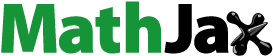
ABSTRACT
We investigated alternative cropping and feeding options for large (>10 cows), medium (5–10 cows) and small (≤4 cows) mixed crop – livestock farm types, to enhance economic and environmental performance in Jhenaidha and Meherpur districts – locations with increasing dairy production – in south western Bangladesh. Following focus group discussions with farmers on constraints and opportunities, we collected baseline data from one representative farm from each farm size class per district (six in total) to parameterize the whole-farm model FarmDESIGN. The six modelled farms were subjected to Pareto-based multi-objective (differential evolution algorithm) optimization to generate alternative dairy farm and fodder configurations. The objectives were to maximize farm profit, soil organic matter balance, and feed self-reliance, in addition to minimizing feed costs and soil nitrogen losses as indicators of sustainability. The cropped areas of the six baseline farms ranged from 0.6 to 4.0 ha and milk production per cow was between 1,640 and 3,560 kg year−1. Feed self-reliance was low (17%–57%) and soil N losses were high (74–342 kg ha−1 year−1). Subsequent trade-off analysis showed that increasing profit and soil organic matter balance was associated with higher risks of N losses. However, we found opportunities to improve economic and environmental performance simultaneously. Feed self-reliance could be increased by intensifying cropping and substituting fallow periods with appropriate fodder crops. For the farm type with the largest opportunity space and room to manoeuvre, we identified four strategies. Three strategies could be economically and environmentally benign, showing different opportunities for farm development with locally available resources.
1. Introduction
Mixed crop – livestock (MCL) farming systems with a focus on dairy production have the potential to be more productive, climate-resilient and sustainable than intensive and specialized systems (Bullock et al., Citation2017). These MCL systems also are key contributors to income and nutrition security in smallholder livelihoods (Sekaran et al., Citation2021). In the dairy production sector, 240 million people are employed worldwide; 133 million dairy farmers keep at least one milking cow (Bos taurus), and dairy products directly and indirectly contribute to the livelihoods of up to one billion people globally (FAO, Citation2016). World annual milk production is about 718 million tons from 264 million dairy cows; however, this will be required to increase by 147 million tons by 2030 to fulfil the increasing demand and nutritional needs of the growing global population (FAO, Citation2020; OECD-FAO, Citation2021). To produce the amount of milk demanded in 2018, total global compound feed production was 1.103 billion tons for all species; 237.8 million tons was used as cattle feed (dairy, calves and beef) (Koeleman, Citation2019). To meet the desired production level for 2030, a larger quantity of feed and increased feeding efficiency will be required (FAO, Citation2016; Mottet et al., Citation2017).
Although Bangladesh is ranked fifteenth in the world in terms of number of dairy cows, total milk yield per animal is low. This results partly from the lower yield achieved by rural (1.2 litres cow−1 day−1) and peri-urban farms (3.5 litres cow−1 day−1) compared to more intensive dairy farm types (9.8 litres cow−1 day−1) (FAO, Citation2016; Huque & Sarker, Citation2014). Despite this, dairy farming in general is marginally profitable, while farmers have ample opportunities to increase output by using more aggregate feed, high yielding breeds, land and labour inputs (Skider et al., Citation2001). Shortages in on-farm production of feed are, however, an important challenge contributing to the low milk yield (Quddus, Citation2018). Producing feed on-farm – for example, by integrating green fodder into cropping systems – could potentially relieve this constraint and would be expected to positively influence milk yield (Ehsanul, Citation2016), but studies are rare which identify what farm reconfiguration (including differing allocation of land to different fodder species) is most advantageous to increasing yield while at the same time achieving profitability increases and limiting environmental externalities.
Cognizant of the need to enhance national milk yield, in 2007 the Government of Bangladesh revised its first national breeding policy (1982) to produce the National Livestock Breeding Policy 2007 (Bhuiyan et al., Citation2017; NLDP, Citation2007). These guidelines focus mainly on crossing indigenous with high-yielding cows, mostly Holstein-Friesian and Sahiwal. As a result, milk yield has increased marginally by up to 1.2 percent annually (Huque & Sarker, Citation2014). Breeding efforts have also been complemented with the growth of commercial artificial insemination entrepreneurs and increased prominence of the Department of Livestock Services (DLS), which provides technical backstopping and extension advice to farmers. The DLS (Citation2021) suggested that as a result of its support, milk production increased by 7.6 percent in 2019–20 compared to the previous year. However, to achieve the potential of the improved genotypes of graded and crossbred cows which are now available to farmers, animal demand for nutrient-rich feed needs to be fulfilled. Since increasing genetic potential through crossbreeding increases milk production, the cow should be provided with sufficient nutrients through feed, depending on the type of production and productivity level. However, this can be challenging when feed is scarce (Khan et al., Citation2009).
To address feed shortages, efforts have been made to encourage farmers’ adoption of cultivated fodder, for instance Napier grass (Pennisetum purpureum Schumach.), and to increase the use and quality of crop residues. The latter is important, as most farmers in Bangladesh provide rice (Oryza sativa L.) straw – which tends to be nutrient poor and difficult for animals to digest – as the primary source of feed, sometimes following urea treatment (Khan et al., Citation2009). In addition, the use of silage (processed and prepared from maize, Napier and others types of forage) may be useful in situations of feed shortage (Khan et al., Citation2013). On occasion, various fodder trees and weeds may also be fed to livestock (Ehsanul, Citation2016; Khan et al., Citation2009). Although fodder crop production is increasing in Bangladesh (Sarker et al., Citation2021), a shortage of fodder of about 45% remains (Huque & Sarker, Citation2014). This is due partly to the scarcity of land available for feed production compared to the cultivation of other economic and food crops. As a result, farmers are increasingly using concentrate to meet the required demand for nutrients needed by animals to ensure appropriate levels of milk production. While this approach has provided a partial stop-gap solution, up to 80 percent of the concentrates used as feed is imported from abroad, making them costly for smaller and resource-poor farmers to access (Huque & Sarker, Citation2014; Quddus, Citation2009). An assessment of the use of imported concentrate is needed to decide whether alternative and more self-reliant options might lower costs and increase profitability for farmers. If alternative options can meet the nutritional requirements of cattle in terms of maintenance and production, this has the potential to improve self-dependency in feed production, which will in turn affect sustainability.
Sustainability is a multi-dimensional concept (Calker et al., Citation2005; Cornelissen et al., Citation2001); in the case of dairy production in Bangladesh, key indicators for sustainability include economic (feed costs, price of products, profits and productivity to ensure food security), environmental (lowered nutrients losses, maintenance and improvement of soil OM balances, reduced greenhouse gas emissions) and animal welfare (health, feed and management of cows) (Cornelissen et al., Citation2001). These issues are directly or indirectly affected by farmer decision-making (Calker et al., Citation2005). Although farmers tend to be more concerned about economic than environmental or animal welfare matters (Ahsan et al., Citation2016; Cabrera et al., Citation2008), convincing farm configuration and management options could be made available which simultaneously improve farm economic performance and address sustainability objectives. When the synergies and potential trade-offs among indicators for different sustainability dimensions are clear, informed choices can be made to direct dairy farm management towards a more sustainable future (Cortez-Arriola et al., Citation2016; Mandryk et al., Citation2014).
Farm sustainability assessments can be based on farm monitoring studies and on in silico modelling, both of which can be used to quantify a suite of sustainability indicators. Modelling tools can be used for farm diagnosis, assessment, re-design, and the analysis of trade-offs and synergies among diverse objectives such as those described above (Groot et al., Citation2010). Exploratory models for MCL farm analysis could also support the re-design of farm management strategies (Cortez-Arriola et al., Citation2014). Simulations permit model users to explore the relationships between various farm performance indicators and to assess options to improve sustainability in an integrated manner (Groot et al., Citation2012; Uddin et al., Citation2012). In this study, we used the whole farm model FarmDESIGN (Groot et al., Citation2012) and the Feed Assessment Tool (FEAST) framework as a discussion support tool to collect field and farm data in focus group discussions related to livestock production and feeding (Duncan & Lukuyu, Citation2017).
This study explored options for improved feeding strategies for small, medium and large dairy farms in two major milk-producing districts in Bangladesh, to enhance sustainability while considering multiple objectives and indicators. The following specific questions were addressed: (1) How do farms of different size categories (i.e. farm types) perform in terms of profitability, resource use efficiency and environmental impact? (2) Which synergies and trade-offs exist between economic and environmental indicators of sustainability for each farm type? and (3) In which ways can the sustainability of smallholder dairy farms be improved?
2. Materials and methods
2.1. Study areas
The study was conducted in the western part of Bangladesh namely Meherpur (71,600 ha) and Jhenaidha (196,500 ha) districts (). These districts are located at an elevation of 13–19 m above sea level, with ambient temperatures ranging between 25–35°C across the year and yearly rainfall ranging between 1374–1857 mm in 2018 (BWDB, Citation2019). The districts were purposefully selected according to four criteria: (1) they feature high density livestock and dairy farms (DLS, Citation2016), (2) the farms commonly raise both indigenous and crossbred cows, (3) farmers choose to cultivate fodder in addition to non-fodder crops, and (4) farmers in these districts were willing to participate in the study. Most farms in the two selected districts can be characterised as MCL systems and according to DLS (Citation2016), arable land for crop cultivation in Meherpur and Jhenaidha – paddy, maize (Zea mays L.), wheat (Triticum aestivum L.) and lentil (Lens culinaris Medik.) – encompasses 65,680 and 173,626 ha, respectively. In terms of livestock found in the two districts, there are 0.14 (Meherpur) and 0.53 (Jhenaidha) million large ruminants (cattle and buffalo), and 0.27 (Meherpur) and 0.49 (Jhenaidha) million small animals (goats and sheep).
2.2. Data collection
Data collection for this study was achieved through focus group discussions (FGDs) with dairy farmers, as well as in-depth surveys with subject matter experts in Bangladesh to hone-in on data required for subsequent simulation modelling.
2.2.1. Focus group discussions
The permission for carrying out FGDs was granted by the district livestock officer, and recruited a local facilitator to manage the process and to purposively identify the focus group areas. We then selected farmers for the FGDs using a snowballing sampling method. A total of 15 farmers verbally agreed to participate in each FGD where we found 2:1 male-to-female ratio. The FGDs lasted about 90 minutes and were held in March 2018 and April 2018 in Dakkhin Shalika (Meherpur) and Bijoypur (Jhenaidha) areas, respectively. Along with the moderator and facilitator, the district livestock officer and local livestock representative joined in the discussion. A village-level approach is typically used for FGDs; instead, we selected questions from the Feed Assessment Tool (FEAST) as a discussion guide. FEAST is an assessment tool developed to collect and analyse data in case study sites on livestock feed resources from animal and crop farming communities (Duncan & Lukuyu, Citation2017). FEAST includes modules that we employed to assess the diversity of farming systems and management strategies in each study district, including those used for livestock management. We used questions from FEAST in order to obtain an overview on farm size, area, animals numbers, crop yields and animal products at the village level. Our questions were therefore focused on general farming system descriptions, management of mixed-crop livestock species, problems and opportunities within the livestock system, and distribution of wealth, land, and other assets among farmers.
2.2.2. Detailed farmer surveys
Following each FGD, depending on the currently available supplied feed, animal numbers, arable land, year round crop-livestock production and farm records, upon their verbal consent, we selected nine farmers from each district to conduct detailed data collection. This was based on Hemme et al. (Citation2004), who suggested and modified a farm typology (small ≤4 cows, medium = 5–10 cows and large ≥ 11 cows) to categorize resource and farm diversity on a generalized basis. In this study, we randomly chose three farms which met these classifications and then one representative farm within the group was selected on the comparative basis of crop cultivation areas, grassland areas, cropping pattern and number of milk producing cows between the farms. To simplify the modelling in FarmDESIGN, one dairy farm was selected from each group. This resulted in six farms (JDL: Jhenaidha large; JDM: Jhenaidha medium; JDS: Jhenaidha small; MPL: Meherpur large; MPM: Meherpur medium; MPS: Meherpur small) being chosen for whole-farm modelling and multi-objective exploration using the FarmDESIGN model. For each interview, a survey tool was prepared to collect detailed data for FarmDESIGN; we collected data from March 2018 to May 2018. In addition to the formal survey, researchers also visited each farm for direct observation of the farming system, and measurement of supplied amount (kg) of daily feed, and of body weight using Schaeffer’s formula (Wangchuk et al., Citation2018). When available, we also assessed records kept by farmers on the use of feed, yield of milk, management of cows, and economic information associated with dairy and farm production. This generated a dataset with a range of information described in , which was used for subsequent modelling efforts.
Table 1. Characteristics, productivity and feeding intensity of selected farms representing large, medium and small-sized dairy farms in Meherpur and Jhenaidha districts in Bangladesh.
2.3. Farm modeling
2.3.1. Model input data
FarmDESIGN is a farm-household bio-economic model which quantifies annual farm socio-economic and environmental indicators and allows the performing of multi-objective optimization to explore potential improvements in farm configuration and performance (Ditzler et al., Citation2019; Groot et al., Citation2012). The model requires a range of information such as soil and climate data (BMD, Citation2018; SRDI, Citation2018), information on farm socio-economic conditions including interest rate (BB, Citation2022; BKB, Citation2022), and costs, labour availability and prices, which we obtained from surveys and farm records as well as from market in the surveyed period. Farm surveys also provided data on cropped area, production, external and farm-grown feed sources, and labour demand and costs. Data on crop and fodder nutrient composition were obtained from the National Research Council (NRC, Citation1988). Observations on animal herd and composition were generated from farm visits, while surveys were used to quantify farm physical assets, dairy herd management and productivity levels, and to identify nutrient requirements, as well as the destination (that is, for consumption or market) for the dairy and other livestock products produced. Finally, surveys were used to generate estimates of manure production and management. The nutrient content of manures produced were sourced from NRC (Citation2001, Citation1989).
2.3.2. Indicator calculations
FarmDESIGN can be used to compute and optimize indicators of feed self-reliance (increasing on-farm feed production and reducing reliance on external feed imports), feed balances, flows and balances of OM, carbon (C), N, phosphorous (P) and potassium (K), operating profit, and feed costs per year (Groot et al., Citation2012). In this study, we selected five important attributes and relevant sustainability indicators for a dairy farming system: operating profit, OM balance and feed self-reliance, feed cost and soil N losses.
The farm operating profit (FP) was calculated as the difference between gross margins of crop and animal production (MC and MA, respectively) and fixed costs for assets (CF), labour costs (CL) and general costs (CG):
The feed self-reliance was the percentage of dry matter in the animal ration that was derived from on-farm produced feeds. The feed costs were the summed costs for all feeds supplied to the livestock.
The organic matter balance (OMB) was calculated at soil level from the difference between OM inputs in crop residues (OMC), green manure and mulch (OMG), feed losses and bedding material (OMF), on-farm produced manure (OMM) and imported manure and fertilizers (OMI), and degradation of manure (OMD), soil organic matter (OMS) and erosion losses (OME):
Soil N loss, is the amount of nitrogen that can be potentially lost by leaching or denitrification, is calculated from the difference between inputs to and known outputs of the soil component, and thus forms the balancing item.
A more detailed description of the calculations is provided in Groot et al. (Citation2012).
2.3.3. Multi-objective optimization
To perform multi-objective optimization, the model uses an evolutionary algorithm (Differential Evolution, or DE; Storn & Price, Citation1997) to generate alternative farm configurations which are evaluated for their performance for selected indicators and iteratively improved. The user can parameterize the algorithm by specifying ranges for decision variables which are related to the production and use of crops, animals, manures on the farm (currently used or newly introduced), and the import and export of resources such as fertilizers, feeds and pesticides. The user can also set ranges for constraints on particular indicators such as feed balance items (for example, intake capacity, dietary energy and protein) and soil nutrient balances (to avoid excessive surpluses or nutrient mining), and determines which indicators are minimized or maximized by the algorithm, that is, sets the objectives.
In the multi-objective optimization, we maximized operating profit, OM balance and feed self-reliance, and minimized feed cost and soil N losses (Calker et al., Citation2001, Citation2005). The multi-objective optimization algorithm generates different reconfigurations of farms by allocating different crop and fodder species to different land areas within the farm, but without expanding farm size or changing the number of fields that farmers reported in surveys. Different types of feed were also included, based on all available options identified by farmers during the FGDs and surveys. We also permitted the model to manipulate the number of animals and source of feed for cows, to improve nutrient composition of on-farm and purchased feed items, and to optimize the existing and future possibility of produced and imported feed options. For the multi-objective optimization algorithm we applied 1,000 iterations to ensure stable outcomes during the optimization process, with DE parameters of 0.85 crossover probability, 0.15 amplitude, and a result set of 1500 alternative solutions.
The result set from multi-objective optimization for the Meherpur large farm was analysed in more detail, because this is where we found the largest room to manoeuvre, and we further explored the opportunity and solution spaces with principal component analysis (PCA) and hierarchical cluster analysis (HCA). Finally, we analysed the Euclidian distance of decision variables (DV) and decision objectives (DO) relative to the original farm configuration for each of the clusters. DO is a measure of the improvement which is achieved, while DV signifies the magnitude of change required in resource allocation, crop area and number of animals; thus, DV reflects the complexity of adjustment needed to reach improvement in the objectives.
3. Results
3.1. Overview of current farm configurations
Three farms representing the large, medium and small farm types were selected from each district for detailed analysis using the FarmDESIGN model. Selected farm characteristics are presented in , and a complete overview of farm characteristics in Table S1. The average crop cultivation area for all farm types was lower in Jhenaidha district (1.3 ha) than Meherpur district (2.6 ha). The use of on-farm feed per livestock unit per year was lower (1,763 kg dry matter (DM)) in Jhenaidha than Meherpur (2,964 kg DM), whereas external feed use per livestock unit was higher in Jhenaidha (2,024 kg DM) than Meherpur (892 kg DM). Milk yield tended to be lower in Meherpur (4.5 kg cow−1 day−1) than Jhenaidha (9.5 kg cow−1 day−1). The number of milk-producing animals per farm ranged from 13 to 14 in large farms, between 5 and 8 in medium-sized, and 2 to 4 in small farms, in both districts. The proportions of external feed use were 71%, 59% and 46% in large, medium and small farms in Jhenaidha, respectively. In Meherpur, all farm types used lower proportions of external feed compared to Jhenaidha (63%, 30% and 34% for large, medium and small farms, respectively). In terms of fodder produced on-farm, rice straw and rice bran were common feeds in both districts. The majority (71%) of farmers in Jhenaidha district also produced “vura” (local grasses available are vura, durba and baksha) and lentil hay; the farmers of Meherpur district produced Napier, wheat grass, maize leaf and maize flour (processed from harvested kernels) for animal feed. In addition, a few farmers bought or processed silage or hay as external feed.
Self-reliance on feed was conversely generally low, regardless of farm size, at 17–57% of feed DM derived from the own farm. Farms in Jhenaidha appeared to have a higher risk of soil N losses and a lower OM balance than the farms in Meherpur. The JDS farm had a high operating profit, while the MPS farm had a high OM balance. The MPM farm demonstrated the highest self-reliance, with low feed costs and soil N losses, although the management practices on this farm, which included a high amount of on-farm fodder production and less dependency on external feed items, were also associated with low profits. Conversely, the JDL farm had a low OM balance (the result of a lower amount of crop residue, and animal and green manure use) but high feed costs, leading to it having the lowest self-reliance.
3.2. Overview of the optimized farming configurations in FarmDESIGN
Optimization modelling of the five sustainability indicators showed how the reconfiguration of components of each farm type could lead to substantial improvements in feed self-reliance, operating profit and OM balance, as well as lower feed costs and soil N losses. Pareto-based multi-objective optimization using the five objectives resulted in contrasting opportunity spaces for each of the three farm types in both districts (). A trade-off was observed between OM balance and N losses (), in which higher OM balances caused by higher feed imports (and thus lower self-reliance; ) were also associated with increased N losses.
Figure 2. Opportunity spaces obtained by multi-objective optimization with five objectives surrounded by a hull (solid line) for the six farm types representing large, medium and small-sized dairy farm types in Meherpur and Jhenaidha districts in Bangladesh. Each dot denotes a different configuration of the farm representing the farm type.

Our model explorations indicated that larger farms in both districts appear to be able to maintain larger livestock numbers than small and medium-sized farms (). Larger farms in Jhenaidha had more dairy cows, while the number of heifers and bulls was greater on large farms in Meherpur (). Rather than showing a trend towards increased self-reliance in terms of feed, larger livestock numbers on these farms were associated with more imported feed (), with the large farms in Jhenaidha more dependent on imported feed than in Meherpur (). Land area devoted to paddy production () and improved or modified cropping systems (), and imported feed imports and animal numbers () was considerably greater on Meherpur’s large farms than for the other farm types. This was clearly reflected in the larger opportunity space to improve the economic and environmental indicators ().
Figure 3. Ranges of fractions of cropping areas, animal numbers, imported feeds and modified alternative cropping areas obtained in the multi-objective FarmDESIGN optimization, with five sustainability indicators for six dairy farm types comprising large, medium and small dairy farm types in Meherpur and Jhenaidha districts, Bangladesh.

3.3. Cluster analysis
Because the Meherpur large farm had the largest opportunity space () and room to manoeuvre (), we further analysed the opportunity and solution spaces of this farm type using principle component and cluster analysis to discern patterns which could indicate coherent strategies for farm development (Figures S1–S4). Hierarchal clustering analysis identified four distinct clusters (Figure S3). Sustainability indicators () and decision variables () suggested that contrasting approaches would be required to reach one or more objectives in unison. Here, cluster 4 (shown in orange in ) could potentially allow farmers to reach high levels of profitability and self-reliance, although this was associated with intermediate levels of feed costs and OM balances, and N losses from the farming system. Cluster 2 (shown in red in ) attained high self-reliance, low feed costs and N losses, but low profitability and OM balance. Clusters 1 and 3 showed a tendency towards higher feed costs and lower self-reliance in feed production and use (cluster 1, shown in green in ), and/or with high N losses (cluster 3, shown in blue in ), which were considered a medium performance for profit.
Figure 4. Indicator values for the objectives of options generated by multi-objective optimization for large farms in Meherpur, clustered into four types.

Figure 5. Areas of cropping patterns from options generated by multi-objective optimization for Meherpur large farms, clustered into four types. Note the differences y-axis ranges between panels a – c and d – e.

Figure 6. Imported feeds used in options generated by multi-objective optimization for Meherpur large farms, clustered into four types.

Figure 7. Animal numbers in options generated by multi-objective optimization for Meherpur large farms, clustered into four types.

Model optimization suggested that clusters 1 and 3 could cultivate larger areas of paddy rice, while clusters 2 and 4 could engage in farm diversification with the production of lentil, wheat and maize, all of which could be used as a green fodder (). Areas of single cropping or less intensified cropping systems – wheat, lentil and paddy rice (non-irrigated) – were limited in the optimized solutions ().
The observed clusters suggested the need for different feeding strategies in order to achieve farm improvement. Cluster 2 contained farm configurations using a small amount of external feed (maize bran, wheat bran, rice straw, maize silage and treated rice straw), while cluster 1 consisted of redesigned farms which would have the highest amount of external feed (), resulting in the lowest self-reliance. In clusters 2, 3 and 4 the use of maize and wheat bran as feed tended to be limited (); cluster 3 used the largest amount of maize silage (); farms in clusters 1, 3 and 4 used large quantities of rice straw with or without ammonia treatment ().
Cluster 2 options had low numbers of animals (); cluster 4 had the largest number of dairy cows and a low number of bulls. For all clusters, the number of heifers was relatively stable (determined by a decision variable of between 4 and 6).
Cluster 2 comprised solutions that would reduce the intensity of farming activities to increase feed self-sufficiency, which would lead to low profitability and OM balance. At the same time, losses of N to the environment were lowest for this cluster. Cluster 4 was livestock-oriented, with the most intensified and profitable farm configurations and a diversified cropping pattern allowing relatively high self-reliance, resulting in an intermediate OM balance and high N losses. Similarly, Cluster 1 was also livestock-oriented but focused on externally obtained feeds. As a result, farms in this cluster would have higher feed imports and associated costs; self-reliance was thus low and profits intermediate. Finally, Cluster 3 appears to have the highest OM balance but also high N losses and feed costs. This is representative of the input-intensive nature of this farm cluster that tended to achieve relatively high profits during simulation.
shows the Euclidian distance of decision variables (DV) and decision objectives (DO), respectively relative to the original farm configuration for each of the clusters. Although the required adjustments increased for consecutive clusters 1–4 (), the relative improvement was the same for clusters 2, 3 and 4 (), although the clusters performed differently for the various objectives.
4. Discussion
4.1. Enhancement of economic performance
Our simulation modelling of dairy operations in MCL farms in Bangladesh showed that increased profitability could be reached by adjustments in cropping patterns (that is, crop sequencing in the field), crop cultivation areas (allocation of different cropping patterns within the farm to different fields and field sizes), sources of external feed acquisition and on-farm feed production, and number of animals and their productivity. We found profitable and intensified livestock-oriented farm configurations with diversified cropping patterns, whereas the trade-offs with environmental indicators resulted in intermediate OM balances and higher N losses. The use of external feed sources, particularly maize silage, could possibly influence higher farm profits.
Although challenging in Bangladesh’s land- and labour-scarce agricultural systems, adjustments to cropping patterns are achievable, as demonstrated during the survey by farmers neighbouring our sample, who we found had started to practice the cropping patterns we had proposed. Modelling found that replacing paddy or increasing cropping intensity, with Napier grass, maize, lentil hay, wheat grass and legumes (maskalai) cultivated as fodder to reduce feed costs, would result in major changes. On-farm feed production can be increased by cultivating leguminous or short-term feed-producing crops in the fallow periods (between or after 2 paddy or other crops). We found that farmers can grow a wide variety of crops, and that replacing species or changing crop rotations or adding additional crops may be possible using existing land areas and resources, at least for the farms studied in this paper. In MCL systems, crop intensification is mainly achieved by changing the crop rotational pattern or adding crops. For example, where soil moisture or irrigation are not limiting, farmers could make use of the fallow period between economic crops to cultivate fodder crops. Utilisation of fallow land and alternative feeds from irregular sources has been effectively used by MCL farmers in India (Alam et al., Citation2022, Citation2023; Srinivasa Rao et al., Citation2016), and seasonal fallow played a role for fodder production in Nepal (Alomia-Hinojosa et al., Citation2018). The utilisation of fallow land, e.g. at the roadside, farm boundaries has played an additional role in meeting the demand for animal feed in sub-Saharan Africa (Paul et al., Citation2020). In addition, the utilisation of alternative feed resources could improve production and reduce the environmental impact (Jaleta et al., Citation2013). Other studies have demonstrated that intensification through integrated mixed-crop-tree-livestock systems can increase sustainable production based on improved fodder production systems (Notenbaert et al., Citation2021; Paul et al., Citation2020).
We simulated the consequences of maximizing operating profits and self-reliance (by increasing on-farm feed production), and minimized feed costs, by decreasing the use of external feed (mostly wheat bran and rice straw) and increasing the production of on-farm feed. Our model results suggested that these changes could be associated with a large reduction in wheat bran and rice straw as external feed in most feeding strategies (clusters 2, 3 and 4; ). To compensate for this, we identified solutions incorporating increased use of urea-treated rice straw and maize silage as a new external feed, and vura, Napier, maize and lentil as green grass as on-farm feed. As the top 10 rice-producing countries, including China, India, Indonesia, Bangladesh, Vietnam, Thailand, Myanmar, Philippines, Brazil, and Japan, collectively generate over 227 million tons of rice straw as a low-cost natural by-products annually (Sarnklong et al., Citation2010). Consequently, the proper utilization of rice straw has become a significant concern. Treated rice straw, employing methods such as physical, chemical, and biological treatments, has been frequently employed for animal feeding in countries like India (Malik et al., Citation2015), Bangladesh (Uddin et al., Citation2012), Egypt (El-Dewany et al., Citation2018) and several other rice-producing nations (Sarnklong et al., Citation2010). In the interest of environmental preservation, biological treatment has been widely recommended (Srinivasa Rao et al., Citation2016).
A study focused on Asian small scale mixed crop livestock systems and found that the use of maize silage in feed rations increased milk yield by 11% over a milk production year, compared to the use of dried leguminous hay (Paris, Citation2002). In addition, silage can be preserved on-farm and used to feed animals economically during times of feed scarcity (Densley et al., Citation2011). Although this has various positive impacts, the processing and adaptation of maize silage is new in Bangladesh, but farmers have 12% more milk yield with a higher profit by using silage from low-cost silage processing technology (Khan et al., Citation2009). They also reported on interest among farmers in using silage as animal feed in future farming. A few silage entrepreneurs currently supply silage throughout Bangladesh on a basis which, although commercial, is still cost-effective as animal feed. Other South Asian countries, including India, Nepal, Bhutan, Sri Lanka, Maldives, Afghanistan, and Pakistan, have highlighted the significance of employing silage for animal feeding, especially to address feed shortages (Samanta et al., Citation2019). Comparable suggestions have also been put forth for nations in sub-Saharan Africa, including South Africa, Kenya, and Nigeria (Balehegn et al., Citation2021).
On-farm fodder production has the potential to decrease external feed dependency and reduce feed costs as a result. Val-Arreola et al. (Citation2004) optimized small-scale farm practices in Mexico by linear programing land use, fodder yield and nutrient availability for cows, concluding that on-farm feed production increased nutrient availability and milk yield (by 40%). Another study conducted by Khan et al. (Citation2009) looked at the effects of feeding strategies on indigenous and crossbred cows in Bangladesh. They found that crossbred cows fed higher grass produced more milk than indigenous cows fed lower grass. Profit was also higher in higher grass fed cows than in lower grass fed cows. An increasing relationship between fodder cultivation and higher profitability was also found by Roy et al. (Citation2012) in Meherpur. The reliance on on-farm feed production for economic sustainability was observed in India (Mahanta et al., Citation2020). This approach has also been recommended in the broader Asian context (Devendra & Leng, Citation2011).
During modelling, we used locally available feed items (such as wheat bran and rice bran) as external feed, and similarly suggested locally cultivated crops or grass species (such as wheat grass and maize grass) as feed, which, if used scientifically, fulfilled dairy cow nutrient requirements in terms of both maintenance and production ration. However, in Bangladesh, farmers generally lack awareness of scientific feeding strategies and how to practice them: most provide rice straw as basal mixed with grass and concentrate as a matter of course, resulting in a highly varied fulfilment of nutrients (Khan et al., Citation2009). Participatory training and awareness raising efforts regarding the relationships between fodder quality, animal health, and milk yield therefore need to be intensified in Bangladesh. The use of locally available feed items was highly frequently was very common as animal feed in India (Alam & Silpa, Citation2020; Alam et al., Citation2022; Samanta et al., Citation2019). In recent years, urban farmers have been trying to collect food waste from the food industry, restaurants and supermarkets as an alternative feed for their animals (Alam et al., Citation2023; Reichenbach, Citation2020).
4.2. Enhancement of environmental sustainability
As discussed above, the maximization of OM balance and minimization of soil N losses could, according to simulation results, enhance the environmental sustainability of farming systems and could potentially be achieved by changing cropping pattern and/or intensity. The opportunities for changing cropping patterns were however found by this study to be greater for large farms than for medium and small farms, owing to the larger crop cultivation area. This presents a social-equity dilemma that will need to be addressed in agricultural development efforts that include dairy feed and milk value chain components, as solutions beneficial to more marginal farmers remain a key area of concern.
Environmental improvement opportunities were also found for clusters 1 and 4. However, due to the trade-off between OM balance (which is higher largely when feed imports are higher) and N losses, compromises would be needed. We identified solutions which had the potential to benefit economic and environmental objectives simultaneously, in particular for cluster 4, that provided compromise solutions with high operating profit and feed self-reliance. Improvement of OM balance by introducing on-farm improvements (rather than by increasing the use of imported feed) could be achieved with the introduction of maskalai, vura, lentil and maize grasses (instead of irrigated paddy rice cultivation) on ca. 13 percent of the farm area. This would reduce the year-round rice yield by a minute amount, while at the same time the altered crops would add crop residues as green manure to the soil and, if fed to animals, result in more manure production. The improvement of soil health can be improved through crop residues (Jaleta et al., Citation2013). In addition, crop rotations with temporary fodder species, where possible, improve soil quality compared to annual crops (Viaud et al., Citation2018).
Our simulations suggest that farmers’ dependence on inorganic fertilizers could be reduced as a result of the inclusion of lentil, maskalai, Napier, wheat, vura and cabbage. This is because leguminous crops symbiotically fix atmospheric nitrogen and non-legume crops require less inorganic fertilizer than paddy cultivation. The decision to introduce new crops or replace others is dependent on soil type, weather and farmer interest. A study of different annual crop rotations in the tropics (specifically, India) (Chander et al., Citation1997) found that green manuring improved the OM level and soil microbial activity (the latter being vital for long-term soil productivity and nutrient turnover). Incorporating leguminous crops can accumulate 2.6 kg N ha−1 day−1 (Fageria, Citation2007). The practice of green manuring with intercropping, specifically focusing on leguminous crops to improve soil fertility, was also practised in sub-Saharan Africa (Bationo et al., Citation2007; Lupwayi et al., Citation2007).
5. Conclusions
Model-based explorations for six MCL farms focusing on dairy production demonstrated that options exist for simultaneously increasing operating profit, self-reliance and OM balance, and reducing feed costs and soil N losses by using existing farm resources, and adjusting and intensifying cropping patterns. Our trade-off analysis showed that increasing profit and OM balance was however often associated with higher N losses, due to larger input levels. For the farm with the largest opportunity space and room to manoeuvre, we identified four strategies for farm development, of which three are likely to be economically viable. Our study also demonstrates how an integrated analysis of alternative feeding systems for sub-tropical dairy farms can enhance understanding of the potential for combined economic and environmental benefits in MCL production systems, thereby overcoming apparent trade-offs and offering farmers a suite of management options to explore. Improvements appear to be achievable with the careful inclusion of fodder and crop species, and through the objective-oriented reconfiguration of existing farm practices. Simultaneously, the four identified strategies for farm development can significantly contribute to the success of integrated crop-livestock farming systems in South Asia and other countries where integrated crop-livestock farming is prevalent.
Data protection and participation consent
All interviewees participating in this study were fully informed about the study purpose and were assured that their information would be treated anonymously. As a result, only persons giving explicit informed verbal consent were interviewed and at the end of the interview, the farmers consent signature was taken.
Supplemental Material
Download MS Word (926 KB)Acknowledgments
This study was supported by the NUFFIC fellowship program in the Netherlands, the Cereal Systems Initiative for South Asia (CSISA) project (https://csisa.org/), funded by the United States Agency for International Development (USAID) and the Bill and Melinda Gates Foundation (BMGF). Analysis and write-up work was also supported in part by the CGIAR Regional Integrated Initiative Transforming Agrifood Systems in South Asia, or TAFSSA (https://www.cgiar.org/initiative/20-transforming-agrifoodsystems-in-south-asia-tafssa/) and the Sustainable Intensification of Mixed Farming Systems (SIMFS) Initiative (https://www.cimmyt.org/projects/cgiar-initiative-sustainable-intensification-of-mixed-farming-systems-si-mfs/). The views of this manuscript do not necessarily reflect those of NUFFIC, USAID, BMGF, CGIAR, or the United States Government, and its contents shall not be used for advertising purposes. We deeply thank all the dairy producers and their families who participated in this research for their time. We also thank our Bangladeshi coordinator and research assistants for their commitment and diligent survey work.
Disclosure statement
No potential conflict of interest was reported by the author(s).
Data availability statement
For scientific purposes, data can be made available to individual scientists upon written request to the corresponding author.
Supplementary material
Supplemental data for this article can be accessed online at https://doi.org/10.1080/27685241.2023.2290046
Additional information
Funding
References
- Ahsan, S., Islam, M. A., & Islam, T. (2016). On-farm welfare assessment of dairy cattle by animal-linked parameters in Bangladesh. Research in Agriculture Livestock and Fisheries, 3, 417–26.
- Alam, M. S., Schlecht, E., & Reichenbach, M. (2022). Impacts of COVID-19 on small-scale dairy enterprises in an Indian megacity—insights from greater bengaluru. Sustainability, 14, 2057. https://doi.org/10.3390/su14042057
- Alam, M. S., & Silpa, M. V. (2020). Impacts of heavy metal feed contaminants in cattle farming. In V. Seijian, S. S. Chauhan, P. K. Malik, G. Krishnan, M. Bagath, A. P. Kolte, & R. Bhatta (Eds.), Transfer of mitigation technologies for heat stress in farm animals (pp. 147–152). NIANP.
- Alam, M. S., Velayudhan, S. M., Dey, D. K., Adilieme, C., Malik, P. K., Bhatta, R., König, S., & Schlecht, E. (2023). Urbanisation threats to dairy cattle health: Insights from greater Bengaluru, India. Tropical Animal Health and Production, 55, 350. https://doi.org/10.1007/s11250-023-03737-7
- Alomia-Hinojosa, V., Speelman, E. N., Thapa, A., Wei, H. E., McDonald, A. J., Tittonell, P., & Groot, J. C. J. (2018). Exploring farmer perceptions of agricultural innovations for maize-legume intensification in the mid-hills region of Nepal. International Journal of Agricultural Sustainability, 16, 74–93. https://doi.org/10.1080/14735903.2018.1423723
- Balehegn, M., Ayantunde, A., Amole, T., Njarui, D., Nkosi, B. D., Müller, F. L., Meeske, R., Tjelele, T. J., Malebana, I. M., Madibela, O. R., Boitumelo, W. S., Lukuyu, B., Weseh, A., Weseh10, A., Minani, E., & Adesogan, A. T. (2021). Forage conservation in sub-Saharan Africa: Review of experiences, challenges, and opportunities. Agronomy Journal, 114, 75–99. https://doi.org/10.1002/agj2.20954
- Bationo, A., Kihara, J., Vanlauwe, B., Waswa, B., & Kimetu, J. (2007). Soil organic carbon dynamics, functions and management in West African agro-ecosystems. Agricultural Systems, 94, 13–25. https://doi.org/10.1016/j.agsy.2005.08.011
- BB. (2022). Financial inclusion and growth expediting programs [WWW Document]. Bangladesh Bank. URL. https://www.bb.org.bd/en/index.php/about/financial_inclusion
- Bhuiyan, M. S. A., Lee, J., & Lee, S. H. (2017). Community based livestock breeding programs in Bangladesh: Present status and challenges. Journal of Animal Breeding and Genetics, 1, 1–8. https://doi.org/10.12972/jabng.20170009
- BKB. (2022). Live stock and continuous loan [WWW Document]. Bangladesh Krishi Bank. URL. https://www.krishibank.org.bd/core-business/credit-programs/live-stock-continuous-loan/
- BMD, 2018. Bangladesh meteorological department [WWW Document]. Retrieved February 4, http://live.bmd.gov.bd//.
- Bullock, J. M., Dhanjal-Adams, K. L., Milne, A., Oliver, T. H., Todman, L. C., Whitmore, A. P., Pywell, R. F., & Bardgett, R. (2017). Resilience and food security: Rethinking an ecological concept. The Journal of Ecology, 105, 880–884. https://doi.org/10.1111/1365-2745.12791
- BWDB. (2019). Summary of rainfall in Bangladesh for the year 2017 & 2018. Bangladesh Water Development Board.
- Cabrera, V. E., Breuer, N. E., & Hildebrand, P. E. (2008). Participatory modeling in dairy farm systems: A method for building consensual environmental sustainability using seasonal climate forecasts. Climatic Change, 89, 395–409. https://doi.org/10.1007/s10584-007-9371-z
- Calker, K. J. V., Berentsen, P. B. M., Giesen, G. W. J., & Huirne, R. B. M. (2001). Method for measuring sustainability in dairy farming. Advances in Ecological Research Science, 46, 69–78. https://doi.org/10.2495/ECO010071
- Calker, K. J. V., Berentsen, P. B. M., Giesen, G. W. L., & Huirne, R. B. (2005). Identifying and ranking attributes that determine sustainability in Dutch dairy farming. Agriculture and Human Values, 22, 53–63. https://doi.org/10.1007/s10460-004-7230-3
- Chander, K., Goyal, S., Mundra, M. C., & Kapoor, K. K. (1997). Organic matter, microbial biomass and enzyme activity of soils under different crop rotations in the tropics. Biology and Fertility of Soils, 24, 306–310. https://doi.org/10.1007/s003740050248
- Cornelissen, A. M. G., Van Den Berg, J., Koops, W. J., Grossman, M., & Udo, H. M. J. (2001). Assessment of the contribution of sustainability indicators to sustainable development: A novel approach using fuzzy set theory. Agriculture, Ecosystems & Environment, 86, 173–185. https://doi.org/10.1016/S0167-8809(00)00272-3
- Cortez-Arriola, J., Groot, J. C. J., Améndola Massiotti, R. D., Scholberg, J. M. S., Valentina Mariscal Aguayo, D., Tittonell, P., & Rossing, W. A. H. (2014). Resource use efficiency and farm productivity gaps of smallholder dairy farming in North-west Michoacán, Mexico. Agricultural Systems, 126, 15–24. https://doi.org/10.1016/j.agsy.2013.11.001
- Cortez-Arriola, J., Groot, J. C. J., Rossing, W. A. H., Scholberg, J. M. S., Améndola Massiotti, R. D., & Tittonell, P. (2016). Alternative options for sustainable intensification of smallholder dairy farms in North-West Michoacán, Mexico. Agricultural Systems, 144, 22–32. https://doi.org/10.1016/j.agsy.2016.02.001
- Densley, R. J., Williams, I. D., Kleinmans, J. J., Mccarter, S. B., & Tsimba, R. (2011). Use of maize silage to improve pasture persistence in dairy farm systems: A review. Pasture Persistence- Grassland Research and Practice Series, 15, 217–222. https://doi.org/10.33584/rps.15.2011.3205
- Devendra, C., & Leng, R. A. (2011). Feed Resources for animals in Asia: Issues, strategies for use, intensification and integration for increased productivity. Asian-Australasian Journal of Animal Sciences, 24, 303–321.
- Ditzler, L., Komarek, A. M., Chiang, T. W., Alvarez, S., Chatterjee, S. A., Timler, C., Raneri, J. E., Carmona, N. E., Kennedy, G., & Groot, J. C. J. (2019). A model to examine farm household trade-offs and synergies with an application to smallholders in Vietnam. Agricultural Systems, 173, 49–63. https://doi.org/10.1016/j.agsy.2019.02.008
- DLS, 2016. Department of livestock services. Ministry of livestock and Fisheries, Dhaka, Bangladesh. [WWW Document]. http://old.dls.gov.bd/database.php
- DLS. (2021). Department of livestock services. Ministry of livestock and Fisheries. Livestock Economy at a Glance [WWW Document]. URL http://www.dls.gov.bd/site/page/22b1143b-9323-44f8-bfd8-647087828c9b/Livestock-Economy
- Duncan, A. J., & Lukuyu, B., 2017. The feed assessment tool (FEAST). Poster prepared for the 7th Multi-Stakeholder Partnership meeting of the Global Agenda for Sustainable Livestock. Addis Ababa, May 8-12. 2017. Nairobi, Kenya: ILRI.
- Ehsanul, H. M. D. (2016). Suitable integrated crop-livestock production System in Char area of Bangladesh. Journal of Fisheries & Livestock Production, 4, 1000156. https://doi.org/10.4172/2332-2608.1000156
- El-Dewany, C., Awad, F., & Zaghloul, A. M. (2018). Utilization of Rice Straw as a low-cost natural by-product in Agriculture. International Journal of Environmental Pollution and Environmental Modelling, 1(4), 91–102.
- Fageria, N. K. (2007). Green manuring in crop production. Journal of Plant Nutrition, 30, 691–719. https://doi.org/10.1080/01904160701289529
- FAO, 2016. FAOSTAT database. Food and Agriculture organization of the United nations [WWW Document]. Retrieved January 17, 2018 http://faostat.fao.org.
- FAO, 2020. FAOSTAT database. Food and Agriculture organization of the United nations [WWW Document]. http://faostat.fao.org/
- Groot, J. C. J., Jellema, A., & Rossing, W. A. H. (2010). Designing a hedgerow network in a multifunctional agricultural landscape: Balancing trade-offs among ecological quality, landscape character and implementation costs. The European Journal of Agronomy, 32, 112–119. https://doi.org/10.1016/j.eja.2009.07.002
- Groot, J. C. J., Oomen, G. J. M., & Rossing, W. A. H. (2012). Multi-objective optimization and design of farming systems. Agricultural Systems, 110, 63–77. https://doi.org/10.1016/j.agsy.2012.03.012
- Hemme, T., Garcia, O., & Khan, A. R. (2004). A review of milk production in Bangladesh with particular emphasis on small-scale producers. Pro-Poor Livest Policy, 7, 1–38.
- Huque, K., & Sarker, N. (2014). Feeds and feeding of livestock in Bangladesh: Performance, constraints and options forward. Bangladesh Journal of Animal Science, 43, 1–10.
- Jaleta, M., Kassie, M., & Shiferaw, B. (2013). Tradeoffs in crop residue utilization in mixed crop-livestock systems and implications for conservation agriculture. Agricultural Systems, 121, 96–105. https://doi.org/10.1016/j.agsy.2013.05.006
- Khan, M. M. R., Miah, S. H., & Irfanullah, H. M. (2013). Small-scale silage-making technology for the extreme poor on floodplains. The International Journal of Environmental Studies, 70, 192–202. https://doi.org/10.1080/00207233.2013.773717
- Khan, M. J., Peters, K. J., & Uddin, M. M. (2009). Feeding strategy for improving dairy cattle productivity in small holder farm in Bangladesh. Bangladesh Journal of Animal Science, 38, 67–85. https://doi.org/10.3329/bjas.v38i1-2.9914
- Koeleman, E. (2019). World produces more cattle feed in 2018 [WWW Document]. Dairy Glob. https://www.dairyglobal.net/industry-and-markets/market-trends/world-produces-more-cattle-feed-in-2018/
- Lupwayi, N. Z., Kennedy, A. C., & Chirwa, R. M. (2007). Grain legume impacts on soil biological processes in sub Saharan Africa. African Journal of Plant Science, 5(1), 1–7.
- Mahanta, S. K., Garcia, S. C., & Islam, M. R. (2020). Forage based feeding systems of dairy animals: Issues, limitations and strategies. Range Management and Agroforestry, 41(1), 188–199.
- Malik, K., Tokkas, J., Anand, R. C., & Kumari, N. (2015). Pretreated rice straw as an improved fodder for ruminants-an overview. Journal of Applied and Natural Science, 7, 514–520. https://doi.org/10.31018/jans.v7i1.640
- Mandryk, M., Reidsma, P., Kanellopoulos, A., Groot, J. C. J., & van Ittersum, M. K. (2014). The role of farmers’ objectives in current farm practices and adaptation preferences: A case study in Flevoland, the Netherlands. Regional Environmental Change, 14, 1463–1478. https://doi.org/10.1007/s10113-014-0589-9
- Mottet, A., de Haan, C., Falcucci, A., Tempio, G., Opio, C., & Gerber, P. (2017). Livestock: On our plates or eating at our table? A new analysis of the feed/food debate. Global Food Security, 14, 1–8. https://doi.org/10.1016/j.gfs.2017.01.001
- NLDP, 2007. National livestock development Policy, department of livestock services, Ministry of Fisheries and livestock, Government of the people’s Republic of Bangladesh [WWW Document]. http://nda.erd.gov.bd/files/1/Publications/SectoralPoliciesandPlans/Livestock_Policy_Final.pdf
- Notenbaert, A. M. O., Douxchamps, S., Villegas, D. M., Arango, J., Paul, B. K., Burkart, S., Rao, I., Kettle, C. J., Rudel, T., Vázquez, E., Teutscherova, N., Chirinda, N., Groot, J. C. J., Wironen, M., Pulleman, M., Louhaichi, M., Hassan, S., Oberson, A. … Peters, M. (2021). Tapping into the environmental co-benefits of improved Tropical forages for an agroecological transformation of livestock production systems. Frontiers in Sustainable Food Systems, 5. https://doi.org/10.3389/fsufs.2021.742842
- NRC. (1988). Nutrient requirements of dairy cattle (7th Edition ed.). National Academy of Sciences Press.
- NRC. (1989). Nutrient requirements of dairy cattle (Sixth Revi. ed.). National Academy Press.
- NRC. (2001). Nutrient requirements of dairy cattle (Seventh Re. ed.). National Academy Press.
- OECD-FAO. (2021). Table C.5 - world dairy projections: Milk, butter and cheese. In OECD-FAO agricultural outlook 2021-2030. OECD Publishing. https://doi.org/10.1787/0ca74b06-en
- Paris, T. R. (2002). Crop–animal systems in Asia: Socio-economic benefits and impacts on rural livelihoods. Agricultural Systems, 71, 147–168. https://doi.org/10.1016/S0308-521X(01)00041-5
- Paul, B. K., Koge, J., Maass, B. L., Notenbaert, A., Peters, M., Groot, J., & Tittonell, P. (2020). Tropical forage technologies can deliver multiple benefits in sub-Sahara Africa: A quantitative review. Agronomy for Sustainable Development, 40, 22. doi:10.1007/s13593-020-00626-3.
- Quddus, M. A. (2009). Adoption of dairy farming technologies by small farm holders: Practices and constraints. Bangladesh Journal of Animal Science, 41, 124–135. https://doi.org/10.3329/bjas.v41i2.14132
- Quddus, M. A. (2018). Smallholder dairy farming in Bangladesh: A socioeconomic analysis. The Bangladesh Journal of Agricultural Economics, 37(1–2), 1–25.
- Reichenbach, M., 2020. Dairy production in an urbanizing environment: A system approach in Bengaluru, India. PhD thesis, University of Kassel, 1–110. https://doi.org/10.17170/kobra‐202010221984
- Roy, B. K., Sarker, N. R., Alam, M. K., & Huque, K. S. (2012). Existing production and marketing system of fodder under Meherpur district as livelihood activity. Bangladesh Journal of Livestock Research, 19, 24–32. https://doi.org/10.3329/bjlr.v19i1-2.26424
- Samanta, A. K., Bokthtiar, S. M., & Ali, M. Y. (2019). Livestock feeds and feeding practices in South Asia. SAARC Agriculture Centre.
- Sarker, N. R., Rahman, S. M. R., & Khan, M. Y. A. (2021). Feeds & fodder production in Bangladesh : Present trend, availability, future requirements and challenges for dairying in 2030. In 3rd international exhibition on dairy, aqua and pet animals (pp. 1–9). BCCIC.
- Sarnklong, C., Coneja, J. W., Pellikaan, W., & Hendriks, W. H. (2010). Utilization of rice straw and different treatments to improve its feed value for ruminants: A review. Asian-Australasian Journal of Animal Sciences, 23, 680–692. https://doi.org/10.5713/ajas.2010.80619
- Sekaran, U., Lai, L., Ussiri, D. A. N., Kumar, S., & Clay, S. (2021). Role of integrated crop-livestock systems in improving agriculture production and addressing food security – a review. Journal of Agriculture and Food Research, 5, 100190. https://doi.org/10.1016/j.jafr.2021.100190
- Skider, M. K. I., Alam, M. J., & Azad, M. S. (2001). Profitability and resource use efficiency of commercial dairy farms in Dhaka and Gazipur districts. Bangladesh Journal of Animal Science, 30, 115–122.
- SRDI, 2018. Soil resource development institute [WWW Document]. http://www.srdi.gov.bd/sites/default/files/files/srdi.portal.gov.bd/annual_reports/482e91a3_1756_4e8e_aa4b_e7480415c402/2020-08-16-15-18-0eec130cc27a84d0b61fa3e4d3e13b0d.pdf
- Srinivasa Rao, C., Gopinath, K. A., Prasad, J. V. N. S., & Prasannakumar, S. A. K. (2016). Climate resilient villages for sustainable food security in Tropical India: Concept, process, technologies, institutions, and impacts. Advances in Agronomy, 140, 101–214. https://doi.org/10.1016/bs.agron.2016.06.003
- Storn, R., & Price, K. (1997). Differential evolution – a simple and efficient heuristic for Global optimization over continuous spaces. Journal of Global Optimization, 11, 341–359. https://doi.org/10.1023/A:1008202821328
- Uddin, M. M., Sultana, M. N., Bruermer, B., & Peters, K. J. (2012). Assessing the impact of dairy policies on farm-level profits in dairy farms in Bangladesh: Benchmarking for rural livelihoods improvement Policy. Journal of Reviews on Global Economics, 1, 124–138. https://doi.org/10.6000/1929-7092.2012.01.11
- Val-Arreola, D., Kebreab, E., Mills, J. A. N., Wiggins, S. L., & France, J. (2004). Forage production and nutrient availability in small-scale dairy systems in central Mexico using linear programming and partial budgeting. Nutrient Cycling in Agroecosystems, 69, 191–201. https://doi.org/10.1023/B:FRES.0000035173.67852.e8
- Viaud, V., Santillàn-Carvantes, P., Akkal-Corfini, N., Le Guillou, C., Prévost-Bouré, N. C., Ranjard, L., & Menasseri-Aubry, S. (2018). Landscape-scale analysis of cropping system effects on soil quality in a context of crop-livestock farming. Agriculture, Ecosystems & Environment, 265, 166–177. https://doi.org/10.1016/j.agee.2018.06.018
- Wangchuk, K., Wangdi, J., & Mindu, M. (2018). Comparison and reliability of techniques to estimate live cattle body weight. Journal of Applied Animal Research, 46, 349–352. https://doi.org/10.1080/09712119.2017.1302876