improved ratio cum exponential ratio class of estimator:
with the bias and minimum MSE at optimum value of scalar
as
Ijaz and Ali (Citation2018) suggested the following class of estimators for the estimation of population mean:
with the bias and minimum MSE at optimum value of scalar
as
Yadav et al. (Citation2019) suggested the following class of estimators for population mean under SRS:
with the bias and MSE as
where , and
4 Proposed estimators
Almost all estimators reviewed in the previous section are either less or equally efficient to the classical regression (BLU) estimator. This work aims to develop an enhanced class of estimators for the estimation of PM of SV using information on AV. Here is the proposed class of estimators:
where kj, j
1, 2 are constant to optimize the MSE, while u and v are either real amounts or some available population parametric values of AV x. Some sub-class of T are tabulated in .
Table 1 Several individuals from the proposed estimators.
By employing the notations provided in “Methodology and notations,” we can express the suggested estimator as
where
.
Here, Taylor’s series expansion is used and the error terms having power greater than 2 are neglected. This provides the following expression:
Considering expectation both the sides to the above expression, we get
Squaring and considering expectation both sides to the above expression provides
The above MSE equation can further be expressed as
where
and
.
Minimizing the MSE(T) against k1 provides the optimum value of k1 as
Using the amount of in the MSE(T), we obtain
The optimization of k1and k2 simultaneously is very typical. Therefore, putting 1 in the estimator T and minimize the MSE of T against k2 provides the optimum value of k2 as
5 Efficiency conditions
The MSE
is compared with the variance/MSE/minimum MSE of the estimators discussed in “Existing estimators” and obtained the efficiency conditions mentioned below.
Under these efficiency conditions, the proposed class of estimators represses the reviewed estimators. In practice, these efficiency conditions are verified through the empirical study performed in the next section.
6 Empirical study
This section presents an empirical study in three subsections. In Section 6.1, a numerical study is presented based on four different real populations, in Section 6.2, a simulation study is presented based on artificially generated symmetric and asymmetric populations, while in Section 6.3, discussion of empirical results is presented.
6.1 Numerical study
To improve the theoretical establishment, we have conducted an empirical investigation on four real populations. The descriptive information of these populations is tabulated in .
Table 2 Descriptive statistics of different populations.
The optimum values of k1 and k2 are computed and the results are listed in for all populations. Further, the range of k1 (for fixed values of and k2 (for fixed values of
are computed and the results are listed in and , respectively, under which the proposed class of estimators T is more efficient than the existing estimators. It is noticed from and that there is sufficient scope of choosing the scalars k1 and k2 to get better estimators than the existing estimators. From the common range of k1 and k2 and optimum values k1 and k2 for all populations, it is noticed that the scope of obtaining better estimators from the proposed class of estimators is wide even if the guessed values of the scalars k1 and k2 departs substantially from the exact optimum values k1 and k2.
Table 3 Optimum values of of the members of the proposed estimator for different populations.
Table 4 Range of k1 at the fixed value of k2 for different populations.
Table 5 Range of k2 at the fixed value of k1 for different populations.
We have also computed the bias, MSE, and PRE of the estimators and reported in . The following expression is used to calculate PRE:
where
Table 6 Bias, MSE and PRE for several estimators.
6.2 Simulation study
To extrapolate the theoretical findings as well as to strengthen the findings of numerical study, we conducted a simulation study based on hypothetically generated symmetric and asymmetric populations. In order to generated the data, following Singh and Horn (Citation1998), we used the models listed below:
where
and
are possessing the corresponding distributions. In particular, we generated the following populations.
We generated a normal population of size N
1000 using
and
.
We generated a gamma population of size N
1000 using
and
.
We have taken a sample of size 200 from the above populations. To observe the behavior of the proposed estimators, utilizing 15,000 replications, we have computed MSE and percent relative efficiency (PRE) for the varying values of correlation coefficient as 0.1, 0.5, and 0.9. The PRE is calculated with the help of following expression.
The simulation findings are reported in and for normal and gamma populations with bias, MSE, and PRE, respectively.
Table 7 Bias, MSE, and PRE of several estimators for simulated normal population.
Table 8 Bias, MSE, and PRE of several estimators for simulated gamma population.
6.3 Discussion of empirical results
After carefully observing the findings of the numerical and simulation studies, we have drawn the following observations:
From , for each population, the outcomes show that the members
of the suggested estimator T obtain the lowest MSE and highest PRE as compare to the existing estimators such as the conventional mean estimator, usual ratio and regression estimators, Srivastava (Citation1967) estimator, Walsh (Citation1970) estimator, Sisodia and Dwivedi (Citation1981) estimator, Bahl and Tuteja (Citation1991) estimator, Singh and Kakran (Citation1993) estimator, Upadhyaya and Singh (Citation1999) estimators, Singh (Citation2003a) estimator, Singh et al. (Citation2009) estimators, Yadav and Kadilar (Citation2013) estimators, Kadilar (Citation2016) estimator, Ijaz and Ali (Citation2018) estimator, and Yadav et al. (Citation2019) estimators.
From , the member T(1) is found to be best among the proposed class of estimator T in each population.
The results reported in are based on normal population which reveal that the members
of the proposed estimator T repress the reviewed estimators with minimum MSE and maximum PRE for each value of
. Moreover, as
increase, the MSE and PRE of the members of the proposed estimators also decreases and increases, respectively.
The results reported in are based on gamma population which demonstrate that the members
of the proposed estimator T repress the reviewed estimators with minimum MSE and maximum PRE for each value of
. Moreover, as
increases, the MSE and PRE of the members of the proposed estimators also increase, respectively.
From and , the member T(1) is found to be best among the proposed class of estimator T in normal and gamma populations.
7 Conclusions
This paper suggested an enhanced class of estimators for the population mean estimation utilizing the simple random sampling scheme. The bias, mean square error, and minimum mean square error of the proposed class of estimators are obtained mathematically to the approximation of first order. By comparing the minimum MSE expression of the proposed estimators with the MSE/minimum MSE of the existing estimators, the efficiency conditions are determined. A numerical illustration involving four real populations and a simulation study involving artificially generated normal and gamma populations provide support for the efficiency conditions developed in “Efficiency conditions.” The empirical findings demonstrate that the proposed estimator represses the conventional estimators in each population which are further extended with the simulation findings. Therefore, it is advised to utilize the suggested estimators for the population mean estimation in practical issues.
Further, the suggested estimators can be examined for the population mean estimation using different sampling schemes like, stratified random sampling, stratified ranked set sampling, two-phase sampling, adaptive cluster sampling, probability proportion to size sampling, for more details refer the studies of Ahmad and Shabbir (Citation2018), Ahmad et al. (Citation2021), (2022), Iftikhar et al. (Citation2022), Bhushan et al. (Citation2022a), (2022b), (2023), Rana et al. (Citation2022), Bhushan and Kumar (Citation2023a), (2023b), Qureshi and Hanif (Citation2019).
Acknowledgment
The authors are extremely grateful to the learned referees for their valuable comments and to Editor-in-Chief.
Disclosure statement
No potential conflict of interest was reported by the author(s).
References
- Alomair MA, Shahzad U. 2023. Compromised-imputation and EWMA-based memory-type mean estimators using quantile regression. Symmetry. 15:1888.
- Ahmad S, Hussain S, Aamir M, Yasmeen U, Shabbir J, Ahmad Z. 2021. Dual use of auxiliary information for estimating the finite population mean under the stratified random sampling scheme. J Math. 2021:1–12.
- Ahmad S, Hussain S, Shabbir J, Aamir M, El-Morshedy M, Ahmad Z, Alrajhi S. 2022. Improved generalized class of estimators in estimating the finite population mean using two auxiliary variables under two-stage sampling. AIMS Math. 7:10609–10624.
- Ahmad S, Shabbir J. 2018. Use of extreme values to estimate finite population mean under pps sampling scheme. J Reliab Stat Stud. 11:99–112.
- Bahl S, Tuteja RK. 1991. Ratio and product type exponential estimators. J Inform Optim Sci. 12:159–164.
- Bhushan S, Kumar A. 2023a. Enhanced estimation of population mean under two-phase sampling. Int J Math Modell Numer Optim. 13:34–48.
- Bhushan S, Kumar A. 2023b. New efficient class of estimators of population mean using two-phase sampling. Int J Math Oper Res. 24:155–172.
- Bhushan S, Kumar A, Shahab S, Lone SA, Akhtar MT. 2022a. On efficient estimation of population mean under stratified ranked set sampling. J Math. 2022:1–20.
- Bhushan S, Kumar A, Shahzad U, Al-Omari AI, Almanjahie AI. 2022b. On some improved class of estimators by using stratified ranked set sampling. Mathematics. 10:3283.
- Bhushan S, Kumar A, Singh S. 2023. Some efficient classes of estimators under stratified sampling. Commun Stat Theory Methods. 52:1767–1796.
- Cochran WG. 1940. The estimation of the yields of cereal experiments by sampling for the ratio of grain to total produce. J Agric Sci. 30:262–275.
- Iftikhar S, Khalil A, Ali A. 2022. A novel and improved logarithmic ratio-product type estimator of mean in stratified random sampling. Math Prob Eng. 2022:1–20.
- Ijaz M, Ali H. 2018. Some improved ratio estimators for estimating mean of finite population. Res Rev J Stat Math Sci. 4:18–23.
- Jeelani MI, Maqbool S, Mir SA. 2013. Modified ratio estimators of population mean using linear combination of co-efficient of skewness and quartile deviation. Int J Mod Math Sci. 6:174–183.
- Jerajuddin M, Kishun J. 2016. Modified ratio estimators for population mean using size of the sample, selected from population. Int J Sci Res Sci Eng Technol. 2:10–16.
- Kadilar C, Cingi H. 2003. Ratio estimators in stratified random sampling. Biometrical J. 45:218–225.
- Kadilar GO. 2016. A new exponential type estimator for the population mean in simple random sampling. J Mod App Stat Meth. 15:207–214.
- Qureshi MN, Hanif M. 2019. Generalized estimator for the estimation of clustered population mean in adaptive cluster sampling. Commun Stat Theory Method. 50:3262–3275.
- Rana Q, Qureshi MN, Hanif M. 2022. Generalized estimators for population mean using an auxiliary attribute in stratified two-phase sampling. J Stat Theory Appl. 21:44–57.
- Sarndal CE, Swensson B, Wretman J. 2003. Model assisted survey sampling. New York (NY): Springer-Verlag.
- Searls DT. 1964. The utilization of a known coefficient of variation in the estimation procedure. J Am Stat Assoc. 59:1225–1226.
- Singh GN. 2003a. On the improvement of product method of estimation in sample surveys. J Ind Soc Agric Stat. 56:267–275.
- Singh R, Chauhan P, Sawan N, Smarandache F. 2009. Improvement in estimating the population mean using exponential estimator in simple random sampling. Bull Stat Econ. 3:13–18.
- Singh HP, Kakran MS. 1993. A modified ratio estimator using known coefficient of kurtosis of an auxiliary character. Unpublished.
- Singh HP, Horn S. 1998. An alternative estimator for multi-character surveys. Metrika. 48:99–107.
- Singh S. 2003b. Advanced sampling theory with applications: how Michael “selected” Amy. Vol. 2. Dordrecht: Springer.
- Sisodia BVS, Dwivedi VK. 1981. A modified ratio estimator using coefficient of variation of auxiliary variable. J Ind Soc Agric Stat. 33:13–18.
- Soponviwatkul K, Lawson N. 2017. New ratio estimators for estimating population mean in simple random sampling using a coefficient of variation, correlation coefficient and a regression coefficient. Gazi Univ J Sci. 30:610–621.
- Srivastava SK. 1967. An estimator using auxiliary information. Calcutta Stat Assoc Bull. 16:121–132.
- Subramani J, Kumarapandiyan G. 2012. Estimation of population mean using co-efficient of variation and median of an auxiliary variable. Int J Prob Stat. 1:36–40.
- Upadhyaya LN, Singh HP. 1999. Use of transformed auxiliary variable in estimating the finite population mean. Biom J. 41:627–636.
- Walsh JE. 1970. Generalization of ratio estimator for population total. Sankhya A. 32:99–106.
- Watson DJ. 1937. The estimation of leaf area in field crops. J Agric Sci. 27:474–483.
- Yadav SK, Dixit MK, Dungana HN, Mishra SS. 2019. Improved estimators for estimating average yield using auxiliary variable. Int J Math Eng Manag Sci. 4:1228–1238.
- Yadav SK, Kadilar C. 2013. Efficient family of exponential estimators for the population mean. Hacettepe J Math Stat. 42:671–677.
- Yan Z, Tian B. 2010. Ratio method to the mean estimation using coefficient of skewness of auxiliary variable. In: Zhu R, Zhang Y, Liu B, Liu C, editors. Information Computing and Applications. ICICA 2010. Communications in Computer and Information Science. Vol. 106. Berlin: Springer.