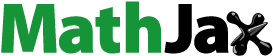
ABSTRACT
This study aims to contribute to the understanding of diversity within the context of banana farming and its implications for the management of banana Xanthomonas wilt (BXW) disease in Rwanda. We used a structured questionnaire to collect data from 690 banana farmers in eight districts, representing various agroecological zones, across Rwanda. We implemented principal component analysis (PCA) and hierarchical cluster analysis (HCA) of the data leading to the delineation of three distinct groups of banana farmers, namely: 1) Beer banana farmers (39.1%), 2) Livestock-based farmers (31.7%), and 3) Cooking banana farmers (29.1%). These farm types exhibit apparent differences in their main farming objectives and adoption of BXW management practices. Cooking banana farmers prioritize BXW prevention over control, whereas beer banana farmers exhibit the opposite trend. Livestock-based farmers show no significant difference in practices in comparison to cooking banana farmers. Beer banana farmers predominantly adopt the officially recommended complete mat uprooting (CMU) approach for disease control, while cooking banana farmers adopt the single diseased stem removal (SDSR) method. These findings provide insights for designing customized and targeted interventions to address BXW management effectively, based on farmer typology.
1. Introduction
Crop diseases are a major threat to agricultural production, food security, and farmers’ livelihood around the world. Banana Xanthomonas Wilt (BXW) is a bacterial infection that is extremely harmful and has become an increasingly severe danger to the cultivation of bananas, especially in East and Central Africa (Biruma et al., Citation2007; McCampbell et al., Citation2018). This disease has resulted in significant financial losses and poses significant obstacles to ensuring food security in the affected regions (Ocimati et al., Citation2019; Petsakos et al., Citation2023). BXW, caused by Xanthomonas campestris pv. musacearum (Xcm), is a highly concerning disease due to its rapid spread, affecting all banana cultivars, and its potential to cause complete yield losses of up to 100% at the farm level (Kabirigi et al., Citation2022; Kilwenge et al., Citation2023). Rwanda, a country heavily reliant on banana cultivation for sustenance and income generation, has experienced severe impacts from the rapid spread of BXW (Blomme et al., Citation2021; Uwamahoro et al., Citation2019). The continued expansion of BXW in East and Central Africa requires urgent attention and the development of effective management strategies that take into account the unique characteristics of the farming systems in the region.
In recent years, Rwanda has implemented a centralized strategy to combat BXW, centred around “Complete Mat Uprooting” (CMU) (Jogo et al., Citation2011; McCampbell et al., Citation2018). However, this approach has not yielded the desired results in containing the spread of the disease (Blomme et al., Citation2017). One significant factor contributing to its limited success is the top-down and “one-size-fits-all” nature of the policy, which overlooks the diversity among banana farmers and their farms in Rwanda (Costanza et al., Citation2020; Hammond et al., Citation2017). To improve the effectiveness of the approach, it is crucial to consider the unique characteristics and challenges faced by different farmers and their banana farms across the country.
This research paper aims to contribute to the understanding of diversity within the context of banana farming and its implications for the management of BXW in Rwanda. We seek to identify key variables that differentiate banana growers into distinct farm types and explore how these farm types are associated with the knowledge of and adoption of innovative BXW management and prevention practices. By comparing how various farm types know and use practices for BXW management, we aim to answer two fundamental questions. Firstly, we determine if farmers of various types have different levels of knowledge regarding BXW management. Secondly, we explore whether the knowledge of these practices is linked to the farmers’ adoption of them.
The understanding of agricultural innovation adoption and the role of farm diversity has long been a matter of concern (Hammond et al., Citation2017). There is an agreement among social scientists that one of the potential barriers to innovation adoption is the failure to take into account the heterogeneity of farmers, especially small-scale farmers (Coe et al., Citation2016; Kabirigi et al., Citation2022). Previous studies on adoption have recognized the typology approach as a valuable method for addressing the diversity and variations among farms, enabling the implementation of targeted interventions (Daxini et al., Citation2019; Köbrich et al., Citation2003). As a result, researchers have extensively investigated the adoption of agricultural innovations through the typology approach, revealing valuable insights into critical aspects of the process (Hammond et al., Citation2020). Some key findings include the absence of a universal solution (Costanza et al., Citation2020), the differing levels of responsiveness to innovations among different farm types (Bidogeza et al., Citation2009), and the necessity for tailoring innovations to suit specific production systems (Berre et al., Citation2022).
Despite significant efforts by researchers to understand farmer heterogeneity and utilize typology approaches, several gaps persist in the literature. Firstly, few studies explore farm typology’s application for managing Banana Xanthomonas Wilt (BXW) challenges or its interplay with varying BXW management strategies. Secondly, no attempts have been made to explore the diversity within the banana farming community in the specific context of Rwanda. Finally, there remains a scarcity of evidence-based studies linking the awareness of a particular technology to its actual adoption. Although typology analysis has been applied to explore the impact of innovative agricultural practices for different purposes, this paper takes the stance that farm types play a significant role in explaining the diversity of crop management behaviours.
Our research contributes to the existing literature by utilizing the typology approach to investigate BXW prevention and control methods in different farm types within Rwanda’s banana production systems. This is justified because banana production involves a diverse production system, with bananas being grown in different agroecological areas and by various categories of farmers with different socio-economic backgrounds. Our study specifically focuses on Rwanda as a case study due to the crucial role of bananas in ensuring food security, despite persistent efforts to control and prevent the BXW disease. Bananas cover a significant portion of arable land in Rwanda (23%) and are cultivated by 90% of households, making them a vital dietary staple for farmers. Yet, the crop is threatened by BXW to the level that it has become a governmental concern. The study’s findings have significant implications for sustainable agricultural development in the region, supporting the adoption of innovative practices that cater to the unique needs of different farmer typologies while mitigating the impact of BXW. Additionally, the study contributes to the scientific understanding of the dynamics between farmer heterogeneity, technology awareness, and technology adoption, bridging a crucial gap in the existing literature.
In this research, we use principal component analysis (PCA) and hierarchical cluster analysis (HCA) to conduct farm typology analysis based on 690 banana farmers in eight districts, representing various agroecological zones, across Rwanda. We further associate various farm types with diverse measurements taken in preventing and controlling BXW. The subsequent sections of this paper are organized as follows: Section 2 provides an overview of the theoretical background. Section 3 describes the methodology employed, including the specific case of managing BXW in Rwanda and the data collection and processing approach. Section 4 presents the findings, including the identified farm typologies among banana growers in Rwanda. Section 4 discusses the implications of these typologies for BXW prevention and control practices. Finally, Section 5 concludes the paper by summarizing the key findings, contributions, and future research directions.
2. Theoretical background
2.1. Farmer heterogeneity in innovation adoption
This study is primarily based on Rogers’ diffusion of innovation theory (2003), which explores how innovations, such as new agricultural practices or technologies, spread and are adopted within a population. Scholars widely agree that the success of technological advancements depends on how well they are accepted and integrated by potential users (Wigboldus et al., Citation2016). Research suggests that the adoption of these new practices is influenced by a variety of factors, encompassing diverse farms, variations in farmers’ characteristics, and the broader socioeconomic, institutional, and environmental context (Makate, Citation2019; Oyetunde Usman et al., Citation2021; Yoon et al., Citation2020). The literature emphasizes the significant role of farmers’ heterogeneity in influencing the uptake of innovative agricultural practices (Hammond et al., Citation2020; Llewellyn & Brown, Citation2020). Previous studies have highlighted the challenge of applying innovations developed for specific contexts to a broader audience, as each farming community has its unique characteristics and requirements (Costanza et al., Citation2020). Consequently, recognizing the diversity among farmers becomes crucial, and innovation strategies must be tailored accordingly to cater to the different types of farms.
In response to this recognition, the evolution of agricultural extension approaches has undergone a gradual shift from technology-oriented models in the past to more participatory and context-specific models in recent times (Schut et al., Citation2014). While the traditional approach assumes all farmers to be homogenous recipients of innovations, the current tailored approach acknowledges farmers’ heterogeneity and actively involves them in the innovation process (Minas et al., Citation2020). In this contemporary approach, farmers are considered integral partners within innovation networks, underscoring the importance of understanding the context-specific sociocultural, economic, and agroecological factors that drive successful agricultural innovation (Schut et al., Citation2014). As agricultural extension methods have evolved, it is now recognized that involving farmers in the innovation process enhances the likelihood of successful adoption and implementation (Hermans et al., Citation2017; Minas et al., Citation2020). Rather than imposing one-size-fits-all solutions, this modern approach promotes engagement with farmers to co-create context-specific solutions (Tödtling & Trippl, Citation2005). By incorporating farmers’ knowledge, preferences, and needs, agricultural innovations become more relevant, accessible, and effectively integrated into their daily practices.
Drawing on the diffusion of innovation theory and recognizing the diversity among farmers, researchers have developed a theoretical method known as the typology approach. This innovative approach aims to tackle the inherent complexity resulting from the wide variety of farmers. In the following sub-section, we will delve deeper into the typology approach, exploring how it effectively addresses the challenges within agricultural innovation systems.
2.2. Typology approach
The typology approach is a method that involves categorizing individuals into distinct groups, known as “types”, based on their shared characteristics (Alvarez et al., Citation2018). The goal of a typology is to simplify complex information and create structured frameworks that aid in understanding, analysis, and communication (Guest, Citation2012). However, scholars employing this approach should approach it thoughtfully, embracing feedback and iteration, as the process of deciding what to include and exclude within it presents several challenges (Steinley & Brusco, Citation2008). These challenges encompass establishing relevant categorization criteria, mitigating subjectivity and bias, striking a balance between complexity and simplicity, managing issues with data availability and reliability, addressing interrelationships and overlaps, accounting for evolving phenomena, and including a range of stakeholder viewpoints (Gnanadesikan et al., Citation1995; Liu et al., Citation2016).
The typology approach is a versatile framework that extends beyond agriculture and has been applied to various fields and industries. For instance, it has been used in biology (Sokal, Citation1962), sociology (Collier et al., Citation2012), geography (Popovich et al., Citation2021), bioeconomy (Ayrapetyan et al., Citation2022), and education (Mitchell & Carbone, Citation2011), to better understand complex phenomena and enable targeted interventions. Each study’s categorization is directly linked to its specific goals or unique factors being investigated. Consequently, research has identified different typology categories like physical-based (Popovich et al., Citation2021), technological-based (Ayrapetyan et al., Citation2022), and organizational-based typologies (Machado & Carvalho, Citation2008), among others.
In the farming community, this approach results in two distinct types of classifications: “farm types” when the focus is on characteristics, attributes, or features of the agricultural production system (Dalgaard et al., Citation2006), and “farmer types” when it delves into the personal traits, behaviours, and attitudes of individual farmers (Sarker et al., Citation2021). These generated types provide invaluable insights into different groups, leading to a deeper understanding of their unique dynamics and circumstances. In the agricultural production systems, the typology approach has led to the identification of different types of farms based on specific objectives or distinguishing factors. These types include size-related (Alvarez et al., Citation2018), agroecological zone-related (Pengelly & Maass, Citation2019), value chain-related (Hammond et al., Citation2017), resource endowment-related (Nabahungu & Visser, Citation2011), risk and resilience-related (Spiegel et al., Citation2021), and others.
Recognizing its immense value, the Food and Agriculture Organization (FAO) has acknowledged and endorsed the typology approach as an invaluable tool for not only documenting the diversity of agricultural systems but also in supporting the design of agriculture-related policies. Its significance extends beyond just capturing the diversity of farm households and livelihoods; it also provides key insights into how farm household types can contribute to driving the development and adoption of innovations within the agricultural sector.
By approaching this research with a focus on context-dependent diversity and employing a suitable research tool, we can shed light on the nuanced dynamics of banana farming systems and contribute to the development of effective strategies for BXW disease management.
3. Materials and methods
3.1. Household survey data
This study used data from a household survey conducted in July and August 2018 by trained enumerators. Farmer household information was collected in eight districts (Burera, Rulindo, Gatsibo, Kayonza, Gisagara, Muhanga, Karongi, and Rubavu), which were purposively selected for their representation of the major agroecological zones as well as their representation of different types of banana producing farmers in four provinces in Rwanda. The questionnaire contained close-ended questions on household information on a wide range of topics. For this paper, we considered only data related to socioeconomic characteristics, banana production system characteristics, extension services received, and adopted practices for BXW prevention and control. These variables were used to identify factors responsible for farmers’ heterogeneities and to categorize them into farm types, whereas variables such as BXW prevention and control practices were used to understand the behaviours of farm types regarding decisions for preventing and controlling BXW.
The selection of districts was conducted based on expert knowledge (mainly through multiple consultations with the banana program leader at the RAB) and raw data from a countrywide rapid assessment of BXW status conducted by RAB between 2017 and 2018. District coverage of main agroecological zones is summarized in , whereas the area covered by banana crops in respective districts is summarized in . In terms of the total area covered by banana, Gatsibo, Kayonza have more land allocated to banana production. However, Muhanga and Karongi have a higher proportion of land allocated to bananas compared to the total cultivated area (). Following a stratified sampling approach, based on expert input from district and sector agronomists, sectors and cells were selected as distinguished by the BXW incidence level. The sampling team aimed the selection of villages with a minimum distance of 5 km between any two villages. The selection of farmers considered the gender of household heads when selecting five farmers in each village, two of which were female. In total, 690 farmers were interviewed in 138 villages.
Table 1. Main characteristics of studied districts (NISR, Citation2017.).
3.2. Respondents
The median age of household heads was 46 years, and the majority of respondents had a primary level of education (67.5%). The majority of respondents were married (83.8%), and the median size of households was five members. The median total land size owned by a household was 0.3 ha and the average tropical livestock unit was 0.94 ± 0.91. Only 30.1% of the households had off-farm income and the average income from banana was 49,790 Rwf (1 Rwf = 0.00097 Euro) per household per year. However, around 50% of them had zero income suggesting that their banana production is of subsistence. A total of 43.8% of farms grow banana intercropped with other food crops, mainly beans, maize, sweet potatoes, cassava, and others. The majority of interviewed household farms (60%) had experienced BXW.
3.3. Developing farm types and statistical analysis
We combined PCA and HCA to develop farm types. Our decision to use PCA-HCA in the analysis was influenced by the nature of the dataset. Furthermore, PCA-HCA was chosen for its straightforward interpretability, providing a clear method for data analysis where dimension-reduced data points and their relationships are easily visualized and interpreted. The decision enhances result comparability within our research community, where PCA-HCA is commonly used for similar datasets (Alvarez et al., Citation2018; Bidogeza et al., Citation2009). We used exploratory PCA was used to identify factors responsible for banana farmers’ heterogeneities and to identify components for categorizing farmers into clusters (Barnes et al., Citation2011; Kourti, Citation2009). The selection of variables, which guided the questionnaire development and data collection, was primarily based on the literature review and expert judgement (Bidogeza et al., Citation2009). With this approach, around 60 variables were identified. The identified variables were subjected to three-step cleaning to identify those that contributed most to the variance of the dataset. The first step was to identify highly correlated variables; once found, one was removed, suggesting that they indicated the same thing (Alvarez et al., Citation2014). The second step was to identify possible outliers in the dataset by running boxplots and histograms. The observed outlier was checked to determine whether it was an outstanding value or a typing error and then dealt with accordingly. In this case, a confirmed outstanding value was kept whereas a typing error was corrected. The third step was to identify variables possibly measuring the same thing by observing if they had the same sign-in components. We ran PCA and observed which of the two correlated variables contributed less in the five first components. The screening of variables was systematic, that is, conducted by removing one variable at a time and then running PCA again to observe changes. A total of 12 variables were identified as contributing most to the heterogeneity of banana farmers.
The 12 identified variables were subjected to PCA, which is considered appropriate for our retained quantitative variables. The Kaiser rule was applied to identify the principal components for further examination in cluster analysis, with retention of components with eigenvalues (λ) > 1 (Jackson, Citation1993). Using this threshold, we retained five such components that explained 63.3% of the variance (). Before clustering, we determine and visualize the optimal number of clusters using elbow and silhouette methods (). The five components retained were subjected to HCA. This generated a dendrogram () of the sequence, in which farm households were merged. The dendrogram provided a default cutting line but also allowed us to envision an alternative cutting line resulting in a different number of clusters. By using the default cutting line we generated three distinct clusters visualized in corresponding to the identified optimal number of clusters. Clusters 1 and 2 are distinct whereas cluster 3 though distinct in particular elements, shares some characteristics with clusters 1 and 2 ().
Figure 2. PCA scree plot (A), the optimal number of clusters (B) cluster dendrogram (C), and clustering results on a factor map (D).

We performed all statistical analyses using statistical packages R, version 4.0.3 (Antoch, Citation2008). Descriptive statistics including mean, standard deviation, frequency, and percentage were used to describe our respondents and developed farm types. The analysis of variance, together with the mean separation, was performed to further compare farm types by selected variables. We applied regression analysis to infer the relationship between our outcome variable (knowing vs. not knowing and used vs. did not use BXW management innovation) and our independent variable, which was farm types. Because our outcome variables were recorded as binary (dichotomously coded as 0 or 1), we applied binary logistic regression analysis (Sperandei, Citation2014). This type of regression is the statistical method most used to predict the probability of occurrence of a binary event by utilizing a logit function (Rutebuka et al., Citation2019).
The formula for binary logistic regression, as specified by (Agresti, Citation1996), is as follows:
where the subscript i is the ith observation in the sample, andis the probability of an event occurring for an observed set of variables Xi – in our case, the probability that the farmer was visited or trained, whereas
is the probability that the visit or the training did not occur. In addition,
is the intercept term (It is the odds ratio when all the categorical variables are zero), and
,
, … ,
are the coefficients of independent variables X1, X2, … , Xk.
In our logistic regression model, (), beer-banana farmers were designated as the reference group. The reference group, or reference category, consists of those individuals presenting the reference level of each variable (Sperandei, Citation2014). The reference group stands as a category of comparison for the other categories. In the other words, we interpreted the output by looking at the coefficients of the other groups, keeping in mind that they were obtained by comparing a particular group to the reference group.
Table 2. Correlation matrix between principal components and variables used.
Table 3. Cluster (farm types) descriptions and naming.
Table 4. Log odds and standard error (in parentheses) for the binary logistic regression model for adoption of BXW prevention and control innovations by farm types.
4. Results
4.1. PCA result
In the PCA analysis, 62 variables were considered, and 12 of them were found to be significant in explaining the diversity among banana growers (as shown in ). These variables included characteristics related to both the farms and the respondents, such as the education level of the household head, the type of banana grown, income from banana crops, livestock ownership, access to extension services, and household nutritional diversity. Using these 12 variables, the PCA produced a total of 12 components. However, only five components with eigenvalues greater than 1 were retained for further analysis. These five components accounted for a combined variance of 63.3% in the dataset. The first five components individually explained 19.5%, 13%, 11.7%, 9.8%, and 9.3% of the dataset’s variance, respectively. To understand the relationship between the principal components and the 12 variables used, a correlation matrix was generated and is summarized in . The loadings in the table indicate how much each variable contributes to the five retained components.
4.2. Cluster analysis result
Table presents an overview of the different clusters based on variables and their associations with each cluster. To name the clusters meaningfully, we examined the V.test values, an indicator of whether the mean of the cluster is lower or greater than the overall mean (Schmidt et al., Citation2015), and the mean values for each category.
Cluster 1, representing 29.1% of the farm households, stands out from the others by having a higher proportion of beer bananas sold, more land allocated to beer bananas, and increased consumption of beer bananas. This indicates that farmers in this cluster specialize in producing and dealing with beer bananas more than the other clusters. Consequently, we named this group “beer-banana farmers”. The in Appendix shows that the beer-banana farmers generally had lower levels of education, less diverse nutrition, lower income from bananas, and limited access to extension services.
Cluster 2, comprising 31.7% of the farm households, is distinguished by having a higher number of tropical livestock units (TLUs), higher education levels, and greater nutritional diversity. Based on these characteristics, we referred to this cluster as “livestock-based banana farmers”. The livestock-based banana farmers had higher education levels, larger households, and more diverse nutrition, but they earned relatively lower income from bananas. The Table 7 in Appendix shows that this cluster also had a substantial number of sheep and better access to extension services, including more frequent visits from extension agents (See in Appendix).
Cluster 3, representing 39.1% of the farm households, stands apart due to its significant allocation of land to cooking bananas, higher proportion of cooking bananas sold, and increased consumption of cooking bananas. This indicates that farmers in this cluster specialize in the production of cooking bananas. Therefore, we named this group “cooking-banana farmers”. Cooking-banana farmers had higher nutritional diversity, the highest banana income, and the largest number of goats. Additionally, they had significant access to extension services, with regular trainings, frequent visits by extension agents, and active interactions with fellow farmers (See in Appendix).
4.3. Farm types adoption behaviors
shows how different farm types adopt BXW prevention and control practices, categorized into two groups: practices known to the farmers and practices used by the farmers. Positive coefficients indicate that a particular practice is more likely to be known or used by a specific group compared to the reference group, which is the beer-banana farmers. Conversely, negative coefficients suggest that the practice is less likely to be known or used by the group compared to beer-banana farmers.
Cooking-banana farmers showed an increased likelihood of knowing and using male bud removal and tool disinfection as BXW prevention practices. While they were aware of the removal of roaming animals, they were less likely to practice it. Cooking-banana farmers were more inclined to use the disinfection of tools compared to other farmer groups. In terms of BXW control practices, cooking-banana farmers were more likely to practice single diseased stem removal.
Livestock-based farmers did not significantly differ from cooking-banana farmers in their likelihood of knowing and using most prevention practices. However, they were more likely to know and practice the removal of roaming animals. Regarding BXW control methods, there was no particular practice of choice for livestock-based farmers. Instead, they were more likely to use other BXW control methods, such as methods different from complete mat uprooting and single diseased-stem removal, compared to cooking-banana farmers.
In contrast to cooking-banana farmers, beer-banana farmers had a significantly decreased likelihood of knowing and practicing BXW prevention practices. The positive coefficients for other farmer groups indicate a statistically significant difference (p < 0.05). Beer-banana farmers were also more likely to do nothing to prevent the spread of BXW, as indicated by the positive coefficients from cooking-banana farmers. Regarding BXW control, beer-banana farmers were more likely to practice complete mat uprooting, as indicated by the negative coefficients compared to cooking-banana farmers.
5. Discussion
Previous efforts to combat Banana Xanthomonas Wilt (BXW) in Rwandan did not adequately consider the diverse range of farmers, which led to difficulties in adoption. This paper supports the view that the diversity of crop management behaviours can be better explained by considering different farm types (Olivares & Hernández, Citation2020). This aspect is especially important when addressing the complex BXW disease that affects banana production systems. Unlike generic and one-size-fits-all methods, the farm typology analysis acknowledges the distinctiveness of each farm type, allowing for targeted interventions tailored to the specific challenges faced by each group (Köbrich et al., Citation2003). Our findings highlight, consistent with previous research (Kansiime et al., Citation2018; Nabahungu & Visser, Citation2011; Tittonell et al., Citation2005), the remarkable diversity among banana farmers in Rwanda. Our results indicate that different farm types may have varying priorities, objectives, and constraints. Beer banana farmers appear to give priority to growing types of banana that are primarily used in the production of local alcoholic beverages. In contrast, cooking banana farmers specialize in the production of bananas specifically used for cooking purposes. On the other hand, livestock-based farmers are more inclined to combine banana cultivation with livestock rearing.
5.1. Farm types exhibit distinct approaches to BXW prevention and control
The results of this study reveal that the three farm types exhibit distinct approaches to BXW prevention and control. Beer banana farmers exhibit a notable inclination towards disease control rather than prevention, primarily relying on the officially recommended complete mat uprooting (CMU) method to manage BXW. This finding raises the question of why economically disadvantaged beer banana farmers, with limited access to extension services, opt for the labour-intensive CMU over the less drastic single diseased stem removal (SDSR) for disease control. Drawing from our findings, it is possible to hypothesize that these farmers might place cultural importance above financial profit in their banana cultivation, which could result in neglecting preventive actions against the spread of BXW and depending on drastic control measures.
Cooking banana farmers prioritize prevention, indicating their proactive efforts to reduce BXW introduction. In contrast to the situation with beer banana farmers, we posit that cooking banana farmers are more committed to implementing BXW preventive measures due to the substantial economic value they associate with banana production. Cooking banana farmers tend to adopt the SDSR method, which allows them to manage BXW without replanting (Blomme et al., Citation2019). Research emphasizes that the effectiveness of the SDSR technique in disease control relies on its integration with preventive cultural practices like debudding and disinfecting tools (Ntamwira et al., Citation2019). For cooking banana farmers who prioritize prevention, SDSR stands as the suitable choice.
Livestock-based farmers show limited interest in BXW prevention, considering bananas as an alternative livelihood, probably not the primary one. Livestock-based farmers may benefit from integrated management practices that consider both banana cultivation and livestock rearing. The combination of bananas and livestock can create synergies, where manure from livestock can be used as organic fertilizer for banana crops, and banana by-products may serve as feed for livestock (Paul et al., Citation2022).
5.2. Different farm types possess different knowledge regarding BXW management practices
The results of this study affirm the assumption that different farm types possess varying levels of knowledge regarding Banana Xanthomonas Wilt (BXW) management practices. The variation could be attributed to the varying accessibility to extension services among different types of farms, leading to distinct levels of exposure to BXW management information. This aligns with the insight from Zamasiya et al. (Citation2017) study, which highlights the pivotal role of extension services in enhancing farmers’ access to vital agricultural knowledge and innovative practices. In this study (refer to in the Appendix), we demonstrate that cooking banana farmers exhibit better access to extension services compared to other farmers. This observation can likely be attributed to the fact that cooking banana farmers primarily concentrate on banana cultivation as their mainstay livelihood activity.
Based on the results, we accept the hypothesis that knowing a particular practice is strongly associated with a high probability of implementing it. This result emphasizes the fundamental role of knowledge in driving behaviour change and adoption of best practices (Kaiser et al., Citation2022). This relationship between knowledge and practice is often seen in various fields, especially in agriculture and public health (Moick et al., Citation2021; Thomas et al., Citation2020). It is known as the knowledge-to-action gap, and closing this gap is essential to achieving positive outcomes and desired results (Moick et al., Citation2021). For agricultural extension services, policymakers, and development organizations, this finding underscores the importance of effective knowledge transfer strategies. Simply providing information or training sessions might not be enough. Instead, efforts should focus on ensuring that farmers truly understand the rationale behind the recommended practices and how to implement them effectively.
5.3. Policy implications
The identification of distinct farm types and their differing approaches to BXW management has significant implications for designing targeted interventions. Our results suggests that in order to successfully combat BXW and promote sustainable banana production, it is crucial to recognize and cater to the diverse knowledge requirements within the farming community. Firstly, tailored training programmes must be developed to cater to the specific needs and knowledge gaps of each type, ensuring relevant and effective information dissemination. Secondly, resource allocation should prioritize types with lower knowledge levels to enhance overall BXW management education. Thirdly, diverse and adaptable knowledge transfer strategies must be employed to account for learning preferences and socio-economic factors.
The identified farm types highlight the main objectives and values of banana growers in Rwanda, which should be taken into account when implementing innovation strategies. During the scaling process, it’s crucial to approach different farm types differently to achieve the desired outcomes. We noticed that cooking-banana farmers prioritize BXW prevention over control, making them more receptive to preventive measures. On the other hand, beer-banana farmers are not as concerned about BXW prevention, and their unique needs require special attention. The choice of banana types by farmers is influenced by factors such as food security, dietary preferences, market availability, and cultural practices. For instance, beer bananas hold significant social value in Rwandan smallholder farming systems, often used in rituals and ceremonies. This cultural aspect should be considered in banana production policy development. To promote successful adoption of innovations, integrating livestock into banana production would incentivize livestock-based farmers. Moreover, introducing new, potentially BXW-resistant banana varieties should account for the preferences and practices of both beer and cooking banana growers. The idea of using farm types for tailored intervention and scaling strategic planning has been discussed in previous research.
summarizes the current practices for BXW prevention and control, as well as the extension mechanisms and capacities that need development to effectively manage BXW in Rwanda. We propose three main extension approaches: government advisory services through extension agents, farmer-to-farmer interactions, and farmer promoters. The farmer promoter approach, which is a structured government initiative based on FFS (Farmer Field School) and FPs (Farmer Promoters), is expected to be an essential tool for designing and scaling banana-farming-related innovations to achieve higher adoption rates and greater development impact.
Table 5. Alternatives to one-size-fits-all policies for BXW management in Rwanda.
5.4. Limitations and future study
While the insights derived from this research provide valuable insights for analogous production systems, especially within Latin America (Olivares et al., Citation2022), it’s important to recognize that the contextual diversity within the study poses challenges to generalization. It’s imperative to acknowledge that the conclusions drawn within this study are tightly linked to the specific circumstances in which the research was carried out. As such, they may not seamlessly translate to different regions or demographic groups without a thoughtful consideration of the indigenous factors that influence the management of BXW. In this study, we were unable to identify a universal typology that could be readily generalized. Therefore, we propose further investigation to explore avenues that could potentially offer such a versatile framework.
Furthermore, while the application of principal component analysis (PCA) for cluster analysis successfully identified distinct farm types based on the retained 5five components, it is important to note that these components collectively account for only 60% of the variation in the dataset. The remaining 40% of unexplained variation may encompass additional factors influencing farm dynamics that were not captured by the selected components. Consequently, the interpretation of farm types should be considered within the context of this unaccounted variability. Also, there remains a need for further research to uncover other potential underlying factors. Researchers should delve into the influence of factors such as geographical location, language barriers, cultural norms, and access to technology on the level of BXW management knowledge across various farm types. Additionally, investigating the knowledge networks between different farm types could yield valuable insights into additional strategies for effectively disseminating information and fostering knowledge sharing within farming communities.
6. Conclusions
The research findings provide valuable insights into the relationship between the heterogeneity of banana farmers in Rwanda and their adoption of BXW management practices. By applying the PCA and HCA methods, three distinct types of banana growers were identified: beer-banana farmers, livestock-based farmers, and cooking-banana farmers.
These distinct clusters are distinguishable based on household socioeconomic conditions, banana production systems, and access to extension services. Importantly, the research highlights the significance of tailoring interventions for effective BXW management to suit the preferences and practices of each type of farmer. Cooking-banana farmers show higher concern for BXW prevention and are more likely to adopt specific techniques, while beer-banana farmers are less inclined to take preventive action. Understanding the link between knowing a practice and practicing it underscores the need for targeted interventions to increase the adoption rates of BXW prevention measures among diverse farming communities.
This study emphasizes the importance of anchoring plant disease control strategies on the understanding of farmer diversity. By recognizing the heterogeneity among banana farmers and accounting for their distinct characteristics, preferences, and practices, interventions can be designed to align with the local context and promote inclusivity. Such tailored approaches increase the likelihood of successful BXW management, foster behaviour change, enhance resilience, and create more sustainable disease control strategies in the agricultural system.
Acknowledgments
This research was done under the framework of Ph.D. studies through the Leibniz Institute of Agricultural Development in Transition Economies (IAMO) in Germany, supported by the project “Citizen science and ICT for advancing the prevention and control of Banana Xanthomonas Wilt”, which is funded by Deutsche Gesellschaft für Internationale Zusammenarbeit (GIZ) on behalf of the German Federal Ministry for Economic Cooperation and Development (BMZ), Grant #81260865. This research also builds on the work that was supported by the Belgian Directorate General for Development Cooperation and Humanitarian Aid (DGD) through the Consortium for Improving Agricultural Livelihoods in Central Africa (CIALCA – www.cialca.org). The research forms an integral part of the CGIAR Research Program on Roots, Tubers, and Bananas (RTB), which is supported by CGIAR Fund Donors (http://www.cgiar.org/about-us/our-funders/).
Disclosure statement
No potential conflict of interest was reported by the author(s).
Additional information
Funding
References
- Agresti, A. (1996). An introduction to categorical data analysis (Wiley series in probability and statistics: Applied probability and statistics). Wiley and Sons, Inc.
- Alvarez, S., Paas, W., Descheemaeker, K., Tittonell, P. A., & Groot, J. C. (2014). Typology construction, a way of dealing with farm diversity: General guidelines for humidtropics. Wageningen University.
- Alvarez, S., Timler, C. J., Michalscheck, M., Paas, W., Descheemaeker, K., Tittonell, P. … Groot, J. C. (2018). Capturing farm diversity with hypothesis-based typologies: An innovative methodological framework for farming system typology development. PloS One, 13(5), e0194757.
- Antoch, J. (2008). Environment for statistical computing. Computer Science Review, 2(2), 113–26.
- Ayrapetyan, D., Befort, N., & Hermans, F. (2022). The role of sustainability in the emergence and evolution of bioeconomy clusters: An application of a multiscalar framework. Journal of Cleaner Production, 376, 134306.
- Barnes, A., Willock, J., Toma, L., & Hall, C. (2011). Utilising a farmer typology to understand farmer behaviour towards water quality management: Nitrate vulnerable zones in Scotland. Journal of Environmental Planning and Management, 54(4), 477–494.
- Berre, D., Adam, M., Koffi, C. K., Vigne, M., & Gautier, D. (2022). Tailoring management practices to the structure of smallholder households in Sudano-Sahelian Burkina Faso: Evidence from current practices. Agricultural Systems, 198, 103369. https://doi.org/10.1016/j.agsy.2022.103369
- Bidogeza, J., Berentsen, P., De Graaff, J., & Lansink, A. O. (2009). A typology of farm households for the Umutara Province in Rwanda. Food Security, 1(3), 321–335.
- Biruma, M., Pillay, M., Tripathi, L., Blomme, G., Abele, S., Mwangi, M. , and Nyine, M. (2007). Banana Xanthomonas wilt: A review of the disease, management strategies and future research directions. African Journal of Biotechnology, 6(8), 953–962. http://www.academicjournals.org/AJB
- Blomme, G., Dusingizimana, P., Ntamwira, J., Kearsley, E., Gaidashova, S., Rietveld, A. … Ocimati, W. (2021). Comparing effectiveness, cost-and time-efficiency of control options for Xanthomonas wilt of banana under Rwandan agro-ecological conditions. European Journal of Plant Pathology, 160(2), 487–501.
- Blomme, G., Ocimati, W., Sivirihauma, C., Lusenge, V., Bumba, M., & Ntamwira, J. (2019). Controlling Xanthomonas wilt of banana: Influence of collective application, frequency of application, and social factors on the effectiveness of the Single Diseased Stem Removal technique in eastern democratic Republic of Congo. Crop Protection, 118, 79–88.
- Blomme, G., Ocimati, W., Sivirihauma, C., Vutseme, L., Mariamu, B., Kamira, M. … Ntamwira, J. (2017). A control package revolving around the removal of single diseased banana stems is effective for the restoration of Xanthomonas wilt infected fields. European Journal of Plant Pathology, 149(2), 385–400.
- Coe, R., Njoloma, J., & Sinclair, F. (2016). Loading the dice in favour of the farmer: Reducing the risk of adopting agronomic innovations. Experimental Agriculture, 55, 1–17.
- Collier, D., LaPorte, J., & Seawright, J. (2012). Putting typologies to work: Concept formation, measurement, and analytic rigor. Political Research Quarterly, 65(1), 217–232.
- Costanza, J. K., Watling, J., Sutherland, R., Belyea, C., Dilkina, B., Cayton, H. … Haddad, N. M. (2020). Preserving connectivity under climate and land-use change: No one-size-fits-all approach for focal species in similar habitats. Biological Conservation, 248, 108678. https://doi.org/10.1016/j.biocon.2020.108678
- Dalgaard, R., Halberg, N., Kristensen, I. S., & Larsen, I. (2006). Modelling representative and coherent Danish farm types based on farm accountancy data for use in environmental assessments. Agriculture, Ecosystems & Environment, 117(4), 223–237.
- Daxini, A., Ryan, M., O’Donoghue, C., Barnes, A. P., & Buckley, C. (2019). Using a typology to understand farmers’ intentions towards following a nutrient management plan. Resources, Conservation and Recycling, 146, 280–290.
- Gnanadesikan, R., Kettenring, J. R., & Tsao, S. L. (1995). Weighting and selection of variables for cluster analysis. Journal of Classification, 12(1), 113–136. https://doi.org/10.1007/BF01202271
- Guest, G. (2012). Describing mixed methods research: An alternative to typologies. Journal of Mixed Methods Research, 7(2), 141–151. https://doi.org/10.1177/1558689812461179
- Hammond, J., Rosenblum, N., Breseman, D., Gorman, L., Manners, R., van Wijk, M. T. … Schut, M. (2020). Towards actionable farm typologies: Scaling adoption of agricultural inputs in Rwanda. Agricultural Systems, 183, 102857. https://doi.org/10.1016/j.agsy.2020.102857
- Hammond, J., van Wijk, M. T., Smajgl, A., Ward, J., Pagella, T., Xu, J. , and Harrison, R. D. (2017). Farm types and farmer motivations to adapt: Implications for design of sustainable agricultural interventions in the rubber plantations of South West China. Agricultural Systems, 154, 1–12. https://doi.org/10.1016/j.agsy.2017.02.009
- Hermans, F., Sartas, M., Van Schagen, B., van Asten, P., Schut, M., & van Rijnsoever, F. (2017). Social network analysis of multi-stakeholder platforms in agricultural research for development: Opportunities and constraints for innovation and scaling. PloS One, 12(2), e0169634.
- Jackson, D. A. (1993). Stopping rules in principal components analysis: A comparison of heuristical and statistical approaches. Ecology, 74(8), 2204–2214.
- Jogo, W., Karamura, E., Kubiriba, J., Tinzaara, W., Rietveld, A., Onyango, M., & Odongo, M. (2011). Farmers’ awareness and application of banana Xanthomonas wilt control options: The case of Uganda and Kenya. Journal of Development and Agricultural Economics, 3(11), 561–571. http://www.academicjournals.org/JDAE
- Kabirigi, M., Abbasiharofteh, M., Sun, Z., & Hermans, F. (2022). The importance of proximity dimensions in agricultural knowledge and innovation systems: The case of banana disease management in Rwanda. Agricultural Systems, 202, 103465. https://doi.org/10.1016/j.agsy.2022.103465
- Kabirigi, M., Sekabira, H., Sun, Z., & Hermans, F. (2022). The use of mobile phones and the heterogeneity of banana farmers in Rwanda. Environment Development and Sustainability, 25, 1–21.
- Kaiser, T., Lusardi, A., Menkhoff, L., & Urban, C. (2022). Financial education affects financial knowledge and downstream behaviors. Journal of Financial Economics, 145(2), 255–272.
- Kansiime, M. K., van Asten, P., & Sneyers, K. (2018). Farm diversity and resource use efficiency: Targeting agricultural policy interventions in East Africa farming systems. NJAS-Wageningen Journal of Life Sciences, 85, 32–41. https://doi.org/10.1016/j.njas.2017.12.001
- Kilwenge, R., Adewopo, J., Manners, R., Mwizerwa, C., Kabirigi, M., Gaidashova, S., & Schut, M. (2023). Climate-related risk modeling of Banana Xanthomonas Wilt (BXW) disease incidence within cropland area of Rwanda. Plant Disease, 107, 2017–2026.
- Köbrich, C., Rehman, T., & Khan, M. (2003). Typification of farming systems for constructing representative farm models: Two illustrations of the application of multi-variate analyses in Chile and Pakistan. Agricultural Systems, 76(1), 141–157.
- Kourti, T. (2009). Multivariate statistical process control and process control, using latent variables. In Comprehensive chemometrics (pp 21–54). https://doi.org/10.1016/B978-044452701-1.00013-2
- Liu, Z., Bessa, M. A., & Liu, W. K. (2016). Self-consistent clustering analysis: An efficient multi-scale scheme for inelastic heterogeneous materials. Computer Methods in Applied Mechanics and Engineering, 306, 319–341. https://doi.org/10.1016/j.cma.2016.04.004
- Llewellyn, R. S., & Brown, B. (2020). Predicting adoption of innovations by farmers: What is different in smallholder agriculture? Applied Economic Perspectives and Policy, 42(1), 100–112.
- Machado, D. D. P. N., & Carvalho, C. E. (2008). Cultural typologies and organizational environment: A conceptual analysis. Latin American Business Review, 9(1), 1–32.
- Makate, C. (2019). Effective scaling of climate smart agriculture innovations in African smallholder agriculture: A review of approaches, policy and institutional strategy needs. Environmental Science & Policy, 96, 37–51.
- McCampbell, M., Schut, M., Van den Bergh, I., van Schagen, B., Vanlauwe, B., Blomme, G. … Leeuwis, C. (2018). Xanthomonas Wilt of banana (BXW) in Central Africa: Opportunities, challenges, and pathways for citizen science and ICT-based control and prevention strategies. NJAS-Wageningen Journal of Life Sciences, 86-87, 89–100.
- Minas, A. M., Mander, S., & McLachlan, C. (2020). How can we engage farmers in bioenergy development? Building a social innovation strategy for rice straw bioenergy in the Philippines and Vietnam. Energy Research & Social Science, 70, 101717. https://doi.org/10.1016/j.erss.2020.101717
- Mitchell, I., & Carbone, A. (2011). A typology of task characteristics and their effects on student engagement. International Journal of Educational Research, 50(5), 257–270. https://doi.org/10.1016/j.ijer.2011.05.001
- Moick, S., Hiesmayr, M., Mouhieddine, M., Kiss, N., Bauer, P., Sulz, I. … Simon, J. (2021). Reducing the knowledge to action gap in hospital nutrition care – developing and implementing nutritionDay 2.0. Clinical Nutrition, 40(3), 936–945. https://doi.org/10.1016/j.clnu.2020.06.021
- Nabahungu, N. L., & Visser, S. (2011). Contribution of wetland agriculture to farmers’ livelihood in Rwanda. Ecological Economics, 71, 4–12.
- NISR. (2017). Seasonal agricultural survey report, 2017. NISR, Season A.
- Ntamwira, J., Blomme, G., Bahati, L., & Ocimati, W. (2019). Effect of timing of diseased plant cutting, altitude and banana cultivar on efficacy of singly removing xanthomonas wilt infected banana plants. European Journal of Plant Pathology, 154(2), 477–489. https://doi.org/10.1007/s10658-019-01671-9
- Ocimati, W., Bouwmeester, H., Groot, J. C., Tittonell, P., Brown, D., Blomme, G., & Ganapathi, T. R. (2019). The risk posed by xanthomonas wilt disease of banana: Mapping of disease hotspots, fronts and vulnerable landscapes. PloS One, 14(4), e0213691.
- Olivares, B. O., Calero, J., Rey, J. C., Lobo, D., Landa, B. B., & Gómez, J. A. (2022). Correlation of banana productivity levels and soil morphological properties using regularized optimal scaling regression. Catena, 208, 105718.
- Olivares, B. O., & Hernández, R. (2020). Application of multivariate techniques in the agricultural lands aptitude in carabobo, venezuela. Tropical and Subtropical Agroecosystems, 23(2), 1–12. http://dx.doi.org/10.56369/tsaes.3233
- Oyetunde Usman, Z., Olagunju, K. O., & Ogunpaimo, O. R. (2021). Determinants of adoption of multiple sustainable agricultural practices among smallholder farmers in Nigeria. International Soil & Water Conservation Research, 9(2), 241–248. https://doi.org/10.1016/j.iswcr.2020.10.007
- Paul, B. K., Epper, C. A., Tschopp, D. J., Long, C. T. M., Tungani, V., Burra, D. … Douxchamps, S. (2022). Crop-livestock integration provides opportunities to mitigate environmental trade-offs in transitioning smallholder agricultural systems of the Greater Mekong Subregion. Agricultural Systems, 195, 103285. https://doi.org/10.1016/j.agsy.2021.103285
- Pengelly, B. C., & Maass, B. L. (2019). Tropical and subtropical forage germplasm conservation and science on their deathbed! 2. Genebanks, FAO and donors must take urgent steps to overcome the crisis. Outlook on Agriculture, 48(3), 210–219.
- Petsakos, A., Kozicka, M., Blomme, G., Nakakawa, J., Ocimati, W., & Gotor, E. (2023). The potential impact of banana xanthomonas wilt on food systems in Africa: Modeling scenarios of policy response and disease control measures. Frontiers in Sustainable Food Systems, 7.
- Popovich, N., Spurlock, C. A., Needell, Z., Jin, L., Wenzel, T., Sheppard, C., & Asudegi, M. (2021). A methodology to develop a geospatial transportation typology. Journal of Transport Geography, 93, 103061. https://doi.org/10.1016/j.jtrangeo.2021.103061
- Rutebuka, J., Kagabo, D. M., & Verdoodt, A. (2019). Farmers’ diagnosis of current soil erosion status and control within two contrasting agro-ecological zones of Rwanda. Agriculture, Ecosystems & Environment, 278, 81–95.
- Sarker, M. R., Galdos, M. V., Challinor, A. J., & Hossain, A. (2021). A farming system typology for the adoption of new technology in Bangladesh. Food and Energy Security, 10(3), e287.
- Schmidt, J. I., Aanesen, M., Klokov, K. B., Khrutschev, S., & Hausner, V. H. (2015). Demographic and economic disparities among Arctic regions. Polar Geography, 38(4), 251–270.
- Schut, M., Rodenburg, J., Klerkx, L., van Ast, A., & Bastiaans, L. (2014). Systems approaches to innovation in crop protection. A systematic literature review. Crop Protection, 56, 98–108.
- Sokal, R. R. (1962). Typology and empiricism in taxonomy. Journal of Theoretical Biology, 3(2), 230–267. https://doi.org/10.1016/S0022-5193(62)80016-2
- Sperandei, S. (2014). Understanding logistic regression analysis. Biochemia medica: Biochemia medica, 24(1), 12–18.
- Spiegel, A., Slijper, T., de Mey, Y., Meuwissen, M. P. M., Poortvliet, P. M., Rommel, J. … Feindt, P. H. (2021). Resilience capacities as perceived by European farmers. Agricultural Systems, 193, 103224. https://doi.org/10.1016/j.agsy.2021.103224
- Steinley, D., & Brusco, M. J. (2008). Selection of variables in cluster analysis: An empirical comparison of eight procedures. Psychometrika, 73(1), 125–144. https://doi.org/10.1007/s11336-007-9019-y
- Thomas, E., Riley, M., & Spees, J. (2020). Knowledge flows: Farmers’ social relations and knowledge sharing practices in ‘catchment sensitive farming’. Land Use Policy, 90, 104254. https://doi.org/10.1016/j.landusepol.2019.104254
- Tittonell, P., Vanlauwe, B., Leffelaar, P., Shepherd, K. D., & Giller, K. E. (2005). Exploring diversity in soil fertility management of smallholder farms in western Kenya: II. Within-farm variability in resource allocation, nutrient flows and soil fertility status. Agriculture, Ecosystems & Environment, 110(3–4), 166–184.
- Tödtling, F., & Trippl, M. (2005). One size fits all?: Towards a differentiated regional innovation policy approach. Research Policy, 34(8), 1203–1219.
- Uwamahoro, F., Berlin, A., Bylund, H., Bucagu, C., & Yuen, J. (2019). Management strategies for banana xanthomonas wilt in Rwanda include mixing indigenous and improved cultivars. Agronomy for Sustainable Development, 39(2), 22. https://doi.org/10.1007/s13593-019-0569-z
- Wigboldus, S., Klerkx, L., Leeuwis, C., Schut, M., Muilerman, S., & Jochemsen, H. (2016). Systemic perspectives on scaling agricultural innovations. A review. Agronomy for Sustainable Development, 36(3), 46.
- Yoon, C., Lim, D., & Park, C. (2020). Factors affecting adoption of smart farms: The case of Korea. Computers in Human Behavior, 108, 106309. https://doi.org/10.1016/j.chb.2020.106309
- Zamasiya, B., Nyikahadzoi, K., & Mukamuri, B. B. (2017). Factors influencing smallholder farmers’ behavioural intention towards adaptation to climate change in transitional climatic zones: A case study of Hwedza District in Zimbabwe. Journal of Environmental Management, 198(1), 233–239. https://doi.org/10.1016/j.jenvman.2017.04.073
Appendix 1
Table A1. Descriptive statistics of clusters.