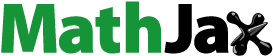
Abstract
Intermediate Public Transport (IPT) modes constitute a significant share as access modes to main haul transit systems in major Indian cities. This study employs a user perception-based approach to prioritize essential attributes affecting service quality of IPT access modes to metro stations. Face-to-face user interviews were conducted using smart tablets at entrance/departure gates and metro platforms in Delhi, India. User responses concerning the importance of 18 identified IPT service attributes were collected on a six-point Likert-type rating scale. The database was analyzed by three multi-attribute decision-making techniques, Relative to an Identified Distribution Integral Transformation, Grey Relational Analysis and Technique for Order Preference by Similarity to the Ideal Solution to obtain user priority-based rankings of attributes. Spearman’s rank-order correlation analysis was performed to check the variations in attribute rankings derived from the three methods. The coefficients indicated consistency in the attribute rankings and a positive relationship among the ranking methods. The research findings indicated that the users perceived the qualitative and design-related attributes of IPT modes to be highly important and need improvement on top priority. These outcomes can aid transportation planners and policymakers in devising rational policies dedicated toward service quality improvement of IPT access modes in developing countries like India.
1. Introduction
The increase in private motorization to meet the mobility needs of the ever-increasing urban population has resulted in several transport-related negative externalities such as traffic congestion, air and noise pollution, fatalities due to road accidents, and a deteriorated quality of life in major Indian cities. The introduction of metro rail facilities in several metropolitan cities of India has been one of the major policy interventions which encourages the use of sustainable transportation to handle the urban mobility requirements suitably and curtail the usage of private vehicles. However, first and last-mile connectivity is a very common issue associated with the metro systems (Ministry of Urban Development [MoUD], Citation2014; Saiyad, Srivastava, & Rathwa, Citation2022). This is supplemented by various types of feeder modes which facilitate suitable accessibility to the metro stations and encourage the use of public transport. Researchers have explored the factors influencing the choice of such modes in past studies as well (Goel & Tiwari, Citation2016; Ji et al., Citation2017; Liu, Bardaka, & Paschalidis, Citation2022; Saiyad et al., Citation2022). Of different feeder modes available, Intermediate Public Transport (IPT) modes serve as prominent sustainable access modes to main haul transit systems in big Indian cities with an extensively planned and organized transit network. Vukan R. Vuchic defined IPT (also termed as paratransit or unconventional/informal forms of public transport (Fouracre & Maunder, Citation1979; Kar, Sadhukhan, & Parida, Citation2022a)) as “a transportation service provided by an operator and available to all parties who meet the conditions of a contract for carriage (i.e. pay prescribed fares or rates), but which is adjustable in various degrees to the individual user’s desires” (Vuchic, Citation2007). Compared to formal transit systems, IPT offers greater flexibility in terms of routing and scheduling according to user needs (Vuchic, Citation2007). In the context of developing nations, IPT mode is typically capable of functioning as the primary mode in smaller or mid-sized cities and auxiliary or feeder mode in large metropolitan areas, supporting main haul transit systems like BRTS or metro (Kunhikrishnan & Srinivasan, Citation2018; Priye & Manoj, Citation2020a). However, users have associated dissatisfaction with various aspects of these modes such as passenger safety, fare structure, and information dissemination (Harding, Badami, Reynolds, & Kandlikar, Citation2016; Joewono & Kubota, Citation2007; Priye & Manoj, Citation2020a). Hence, service quality improvement of IPT access modes as per the users’ perceptions is essential for promoting and strengthening metro ridership. Interventions in such sustainable modes, in turn, align with the dimension of livability in terms of improved safety, accessibility, affordability, and environmental quality. Contrarily, very limited studies have focused on the service quality evaluation of such modes based on user perception. Thus, it is crucial to identify the important attributes affecting the service quality of IPT access modes according to users so that the transport planners and policymakers can devise suitable strategies to improve these attributes.
The current manuscript explains a user perception-based approach to prioritize and improve essential attributes determining service quality of IPT modes to access metro stations. The study is conducted with reference to metro users in Delhi, India. A tablet-based survey instrument was designed to collect the responses from users who reached the metro stations using IPT access modes toward the importance of selected IPT service quality attributes using a six-point Likert-type rating scale. Three widely used multi-attribute decision making techniques, Relative to an Identified Distribution Integral Transformation (RIDIT), Grey Relational Analysis (GRA) and Technique for Order Preference by Similarity to the Ideal Solution (TOPSIS) were used to analyze the data and obtain importance-based rankings of the selected IPT service attributes. Spearman rank-order correlation analysis was performed to verify any variations in attribute rankings derived from the three methods.
This manuscript contains six sections. The following section reviews the relevant literature to identify crucial IPT service quality attributes and choose the most appropriate attribute prioritization techniques for the present study. Section 3 briefly describes the methodology adopted in the present study. Section 4 depicts the results of the data analysis. Section 5 discusses the study outcomes and outlines some policy recommendations. Finally, Section 6 includes the concluding remarks with some potential future research avenues.
2. Literature review
This section consists of a review of literature related to IPT service quality arranged in the first sub-section to prepare a list of crucial attributes for the present study, followed by a review of suitable techniques for evaluating attributes in the second sub-section.
2.1. IPT service quality attributes
“Service Quality (SQ)” is a well-established measure (Parasuraman, Zeithaml, & Berry, Citation1988) dependent on multiple factors, which are further determined by several attributes (Echaniz, Dell’Olio, & Ibeas, Citation2018). It is essential to consider users’ perspectives for evaluating the service quality of any facility or product or service and identify and improve the factors or attributes that significantly influence service quality. Very limited studies focussed on the service quality of IPT operations in developing countries (Javid, Okamura, Nakamura, & Wang, Citation2013; Joewono & Kubota, Citation2005). Joewono and Kubota (Citation2007) attempted to reconnoiter and evaluate user satisfaction with the existing performance levels of IPT modes in Indonesia and anticipate its future use. The researchers highlighted the intervention areas which could significantly enhance the existing service quality offered by the IPT modes. Also, the authors underscored the influence of price on loyalty and perceptions of users toward IPT service. Contrarily, Tarigan, Susilo, and Joewono (Citation2014) recorded and analyzed the negative experiences and dissatisfaction of different groups of users pertaining to several IPT SQ aspects in Bandung city for improving the IPT service quality. Of the six identified negative experience cases associated with IPT service, “consistent departure time,” “consistent arrival time” and “security in vehicle” were found to be highly critical issues as perceived by the users. Additionally, a majority of the users showed dissatisfaction with several poorly performing shelter/stop and mode-related attributes (such as “condition,” “cleanliness,” “comfort,” “safety,” “security”), “route coverage,” “schedule punctuality” and “operator”s driving skill’. Phun, Kato, and Yai (Citation2018) also emphasized on improving safety aspects of IPT modes to ensure continued use by users. Chowdhury, Uddin, Datta, and Taraz (Citation2018) conducted a study to identify important SQ attributes influencing the IPT modes operating in Sylhet City. The authors considered users’ perspectives to understand their satisfaction with the existing service levels of these attributes and their expectations to improve the IPT services accordingly. “Vehicle fitness,” “cleanliness,” “safety” and “security,” “fare structure,” and “traveling comfort” were perceived as important features influencing the SQ of IPT modes. Javid, Hussain, and Anwaar (Citation2020) investigated the future intended use of Qingqi IPT mode in Lahore city by analyzing users’ perception on the existing service levels and developing structural equation models. Reduction of “discomfort due to overcrowding and music,” “dedicated planning and regulating authority to decide the tariff structure,” “operating routes and schedules,” “improved passenger safety and security” by keeping skilled and trained drivers for the service could have potential contributions toward the improvement of the existing performance levels of Qingqi IPT mode. The authors emphasized that an improved service quality could ensure future use of the IPT mode. Hence, this aforementioned review of literature assisted in the selection of IPT service quality attributes for the present study.
2.2. Attribute prioritization techniques
Generally, priority-based decisions involve multiple mutually correlated and conflicting criteria, complicating the decision-making process (Tsamboulas, Citation2007). Such a chaotic decision-making process is successfully addressed by a class of scientific analysis, namely, multi-attribute decision making (MADM) techniques. The existing literature identifies various MADM methods such as, Analytic Hierarchy Process (AHP) (Gutiérrez, De Vicente Oliva, & Romero-Ania, Citation2021; Moslem, Farooq, Ghorbanzadeh, & Blaschke, Citation2020), Analytic Network Process (ANP) (Liu, Lo, & Liou, Citation2020; Sayyadi & Awasthi, Citation2020), Best Worst Method (Ahmadi, Lo, Gupta, Kusi-Sarpong, & Liou, Citation2020; Vardin, Ansari, Khalilzadeh, Antucheviciene, & Bausys, Citation2021), VIKOR (Liang, Chen, Ye, Lin, & Li, Citation2021; Patil & Majumdar, Citation2021), Elimination and Choice Expressing Reality (ELECTRE) (Kalifa, Özdemir, Özkan, & Banar, Citation2022; Romero-Ania, Rivero Gutiérrez, & De Vicente Oliva, Citation2021), Grey Relational Analysis (GRA) (Kar et al., Citation2022a; Majumdar, Mitra, & Pareekh, Citation2020b; Roy & Basu, Citation2019; Sadhukhan, Banerjee, & Maitra, Citation2015; Wu, Citation2007), Preference Ranking Organization Method for Enrichment of Evaluations (PROMETHEE) (Ahmadi et al., Citation2020; Rogulj & Jajac, Citation2018), Relative to an Identified Distribution Integral Transformation (RIDIT) (Kar et al., Citation2022a, Kar, Sadhukhan, & Parida, Citation2022b; Majumdar et al., Citation2020b; Pitale, Sadhukhan, & Parida, Citation2023; Roy & Basu, Citation2019; Sadhukhan, Banerjee, & Maitra, Citation2018, Sadhukhan et al., Citation2015; Wu, Citation2007), Technique for Order Preference by Similarity to Ideal Solution (TOPSIS) (Kar et al., Citation2022a; Majumdar, Sahu, Patil, & Vendotti, Citation2021, Majumdar, Dissanayake, Rajput, Saw, & Sahu, Citation2020a; Roy & Basu, Citation2019; Sadhukhan et al., Citation2015) which establish priority of selected alternatives by assigning weights or scores and preparing a rank order. Even superior techniques in terms of fuzzy theory integrated with the above-mentioned methods have also been used to address the ambiguity, uncertainty in the decision-making process and better analyze the real-world problem situations. While techniques like AHP and ANP analyze expert opinions and determine criteria weights, the remaining techniques can analyze user perception data collected on a Likert scale to develop criteria scores. AHP may not be suitable because of the incapability to handle higher inconsistencies involved in large sized data, and rank reversal issues (Aires & Ferreira, Citation2018; Cheng & Li, Citation2002). The other attribute Prioritization techniques yield better outcomes for user perception data recorded at disaggregate levels as it is easier for users to fairly rate individual attributes than the overall facility or service (Deakin, Ferrell, Mason, & Thomas, Citation2002).
The present study uses three prominent MADM methods, namely, RIDIT, GRA, TOPSIS to prioritize IPT service quality attributes considering user perspectives. The rationale behind choosing the three above-mentioned methods is due to their associated advantages such as: being distribution-free (RIDIT and GRA), suitable for analyzing Likert-type ordinal data, can be used to evaluate incomplete, poor, uncertain, and unreliable data (Kuo, Yang, & Huang, Citation2008). Additionally, TOPSIS involves simpler scalar quantity estimations and shows good calculation efficiency (Hung & Chen, Citation2009).
3. Methodology
This section briefly outlines the approach adopted in the present study arranged in the following sub-sections. The first sub-section highlights the study context followed by description of the attribute selection and data collection process in the second sub-section. The third sub-section explains the preliminary analysis performed on the collected database.
3.1. Study context
The present research work has been demonstrated with reference to Delhi which is the capital of India. The Delhi metro rail network, consisting of twelve operational lines, 285 stations and spanning over a length of 389 km (Delhi Metro Rail Corporation (DMRC), Citation2020), plays a pivotal role in catering to the travel needs of the commuters in and around Delhi. However, the metro system suffers from insufficient network coverage. This is supplemented by the availability of various IPT modes in Delhi, which constitute a substantial share in facilitating access to the metro stations (Goel & Tiwari, Citation2014; Mandhani, Nayak, & Parida, Citation2020). Hence, the present study aims to employ a user perception-based approach to prioritize attributes affecting the service quality of IPT access modes to metro stations and suggest suitable policy interventions for improvement.
3.2. IPT service quality attribute selection and data collection
The present study uses a comprehensive set of 18 service attributes of IPT modes which was directly taken up from a recently published study by Kar et al. (Citation2022b), carried out by the same group of authors as the current paper, and briefly explained in . The attributes were finalized through an exhaustive review of related literature, frequency analysis and context relevance. A travel behavior survey instrument was designed on a survey application software platform “Go Survey” (https://www.gosurvey.in/) to record the importance ratings of the respondents for the selected attributes on a six-point Likert scale. A preliminary study (100 samples) was organized to validate the questionnaire in terms of accuracy, respondents’ comprehension ability, acceptability, and relevance to the study context and to train the surveyors and prepare them for the main survey. COVID-19 appropriate behavior was strictly followed throughout the survey. The survey respondents were primarily intercepted at the entrance/departure gates, platforms, and inside the metro. With due consent from the respondents, responses were recorded through face-to-face interviews using smart tablets. The survey respondents were initially questioned about the access modes used by them to reach the metro stations to establish their eligibility for the survey. Responses from users of all lines of Delhi metro were collected on weekdays and weekends. A simple random sampling-based data collection ensured an equal probability of choosing the respondents. The survey instrument captured the socio-economic and trip information in the first two segments, followed by user importance ratings with respect to the selected 18 service attributes in the last segment. Finally, 1121 responses on IPT service quality attributes were collected from the study area. However, 20 responses were detected as outliers using the inter-quartile range method and 1101 responses were considered for further analysis.
Table 1. List of IPT service quality attributes considered in the study (Kar et al., Citation2022b).
A frequency analysis of the user responses in terms of stated importance ratings of the selected attributes is illustrated in . Such an analysis provides useful insights on user needs and also on the importance of attributes from users’ perspectives. For instance, shows that a significant majority of respondents have rated attributes namely, “Riding Comfort” (81.5%), “Convenience” (80.8%), “Travel Incentives” (78.5%), “Travel Fare” (74.7%) to have extremely high importance which is the highest level on the importance rating scale. This implies users assign higher importance to the qualitative attributes along with fare which shows that these attributes highly influence the service quality offered by the IPT access modes. Further, a fair proportion of users have rated “Universal Design Considerations” (35.5%), “Safety” (30.4%), “Security” (30.3%), “Access Time” (29.2%) as highly important. Such analysis provides a preliminary idea to allocate resources toward the crucial attributes to improve IPT service quality.
3.3. Preliminary data analysis
Prior to any major analysis, the cleaned database undergoes an initial analysis to check the data adequacy, quality and confirm the nature of the data. In the present study, the initial data analysis comprises of three different analyses namely, descriptive analysis which represents the sample data statistics, normality test which checks if the sample data follow normal distribution, and reliability analysis using Cronbach’s alpha values to check the internal consistency.
3.3.1. Descriptive analysis
As per Krejcie and Morgan’s sample size requirements (Krejcie & Morgan, Citation1970), a size of 1101 user samples was found adequate for analysis. The descriptive statistics of data recorded in terms of socio-economic and travel information of the respondents are presented in . The survey included 56.22% male and 43.78% female respondents. Use of household private vehicle by the primary earner, ignorance or lack of confidence in driving, absence of driving license might be responsible behind a comparatively higher proportion of females using IPT modes than private modes to access metro stations. The respondents were primarily working, young individuals (18–40 years old) belonging to typical middle-class families (household income: 30,000 –75,000 Indian rupee/month). While 71.66% of the respondents were choice riders (owned private vehicles), 28.34% of the respondents were captive riders and solely depended on IPT modes to access the metro. The travel profiles indicate that the respondents essentially made work trips (49.03%) and social/personal purpose trips (35.40%). Other travel purposes were severely affected by the COVID-19 pandemic. A higher proportion of work trips is also explained by a higher percentage of respondents (54.22%) commuting frequently using IPT access modes. E-rickshaw (46.10%) and auto-rickshaw (33.90%) were the predominant IPT access modes preferred by the respondents. These were easily available near trip origins at cheaper prices than other IPT access modes.
3.3.2. Normality and reliability analysis
Generally, the data undergoes tests for normality prior to any type of data analysis as normal data is an essential requirement to perform parametric tests. One-sample Kolmogorov-Smirnov (K-S) and Shapiro-Wilk (S-W) tests checked the normality of the cleaned database in the present study (Mohd Razali & Bee Wah, Citation2011; Shapiro & Francia, Citation1972). The outcomes of the tests indicated that the K-S test statistics ranged between 0.157-0.491 while the S-W statistics ranged between 0.458-0.922. The p-values were significant at 95% confidence level indicating that the analyzed data did not follow normal distribution.
For Likert-type scale data, internal consistency reliability check by calculation and reporting of Cronbach’s alpha (α) values is mandatory (Gliem & Gliem, Citation2003). Cronbach’s alpha reliability test generates a unique estimate i.e. the mean of the reliability coefficient values obtained for all possible item combinations when divided into two half-tests. The alpha value depends on the mean inter-item correlations and number of items in the scale. It generally lies between 0 and 1. Alpha values nearer to 1 indicate higher internal consistency of the scale items. The internal consistency reliability of data was checked for user-stated importance ratings. The α value for importance rating data was found to be 0.74 which indicated acceptable internal consistency reliability.
3.4. Multi-Attribute decision making methods
This section presents in brief, the steps of implementation of the three MADM methods, RIDIT, GRA, TOPSIS employed in the present study.
3.4.1. RIDIT method
Step 1: Selection of a reference dataset (In case of Likert-type ordinal data, total survey responses = reference dataset)
Step 2: Calculation of frequency fj for each response category, where j = 1, 2, ……, n
Step 3: Computation of mid-point accumulated frequency Fj for each response category
(1)
(1)
(2)
(2)
Step 4: Calculation of RIDIT value Rj for each response category in reference data set
(3)
(3)
N = Total number of Likert scale survey responses, R = 0.5 for reference data set
Step 5: Computation of RIDITs (rij) and mean RIDITs (ρi) for each response category
(4)
(4)
πij: Frequency of category j for ith scale item; πi: Summation of frequencies of all categories of ith scale item
(5)
(5)
(6)
(6)
Step 6: Hypothesis testing using Kruskal-Wallis W
(7)
(7)
W follows χ2 distribution with (m-1) degrees of freedom.
3.4.2. GRA method
Step 1: Reference data series containing the most favored responses
(8)
(8)
Step 2: Generation of comparison data series
(9)
(9)
Step 3: Computation of Difference Data Series Δi
(10)
(10)
Step 4: Determination of Global Maximum Value Δmax and Minimum Value Δmin in Difference Data Series
(11)
(11)
Step 5: Transformation of Data points in Difference Data Series to a Gray Relation Coefficient
(12)
(12)
where
= A coefficient with value between 0 and 1, Default value = 0.5 (Kumar & Bhattacharya, Citation2017)
Step 6: Computation of Gray Relation for each Difference Data Series
(13)
(13)
3.4.3. TOPSIS method
Step 1: Formulation of a Decision Matrix
(14)
(14)
where A1,…, Aj: Available alternatives; B1,…, Bn: Criteria; fji: crisp value showing the relative performance rating of the alternative Aj for Bi criteria
Step 2: Normalization of the Decision Matrix R (= [rji])
(15)
(15)
Step 3: Calculation of Weighted Normalized Matrix
(16)
(16)
Step 4: Determination of Positive (S+) and Negative (S-) Ideal Solutions
(17)
(17)
Step 5: Estimation of Separation Measures from Positive (dj+) and Negative (dj−) Ideal Solutions
(using the n-dimensional Euclidean distance)
(18)
(18)
Step 6: Calculation of Closeness Index (Cj*) and Determination of Ranks
(19)
(19)
4. Prioritization of IPT service quality attributes
The prepared database was analyzed using RIDIT, GRA and TOPSIS to obtain importance-based priority rankings of attributes influencing the service quality of IPT access modes to metro stations, explained in subsequent sections.
4.1. Prioritization using RIDIT
Relative to an identified distribution integral transformation analysis is a distribution-free method, developed by Bross (Citation1958), favorable to statistically analyze Likert-type ordinal rating data and prioritize the attributes (Beder & Heim, Citation1990; Kar et al., Citation2022a; Pitale et al., Citation2023; Sadhukhan et al., Citation2018; Sermeus & Delesie, Citation1996; Wu, Citation2007). RIDIT analysis considers a reference population under study and adopts its relative distribution instead of any theoretical distribution. Additionally, it has fewer assumptions hence, easier calculations and interpretations. The importance ratings provided by the survey respondents toward the selected attributes were analyzed using the RIDIT method to obtain derived rankings and determine the priority attributes. The attribute importance-based prioritization outcomes are reported in . A comparison between the Kruskal-Wallis (W) statistic and the critical Chi-square value associated with specific degrees of freedom determined the statistical significance of the method. The Kruskal-Wallis (W) value for importance-based attribute prioritization is 27235.76, which is significantly greater than the critical Chi-square value for 17 degrees of freedom (χ218-1 = 27.587). This implies, the user responses toward the importance of IPT service quality attributes are statistically significant and different. The importance-based priority rankings of selected attributes are determined based on the RIDIT scores. The higher the RIDIT scores, the higher are the importance rankings associated with the attributes.
Table 2. Importance-based attribute prioritization using RIDIT.
Mostly, it is observed that the users perceive the qualitative attributes of IPT access modes to be more important than fare and travel time. It is evident from where the IPT access mode users have conferred greater importance to “Safety,” “Security,” “Universal Design Considerations,” “Accessibility in Bad Weather,” “Cleanliness” than “Travel Fare.” The RIDIT analysis prioritizes “Safety” attribute (ρi = 1.0538) followed by “Security” attribute (ρi = 1.0485) based on user stated importance. This can be explained by the fact that the predominantly used IPT modes (auto rickshaws and e-rickshaws) are highly susceptible to accidents while operating under mixed traffic conditions. The passengers’ safety and security is a matter of grave concern due to the unstable structural design, absence of closed sides and safety gadgets such as safety belts in the vehicles. The outcomes of RIDIT analysis reflect “Universal Design Considerations” (ρi = 1.0122) as another very important attribute which indicates the presence of facilities to make the IPT access modes universally accessible and suitably usable for travel by all types of user groups. The absence of such inclusive design justifies emphasis on the attribute in terms of importance with respect to service quality of IPT access modes and is well depicted from the user responses. The RIDIT analysis results indicate users’ desire for having universally inclusive IPT access modes.
Accessing and traveling in the IPT access modes becomes very difficult during bad weather conditions, especially for auto rickshaws, e-rickshaws, cycle rickshaws due to open sides. This may be responsible for users assigning higher levels of importance to the attribute “Accessibility in Bad Weather” (ρi = 0.9822). “Cleanliness” is perceived as the next important attribute in priority order (ρi = 0.9214). This is justified in the sense that the data have been collected during the pandemic when the national government is insisting to follow appropriate COVID-19 behavior. The guidelines fixed at the national level encourage social distancing along with frequent sanitation to maintain hygiene. Since, the IPT modes are public means of transport, the users are highly sensitive about the cleanliness maintained inside the IPT access modes. “Travel Fare” (ρi = 0.9134) is very important for the IPT access mode users. This is because there is no formal regulating authority which decides the fare structure rather, it is mostly fixed and regulated by informal unions. Hence, the IPT access mode users typically the captive riders seem to be more vulnerable and concerned about the changes in the fare structure. Users associate “Seat Availability” (ρi = 0.8996) attribute with moderate importance because the users have the freedom of choice to board an IPT mode depending upon the availability of seat in that mode. In case seat is not available, the users can choose to wait and board another such vehicle because these IPT access modes are readily available.
It may be noted that the users confer nearly same importance to “Comfort” (ρi = 0.7869) and “Convenience” (ρi = 0.7868) attributes in terms of service quality of the IPT access modes. “In-Vehicle Travel Time” (ρi = 0.7518) and “Frequency” (ρi = 0.7334) are perceived to be more important than the other components of total travel time namely, “Access Time” (ρi = 0.6835), “Egress Time” (ρi = 0.6785) and “Waiting Time” (ρi = 0.6742) by the users. This may be because the IPT access modes are easily available near trip origins at frequent time intervals during peak traffic hours hence, the access time and waiting time are not very high. Further, the egress time is also less because these vehicles drop the passengers at entrance gates of the metro stations. However, the in-vehicle travel time depends entirely on the enroute traffic conditions, driver behavior and on the condition of the vehicle. Hence, the in-vehicle travel time is perceived to be more important as it chiefly influences the total travel time. The users assign low importance to “Incentives” (ρi = 0.6309), “On-board Information” (ρi = 0.5952) and “Information at IPT Stop” (ρi = 0.5643). The travel information related attributes are less valued by the users because the responses were primarily collected from residents of Delhi who commute on a daily basis hence, they are acquainted with the travel modes and also have the required travel-related information.
4.2. Prioritization using GRA
Grey Relational Analysis (GRA), proposed by D. Julong (Julong, Citation1989), belongs to Grey system theory and is a successful MADM technique employed in various contexts (Kuo & Liang, Citation2011; Onyegiri & Oke, Citation2017; Priye & Manoj, Citation2020b; Sadhukhan et al., Citation2015). Besides being a distribution-free method, GRA effectively analyzes small sample- sized, unreliable Likert-scale type user perception data and produces precise outcomes (Kumar & Bhattacharya, Citation2017; Priye & Manoj, Citation2020b; Roy & Basu, Citation2019; Sadhukhan et al., Citation2015). The importance rankings of the attributes are derived from the average GRA scores. The higher the average GRA scores, the higher are the rankings of the attributes.
Similar to the outcomes of the RIDIT analysis technique, GRA method has also prioritized the IPT service attributes based on the importance ratings of respondents. The importance-based rankings of the selected IPT attributes from GRA are reported in . The GRA results show that “Safety” is the most important attribute influencing the service quality of IPT access modes followed by “Security.” Further, it is evident from the GRA-based attribute rankings that users confer higher importance to the qualitative attributes of IPT access modes than the associated travel fare and time, similarly as the findings of RIDIT method. The higher importance levels associated with attributes “Universal Design Considerations,” “Accessibility in Bad Weather” and “Cleanliness” explains the urgent need for enhancing the IPT access modes to make them universally inclusive, accessible and travel worthy during bad weather, and maintaining proper hygiene inside the modes. The priority rankings of the attributes in terms of importance derived from GRA method are consistent with the RIDIT method apart from a few deviations. These include priority ranking of “Seat Availability” over “Travel Fare” and “Convenience” over “Riding Comfort.” “Travel Incentives,” “On-board Information” and “Information at IPT Stop” are the bottom three attributes according to the importance rankings derived from GRA.
Table 3. Importance-based attribute importance using GRA.
4.3. Prioritization using TOPSIS
The technique for order preference by similarity to ideal solution (TOPSIS) is a popular MADM technique which uses a set of criteria and assigns rankings to the alternatives (Majumdar et al., Citation2021, Citation2020a; Roy & Basu, Citation2019; Sadhukhan et al., Citation2015). TOPSIS includes identifying the positive-ideal and negative-ideal solutions comprising of the best and worst possible values from the criteria, respectively. Subsequently, the alternatives are assigned ranks as per their closeness to and distance from the positive and negative-ideal solutions, respectively.
TOPSIS analysis was also performed on user importance responses toward the IPT attributes to identify the preference attributes. For the data analysis using TOPSIS, 18 IPT service quality attributes were assumed as alternatives to fit across the model. The importance rating scale (i.e. 0, 1, 2, 3, 4, 5) was considered as the selection criteria to show preference toward an attribute (Majumdar et al., Citation2021; Sadhukhan et al., Citation2015). Each of the six levels had equal weight and equal chances of being chosen. Analysis by TOPSIS assumed levels 4 and 5 positive (hence, maximized) and levels 0, 1, 2, 3 negative (hence, minimized) for the positive-ideal solution. On the contrary, levels 0, 1, 2, 3 were considered positive (hence, maximized) and levels 4 and 5 were considered negative (hence, minimized) for the negative-ideal solution. Consequently, the TOPSIS scores/closeness index (Cj*) values were computed using the positive and negative-ideal solutions. The calculated scores and the associated attribute ranks are reported in . While the scores (0, 1), higher TOPSIS scores indicated higher importance rankings associated with the attributes.
Table 4. User stated importance-based attribute prioritization using TOPSIS.
It is worth mentioning that TOPSIS-based attribute rankings are quite different from rankings derived from RIDIT and GRA. Among all the IPT service attributes, the respondents confer the highest importance (Cj* = 0.8944, rank-1) to “Seat Availability” attribute followed by “Cleanliness” (Cj* = 0.8919). Reduction in the carrying capacities of the IPT modes to reduce crowding and need for frequent sanitization due to the COVID-19 pandemic appropriately justify the above findings. “Security” (Cj* = 0.8880, rank-3), “Accessibility in Bad Weather” (Cj* = 0.8856, rank-4), “Safety”(Cj* = 0.8821, rank-5) are also perceived to be highly important IPT service quality attributes. Among the travel time attributes, “In-vehicle Travel Time” (Cj* = 0.8810, rank-6) is found to be more important compared to “Access Time” (Cj* = 0.8569, rank-8), “Egress Time” (Cj* = 0.8420, rank-12) and “Waiting Time”(Cj* = 0.8413, rank-13). This order of importance priority of is consistent across the three ranking methods. “Convenience” (Cj* = 0.8796, rank-7), “Riding Comfort” (Cj* = 0.8486, rank-9), “Universal Design Considerations” (Cj* = 0.8475, rank-10) and “Travel Fare” (Cj* = 0.854, rank-11) are considered as moderately important IPT service quality attributes by the users. Further, the results of TOPSIS analysis show “Luggage Space” (Cj* = 0.8068, rank-14), “Frequency” (Cj* = 0.8052, rank-15), “On-board Information” (Cj* = 0.5485, rank-16) and “Information at IPT Stop” (Cj* = 0.5057 rank-17) have received less importance while, “Travel Incentives” (Cj* = 0.4994, rank-18) is the least important attribute.
4.4. Comparative ranking of attributes
The present study employs three established yet methodologically different MADM techniques (RIDIT, GRA and TOPSIS) to determine the rankings of 18 IPT service quality attributes based on user-stated importance. Although the weights or scores associated with the selected service attributes may not be directly analogous across the three ranking methods owing to the differences in approaches, yet all the three methods are in consensus with respect to high importance toward “Safety,” “Security,” “Accessibility in Bad Weather,” “Cleanliness” attributes, moderate importance toward “Travel Fare,” “Riding Comfort,” “Convenience,” “In-Vehicle Travel Time” attributes, low importance toward “Frequency,” “Luggage Space,” “Egress Time,” “Waiting Time” attributes and the least importance toward “On-board Information,” “Information at IPT Stop” and “Travel Incentives” attributes. The importance-based rankings of attributes specified by RIDIT and GRA are consistent except for a few attributes. However, the TOPSIS-specified attribute ranks for importance vary from the other two ranking methods. Hence, Spearman’s rank-order correlation coefficient (ρ) is computed using EquationEquation (1)(1)
(1) to identify the presence of monotonicity in the relationship between the ranks derived from two ranking methods at a time (Kothari, Citation2004). Finally, the statistical significance of the correlation values determined selection of the suitable ranking method.
(1)
(1)
Here, di = difference between the paired ranks by any two methods and n = number of attributes. The ranks by RIDIT, GRA and TOPSIS were compared pairwise and the “ρ” values were reported in .
Table 5. Results of Spearman’s rank order correlation analysis.
The rank-order correlations between RIDIT, GRA and TOPSIS with respect to importance-based prioritization are found to be statistically significant at 99% confidence level. However, Spearman’s rank-order correlation coefficient values indicate a higher correlation between rankings of GRA method with RIDIT and TOPSIS methods. Hence, the present study prefers the prioritization of attributes specified by GRA over the other ranking methods for policy address although, the rankings derived from all the three methods are acceptable.
5. Discussion
The present study can be useful to the transport officials and local planning authorities in identifying the higher importance IPT service quality attributes which require immediate intervention based on attribute prioritization suggested by GRA method and endorsing suitable policies for overall augmentation of the IPT access modes. These policy recommendations are expected to exert significant positive influences on the improvement and operation of IPT as access modes to metro stations.
The research outcomes indicate “Safety” and “Security” are perceived as the most important attributes affecting the service quality of IPT access modes. Similar observation has been found in past studies which have identified “Safety” and “Security” as important attributes influencing the service quality of IPT modes (Harding et al., Citation2016; Javid et al., Citation2020; Joewono & Kubota, Citation2007; Priye & Manoj, Citation2020a, Citation2020b). The predominant IPT access modes, i.e. auto-rickshaws and E-rickshaws are associated with higher risks of safety and security considering the present traffic situations in India. Including seat belts, solid covers, railings and rear end protection into the vehicle design can have direct positive influences on the performance of auto-rickshaws and E-rickshaws by rendering these vehicles safer and more secured (Priye & Manoj, Citation2020b). Moreover, attributes namely “Universal Design Considerations,” “Accessibility in Bad Weather” also significantly influence IPT service quality. A previous study by Kar et al. (Citation2022b) records similar observation. Various facilities like support for boarding and alighting activities, provision of low floor design, wide entrances, ramps having gentle slopes, slip resistant surfaces, first-aid kit in the vehicle, signage can be introduced to make the IPT modes universally inclusive for all user groups including pregnant ladies, senior citizens and differently-abled persons. Further, rain covers can be provided to cover the IPT modes having open sides and facilitate accessibility during bad weather such as heavy rain or cold winds. “Cleanliness” is also perceived as an important IPT service quality attribute and need immediate interventions for improving the performance of IPT access modes. It may be mentioned that implementation of the above improvements will contribute toward an enhanced IPT access mode and metro ridership.
Besides the top-priority attributes, attention should also be given to attributes associated with moderate importance such as, “Travel Fare,” “Riding Comfort,” “Convenience,” “In-Vehicle Travel Time” and low importance like, “Frequency,” “Luggage Space,” “Egress Time,” “Waiting Time,” “On-board Information,” “Information at IPT Stop” and “Travel Incentives.” Improvements in the service levels of these attributes can also contribute toward enhancing the overall service quality of IPT access modes as identified in the literature (Chowdhury et al., Citation2018; Joewono & Kubota, Citation2007; Phun et al., Citation2018; Yang et al., Citation2015). Further, maintaining satisfactory performance levels of the above influencing attributes is also essential to make the overall IPT access mode services more attractive to the users. The suggested interventions can be used to enforce policy guidelines at city and country level for development of IPT access modes. These policies can be pivotal in providing better performing, appealing IPT access modes to users, and improving the accessibility to metro stations. Improved quality and easily available IPT access modes can also attract the choice riders to shift to metro rail, thereby, increasing metro ridership, reducing private vehicle use and ultimately, contributing toward a sustainable urban environment in developing countries like India.
Further, to facilitate the implementation of the MADM approach proposed in the present study for transport planners and field officials, several strategies can be employed to simplify the process and enhance usability. User-friendly software tools specifically tailored for transportation planning applications can be developed to provide simplified interface to officials which will systematically guide them through the decision-making process, from inputing data, defining criteria, until analyzing the results. Templates that represent common transportation planning scenarios can be provided to the officials to enable a quick adaptation and customization of the models to their specific context. Moreover, training programs, workshops can be organized where researchers and academicians from the domain can provide hands-on experience with real-world case studies, pilot projects to familiarize transport officials, practitioners and field experts with the effective implementation of MADM techniques.
6. Conclusion
The present study conducts a thorough investigation to identify and prioritize service quality attributes affecting IPT access modes to metro stations based on user-stated importance using Multi-Attribute Decision Making (MADM) techniques namely, RIDIT, GRA, and TOPSIS in an urban Indian context. These MADM techniques are being highly employed for data analysis in recent times because of their several advantages (already mentioned in literature review section of the manuscript). Hence, the utilization of these MADM techniques aligns impeccably with the latest literature aiming to enhance the analysis of service quality of IPT modes. These methods enable researchers to comprehensively evaluate and prioritize various attributes contributing to IPT service quality. Recent studies advocate for the adoption RIDIT, GRA, and TOPSIS methods due to their ability to accommodate multiple criteria simultaneously, reflecting the multifaceted nature of service quality of IPT. These methods facilitate a systematic approach to analyze IPT service quality by quantitatively assessing the relative importance of each criterion and aggregating diverse user preferences. This enables deriving objective insights into the performance of IPT modes and identifying areas for improvement. Moreover, these techniques are capable of capturing the complex interactions between various criteria thereby, enhancing the accuracy and robustness of service quality assessments. Thus, the findings of the present study can provide assistance to the transport planning authorities in informed decision-making.
Based on the research outcomes, the following final remarks can be drawn from the study. Firstly, the present study identifies a list of key service quality attributes which can be readily used to evaluate the existing service levels and devise a priority scheme to assist the transport planners and operators to improve the performance of IPT access modes. The qualitative and design related attributes of IPT access modes like “Safety,” “Security,” “Accessibility in Bad Weather,” “Universal Design Considerations” and “Cleanliness” have been recognized as areas of major concern and top priority in terms of improvement. The study also seeks interventions of some moderately important qualitative attributes of IPT namely, “Travel Fare,” “Riding Comfort,” “Convenience,” “In-Vehicle Travel Time” and attributes of low importance such as, “Frequency,” “Luggage Space,” “Egress Time,” “Waiting Time.” Secondly, the user-stated importance-based priority rankings of the IPT service quality attributes derived from three MADM techniques, RIDIT, GRA and TOPSIS are observed to show consistency and no statistically significant differences. However, the present study adopts the GRA-based prioritization of attributes as GRA shows significantly higher Spearman’s rank order correlation with RIDIT (ρ = 0.996) and TOPSIS (ρ = 0.802) at 99% confidence level. The outcomes and associated policy propositions of the present study are inclined toward one of the targets of the 11th sustainable development goal by United Nations Development Programme (UNDP) (Citation2015) which aims to “provide access to safe, affordable, accessible and sustainable transport systems for all, by improving road safety, notably by expanding public transport, with special attention to the needs of those in vulnerable situations, women, children, persons with disabilities and older persons by 2030” (United Nations Development Programme (UNDP), Citation2015). Further, the study findings aim at improvement in the service quality of sustainable transport modes like IPT thereby, establishing linkage with livability in terms of improved safety, accessibility, affordability, and environmental quality.
The present study has certain limitations. First, the present study is demonstrated in the context of Delhi, India. Hence, the collected database typically captures the travel behavior of commuters in India indicating that the study outcomes are case-specific. Second, the references used for finalizing attributes influencing service quality of IPT modes for evaluation in the present study are basically studies on Asiatic countries. However, the approach of the present study is capable of universal applicability to prioritize critical IPT service quality attributes in different contexts. As an immediate extension of the present work, gap analysis can be performed using the GRA-based scores of selected attributes to obtain a more logical priority order of attributes for allocating resources. The present work can be further extended to derive attribute prioritization according to user groups classified on the basis of various socioeconomic or travel cohorts and formulate user group-specific policies. Furthermore, the present study encourages to conduct advance research related to quantification of user benefits and impacts on mode choice behavior due to improvements in various priority attributes affecting the service quality of IPT access modes.
Disclosure statement
No potential conflict of interest was reported by the author(s).
Data availability statement
Some or all data, models, or code generated or used during the study are available from the corresponding author by request.
References
- Ahmadi, H. B., Lo, H. W., Gupta, H., Kusi-Sarpong, S., & Liou, J. J. H. (2020). An integrated model for selecting suppliers on the basis of sustainability innovation. Journal of Cleaner Production, 277, 1. https://doi.org/10.1016/j.jclepro.2020.123261
- Aires, R. F. D. F., & Ferreira, L. (2018). The rank reversal problem in multi-criteria decision making: A literature review. Pesquisa Operacional, 38(2), 331–27. https://doi.org/10.1590/0101-7438.2018.038.02.0331
- Beder, J. H., & Heim, R. C. (1990). On the use of RIDIT analysis. Psychometrika, 55(4), 603–616. https://doi.org/10.1007/BF02294665
- Bross, I. D. J. (1958). How to use ridit analysis. Biometrics, 14(1), 18–38. https://doi.org/10.2307/2527727
- Cheng, E. W. L., & Li, H. (2002). Construction partnering process and associated critical success factors: Quantitative investigation. Journal of Management in Engineering, 18(4), 194–202. https://doi.org/10.1061/(asce)0742-597x(2002)18:4(194)
- Chowdhury, T. D., Uddin, M. S., Datta, D., & Taraz, M. A. K. (2018). Identifying important features of paratransit modes in Sylhet City, Bangladesh: A case study based on travelers perception. Civil Engineering Journal, 4(4), 796–811. https://doi.org/10.28991/cej-0309134?
- Deakin, E., Ferrell, C., Mason, J., & Thomas, J. (2002). Policies and practices for cost-effective transit investments: Recent experiences in the United States. Transportation Research Record: Journal of the Transportation Research Board, 1799(1), 1–9. https://doi.org/10.3141/1799-01
- Delhi Metro Rail Corporation (DMRC). (2020). DMRC Annual Report 2019–2020.
- Echaniz, E., Dell’Olio, L., & Ibeas, Á. (2018). Modelling perceived quality for urban public transport systems using weighted variables and random parameters. Transport Policy, 67, 31–39. https://doi.org/10.1016/j.tranpol.2017.05.006
- Fouracre, P. R., & Maunder, D. A. C. (1979). A review of intermediate public transport in third world cities. In PTRC Summer Annual Meeting. University of Warwick.
- Gadepalli, R., Tiwari, G., & Bolia, N. (2020). Role of user’s socio-economic and travel characteristics in mode choice between city bus and informal transit services: Lessons from household surveys in Visakhapatnam, India. Journal of Transport Geography, 88, 102307. https://doi.org/10.1016/j.jtrangeo.2018.08.017
- Gliem, J. A., & Gliem, R. R. (2003). Calculating, interpreting, and reporting Cronbach’s alpha reliability coefficient for Likert-type scales. In Midwest Research-to-Practice Conference in Adult, Continuing, and Community Education, pp. 82–88. https://doi.org/10.1016/B978-0-444-88933-1.50023-4
- Goel, R., & Tiwari, G. (2014). Promoting low carbon transport in India: Case study of metro rails in Indian Cities. United Nations Environment Programme.
- Goel, R., & Tiwari, G. (2016). Access-egress and other travel characteristics of metro users in Delhi and its satellite cities. IATSS Research, 39(2), 164–172. https://doi.org/10.1016/j.iatssr.2015.10.001
- Gutiérrez, L. R., De Vicente Oliva, M. A., & Romero-Ania, A. (2021). Managing sustainable urban public transport systems: An AHP multicriteria decision model. Sustainability, 13(9), 4614. https://doi.org/10.3390/su13094614
- Harding, S. E., Badami, M. G., Reynolds, C. C. O., & Kandlikar, M. (2016). Auto-rickshaws in Indian cities: Public perceptions and operational realities. Transport Policy, 52, 143–152. https://doi.org/10.1016/j.tranpol.2016.07.013
- Hung, C. C., & Chen, L. H. (2009). A fuzzy TOPSIS decision making model with entropy weight under intuitionistic fuzzy environment. In Proceedings of the International MultiConference of Engineers and Computer Scientists, IMECS, Hong Kong, pp. 13–16.
- Javid, M. A., Hussain, S., & Anwaar, M. F. (2020). Passenger’s perceptions on prospects of Qingqi paratransit public transport service in Lahore. Iranian Journal of Science and Technology, Transactions of Civil Engineering, 44(1), 185–195. https://doi.org/10.1007/s40996-019-00273-z
- Javid, M. A., Okamura, T., Nakamura, F., & Wang, R. (2013). Comparison of commuters’ satisfaction and preferences with public transport: A case of wagon service in Lahore. Jordan Journal of Civil Engineering, 7, 461–472.
- Ji, Y., Fan, Y., Ermagun, A., Cao, X., Wang, W., & Das, K. (2017). Public bicycle as a feeder mode to rail transit in China: The role of gender, age, income, trip purpose, and bicycle theft experience. International Journal of Sustainable Transportation, 11(4), 308–317. https://doi.org/10.1080/15568318.2016.1253802
- Joewono, T. B., & Kubota, H. (2005). The characteristics of paratransit and non-motorized transport in Bandung, Indonesia. Journal of the Eastern Asia Society for Transportation Studies, 6, 262–277.
- Joewono, T. B., & Kubota, H. (2007). User satisfaction with paratransit in competition with motorization in Indonesia: Anticipation of future implications. Transportation, 34(3), 337–354. https://doi.org/10.1007/s11116-007-9119-7
- Julong, D. (1989). Introduction to grey system theory. The Journal of Grey System, 1, 1–24. https://doi.org/10.1007/978-3-642-16158-2_1
- Kalifa, M., Özdemir, A., Özkan, A., & Banar, M. (2022). Application of multi-criteria decision analysis including sustainable indicators for prioritization of public transport system. Integrated Environmental Assessment and Management, 18(1), 25–38. https://doi.org/10.1002/ieam.4486
- Kar, M., Sadhukhan, S., & Parida, M. (2022a). Measuring heterogeneity in perceived satisfaction of private vehicle users towards attributes affecting access to metro stations : A case study of Delhi. Case Studies on Transport Policy, 10(3), 1790–1803. https://doi.org/10.1016/j.cstp.2022.07.009
- Kar, M., Sadhukhan, S., & Parida, M. (2022b). Assessing commuters’ perceptions towards improvement of intermediate public transport as access modes to metro stations. Transport Policy, 129, 140–155. https://doi.org/10.1016/j.tranpol.2022.10.011
- Kothari, C. R. (2004). Research methodology: Methods and techniques. New Age International Publishers.
- Krejcie, R. V., & Morgan, D. W. (1970). Determining sample size for research activities. Educational and Psychological Measurement, 30(3), 607–610. https://doi.org/10.1177/001316447003000308
- Kumar, R. V., & Bhattacharya, S. (2017). Modeling consumer opinion using RIDIT and grey relational analysis. In A. Kumar, M. K. Dash, S. K. Trivedi, & T. K. Panda (Eds.), Handbook of research on intelligent techniques and modeling applications in marketing analytics (pp. 185–201). IGI Global.
- Kunhikrishnan, P., & Srinivasan, K. K. (2018). Investigating behavioral differences in the choice of distinct Intermediate Public Transport (IPT) modes for work trips in Chennai city. Transport Policy, 61, 111–122. https://doi.org/10.1016/j.tranpol.2017.10.006
- Kuo, M.-S., & Liang, G.-S. (2011). Combining VIKOR with GRA techniques to evaluate service quality of airports under fuzzy environment. Expert Systems with Applications, 38(3), 1304–1312. https://doi.org/10.1016/j.eswa.2010.07.003
- Kuo, Y., Yang, T., & Huang, G. W. (2008). The use of grey relational analysis in solving multiple attribute decision-making problems. Computers and Industrial Engineering, 55(1), 80–93. https://doi.org/10.1016/j.cie.2007.12.002
- Liang, X., Chen, T., Ye, M., Lin, H., & Li, Z. (2021). A hybrid fuzzy BWM-VIKOR MCDM to evaluate the service level of bike-sharing companies: A case study from Chengdu, China. Journal of Cleaner Production, 298, 126759. https://doi.org/10.1016/j.jclepro.2021.126759
- Liu, C., Bardaka, E., & Paschalidis, E. (2022). Sustainable transport choices in public transit access: Travel behavior differences between university students and other young adults. International Journal of Sustainable Transportation, 17(6), 679–695. https://doi.org/10.1080/15568318.2022.2084656
- Liu, P. C. Y., Lo, H. W., & Liou, J. J. H. (2020). A combination of DEMATEL and BWM-based ANP methods for exploring the green building rating system in Taiwan. Sustainability, 12(8), 3216. https://doi.org/10.3390/su12083216
- Majumdar, B. B., Dissanayake, D., Rajput, A. S., Saw, Y. Q., & Sahu, P. K. (2020a). Prioritizing metro service quality attributes to enhance commuter experience: TOPSIS ranking and importance satisfaction analysis methods. Transportation Research Record: Journal of the Transportation Research Board, 2674(6), 124–139. https://doi.org/10.1177/0361198120917972
- Majumdar, B. B., Mitra, S., & Pareekh, P. (2020b). On identification and prioritization of motivators and deterrents of bicycling. Transportation Letters, 12(9), 591–603. https://doi.org/10.1080/19427867.2019.1671042
- Majumdar, B. B., Sahu, P. K., Patil, M., & Vendotti, N. (2021). Pedestrian satisfaction-based methodology for prioritization of critical sidewalk and crosswalk attributes influencing walkability. Journal of Urban Planning and Development, 147(3), 1–16. https://doi.org/10.1061/(asce)up.1943-5444.0000718
- Mandhani, J., Nayak, J. K., & Parida, M. (2020). Interrelationships among service quality factors of metro Rail Transit System: An integrated Bayesian networks and PLS-SEM approach. Transportation Research Part A: Policy and Practice, 140, 320–336. https://doi.org/10.1016/j.tra.2020.08.014
- Ministry of Housing and Urban Affairs Government of India. (2021). Harmonised guidelines & standards for universal accessibility in India 2021.
- Ministry of Urban Development. (2014). National Urban Transport Policy. Govt. of India.
- Mohd Razali, N., & Bee Wah, Y. (2011). Power comparisons of Shapiro-Wilk, Kolmogorov-Smirnov, Lilliefors and Anderson-Darling tests. Journal of Statistical Modeling and Analytics, 2(1), 21–33.
- Moslem, S., Farooq, D., Ghorbanzadeh, O., & Blaschke, T. (2020). Application of the AHP-BWM model for evaluating driver behavior factors related to road safety: A case study for Budapest. Symmetry, 12(2), 243. https://doi.org/10.3390/sym12020243
- Onyegiri, I. E., & Oke, S. A. (2017). A grey relational analytical approach to safety performance assessment in an aviation industry of a developing country. Engineering and Applied Science Research, 44, 1–15. https://doi.org/10.14456/easr.2017.1
- Parasuraman, A., Zeithaml, V. A., & Berry, L. L. (1988). SERVQUAL: A multiple-item scale for measuring consumer perceptions of service quality. Journal of Retailing, 64, 12–40.
- Patil, M., & Majumdar, B. B. (2021). Prioritizing key attributes influencing electric two-wheeler usage: A multi criteria decision making (MCDM) approach – A case study of Hyderabad, India. Case Stud. Transp. Policy,.9(2), 913–929. https://doi.org/10.1016/j.cstp.2021.04.011
- Phun, V. K., Kato, H., & Yai, T. (2018). Traffic risk perception and behavioral intentions of paratransit users in Phnom Penh. Transportation Research Part F: Traffic Psychology and Behaviour, 55, 175–187. https://doi.org/10.1016/j.trf.2018.03.008
- Pitale, A. M., Sadhukhan, S., & Parida, M. (2023). RIDIT-ISA approach to investigate commuter perceptions of an existing regional transit system : A case study in the National Capital Region, India. Transportation Research Record. 2677(9), 450–463. https://doi.org/10.1177/03611981231159400
- Priye, S., & Manoj, M. (2020a). Passengers’ perceptions of safety in paratransit in the context of three-wheeled electric rickshaws in urban India. Safety Science. 124, 104591. https://doi.org/10.1016/j.ssci.2019.104591
- Priye, S., & Manoj, M. (2020b). Exploring usage patterns and safety perceptions of the users of electric three-wheeled paratransit in Patna, India. Case Stud. Transp. Policy,.8(1), 39–48. https://doi.org/10.1016/j.cstp.2020.01.001
- Rahman, F., Das, T., Hadiuzzaman, M., & Hossain, S. (2016). Perceived service quality of paratransit in developing countries : A structural equation approach. Transportation Research Part A: Policy and Practice, 93, 23–38. https://doi.org/10.1016/j.tra.2016.08.008
- Rogulj, K., & Jajac, N. (2018). Achieving a construction barrier–free environment: Decision support to policy selection. Journal of Management in Engineering, 34(4), 1–18. https://doi.org/10.1061/(asce)me.1943-5479.0000618
- Romero-Ania, A., Rivero Gutiérrez, L., & De Vicente Oliva, M. A. (2021). Multiple criteria decision analysis of sustainable urban public transport systems. Mathematics, 9(16), 1844. https://doi.org/10.3390/math9161844
- Roy, S., & Basu, D. (2019). Ranking urban catchment areas according to service condition of walk environment. Journal of Transportation Engineering, Part A: Systems, 145(4), 04019005. https://doi.org/10.1061/JTEPBS.0000225
- Sadhukhan, S., Banerjee, U. K., & Maitra, B. (2015). Commuters’ perception towards transfer facility attributes in and around metro stations: Experience in Kolkata. Journal of Urban Planning and Development, 141(4), 04014038. https://doi.org/10.1061/(asce)up.1943-5444.0000243
- Sadhukhan, S., Banerjee, U. K., & Maitra, B. (2018). Preference heterogeneity towards the importance of transfer facility attributes at metro stations in Kolkata. Travel Behaviour and Society, 12, 72–83. https://doi.org/10.1016/j.tbs.2017.05.001
- Saiyad, G., Srivastava, M., & Rathwa, D. (2022). Exploring determinants of feeder mode choice behavior using artificial neural network: Evidences from Delhi metro. Physica A: Statistical Mechanics and Its Applications, 598, 127363. https://doi.org/10.1016/j.physa.2022.127363
- Sayyadi, R., & Awasthi, A. (2020). An integrated approach based on system dynamics and ANP for evaluating sustainable transportation policies. International Journal of Systems Science: Operations & Logistics, 7(2), 182–191. https://doi.org/10.1080/23302674.2018.1554168
- Sermeus, W., & Delesie, L. (1996). RIDIT analysis on ordinal data. Western Journal of Nursing Research, 18(3), 351–359. https://doi.org/10.1177/019394599601800309
- Shapiro, S. S., & Francia, R. S. (1972). An approximate analysis of variance test for normality. Journal of the American Statistical Association, 67(337), 215–216. https://doi.org/10.2307/2284728
- Tarigan, A. K. M., Susilo, Y. O., & Joewono, T. B. (2014). Segmentation of paratransit users based on service quality and travel behaviour in Bandung, Indonesia. Transportation Planning and Technology, 37(2), 200–218. https://doi.org/10.1080/03081060.2013.870792
- Tsamboulas, D. A. (2007). A tool for prioritizing multinational transport infrastructure investments. Transport Policy, 14(1), 11–26. https://doi.org/10.1016/j.tranpol.2006.06.001
- United Nations Development Programme (UNDP). (2015). Goal 11 targets [WWW Document]. https://www.undp.org/sustainable-development-goals#sustainable-cities-and-communities
- Vardin, A. N., Ansari, R., Khalilzadeh, M., Antucheviciene, J., & Bausys, R. (2021). An integrated decision support model based on bwm and fuzzy-vikor techniques for contractor selection in construction projects. Sustainability, 13(12), 6933. https://doi.org/10.3390/su13126933
- Vuchic, V. R. (2007). Urban transit and systems technology. John Wiley & Sons, Inc. All. https://doi.org/10.1201/9781420041217-64
- Wen, C. H., Wang, W. C., & Fu, C. (2012). Latent class nested logit model for analyzing high-speed rail access mode choice. Transportation Research Part E: Logistics and Transportation Review, 48(2), 545–554. https://doi.org/10.1016/j.tre.2011.09.002
- Wu, C.-H. (2007). On the application of grey relational analysis and RIDIT analysis to Likert scale surveys. International Mathematical Forum, 2, 675–687. https://doi.org/10.12988/imf.2007.07059
- Yang, M., Zhao, J., Wang, W., Liu, Z., & Li, Z. (2015). metro commuters’ satisfaction in multi-type access and egress transferring groups. Transportation Research Part D: Transport and Environment, 34, 179–194. https://doi.org/10.1016/j.trd.2014.11.004