Abstract
Aim: Evaluate the association of race/ethnicity and socioeconomic position (SEP) on emergency department (ED) visits for patients with hepatocellular carcinoma (HCC), which may reflect access to and quality of cancer care. Materials & methods: Patients with HCC identified from a commercial multi-payer claims database between 2015 and 2018 were matched to near-neighborhood social determinants of health (SDOH) and stratified by race/ethnicity and SEP (proxied by annual household income). Analyses evaluated the effect of race/ethnicity and SEP on ED utilization, adjusting for SDOH, demographic and clinical characteristics using multivariable regression methods. Results: A total of 22,247 patients were included. Black and Hispanic patients had 43 and 18% higher ED utilization than White patients at higher-income levels (p < 0.01); these differences were nonsignificant at lower-income. Regardless of income level, Asian patients had lower ED utilization. Conclusion: Further research on the intersectionality between race/ethnicity, SEP and other SDOH may guide structural-level interventions to address health inequities.
Plain language summary
Health disparities among racial/ethnic minorities have been observed in patients with hepatocellular carcinoma (HCC). We conducted a real-world retrospective insurance claims study of more than 22,200 adult patients with HCC between 2015 and 2018. We evaluated the association of race/ethnicity and socioeconomic position (measured by income level) with emergency department (ED) utilization. Our study consisted of 69% White, 14% Black, 7% Hispanic, 6% Asian and 4% other patient populations. Black and Hispanic patients had the highest number of ED visits, followed by White and Asian patients. Compared with White patients, ED visits were 27% higher for Black, 17% higher for Hispanic and 36% lower for Asian patients. Compared with low income, middle income was associated with 4% more and high income with 6% less ED use, regardless of race/ethnicity. At higher income levels, Black and Hispanic but not Asian patients demonstrated higher ED use than White patients. These findings suggest that improved socioeconomic position of Black and Hispanic patients may not provide as protective an effect on health outcomes, potentially due to structural health inequities.
Tweetable abstract
Emergency department use for liver cancer care is higher in Black and Hispanic patients compared with White patients regardless of socioeconomic status, suggesting ongoing structural racial health disparities despite improved socioeconomic position.
Despite advances in diagnosis and treatment of hepatocellular carcinoma (HCC), disparities in care access, utilization and health outcomes persist.
This study evaluated the association of race/ethnicity and socioeconomic position on incidence of emergency department (ED) visits, which may reflect access to and quality of cancer care.
Compared with White patients, ED visits were higher for Black (IRR: 1.27) and Hispanic patients (IRR: 1.17) and lower for Asian patients (IRR: 0.67).
When stratified by income, Black and Hispanic patients had higher ED utilization than White patients at higher income levels, but these differences became insignificant at lower-income levels.
Asian patients had lower ED utilization regardless of income.
In summary, racial/ethnicity differences in ED utilization were more pronounced at higher income levels, suggesting racial disparities may differ by socioeconomic position.
Further research is needed to understand the intersectionality between race/ethnicity, socioeconomic position, and other social determinants of health, to guide structural-level interventions to address health inequities.
Liver cancer remains the third leading cause of cancer death worldwide, accounting for an estimated 906,000 new cases and 830,000 deaths in 2020 [Citation1]. Hepatocellular carcinoma (HCC) is the most common form of liver cancer, comprising up to 85% of cases, and is the fastest rising cause of cancer-related deaths in the USA [Citation2]. Racial and ethnic disparities in HCC have been observed across the cancer care continuum, from surveillance and diagnosis to treatment and mortality. Lower rates of HCC surveillance in patients diagnosed with cirrhosis have been observed in Black patients compared with non-Hispanic White and Hispanic patients [Citation3]. Compared with non-Hispanic Whites, age-adjusted HCC incidence rates are disproportionally higher in the non-Hispanic Black and Hispanic populations; Black and Hispanic patients are also more likely to have HCC diagnosed at a later disease stage [Citation4–6]. Furthermore, Black and Hispanic patients are less likely to receive curative treatments compared with White and Asian patients [Citation6–9], and exhibit shorter survival [Citation6,Citation8,Citation10].
Racial and ethnic disparities in cancer care are further impacted by social determinants of health (SDOH), which encompass factors shaped by socioeconomic position (SEP), such as income and economic stability, education, access to care and community environment [Citation11]. Income has commonly been considered a surrogate marker for SEP across studies investigating cancer disparities and has been correlated with health outcomes in HCC [Citation12–15]. Patients with HCC from low-income households are more likely to be diagnosed at an advanced stage and have higher mortality rates, possibly due to difficulties with access to care and suboptimal monitoring and treatment [Citation16]. Although race/ethnicity and SEP may both influence health outcomes independently in HCC, further understanding is needed regarding the interplay between these factors.
Few studies have evaluated disparities in healthcare resource utilization in HCC, such as incidence of emergency department (ED) visits. Hospitalization rates for patients with HCC have notably increased over the past decade, and the primary source of nearly half of admissions is through the ED [Citation17]. Studies have shown that patients with cancer are increasingly likely to visit an ED at some point over the course of their care [Citation18,Citation19]. In that setting, patients may experience long wait times, may be at risk for exposure to infection, and may ultimately receive treatment from staff less experienced with cancer-related problems [Citation20,Citation21]. In addition, ED visits may also be more costly for oncology patients than non-oncology patients, further increasing the cost of care for patients who already face financial toxicities associated with their routine cancer treatments [Citation20–24].
Evaluation of ED visit utilization in the context of cancer care has been identified as an important quality metric. Notably, the Centers for Medicare and Medicaid Services (CMS) recently added measure OP-35 to its Hospital Outpatient Quality Reporting Program [Citation25], which encourages providers to determine ways to reduce ED visits and hospital admissions through improved processes in outpatient care. The evaluation of ED visit utilization may also help identify potential disparities in quality of cancer care across different patient populations [Citation26–28]. Racial and ethnic disparities in ED utilization have been noted in patients with other cancer types, such as breast cancer, even after adjusting for socioeconomic and clinical factors [Citation29]. To our knowledge, no studies have examined how race/ethnicity and income may impact rates of ED utilization in patients with HCC. We performed a retrospective analysis in patients with HCC using a multi-payer claims database linked to nine-digit ZIP code-level SDOH data to examine the interaction between race/ethnicity and SEP, and its potential differential impact on ED visits in patients with HCC. We hypothesized that racial difference in ED utilization may differ across income levels, independent of other SDOH.
Materials & methods
Study design & data sources
This retrospective claims-based cohort study used 100% Medicare Fee-For-Service (FFS) data (parts A, B and D) and Medicare Advantage, commercial and managed Medicaid claims from Inovalon's Medical Outcomes Research for Effectiveness and Economics Registry (MORE2 Registry®) [Citation30]. The payer-sourced data constitutes a warehouse of healthcare administrative claims from more than 150 US health plans operating in all 50 states. The FFS and payer-sourced data include complete enrollment and claims histories across all healthcare settings and service types.
Data on income and other SDOH were sourced from Acxiom's InfoBase® Geo data, which is an aggregation of data drawn from multiple individual and household databases at the nine-digit ZIP code level [Citation31]. This produces roughly 30 million discrete data points, which represent approximately five households on average. To maintain compliance with HIPAA regulations, our claims dataset does not provide direct access to patient-level SDOH data. Therefore, the highest degree of granularity we are able to achieve is neighborhood-level characteristics imputed down to the individual patient. Research has demonstrated the close association between the social and economic characteristics of individuals' neighborhoods and their health behaviors and outcomes [Citation32,Citation33]. Patients in claims were assigned the SDOH characteristics of their corresponding ZIP code via a secure tokenization process based on their home address from enrollment records.
Study population
Patients newly diagnosed with primary HCC between 1 January 2015 and 30 September 2018 were included. Initial HCC diagnosis (index date) was defined by the presence of at least two claims on different dates with ICD-9-CM diagnosis codes of 155.0 or 155.2, or ICD-10-CM codes of C22.0, C22.8 or C22.9. Codes 155.2, C22.8 and C22.9 are nonspecific liver cancer codes which are sometimes used in practice as placeholders until a more specific diagnosis can be made. We included these codes to establish the index date but required there be a primary HCC-specific code – 155.0 or C22.0 – at some point for the patient to be included in the study. Patients were required to be ≥18 years of age and continuously enrolled in their health plan with medical and pharmacy coverage for at least 12 months prior to index (baseline period) and at least 3 months after index (follow-up period). Patients were excluded if they had any cancer diagnosis during baseline or had missing or unknown values for race/ethnicity or any of the SDOH variables defined below.
Study variables
Demographic variables measured at baseline included age at index (<65, 65–74, 75–79 and ≥80), sex, census region (Northeast, Midwest, South, West, Unknown) and payer type (Medicare FFS, Medicare Advantage, commercial, managed Medicaid). Race/ethnicity was categorized in the insurance enrollment records as Asian, Black, Hispanic, North American Native, White and Other. Clinical characteristics included Charlson Comorbidity Index scores (CCI: 0–2, 3–4, 5–6, 7+) [Citation34,Citation35] as well as indicators for the presence of a set of HCC etiology and related conditions including alcohol, drug or substance abuse; hepatitis B or C; cirrhosis; nonalcoholic fatty liver disease; ascites; and hepatic encephalopathy.
Type of HCC treatments were identified using ICD-10-PCS procedure, Healthcare Common Procedure Coding System (HCPCS), Common Procedural Terminology (CPT) and National Drug Codes (NDC) and categorized as curative, noncurative or no treatment [Citation36,Citation37]. Treatment was considered curative if patients received surgery (transplantation, resection), ablation, stereotactic body radiation therapy (SBRT), or other potential downstaging therapies when followed by surgery. Patients whose treatment regimens involved systemic therapy and/or embolization not followed by surgery were considered to have received noncurative treatment.
Income was measured as a neighborhood-level variable reflecting household income relative to the federal poverty level (FPL) and categorized as low (<200% FPL), middle (201–300% FPL) and high (>300% FPL). Utilizing income relative to FPL rather than median household income adjusts for both regional cost-of-living differences and the number of people in the household. Other SDOH variables included education level (less than high school diploma, high school graduate or some college and undergraduate or higher degree); rurality (urban, suburban, large rural town and small town/isolated rural) [Citation38]; primary care provider shortage area (full county shortage, partial county shortage, no shortage) [Citation39]; percentage of population speaking English not well or not at all; percentage living alone; and percentage who do not own a vehicle. The primary study outcome was incidence rate ratios (IRR) of ED visits, measured from index until health plan disenrollment or end of the study period and reported as the number of visits per 1000 patients per month (P1000PPM).
Statistical analysis
Descriptive statistics including means, standard deviations (SD), and medians for continuous data, and frequencies and percentages for categorical data are reported for baseline patient characteristics, type of HCC treatment, SDOH variables, overall and stratified by race/ethnicity categories. Generalized linear regression modeling (GLM) analyses were used to assess the relationship between ED visits and race/ethnicity in all patients, adjusting for SDOH, demographic and clinical characteristics, and using a negative binomial distribution and log link function. An offset term was used to normalize the data for differential follow-up period durations. To further assess the intersectionality between race/ethnicity and income, GLM analyses were performed in subgroups of patients stratified by income categories (low, middle and high). Covariates in all models included the demographic, clinical and other SDOH variables listed in the study variables section above. Regression results were summarized by IRR and 95% CI. All analyses were performed using SAS software, version 9.4 for Unix (SAS Institute, Inc., Cary, NC).
Results
Patient demographic characteristics
Overall, 22,247 patients with HCC were identified based on study selection criteria (). Of these patients, 69.4% were White, 14.2% were Black, 6.5% were Hispanic, 5.8% were Asian and 4.1% ‘other’ race/ethnicities. Median follow-up time was 408 days (mean 512, SD 362). reports patient demographic, baseline clinical and SDOH characteristics by race/ethnicity. Black and Hispanic patients were more likely than Asian and White patients to be diagnosed at a younger age (<65). The sex distribution was comparable among groups (males: Hispanic, 60.5%; Asian, 61.0%; White, 63.1%; Black, 63.4%). Geographically, compared with White patients, Black patients were more likely to live in the Northeast (31.8 vs 23.6%) and less likely to live in the West (8.3 vs 13.4%). Hispanic and Asian patients were more likely to live in the South compared with Whites (50.8% and 50.7 vs 36.0%). More than a quarter (26.1%) of Hispanics patients resided in the West, while they were under-represented (6.8%) in the Midwest. The distribution of payer type also varied by race/ethnicity. Black, Asian and Hispanic patients were more likely to be in managed Medicaid than White patients (19.2, 15.9%, and 35.2 vs 7.5%).
Figure 1. Patient selection.
†Patients who qualified for cohort-based solely on ICD-9-CM diagnosis code 155.2 or ICD-10-CM diagnosis code C22.9 (malignant neoplasm of liver, not specified as primary or secondary) were excluded.

Table 1. Baseline patient demographics, clinical characteristics and social determinants of health by race/ethnicityTable Footnote†.
Clinical characteristics & receipt of treatment
Racial/ethnic differences were observed with regards to baseline medical comorbidities and follow-up treatment type (). Approximately one-quarter of Black, Hispanic and White patients had CCI scores of seven or higher versus 13.1% of Asian patients. Asian patients were less likely to have had a pre-index diagnosis of alcohol, drug or substance abuse (4.6% for Asians vs 19.5, 23.4 and 24.5% for White, Hispanic and Black patients). Hepatitis B and C were more common in Black and Asian patients (46.4 and 43.6%) than in Hispanic and White patients (30.8 and 28.8%). Cirrhosis was observed in over half of the patients overall but was most common in Hispanic patients (65.0%) and least common among Asian patients (38.9%). During the follow-up period, 38.5% of patients received no treatment and nearly half (46.2%) received noncurative treatment rather than curative treatment (15.3%). Treatment type was similar among racial and ethnic groups.
Social determinants of health
Racial/ethnic differences were also observed across SDOH (). There was a higher proportion of Black and Hispanic patients in the low-income category (39.7 and 37.1%) compared with Asian and White patients (12.1 and 14.0%). A higher percentage of Asian and White patients (42.1 and 37.4%) lived in a neighborhood with education level of college degree or higher compared with Hispanic and Black patients (27.7 and 30.9%). Hispanic and Asian patients were more likely to live in areas with limited English proficiency (13.6% and 11.6 vs 4.9% overall). Black patients were more likely to live in areas with lower rates of vehicle ownership (19.6 vs 10.6% overall). White patients were more likely to live in a county with a sufficient supply of primary care providers compared with Hispanic patients (7.8 vs 2.4%).
Emergency department visits
Differences in ED visits were observed based on race/ethnicity and income (). Overall, Black and Hispanic patients exhibited higher rates of ED visits compared with White patients. ED utilization rates generally declined as income levels increased. In multivariate analysis, after adjusting for other covariates (i.e., demographic, clinical and SDOH variables), racial/ethnic differences in ED utilization remained significant ( & Supplementary Table 1). Compared with White patients, the incidence of ED visits in Black and Hispanic patients was 27% (IRR: 1.27; p < 0.05) and 17% (IRR: 1.17; p < 0.05) higher, respectively; Asian patients had 36% (IRR: 0.64; p < 0.05) lower rates of ED visits. ED utilization of ‘Other’ race patients did not significantly differ from White patients. There was a trend toward lower ED visits for patients in the middle- and high-income groups compared with low-income patients, although the differences were not statistically significant.
Figure 2. Number of emergency department visits (unadjusted) per 1000 patients per month, by race/ethnicity and income level (±95% CI).
ED: Emergency department.

Figure 3. Rate of emergency department visits (adjusted): incidence rate ratios for ED visits for race/ethnicity (reference group: White) and for income level (reference group: low income).
ED: Emergency department; IRR: Incidence rate ratio.
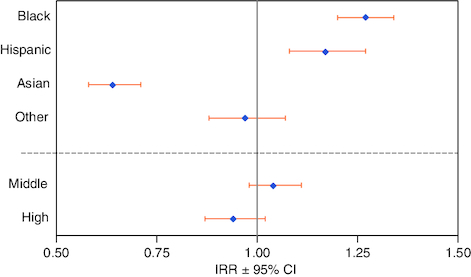
Other demographic, clinical and SDOH characteristics were also associated with differences in ED visits (Supplementary Table 1). Medicare Advantage and managed Medicaid patients had higher rates of ED visits (IRRs 1.23 and 1.27; p < 0.05) while commercially insured patients had lower rates (IRR: 0.56; p < 0.05) than those in FFS Medicare. ED utilization was higher for female patients (IRR: 1.08; p < 0.05) and patients under age 65 (IRRs 0.63, 0.58 and 0.62; p < 0.05 for ages 65–74, 75–79 and 80+, respectively). Patients receiving noncurative treatment were more likely to utilize the ED (IRR: 1.07; p < 0.05), and those receiving curative treatment were less likely to utilize the ED (IRR: 0.73; p < 0.05) than patients receiving no treatment. Increasing comorbidity burden was associated with higher rates of ED visits (IRRs of 1.02, 1.21, 1.34 and 1.77; p < 0.05 for patients with CCI scores of 1–2, 3–4, 5–6 and 7 or more vs 0).
To further understand the interplay between race/ethnicity and SEP and to assess whether racial differences in ED utilization differed across income levels, three separate models were run for patients in the low-, middle- and high-income groups ( & Supplementary Table 2). At middle- and high-income levels, Black and Hispanic patients exhibited significantly higher rates of ED visits compared with White patients (reference group) (middle-income: Black IRR: 1.36; p < 0.0001; Hispanic IRR: 1.30; p < 0.0001. High-income: Black: 1.43; p < 0.0001; Hispanic IRR: 1.18; p < 0.05). In the low-income group, ED visit rates for Black and Hispanic patients did not differ significantly from White patients (reference) (Black IRR: 1.03; p = 0.58; Hispanic IRR: 1.04; p = 0.61). Asian patients exhibited lower ED utilization rates compared with White patients at all levels of income (high IRR: 0.63; p < 0.0001; middle IRR: 0.68; p < 0.0001; low IRR: 0.66; p < 0.001).
Discussion
High rates of ED utilization among patients with cancer may be associated with less specialized cancer-related care, increased risk of exposure to nosocomial infections and increased financial toxicity to patients [Citation20,Citation21,Citation24]. ED visit utilization has therefore become a quality-of-care metric, with quality improvement initiatives currently being implemented to reduce potentially avoidable ED visits. Furthermore, evaluating ED utilization rates across diverse racial/ethnic and socioeconomic patient populations may uncover disparities in care and identify potentially vulnerable populations.
In this large, national cohort of patients diagnosed with HCC, our findings suggest that racial/ethnic disparities in ED visits may differ by income, our proxy measure of SEP. Black and Hispanic patients demonstrated overall higher rates of ED utilization compared with White patients. However, these differences in the incidence of ED visits were more pronounced at higher income levels, particularly for Black and Hispanic patients, after controlling for relevant demographic, clinical, and SDOH variables. Moreover, Asian patients exhibited the lowest levels of ED utilization regardless of income. To our knowledge, our analysis is the first real-world study to examine these racial differences in the context of ED visits for patients with HCC, and to evaluate whether such differences may be further affected by SEP. These findings may ultimately provide insight into healthcare disparities in patients with HCC.
The differential effect of income on racial/ethnic disparities in ED utilization observed in our findings may be explained by the ‘diminishing returns hypothesis’. This theory postulates that racial/ethnic minorities, such as Blacks and Hispanics, who advance to higher levels of socioeconomic position may not derive the same health advantages as Whites with similar SEP. Potential reasons for this disparity include structural racism, interpersonal discrimination and racism-related stress [Citation13,Citation40,Citation41]. Results consistent with minorities' diminished returns have been reported for health-related measures, such as self-reported health and chronic conditions, heart disease, body mass index and depressive symptoms. In a cross-sectional study using data from the National Survey of American Life, Assari et al. found that self-reported chronic medical conditions declined more among White respondents than among African–Americans with rising income, suggesting that the protective effect of income was larger for Whites than African–Americans [Citation42]. Similar patterns of diminished returns with other measures of SEP, including educational advancement, have also been noted with subjective health, heart disease and hospitalization risk [Citation41,Citation43–45]. Of note, we did not observe differences in ED utilization at lower SEP levels. This may be due to the multitude of challenges faced by individuals of lower SEP, including employment, food acquisition and adequate housing, such that these socioeconomic difficulties may impact health status to a larger extent [Citation46]. Nonetheless, with increasing evidence of diminished returns despite SEP advancement in Blacks and Hispanics, efforts targeting specific SEP variables, such as income or education alone, may be insufficient to eliminate existing disparities in health outcomes for minorities. Further understanding of this complex relationship between race/ethnicity and SEP and their differential impact on healthcare outcomes is strongly needed to develop interventions to reduce disparities and promote health equity for all patients.
Compared with Black and Hispanic patients, Asian patients in our study demonstrated lower rates of ED visits overall, and ED utilization did not differ by SEP. Similar findings have been observed in other population-based studies. In a study using linked data from the California Cancer Registry and California Office Statewide Health Planning and Development, Falcone et al. observed higher likelihood of an ED visit within 90 days of surgical treatment for women with breast cancer in Blacks and Hispanics compared with Whites, but a lower likelihood in Asian/Pacific Islanders [Citation29]. Similarly, Asian and Pacific Islander patients have been observed to have lower ED visit rates for other diseases, such as hypertension, diabetes, stroke and heart failure compared with other racial/ethnic groups [Citation47]. Moreover, differences may exist among different Asian subgroups. In a study investigating racial/ethnic differences in treatment and survival for patients with HCC, Stewart and colleagues noted that Laotian/Hmong and Cambodians were less likely to receive surgical treatment and had higher cause-specific mortality compared with Korean, Chinese, Vietnamese and Japanese patients [Citation9]. These findings thus highlight the heterogeneity of the Asian population and suggest that disaggregation of Asians by subgroups will be needed in future research to identify and better understand health disparities in specific at-risk Asian groups.
We also observed associations of ED utilization with other clinical and sociodemographic variables. Compared with patients who received no treatment for HCC, those who received noncurative treatments including locoregional therapy and systemic therapy were more likely to utilize the ED, possibly due to having more advanced stage of disease and associated complications; on the other hand, those who received curative treatments were less likely to utilize the ED. Higher CCI scores were also associated with increased ED utilization, which likely reflects an increase in complex care needs, including emergency care, as the burden of comorbidities rises. Compared with FFS Medicare patients, patients under public managed care coverage (Medicare and/or Medicaid) were more likely to use the ED, whereas commercially insured patients were less likely. Finally, although the effect size of regression coefficients for the SDOH variables was small, their directionality was associated with expected findings. Living alone, lower levels of education, and living in more rural areas were associated with a higher likelihood of ED visits. Poor English language proficiency was associated with fewer ED visits; this may be due to the lower likelihood of seeking care due to language barriers, a finding that has been observed in other studies [Citation48,Citation49].
Limitations
Although our study uses a large, diverse multi-payer database, we note several limitations that are inherent to retrospective claims databases. First, medical claims do not capture clinically relevant details which may impact healthcare utilization. Missing information on variables such as disease stage, tumor characteristics, liver function, and response to treatment may lead to misclassification and unmeasured confounding. Other potential confounding factors that may impact ED utilization include distance to and general accessibility of emergency rooms. Second, income and other SDOH measures were aggregated at the nine-digit ZIP code level. Although nine-digit ZIP code is granular, these SDOH measures remain aggregations across multiple individuals who reside in that neighborhood. Thus, individual characteristics may vary, and this variation may not be well captured. Third, patients in the study cohort were predominantly Medicare FFS beneficiaries. Although this analysis accounted for all patients covered by Medicare FFS, it should be noted that the MORE2 registry, the other component of the database, cannot be assumed to be representative for patients covered by other payers such as the Department of Veterans Affairs (VA's) healthcare system or for uninsured patients.
Additional findings of this study should be interpreted considering potential biases. First, age and payer type were highly correlated in this population. Patients with Medicaid in this study cohort were predominantly under age 65, whereas most HCC patients covered by Medicare Advantage and FFS were over age 65. In this study, patients under age 65 were found to have higher rates of ED utilization than older age groups. Additional subset analyses performed (not reported here) suggested that the age effect was likely driven by the differential effect of payer types on outcomes. Second, although we normalized ED utilization as per 1000 patients per month to account for variable follow-up periods, all patients were required to have at least 3 months of follow-up to capture outcomes. Patients who died within the first 3 months of diagnosis were excluded from the study, resulting in potential immortal time bias. However, it is unclear whether racial/ethnic minorities or patients with low SEP were more likely to be subject to such immortal bias. Lastly, it is likely that some patients had ED visits due to treatment-related complications. Without reviewing the full medical record to better understand the nature and reason for ED visits, it is difficult to draw conclusions regarding quality of cancer care in relation to ED visit utilization.
Conclusion
In summary, our study highlights that racial/ethnic disparities in ED utilization in patients with HCC may differ based on SEP. Advancement in SEP, namely higher income status, may not provide as protective an effect in health outcomes for Black and Hispanic patients as they do for White patients, resulting in ‘diminished returns’. These findings underscore the need to further understand the interplay between race/ethnicity and SEP when evaluating disparities in health outcomes and resource utilization, and draws attention to the potential structural and environmental barriers that may disproportionately impact certain races and ethnicities as they ascend in SEP. Future research should further investigate this intersectionality between race/ethnicity and other SDOH to guide structural-level interventions to address these disparities to improve health outcomes for all patients.
Author contributions
All authors contributed to the conception and design of the research; S Kim, KM Kilgore, R Tan contributed to the acquisition and analysis of the data; all authors contributed to the interpretation of the data; R Tan, KM Kilgore drafted the manuscript; all authors critically revised the manuscript, agree to be fully accountable for ensuring the integrity and accuracy of the work, and read and approved the final manuscript.
Financial disclosure
This study was funded by Genentech, Inc. R Tan and S Hernandez are employees of Genentech, Inc. C Teigland, S Kim and KM Kilgore are (or were) employees of Inovalon, Inc. who were paid consultants to Genentech for the conduct of the study. The authors have no other relevant affiliations or financial involvement with any organization or entity with a financial interest in or financial conflict with the subject matter or materials discussed in the manuscript apart from those disclosed.
Writing disclosure
C Cohen, senior medical writer employed by Inovalon, provided medical writing and editorial support.
Ethical disclosure
This retrospective study involved only secondary analysis of existing, de-identified datasets, and therefore does not constitute human subjects research as defined at 45 CRF 46.102.
Supplementary Tables S1-S2
Download Zip (36.2 KB)Competing interests disclosure
The authors have no competing interests or relevant affiliations with any organization or entity with the subject matter or materials discussed in the manuscript. This includes employment, consultancies, stock ownership or options and expert testimony.
Additional information
Funding
References
- Sung H, Ferlay J, Siegel RL et al. Global Cancer Statistics 2020: GLOBOCAN Estimates of Incidence and Mortality Worldwide for 36 Cancers in 185 Countries. CA Cancer J. Clin. 71(3), 209–249 (2021).
- Siegel RL, Miller KD, Jemal A. Cancer statistics, 2020. CA Cancer J. Clin. 70(1), 7–30 (2020).
- Davila JA, Morgan RO, Richardson PA, Du XL, McGlynn KA, El-Serag HB. Use of surveillance for hepatocellular carcinoma among patients with cirrhosis in the United States. Hepatology 52(1), 132–141 (2010).
- El-Serag HB, Rudolph KL. Hepatocellular carcinoma: epidemiology and molecular carcinogenesis. Gastroenterology 132(7), 2557–2576 (2007).
- McGlynn KA, Petrick JL, El-Serag HB. Epidemiology of hepatocellular carcinoma. Hepatology 73(Suppl. 1), 4–13 (2021).
- Rich NE, Hester C, Odewole M et al. Racial and ethnic differences in presentation and outcomes of hepatocellular carcinoma. Clin. Gastroenterol. Hepatol. 17(3), 551–559; e1 (2019).
- Kim AK, Singal AG. Health disparities in diagnosis and treatment of hepatocellular carcinoma. Clin. Liver Dis. (Hoboken) 4(6), 143–145 (2015).
- Wagle NS, Park S, Washburn D et al. Racial, ethnic, and socioeconomic disparities in curative treatment receipt and survival in hepatocellular carcinoma. Hepatol. Commun. 6(5), 1186–1197 (2022).
- Stewart SL, Kwong SL, Bowlus CL et al. Racial/ethnic disparities in hepatocellular carcinoma treatment and survival in California, 1988–2012. World J. Gastroenterol. 22(38), 8584–8595 (2016).
- Xu L, Kim Y, Spolverato G, Gani F, Pawlik TM. Racial disparities in treatment and survival of patients with hepatocellular carcinoma in the United States. Hepatobiliary Surg. Nutr. 5(1), 43–52 (2016).
- American Medical Association and Association of American Medical Colleges. Advancing health equity: guide on language, Narrative and Concepts (2021). www.ama-assn.org/about/ama-center-health-equity/advancing-health-equity-guide-language-narrative-and-concepts-0 ( Accessed 11/03/2023).
- National Academies of Sciences, Engineering, and Medicine. Accounting for social risk factors in Medicare payment: Identifying social risk factors. The National Academies Press, Washington, DC (2016). Available at: https://nap.nationalacademies.org/catalog/21858/accounting-for-social-risk-factors-in-medicare-payment-identifying-social ( Accessed 11/03/2023).
- Nuru-Jeter AM, Michaels EK, Thomas MD, Reeves AN, Thorpe RJ Jr, LaVeist TA. Relative roles of race versus socioeconomic position in studies of health inequalities: a matter of interpretation. Annu. Rev. Public Health 39, 169–188 (2018).
- Kaur M, Joshu CE, Visvanathan K, Connor AE. Trends in breast cancer incidence rates by race/ethnicity: patterns by stage, socioeconomic position, and geography in the United States, 1999–2017. Cancer 128(5), 1015–1023 (2022).
- Iyer HS, Gomez SL, Chen JT, Trinh QD, Rebbeck TR. Trends in mortality among Black and White men with prostate cancer in Massachusetts and Pennsylvania: race and neighborhood socioeconomic position. Cancer 127(14), 2525–2534 (2021).
- Wong RJ, Kim D, Ahmed A, Singal AK. Patients with hepatocellular carcinoma from more rural and lower-income households have more advanced tumor stage at diagnosis and significantly higher mortality. Cancer 127(1), 45–55 (2021).
- Jinjuvadia R, Salami A, Lenhart A, Jinjuvadia K, Liangpunsakul S, Salgia R. Hepatocellular carcinoma: a decade of hospitalizations and financial burden in the United States. Am. J. Med. Sci. 354(4), 362–369 (2017).
- Cronin KA, Lake AJ, Scott S et al. Annual report to the nation on the status of cancer, Part I: national cancer statistics. Cancer 124(13), 2785–2800 (2018).
- Yang Z, Yang R, Min JK et al. Oncologic emergencies in a cancer center emergency department and in general emergency departments countywide and nationwide. PLOS ONE 13(2), e0191658 (2018).
- Mayer DK, Travers D, Wyss A et al. Why do patients with cancer visit emergency departments? Results of a 2008 population study in North Carolina. J. Clin. Oncol. 29(19), 2683–2688 (2011).
- Vandyk AD, Harrison MB, Macartney G et al. Emergency department visits for symptoms experienced by oncology patients: a systematic review. Support. Care Cancer 20(8), 1589–1599 (2012).
- Panattoni L, Fedorenka C, Greenwood-Hickman M et al. Characterizing potentially preventable cancer- and chronic disease-related emergency department use in the year after treatment initiation: a regional study. J. Oncol. Pract. 14(3), e176–e185 (2018).
- Lash RS, Bell JF, Bold RG et al. Emergency department use in recently diagnosed cancer patients in California. J. Community Support Oncol. 15(2), 95–102 (2017).
- Alishahi Tabriz A, Turner K, Hong YR et al. Trends and characteristics of potentially preventable emergency department visits among patients with cancer in the US. JAMA Netw. Open 6(1), e2250423 (2023).
- Centers for Medicare & Medicaid Services. Hospital Outpatient Quality Reporting Program. www.cms.gov/medicare/quality/initiatives/hospital-quality-initiative/hospital-outpatient-quality-reporting-program ( Accessed 11/3/2023).
- Handley NR, Schuchter LM, Bekelman JE. Best practices for reducing unplanned acute care for patients with cancer. J. Oncol. Pract. 14(5), 306–313 (2018).
- Earle CC, Park ER, Lai B, Weeks JC, Ayanian JZ, Block S. Identifying potential indicators of the quality of end-of-life cancer care from administrative data. J. Clin. Oncol. 21(6), 1133–1138 (2003).
- Gallaway MS, Idaikkadar N, Tai E et al. Emergency department visits among people with cancer: frequency, symptoms, and characteristics. J. Am. Coll. Emerg. Physicians Open 2(3), e12438 (2021).
- Falcone M, Liu L, Farias A et al. Evidence for racial/ethnic disparities in emergency department visits following breast cancer surgery among women in California: a population-based study. Breast Cancer Res. Treat. 187(3), 831–841 (2021).
- MORE2 Registry® Overview. www.inovalon.com/resource/more2-overview/ ( Accessed 3/10/2022).
- Acxiom Corporation. ZIP+4 InfoBase® Geo Files: Demographic, Financial and Property, Sept 2019 release; Market Indices ACS, Feb 2019 release (2020). www.acxiom.com ( Accessed 3/10/2022).
- Kilgore K, McClellan M, Teigland C, Pulungan Z. Using aggregate data to proxy individual-level characteristics in health services research: nine-digit zipcode vs. census block group. Value Health 21(May 1), S218–S219 (2018).
- Danos D, Leonardi C, Gilliland A et al. Increased risk of hepatocellular carcinoma associated with neighborhood concentrated disadvantage. Front. Oncol. 8, 375 (2018).
- Deyo RA, Cherkin DC, Ciol MA. Adapting a clinical comorbidity index for use with ICD-9-CM administrative databases. J. Clin. Epidemiol. 45(6), 613–619 (1992).
- Quan H, Sundararajan V, Halfon P et al. Coding algorithms for defining comorbidities in ICD-9-CM and ICD-10 administrative data. Med. Care 43(11), 1130–1139 (2005).
- Brar G, Greten TF, Graubard BI et al. Hepatocellular carcinoma survival by etiology: a SEER-Medicare database analysis. Hepatol. Commun. 4(10), 1541–1551 (2020).
- Sobotka LA, Hinton A, Conteh LF. Insurance status impacts treatment for hepatocellular carcinoma. Ann. Hepatol. 18(3), 461–465 (2019).
- US Dept. of Agriculture Economic Research Service. Rural-Urban Commuting Areas. www.ers.usda.gov/data-products/rural-urban-commuting-area-codes ( Accessed 11/3/2023).
- U.S. Department of Health and Human Services. User Documentation for the County Area Health Resources File (AHRF) 2020–2021 Release. Washington DC: U.S. Department of Health and Human Services, Health Resources and Services Administration, Bureau of Health Workforce, National Center for Health Workforce Analysis. https://data.hrsa.gov/topics/health-workforce/ahrf ( Accessed 11/03/2023).
- Farmer MM, Ferraro KF. Are racial disparities in health conditional on socioeconomic status?. Soc. Sci. Med. 60(1), 191–204 (2005).
- Assari S. Blacks' diminished health returns of educational attainment: health and retirement study. J. Med. Res. Innov. 4(2), e000212 (2020).
- Assari S. The benefits of higher income in protecting against chronic medical conditions are smaller for African Americans than Whites. Healthcare (Basel) 6(1), 2 (2018).
- Assari S. Blacks' diminished return of education attainment on subjective health; mediating effect of income. Brain Sci. 8(9), 176 (2018).
- Assari S, Cobb S, Saqib M, Bazargan M. Diminished returns of educational attainment on heart disease among Black Americans. Open Cardiovasc. Med. J. 14, 5–12 (2020).
- Assari S, Bazargan M. Minorities' diminished returns of educational attainment on hospitalization risk: National Health Interview Survey (NHIS). Hosp. Pract. Res. 4(3), 86–91 (2019).
- Winkleby MA, Cubbin C. Influence of individual and neighbourhood socioeconomic status on mortality among black, Mexican-American, and white women and men in the United States. J. Epidemiol. Community Health 57(6), 444–452 (2003).
- Ochieng N, Cubanski J, Neuman T, Artiga S, Damico A. Racial and ethnic health inequities and Medicare. Available at: www.kff.org/report-section/racial-and-ethnic-health-inequities-and-medicare-access-to-care-and-service-utilization/ ( Accessed 11/03/2023).
- Qureshi MM, Romesser PB, Jalisi S et al. The influence of limited English proficiency on outcome in patients treated with radiotherapy for head and neck cancer. Patient Educ. Couns. 97(2), 276–282 (2014).
- Pandey M, Maina RG, Amoyaw J et al. Impacts of English language proficiency on healthcare access, use, and outcomes among immigrants: a qualitative study. BMC Health Serv. Res. 21(1), 741 (2021).