Figures & data
Table 1. Materials’ thermal and physical properties.
Figure 3. (a-g): VS (a)
(b) AE performance, (c) MSE measures,(d) STs performance (e) EHs performance, (f) fitting curve, (g) performance of the Regression.

Figure 4. (a-g): VS (a)
(b) AE performance, (c) MSE measures,(d) STs performance (e) EHs performance, (f) fitting curve, (g) performance of the Regression.

Figure 5. (a-g): VS (a)
(b) AE performance, (c) MSE measures,(d) STs performance (e) EHs performance, (f) fitting curve, (g) performance of the Regression.
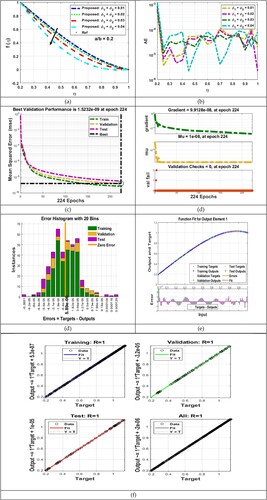
Figure 6. (a-g): VS (a)
(b) AE performance, (c) MSE measures,(d) STs performance (e) EHs performance, (f) fitting curve, (g) performance of the Regression.
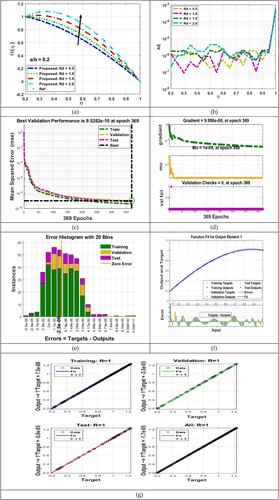
Figure 7. (a-g): VS (a)
(b) AE performance, (c) MSE measures,(d) STs performance (e) EHs performance, (f) fitting curve, (g) performance of the Regression.

Table 2. Outcomes of LMS-NNA for different scenarios of NCM-HMTMNFF-NNA.
Table 3. The skin friction outputs on the variations of the embedded parameters.
Table 4. The heat transfer rate versus .
Table 5. The parameters values range as per stability analysis.
Table 6. Based on the common parameter of gap between two cylinders the current work is compared with (Gouran et al., Citation2022).
Data availability statement
Data will be available on a reasonable request.