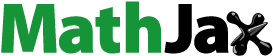
ABSTRACT
Purpose: The study evaluates new approach of digital extension services for the long-term adoption of digital extension technologies. We propose an indirect adoption approach to address following research questions. What socioeconomic factors influence rice farmers’ decision to prefer one business profile over another? Which business profile is most preferred and likely to be adopted by rice farmers? What are the attributes and socioeconomic characteristics required to create the best business profile?
Design/Methodology/Approach: Ten business profiles were tested with a sample size of 1440 farmers. Using the RiceAdvice as case study, we used choice experiment and the alternative-specific mixed logit model to determine most preferred business profile and analyze its determinants.
Findings: The preferred business profile was predicted by gender, age, education level, rice production experience, technology knowledge, contact with extension agents, rice farm size, and household income. 49.4% of farmers selected cash payment after harvest at 9.70 USD/hectare for more than two seasons-contract as first adoption approach. Cash payment after harvest at 14.50 USD/hectare for one season-contract was chosen by 44.7% of farmers as the second option.
Practical implications: Results highlight the ideal business profile, which considers all levels of education, with 14.50 USD/hectare/season as optimum price for cash payment after harvest and no access to credit.
Theoretical implications: The study expands the applicability of new adoption approach combined with econometric approach in the context of digital extension service adoption.
Originality/Value: This study revealed an indirect approach to the long-term adoption of digital extension technologies as most suitable for small holder farmers’ adoption.
1. Introduction
1.1. Overview of digital extension technologies
Agricultural extension services play an essential role in guiding farmers to improve the efficiency and effectiveness of their production by transferring new technologies through training, visits, or farm school (Van Campenhout, Spielman, and Lecoutere Citation2021). An effective agricultural extension service improves farmers’ technical habits, such as fertilizer use, and leads to more efficient decision-making and better outcomes for farmers (profit, income, food security, etc.). However, the traditional approach of government extension services (Sylla et al. Citation2019; Wuepper, Roleff, and Finger Citation2021) and external support provided by development partners such as NGOs and other international institutions, on the other hand, have proven their limits and must be updated to address today’s challenges. This is especially true in Sub-Saharan Africa, where public agricultural extension frequently fails to adequately serve farmers due to financial insecurity and a high reliance on government or donor funding, which is unreliable and leads to the discontinuation of many promising solutions. The traditional agricultural extension approach was criticized for its limited action based on systemic advice delivery, including the role of intermediation (Leeuwis and Aarts Citation2011; Munthali et al. Citation2022). Furthermore, the traditional approach’s insufficient performance in collaborating with value chain actors in problem-solving situations and providing effective market and credit information was highlighted (Karpouzoglou et al. Citation2016; Klerkx and Rose Citation2020).
The rise of digital extension technologies presents an opportunity to boost agricultural output, strengthen the value chain and contribute to food security (Gow et al. Citation2020; Rodríguez et al. Citation2021). Digital extension technology is a tool to better provide agricultural recommendations to farmers through digital applications. Any digital tool or technology used in the management of decision-making processes in agriculture or along the value chain are referred to as digital extension technologies (Gow et al. Citation2020; Klerkx, Jakku, and Labarthe Citation2022; McCampbell et al. Citation2021). It is arguably the best solution to climate variability, providing relevant information and advice for effective decision-making (Coggins et al. Citation2022). Extension digitalization is expected to improve farmers’ technical skills, address socio-economic challenges, improve food traceability, and reduce environmental impact (Balafoutis et al. Citation2017; Dawkins Citation2016; Klerkx, Jakku, and Labarthe Citation2022). Digital extension technologies aim to reduce inefficiencies coming from grossly simplified recommendations from traditional extension, thereby raising the productivity and profitability from adopting improved inputs (Aminou Arouna et al. Citation2020). Digital extension technologies may also help to reach large number of beneficiaries. The literature on digital extension focused on technology development and technical performance, with less emphasis on policy and innovative adoption approaches to ensure the technology's long-term adoption (Wolfert et al. Citation2017). Although the literature has demonstrated the potential impact of digital extension services, farmers must also see how the technology adds value to their activities and fits their needs before we can expect a long-term adoption (Amoussouhoui et al. Citation2022). The digital extension service (refers to the use of Information and Technology (IT) systems such as mobile applications or devices to provide extension service to farmers) can help farmers overcome the abovementioned limitations and improve their performance. The significance of digital extension technologies is reflected in the numerous investments and projects made in the last decade (Oliveira et al. Citation2020). However, the direct adoption of digital extension technologies by farmers, especially smallholder farmers, is still a challenge when considering the barriers to the adoption of digital technologies. According to Ayim et al. (Citation2022) and Barakabitze et al. (Citation2015), the main challenges to smallholder farmers’ adoption of digital extension technologies are poor infrastructure, a lack of ICT knowledge, financial capability, ineffective ICT policies, and inefficiencies in agricultural institutions. The authors argued that most African countries’ infrastructure development is still deficient, with poor agricultural development policies. Ferrari et al. (Citation2022) classified digital agricultural technology adoption barriers as socio-cultural, technical, economic, environmental, and regulatory-institutional. As a result of these barriers, smallholder farmers adopt digital extension technologies at a low rate (Coggins et al. Citation2022). Given the requirements for the direct use of digital technologies and the barriers mentioned above, a new mechanism for adoption and dissemination must be developed in collaboration with smallholder farmers (Shang et al. Citation2021). Such an approach will aid in addressing various factors influencing the proposed technology's adoption.
1.2. Case study of digital extension application: RiceAdvice
The Consultative Group for International Agricultural Research (CGIAR) center AfricaRice developed a digital extension technology called RiceAdvice to improve the performance of rice farmers. RiceAdvice is a free Android application, a digital advisory application for science-based crop management, and a decision support tool that provides rice farmers with personalized farm management advice. RiceAdvice generates recommendations based on field characteristics to increase farmer efficiency. RiceAdvice's recommendations include a nutrient management plan, an appropriate production plan, and a production calendar. The application is free and available to Africa Rice's twenty-seven member countries. Studies conducted by Arouna et al. (Citation2020), Zossou et al. (Citation2020), and Cotter et al. (Citation2020) revealed: (1) many rice farmers agreed to use the application, (2) to adopt the application or pay for services provided using the application, and (3) adoption of the application has a positive impact on rice yield and food security.
Nonetheless, due to several barriers, direct adoption of the application by smallholder farmers remains a topic of debate. As evidence, the Competitive African Rice Initiative (CARI) has taken over the application in 2015 and established a semi-commercial initiative in collaboration with rice miller companies since its development. Using the application, trained extension agents (working for the rice millers’ private company) were to provide personalized extension service to rice farmers. CARI and the Rice millers then covered the service fee. However, because it is a project, the initiative ended in 2020. Through this initiative and other scaling, about over 100,000 recommendations from RiceAdvice had been generated for use by farmers by the end of 2020. The main barriers to direct adoption of digital technologies by smallholder farmers in African countries, especially in Sub-Sahara-Africa, are a lack of or insufficient internet access, a lack of financial resources and proper equipment or infrastructure (mobile phone, internet, and smartphone) (Kieti et al. Citation2022; Oliveira et al. Citation2020; Porciello et al. Citation2022; Smidt Citation2021) and a lack of knowledge on how to use the technology (Owusu, Yankson, and Frimpong Citation2017). Given the aforementioned adoption barriers, new approaches are required to facilitate and ensure the long-term adoption of digital extension technologies.
Given these obstacles, this study proposes an indirect adoption approach based on a paid extension service provision via the application. This approach considers local conditions to overcome the barriers and increase farmer adoption (Birner, Daum, and Pray Citation2021; Zossou et al. Citation2020). The indirect adoption approach entails a business framework (Amoussouhoui et al. Citation2022) that proposes and provides farmers with quality extension services in exchange for a fee. Therefore, the approach is expected to result in broader and more sustainable adoption since it will remove two major constraints: farmers’ limited knowledge of direct digital technology use and limited access to adequate infrastructure or devices. It should be noted that this adoption approach could also provide them with a solution to their financial problem. In this approach, farmers are not expected to adopt digital extension technology directly but rather to adopt a business profile or a proposed service. However, given that smallholder farmers are already experiencing liquidity issues along the supply chain and have limited access to financial institutions, the challenge will be to find the best service fee for both farmers and service providers, which is why an ex-ante evaluation of the approach is required.
As a result, an ex-ante evaluation of the proposed businesses is required to select the most appropriate business profile, make necessary corrections before implementation, and thus achieve a higher adoption rate. In this study, a business profile refers to a set of services, also known as attributes, offered to farmers as a package. It includes the service price, payment method, type of contract, and other services that may be useful to the farmer. It is referred to as a ‘Business profile’ because it is a pay-for-service approach that can be used by both private investors looking to invest in agribusiness and institutions looking to design new extension policies. This study proposed ten theoretical business profiles for rice farmers based on preliminary information from farmers and actors. The research questions addressed by this study are as follows:
- What socioeconomic factors influence rice farmers’ decision to pursue one business profile over another?
- Given the characteristics of the proposed extension services, which business profile is most preferred and likely to be adopted by rice farmers?
- What are the attributes and socioeconomic characteristics required to create the best business profile?
The ex-ante assessment of the theoretical business profiles was conducted using a choice experiment approach in this study. Information was provided to identify the appropriate and promising business profile for the application’s long-term adoption. The study proposes a business approach with applicability to overcome the constraints and barriers (e.g. limited access to technology infrastructures such as smartphones, internet, knowledge of the use of new technologies, and lower education level) of smallholder farmers’ direct adoption of the application.
2. Methodology
2.1. Study area, sampling method, and data collection
The research was carried out in Nigeria’s Jigawa State. It is located in the country's northwest and encompasses 27 Local Government Areas (LGA). The agricultural sector served as the state's economic backbone in the previous decade, with rice as the primary crop. Therefore, this state was chosen because it is a major rice hub in Nigeria. Furthermore, the CARI initiative was completed in this state, allowing us to train extension agents for the experiment. As a result, we have extension agents in this state who are familiar with the RiceAdvice application and rice partners (e.g. millers and intermediaries) who already do business with rice farmers.
The data for this study were collected as part of the first phase of a Randomized Control Trial experiment on the RiceAdvice application. Data on rice production, food security, and sociodemographic and economic characteristics were collected using a questionnaire. The questionnaire was created with the CSPro software and then transferred to Android tablets for data collection. The data was collected in June 2021. The LGAs, villages, and households to be surveyed were chosen using a multi-stage stratified sampling method. Nine LGAs were chosen on purpose based on the number of rice farmers and the LGA where the rice millers work. In addition, the security issue of terrorist threats in northern Nigeria was also considered. The security issues were discussed with the extension agents, and high-risk areas were excluded. The sample size was determined using power calculation. Based on the financial means, 20 rice farmers per village in 72 villages were surveyed, giving a sample size of 1440 rice farmers. The number of villages surveyed was determined proportionally and based on the number of villages in each LGA. The villages and farmers in each LGA were then chosen at random.
2.2. Experimental design of the business profiles
2.2.1. Choice experiment approach
The choice experiment is a widely used method to determine users’ preferences (Ellison et al. Citation2016; Krah et al. Citation2019; Martey et al. Citation2021; Waldman et al. Citation2017). It is based on Lancaster's theory (Lancaster Citation1966), which assumes that individual choice is based on utility derived from the attributes of the proposed product. Following this theory, the attributes in this study represent the characteristics that define each proposed business profile. The choice experiment is the most straightforward and robust approach and is also called the Discrete Choice Experiment (DCE) (Weber Citation2019). Unlike conjoint analysis (CA), which is based on conjoint measurement (which is not a behavioral theory), DCE is based on the theory of choice behavior proposed by Thurstone (Thurstone Citation1927), called random utility theory (RUT). The RUT states that each choice characteristic cannot observe a latent factor ‘utility.’ Therefore, for each individual who chooses a business profile
, the indirect utility function
is decomposed into a deterministic factor (V) and a stochastic factor (ϵ) that represents the unobservable of the individual’s choice. The utility
of a rice farmer
when choosing a business profile
is expressed as follows:
(1)
(1) where
represents the latent unobservable utility that an individual
associates with the choice of a business profile
,
is the deterministic or observable component representing the vector of characteristics associated with the business profile
;
is the vector of the characteristic of a business profile
for an individual
, and
is the non-observable stochastic part of the utility that considers the uncertainty.
Based on the assumption that the stochastic factor is independently and identically distributed, the probability of a business profile j is chosen as the most preferred is stated in a logistic distribution. The multinomial logit (MNL) model is commonly used in discrete choice models (Crastes dit Sourd Citation2023), but it has two limitations. The first is related to the Gumbel hypothesis of identically distributed independence (IDI) of the error terms between the alternatives and the individuals, which assumes homogeneity of preferences, which is impossible because farmers’ preferences are heterogeneous. The second limitation is the hypothesis of independence of irrelevant alternatives (IIA). To overcome the limits, the mixed logit model (MXL) is the most used and most appropriate because it helps to identify the unobserved heterogeneity in preference choice (Faustin et al. Citation2010). Compared to the original MNL, the MXL provides an additional parameter representing the coefficient of the mean attributes; and the parameter
for the presence of heterogeneity (A. Arouna et al. Citation2017). When significant, it indicates the heterogeneity of preference in the data (A. Arouna et al. Citation2017). However, the mixed logit implies the decision between a set of alternatives, sometimes resulting in numerous alternatives between which the decision-maker must choose. Since each alternative has different attributes, this choice is sometimes difficult for respondents. For this reason, we use the alternative-specific mixed logit in this study, which is more flexible than the simple mixed logit model (Streletskaya et al. Citation2023). The alternative-specific mixed logit model estimates the parameters of the mixed logit model using the maximum simulated likelihood (MSL) and relaxes the assumption of the independence of irrelevant alternatives (IIA).
In this study, we proposed ten hypothetical business profiles to be tested with farmers for the choice experiment. However, for the same reasons stated above, we randomly divided both the farmer sample and the ten business profiles into two groups (five business profiles for each group). This allows farmers to select from five business profiles rather than ten, ensuring the most realistic choice. The dependent variable is the chosen business profile (BP1 … .BP10), which has the value ‘1’ if the farmer chose the business profile and ‘0’ otherwise. We also included in the model case-specific variables that vary across farmers, such as sociodemographic and economic characteristics. The alternative-specific mixed logit analysis aims to examine rice farmers’ decisions. The analysis allows us to predict which independent variables significantly predict whether or not a farmer will select a business profile. We assume no business profile is the default result (status quo). Based on the alternative-specific variables (attributes/characteristics of the proposed business profile), we expected rice farmers in each group to select one of the business profiles, one through five and six through ten.
We ran two models, each with five business profiles and the status quo (see ). Stata/SE 16 was used for the analysis. A sample of the dataset for two rice farmers is shown in appendix A1.
Table 1. Attributes and levels of the business profiles.
We also use Cronbach’s alpha test to measure the internal consistency (Grunert et al. Citation2018) of farmer’s choice and Pairwise correlation to assess the validity (appendices A2 and A3). The test shows a calculated Cronbach’s alpha of ‘’Good’’ (scale reliability coefficient: 0.87) for Group 1, meaning good reliability, and acceptable reliability (scale reliability coefficient: 0.76) for Group 2.
2.2.2. Attribute choice and level of attributes
The choice experiment approach comprises six key stages: attribute selection, attribute level assignment, experimental design selection, choice set construction, preference measurement, and estimation procedure (Weber Citation2019). The attributes represent a critical feature of the offered product or service that can influence individual preference. We determined the preferences of rice farmers based on a literature review and a workshop organized on May 28th, 2021, with the stakeholders involved (rice farmers, millers, traders, extension agents, and the external partner CARI) as the experiment in this study is based on a statistical design and a hypothetical profile. The workshop consisted of a discussion and interactive session between rice farmers and service providers to define the various components of a business profile and their options. As moderators and facilitators, AfricaRice and CARI were present. As a result, we set the attributes and their level based on both sides discussion and agreement.
We first considered (i) rice farmers’ preferences based on Lancaster's utility maximization theory and (ii) service providers’ interests in reducing prices and maximizing profit. Then, eight attributes and their levels were chosen based on these two factors and discussions ().
2.2.3. Logics behind the selection of attributes and the related levels are as follows
Payment method – one of the most significant constraints in agriculture and rice cultivation, particularly during the cropping season, is a financial constraint (Nonvide et al. Citation2018; Soullier et al. Citation2020). Because the technology is new, asking rice farmers to pay for an unusual new service is a significant challenge. As a result, three payment methods were proposed based on the literature and farmers’ contract farming experience. The business profile is a collection of activities and services provided to rice farmers, but the most crucial factor is the payment method. We hope to make payment to rice farmers (end users) easier so that it does not become a barrier to adoption. The first payment method is postharvest cash payment, meaning that the rice farmer will benefit from personalized advice and pay after harvest. We assume this will give the farmers enough time to find a buyer to cover their expenses. The second payment method is also after harvest, but the service fee is included in the rice price. This payment method gives rice farmers more flexibility because it is only based on the quantity sold. When the service provider or miller purchases the rice, the service price is fixed and deducted directly from the rice price. The third payment method is cash on delivery, which requires the farmer to pay immediately after the service is rendered. On the other hand, some farmers would rather not owe anything and be free to make their own decisions after harvest.
Price of service – the challenge is convincing rice farmers to pay for a new service that they are unfamiliar with and are also unfamiliar with paying for extension services. Rice farmers were offered a free personalized extension service in a previous experience where extension agents, CARI, and millers/rice traders collaborated to disseminate RiceAdvice, but the approach could not last long. This approach failed due to CARI's limited financial support and the lack of a business framework to assist rice millers in taking over. According to recent research, adopting new technology in agriculture is influenced by performance expectations, the effort required (Beza et al. Citation2018; Ogwuike, Arouna, and Ogwuike Citation2021), and the cost of use (Beza et al. Citation2018). These two factors could be evaluated by testing the technology and polling users on their willingness to use and pay for it. As a result, the optimal price must be determined for both technology users (rice farmers) and service providers. During the workshop, various business profiles were presented and explained to the audience. We collected rice farmers’ willingness to use and pay for the advice provided to them using the RiceAdvice application. The information gathered enabled us to propose prices, attributes, and levels for the business profiles presented in this study ().
Length of partnership – the length of a partnership reflects the acceptance of technology by rice farmers. A long-term partnership benefits both partners (rice farmers and service providers) by ensuring farmer efficiency and a sustainable business for service providers, increasing the stability of the whole chain. On the other hand, farmers may choose a short-term partnership as a test or may be unwilling to make a long-term commitment. The duration of the partnership is also a means of following up with the farmers and providing them with up-to-date information to ensure their efficiency throughout the partnership. On a business level, the service provider would prefer a long-term partnership, which the farmers may be unwilling to sign for. As a result, we propose in this study to rice farmers both short-term and long-term partnerships based on the length of a season. We have a partnership based on a single season or more than two seasons and the option of not signing any partnership. This means they can request the service provider's services whenever they need them, with the risk that the price will vary depending on the service provider's availability. When rice farmers decide on their preferred business profile, this is clearly explained to them.
Contract farming – as an additional service, the service provider could offer to buy rice from rice farmers after harvesting, which would be a separate contract. The rice farmers can sell their products to the service provider in addition to the RiceAdvice service. The farmer's payment method could be harvest-related if the farmer accepts a commercial contract. The RiceAdvice service will be paid for after harvest, or the service fee will be included in the rice price. This is a trading contract offered to farmers to guarantee a market and give them a reason to use digital extension services. The terms of this contract must be agreed upon and signed by both parties by the country's formal trading contract regulations. Farmers could use this as a guarantee to request agricultural credit to finance production and post-production activities. It should be noted that the payment method and the price of the rice purchased by the service provider are separate contract terms that must be specified in the trading contract. This business option provides rice farmers with two guarantees that can be used to improve their access to credit. The first guarantee is that they will be provided with accurate advice, increasing their efficiency and reducing the risks that financial institutions are afraid of. The second is when farmers sign up for a trading contract with a service provider, which could also be used as leverage to gain access to a financial institution.
Credit – rice farmers face significant financial constraints (Soullier et al. Citation2020), and few have access to financial institutions (Ojo et al. Citation2021). However, some rice farmers may benefit from a cash or in-kind loans from partners such as millers or traders. Due to their limited access to financial institutions and the need to finance production and post-production activities, rice farmers may occasionally apply for in-kind or cash credit through an unofficial structure. These deals are not always beneficial to farmers, but they appear to be the most accessible option for rice farmers. In rural areas, this practice is very common and well developed. That is why we offer rice farmers the option of applying for credit or not. Both parties should discuss and agree on the nature of the credit (cash or in-kind).
Additional paid service – because this is a collaboration and rice farmers are used to paying for other agricultural services, it will be interesting to see if they are open to additional paid services. It is viewed as an opportunity for other services that farmers may be interested in or require. It could also be viewed as an incentive approach for farmers to respond to their needs individually during the production process. Rice farmers and service providers could discuss the potential additional service and decide whether or not to sign up for it.
Agreement on the quantity and the quality – these features are consistent with the trading contract. Depending on the partnership agreement, they may or may not be included in the trading contract. These features are required for the service provider to ensure the quantity and quality of the product. On the other hand, rice farmers may see this as a constraint. The options here are then agreement or not on the rice's quantity and quality.
2.2.4. Experimental design of the choice set and implementation
The success of the experimental design depends on the attributes and their level. With a total of eight attributes (), two of which have three levels and six with two levels, we could have a total of combinations and possible theoretical business profiles. However, it is unrealistic for rice farmers to choose between 288 business profiles. Therefore, the orthogonal fractional factorial approach in SPSS version 16 software was used to reduce the number of business profiles. This approach reduces the number of profiles without affecting the experiment’s validity. We obtained sixteen business profiles, which we consider much more realistic than the 288 profiles. As explained by Teece (Citation2018), two important conditions can ensure the sustainable adoption of the application and the business: (i) the viability of the business and (ii) the feasibility of the business. In addition to the external factors that may positively or negatively affect the business, we must consider these two factors when selecting the potential business profile. Out of the sixteen theoretical business profiles, we retain ten business profiles that we believe meet the two conditions. The reasons for deleting six business profiles are explained in appendix A4.
Table 2. Experimental design group 1.
and show the groups of business profiles (BP) presented to the rice farmers. At this point, we explained (providing the details and the content of each business profile) the five business profiles to each farmer in each group, and then the farmer stated their preferred business profile.
Table 3. Experimental design group 2.
2.2.5. Overview of the variables included in the models
presents the definition and details of the variables included in the models and the expected sign of the socioeconomic characteristics. The dependent variable is binary (1 = Yes; 0 = No), indicating each rice farmer’s choice of a business profile. The alternative variable represents the ten business profiles divided into two groups of five business profiles, each plus the status quo. The choice of the independent variables was based on the existing literature on the adoption of digital farming technologies and the adoption of business profiles (Hoang Citation2020; Khan et al. Citation2019; Leng et al. Citation2020; López-Becerra, Arcas-Lario, and Alcon Citation2016).
Table 4. Definition of variables used in the models.
Greater education, we believe, could contribute and help rice farmers better understand the proposed value and assist them in their decision. Since the proposed business profile implies rice farmers paying for a service, purchasing power is an essential factor influencing rice farmers’ decisions. Another important parameter is the knowledge of RiceAdvice, which is the core of the proposed business profile. A previous study shows that rice farmers appreciate the technology, use it, and would be willing to use it again and pay for it (Aminou Arouna et al. Citation2020). We know that the reality might be different and challenging as rice farmers face financial constraints every season. According to Miranda et al. (Citation2016), Amoussouhoui et al. (Citation2022) and Arouna et al. (Citation2020), one of the key steps to the adoption of a new technology is knowledge of the technology.
2.3. Identification of the most preferred business profiles
The most preferred business profile was identified using a post-estimation command from the alternative-specific mixed logit model. It generates a prediction probability that a farmer j chooses a business profile or alternative i. The predicted probability of farmer j choosing alternative or business profile i is calculated as in Equationequation 2(2)
(2) .
(2)
(2) where M is the number of random draws and
are the logistic probabilities.
2.4. Building the optimum business profile
Even if the predicted probability of choosing a business profile will provide the most preferred business profile, it does not inform about the attributes and socioeconomic characteristics essential to propose an optimum business profile. Therefore, we use the Chi-square automatic interaction detection algorithm (CHAID) analysis to identify the essential attributes of a successful business profile. The CHAID analysis implies algorithms that determine the close interaction between the independent variables (the attributes of business profiles and the socioeconomic characteristics) and the dependent variable (the most preferred business profiles) using the chi-square test and the likelihood ratio method (Dollman, Gunn, and Hull Citation2021; Vial et al. Citation2021). This methodology presents four main advantages: (i) it allows the use of both quantitative and qualitative data; (ii) the result generates a decision tree straightforward to interpret; (iii) it facilitates the identification of the interaction between independent and dependent variables and, (iv) does not require the assumption of homoscedasticity, multicollinearity, and independence (Rodríguez-Sabiote et al. Citation2021). Therefore, this study considers the first three most preferred business profiles (based on the predicted probability) as the dependent variables and their attributes and socio-economic characteristics as the independent variables.
3. Results
This section discusses the findings in detail by responding to the research questions. The long-term adoption approach of the digital extension service is based on the determinant factors and primary attributes for successful service provision. Before looking deeper into the analysis details, summarizes the findings.
3.1. Determining factors of the chosen business profile
and present the results of the determinant analysis for the two groups. Results showed that based on the Wald chi-square test [Waldχ2(45) = 256.59, p < 0.001] and [Waldχ2(45) = 246.02, p < 0.001] for the overall models for groups 1 and 2, respectively, containing the complete set of predictors are significant. This implies that at least one of the regression coefficients is not equal to zero.
Table 5. Alternative-specific mixed logit of group 1.
Table 6. Alternative-specific mixed logit of group 2.
Overall, socioeconomic and institutional characteristics affect the choice of farmers. Among the nine variables included in the models, six were statistically significant for group 1, and eight were found statistically significant for group 2. Specifically, the variable gender is significant for group 2, and the log-odds of female rice farmers adopting business profiles 6, 8, 9, and 10 were 1.718, 1.107, 1.120, and 1.867. This means that females are more likely to adopt these business profiles. The variable age is significant in both blocks 1 and 2. In group 1, age is significant at 5% for business profile 1 and 1% for business profiles 2, 3, and 4. In group 2, age is significant at 10% for business profile 8, 5% for business profiles 6 and 7, and 1% for business profile 9. The positive slope indicates that the older the farmer, the more likely he is to choose a business profile.
The education level, considered an essential parameter, is significant in both models. The variable is significant at 1% for business profiles 1, 2, 3, 4, 6, 7, and 8; at 5% for business profile 5, and 10% for business profile 9. This result implies that more educated rice farmers with formal education are more likely to choose a business profile.
The variable experience in rice production is significant and surprisingly negative for business profiles 3 and 4 in group 1 and all the business profiles for group 2. This is an unexpected sign as we assumed that rice farmers with more rice production experience would better understand the necessity of the opportunity in the proposed business profiles and, therefore, could be more open to adoption. However, rice farmers choose a business profile based on their experience individually.
Knowledge of the RiceAdvice, as expected, is positive and significant for business profiles 1, 3, and 6 at 1% and surprisingly negative and significant for business profile 9. The positive slope confirms the importance of first sharing the information and informing the rice farmers about the technology and the business profiles. However, note that the significance and positive slope were found on three business profiles over ten, which is not enough to generalize overall models.
The variable contact with the extension agent is significant and positive for business profile 1 and negative for business profile 7. This result confirms the uncertainty regarding the knowledge of the technology. Furthermore, if the agents do not have the information or do not transfer the information efficiently, this could have a negative effect, as recorded on the business profile 7. Otherwise, the extension agents could play a role in disseminating the information and proposing the service to the farmers.
Along the same line, the association, also known as a channel of learning and sharing information, is significant and positive at 1% for business profiles 1, 6, and 9; and 5% for business profile 10. This means that farmers of an agricultural association are more likely to choose these business profiles.
The size of the rice production is significant and positive at 1% for business profiles 2, 3, 6, and 8; at 10% for business profile 10. This is an expected sign since we assume that the bigger the farm size, the more interested rice farmers will be in using the technology and choosing a business profile.
The household income is significant and positive for business profiles 1, 3, 4, 5, and 9 but is negative for business profiles 6 and 7. The positive sign implies that the higher the household income, the more likely rice farmers will adopt the technology and therefore be open to choosing the business profiles. However, the negative sign could be due to the payment method, which is the common attribute of business profiles 6 and 7.
3.2. Identification of the most preferred business profiles
displays the predicted likelihood of the selected business profiles by group. According to the findings, business profile 3 has the highest probability in group 1 and thus is the most likely business profile to be adopted by 49.4% of the farmers in group 1. Business profile 6 (44.7%) in group 2 is the second most preferred, and business profile 1 (26.8%) in group 1 is the third most preferred. Intuitively, we will keep/promote the business profile with a 25% chance of being chosen. At this point, we can keep the first three business profiles that rice farmers are likely to select: business profiles 1, 3, and 6.
Table 7. Summary statistics of the predicted probability.
3.3. Identifying the attributes and socio-economics characteristics for the optimum business profile
The first three business profiles are made up of attributes that do not help determine which business profile is most likely to be suitable. depicts the selected variable by the algorithm as the essential attributes among the variables (length of partnership; payment methods; price of service; access to credit; additional pay services; contract farming; agreement on quantity; quality agreement; household income; gender; knowledge of RiceAdvice; education level; farm size; experience in rice production; member of agricultural association and contact with extension agent) included in the model.
The algorithm identified education level as the first predictor, followed by service price, credit availability, and payment method. According to the farmer's education level, the model recommends three optimal business profiles. For farmers with no education, the model recommends a price of 14.50 USD/hectare (6000 Naira/hectare). However, when it comes to payment methods, we have two suggestions. The first has the highest percentage (35.4%), indicating that the payment method is ‘cash at delivery.’ The second option is to leave the payment method open and allow rice farmers to select a suitable payment method. The model suggests not providing credit to farmers with a primary education level.
Cash after harvest at 9.70 USD/hectare (4000 Naira/hectare) would be the most appropriate payment method for senior or tertiary-level farmers.
4. Discussion
Digital agricultural extension technologies provide an opportunity to improve farmer efficiency and public extension quality. However, adoption remains a topic of interest that requires attention. In response to farmers’ constraints and barriers to direct adoption of digital extension technologies faced by developing countries, the study proposed an indirect adoption approach based on a personalized service business model. An ex-ante evaluation of the proposed business could increase its chances of success. Our research employs a digital extension application designed to provide rice farmers with personalized advice. Using a choice experiment approach, the study determines the factors that may lead farmers to select a business profile offering a remunerated service through the application. In addition, we were interested in the socioeconomic characteristics to extrapolate our findings.
The findings revealed several significant parameters that determine the choice of rice farmers. The gender parameter is a crucial factor for adopting a business profile, as Zossou et al. (Citation2020) highlighted. Results revealed that women are more likely to adopt four over ten business profiles. The role of women in the adoption process is widely covered in the literature (Chatterjee, Dutta Gupta, and Upadhyay Citation2020; Orser, Riding, and Li Citation2019). A study conducted by Hay and Pearce (Citation2014) revealed that women used rural technology three times more than men. Similarly, Gichuki and Mulu-Mutuku (Citation2018) showed the importance of women in adopting mobile money technologies. However, the authors also mention that women are less likely to adopt mobile banking technologies viewed as prestigious services with hidden fees out of their social class. Therefore, mobile banking technology may not fit the needs of women. Along the same line, Chatterjee, Gupta, and Upadhyay (Citation2020) and Orser, Riding, and Li (Citation2019) developed the role of technology in women's empowerment and its importance for gender-inclusive entrepreneurship. Other research found that women are less likely to adopt agricultural technology than men (Aryal et al. Citation2020). Although both men and women have access to new technologies, as long as they are in the same environment, women are less likely to adopt digital technology. Voss et al. (Citation2021) explained this by referring to the social-cultural reality in rural area in which IT devices such as Cellphones, radios, and televisions are primarily controlled by men, implying that men are the primary owners and have authority over the device in the household. Involving women in developing and adopting digital extension technologies would significantly reduce the gap and increase the adoption rate (Voss et al. Citation2021). It should be noted that most of the abovementioned studies focus on women using the technology by themselves, which is different from the approach of our study since we propose a service using the technology based on indirect adoption of the technology, which can be considered as an advantage.
The parameter Age is positive and significant for the overall business profiles. This implies that older rice farmers would be more likely to choose a business profile and therefore adopt the technology. This could be explained by the fact that older farmers (hypothetically based on their experiences) are more aware of IT advantages than young rice farmers. However, they do not know how to use it or access the required infrastructure, which makes this an opportunity to take advantage of and guarantee more successful production. Furthermore, it makes farming more manageable since farmers will execute the activities according to the advice. Also, since older farmers have more experience in farming, they could be in a better position to evaluate more accurately the necessity of adopting digital extension technologies through the business profile. However, the opposite effect could be seen (Berkowsky, Sharit, and Czaja Citation2017), showing the unlikely probability of older farmers adopting new technology. This result is opposite to that of Paudel et al. (Citation2021), who found that older farmers are less likely to adopt precision agriculture technologies, while Roberts et al. (Citation2004) stated that older farmers do not adopt technology compared to younger farmers. This is understandable as we cannot objectively expect older farmers (usually with no/or limited access or knowledge of digital technology) to adopt digital farming technology directly. However, the adoption rate could increase if the adoption is indirect, as proposed in the case of this study.
The education level, perceived as an open-minded factor, could play a determining role in rice farmers’ decision-making and effectively contribute to a better understanding of the concept. Therefore, the positive coefficient of the parameter is expected. It should guide the business design by involving educated rice farmers and providing basic educational knowledge as a supplementary service to those who are not educated. This could help to improve their decision-making capacity and make them feel more comfortable and open to new technologies and opportunities. Carrer, de Souza Filho, and Otávio Batalha (Citation2017) reported the same positive effect of education on the likelihood of adopting computers. The authors argued that highly educated farmers express an interest and the need for accurate information and could appreciate and evaluate the advantages of using IT as a tool for decision-making. On the other hand, the experience in rice production is significant and negative for seven of the ten business profiles. This is unexpected since we hypothesized that farmers with more experience would be more open to choosing a business profile. Nevertheless, the negative sign advises that this variable should be looked at during the dissemination process to understand and determine how to turn this parameter in favor of a better adoption. The result could also be justified by the fact that farmers with more experience in rice production were aware of the importance of the proposed service, especially in terms of using digital extension applications for advice. Still, they cannot see the impact on their activities without mentioning the financial constraints that lead most rice farmers to informal loans. In addition, the variable experience could be a double-sided blade where their experience provided valuable insight. It can also hinder their willingness to use or adopt the service as they might consider themselves too knowledgeable.
Knowledge about the technology as a driver in the adoption process is necessary and, if well done, should provide farmers required information on the technology and therefore ensure that potential adopter is aware of the value proposition and that their decision is entirely based on their utility function and that the non-adoption is not due to a lack of information. This finding is in line with Ferrari et al. (Citation2022) and Coggins et al. (Citation2022), who argue that educational support should be used to facilitate digital knowledge by training farmers, followed by the creation of innovative digital centers. In the same line as Voss et al. (Citation2021), farmers’ knowledge about the new technology influences their decision to adopt it, along with the availability of the technology and how farmers perceive the impact of the adoption on their activities. Smallholder farmers are very cautious when adding additional costs to their production line, especially when considering financial constraints and the high-risk environment, unless they have the appropriate knowledge and information, particularly in terms of outcome (Vercillo, Weis, and Luginaah Citation2020; Voss et al. Citation2021). Along the same line, we believe that the role of the extension agent is crucial for sharing information and educating farmers on the potential and opportunities related to the adoption of digital technologies (Amoussouhoui et al. Citation2022; Fabregas et al. Citation2022). Since there is direct contact with the user, the extension agents would explain better. Even if the variable is significant and positive in business profile 1, it is negative in business profile 7. It could be due to the quality of the information or the lack of information. This result is in line with Walisinghe et al. (Citation2017) and Suvedi, Ghimire, and Kaplowitz (Citation2017), who found that extension services positively affect farmers’ decisions to adopt new technologies.
Farmers could also receive information about technology adoption or service proposition through agricultural associations. Farmers of an agricultural association are likelier to choose business profiles 1, 6, 9, or 10, including all the different payment methods. This shows the diversity in the choice, especially if we assume that the choice is mainly based on the payment method. Therefore, involving farmers in agricultural associations would be beneficial to disseminate the information better. This result is in line with Suvedi, Ghimire, and Kaplowitz (Citation2017), who also found a positive effect of membership, extension service, and age on technology adoption.
The choice of a business profile could also depend on whether the rice farmer has a small or big rice farm when it needs more human and financial resources. As hypothesized, the farmers with big rice farms would be more interested in choosing a business profile. In the study, five of the ten business profiles were chosen with diversity in the payment method and other attributes of the business profiles. The business profile implies rice farmers paying for a service that we know could be a barrier to adoption. It is also well known that farmers face many financial difficulties along the production chain. Therefore, adding a new expense would be very challenging, even if the service is beneficial and impactful. That is why we proposed different payment methods. However, we hypothesized that if the household income were more open, this household could choose a business profile. This hypothesis is verified with five (1, 3, 4, 5, and 9) of the ten business profiles. This result is consistent with the findings of Paudel et al. (Citation2021), who reported that higher-income households could easily access the technology since they have cash, which is not the case for lower-income households. The same effect is also reported by Isgin et al. (Citation2008), with a positive effect of income on the adoption of precision farming technologies. However, two business profiles (6 and 7) negatively affected household income. This result could be due to the payment method (Cash payment after harvest), which is the common attribute of the two business profiles that users can see as a constraint.
Besides analyzing determinants, we also generate the predicted probability of the chosen business profiles, which show the most preferred business profile. Intuitively we consider business profiles that have a minimum of 25% probability. Based on that, depicts the shape of the business profiles and shows the three business profiles (1, 3, and 6 see appendix A5) that fit the criteria and are considered the most preferred.
Business profile 3 has the highest predicted probability of being adopted at 49.4%, with a cash payment after harvest for the service provided at 9.70 USD/hectare (4000 Naira/hectare) for more than two seasons contract, followed by business profile 6 with a predicted probability of 44.7%, with cash payment after harvest for the service provided at 14.50 USD/hectare (6000 Naira/hectare) for 1 season contract, and the business profile 1 with predicted of 26.8% with a cash payment at delivery for 14.50 USD/hectare (6000 Naira/hectare) for the one-season contract. Most farmers in the two blocks choose business profiles 3 and 6. The result confirms that farmers would have difficulty paying cash, but this is an affordable option after harvest. It is also to the advantage of the service provider to have an overview of his customers (farmers) from the beginning of the season and, therefore, put in place a financial and structural plan for the intervention. Further analysis was also conducted to identify the crucial attributes and socioeconomic factors. This could help to compute the optimum business profile. Results reveal that for an optimum business profile, the attributes of the price of service, access to credit, and payment method are the most important ones that need to be considered. The education level was the most important factor among all the variables included in the model and the foundation for an optimum business profile. Therefore, the algorithm proposes three possibilities according to the education level. Overall, the optimum business profile would include all education levels, 14.50 USD/hectare (6000 Naira/hectare) as an optimum price for a cash payment after harvest with no access to credit. However, access to credit (cash or input) could be an attractive option to convince farmers.
5. Limits of the study
This is the first study in the literature to propose a new adoption approach for digital extension services, and an evaluation for long-term adoption. However, we would like to highlight some limitations of the study.
We did not use the traditional choice experiment, which involves submitting a group of attributes to farmers to make a choice uncomplicated and more efficient for them. We believe that explaining this to farmers would have been more complex, and we see this study as a first step.
Some of the business profile attributes could be combined (Agreement on quality, Agreement on quality, trading contract) to reduce the number of alternatives and thus make the choice easier for farmers.
We proposed a hypothetical model for adoption, and it would be beneficial to conduct a follow-up study in collaboration with rice value chain actors such as extension agents, private advisors, farmers, and rice traders. On the other hand, in a future study, we recommend reducing the number of business profiles as well as the attributes and conducting a randomized control trial study to see if farmers would indeed adopt and pay for digital extension services, as well as evaluating the impact of their adoption on key factors such as production, wellbeing, and food security.
6. Conclusion and policy implications
The traditional extension service provided by government extension agents has shown its limits. Therefore, new approaches and policies are required to provide farmers with more efficient and impactful advice. With the rise of new digital extension technologies, the study provided an operational solution to support adopting the digital extension approach. The case of the present study is a digital extension technology designed to provide tailored advice for rice farmers in Sub-Sahara Africa. As a solution to facilitate and guarantee a sustainable adoption of digital extension technology, the study proposes a business approach based on service providing as an indirect adoption approach. This approach can be used by the private sector interested in investing in agribusiness and external agricultural partners (NGO and International Organizations) to support the digitalization of extension and also for policymakers to adopt policies and make government extension agents more efficient. The study also provides a solution to decreasing public support for agriculture, especially regarding extension in developing countries. The outcome provided by the experimental choice approach offers much more information that we believe is useful to build a more appropriate business profile with a higher adoption rate of digital extension technologies. The results of this study lead to three main conclusions:
- Age, education level, rice production experience, knowledge of RiceAdvice, contact with extension agent, association membership, rice production size, and household income are socioeconomic and institutional parameters that influence rice farmers’ choice of a business profile;
- Cash payment after harvest at 9.70 USD/hectare for more than two seasons contract, cash payment after harvest at 14.50 USD/hectare for one season contract, and cash payment at delivery at 14.50 USD/hectare for a one-season contract are the most preferred profiles and thus more likely to adoption by rice farmers;
- The best business profile should prioritize education level as the first and most important predictor, followed by service price, credit access, and payment options that are appealing to rice farmers.
However, as a technology design, this study is a step forward toward a long-term approach for ensuring the adoption of digital extension technologies despite existing barriers. Government and extensionists must support, promote, and disseminate the idea of a business approach using digital extension technologies to support smallholder farmers. This adoption approach represents a business opportunity for private investors and an opening for developing new policies and strategies to assess farmers’ needs and develop appropriate technologies to support them. With this approach, all farmers willing to pay for the proposed service can receive advice from digital extension technologies even if they do not know how to use them.
Availability of data
The data supporting this study's findings are available from the corresponding author upon reasonable request.
Acknowledgements
We thank all CGIAR Trust Fund and the Government of Belgium for their financial contribution through the Transforming Agrifood Systems in West and Central Africa Initiative (TAFS-WCA). We also thank The International Fund for Agricultural Development (IFAD) for financing the project ‘Sustainable and Diversified Rice-based Farming Systems’ (DCI-FOOD/2015/360-968) and the Excellency in Agronomy (EiA) as well as the Internal Grant Agency of the Faculty of Tropical AgriScience (grant No.: 20233101) for their financial support. The authors would also like to thank the Competitive African Rice Initiative (CARI), Green Sahel, and ATAFI for their assistance with the survey.
Disclosure statement
No potential conflict of interest was reported by the author(s).
Additional information
Funding
Notes on contributors
Rico Amoussohoui
Rico Amoussouhoui completed a bachelor's degree in Agronomy, a Master's in management of the rural enterprise, an MBA in Agribusiness and Food management enterprise, and is currently a Ph.D. candidate in sustainable agricultural technologies at the Czech University of Life Sciences in Prague. He worked with AfricaRice as a research assistant and monitoring and evaluation specialist. He is also an independent consultant in agricultural technology adoption and agribusiness and the author of articles published in SCI journals.
Aminou Arouna
Dr. Aminou Arouna is the Program Leader for the Policy, Innovation Systems, and Impact Assessment Program, one of the four research programs at AfricaRice. His works include ex-post impact assessment of NERICA varieties on poverty and food security in 16 countries, the experimental impact of digital and personalized extension advice tools and contract farming schemes on livelihood using randomized control trial approach, gender learning, and adoption in agriculture, rice value chain upgrading in West Africa and impact of rice policy. Aminou also has substantial experience in ME&L for development intervention. He published more than 70 papers in different journals, including the American Journal of Agricultural Economist and Development Economics. Aminou obtained his Ph.D. with distinction (Magna cum laude) at the University of Hohenheim (Germany) in 2009. He has more than 20 years of research for development in Sub-Saharan Africa.
Miroslava Bavorova
Ass. Prof. Bavorova Miroslava is an Associate Professor in the Department of Economics and Development at the Czech University of Life Sciences in Prague. She received her Ph.D. in agriculture from the University of Göttingen. Since 2002, she has held a position as a researcher at IAMO in Halle and since 2008–2017 at the Martin-Luther University in Halle-Wittenberg. Her interdisciplinary research is mainly based on economic, sociologic, and psychologic theories. She is interested in analyzing actors’ behavior regarding the environment, food safety and security, migration, willingness to work in agriculture, and consumer behavior. She established a research group, ‘Behavioural study in the agri-food sector,’ and has published several papers in high-ranking journals.
Vladimir Verner
Ass. Prof. Vladimir Verner currently holds the position of Professor assistant at the Faculty of Tropical AgriSciences, Czech University of Life Sciences Prague, Czechia. He obtained his Ph.D. degree in agricultural economics in 2007. He has been the leader of the research group TRACE (Tropical Farming Systems & Ecological Economics) since 2015 and, since 2020, also vice-head of the department of economics and development. His specialization is resource and process economics, valuating investments for agricultural development, nutrition-sensitive and biodiversity-based value chains, and the efficiency of poverty alleviation strategies. He provided consultancy on the commercial transformation of subsistence farming systems, land use dynamics, and sustainable agricultural production for FAO, UNDP, IFAD, and the EC. He is also the author of several papers published in SCI journals.
Wilfried Yergo
Wilfried Yergo has a master's in economics. He is currently a Research Assistant at AfricaRice in Policy, Innovation Systems, and Impact Assessment Program. He is a research assistant in Economic Data Management & Analysis. His research interests include establishing ex-ante and ex-post impact assessment methods and conducted several data collection and management systems and software. He is the author and co-author of several papers published in SCI journals.
Jan Banout
Prof. Jan Banout received his Ph.D. in tropical agriculture at the Czech University of Life Sciences Prague in 2005. He is a professor and senior Food Security and Food Processing Technology researcher. He is the head of the Department of Sustainable Technology, Faculty of Tropical AgriSciences, Czech University of Life Sciences Prague. He has a significant level of experience in both teaching and research, including field investigation and surveys conducted in different developing countries. He has strong experience as a senior consultant in the disciplines mentioned above. He was a manager or member of research teams of several projects conducted in Latin America, Africa, and Asia, focusing on sustainable development of agriculture technology, food processing, food security, and organic waste management. He was an invited speaker and lecturer at several universities in Europe, Asia, and Africa. The publications are available on ORCID: 0000-0002-9647-1426.
References
- Amoussouhoui, Rico, Aminou Arouna, Miroslava Bavorova, Haritini Tsangari, and Jan Banout. 2022. “An Extended Canvas Business Model: A Tool for Sustainable Technology Transfer and Adoption.” Technology in Society 68 (February), https://doi.org/10.1016/J.TECHSOC.2022.101901.
- Arouna, A., P. Y. Adegbola, R. C. Zossou, R. Babatunde, and A. Diagne. 2017. “Contract Farming Preferences by Smallholder Rice Producers in Africa: A Stated Choice Model Using Mixed Logit.” Tropicultura 35 (3): 179–191. https://doi.org/10.25518/2295-8010.1257.
- Arouna, Aminou, Jeffrey D. Michler, Wilfried G. Yergo, and Kazuki Saito. 2020. “One Size Fits All? Experimental Evidence on the Digital Delivery of Personalized Extension Advice in Nigeria.” American Journal of Agricultural Economics, 1–24. https://doi.org/10.1111/ajae.12151.
- Aryal, Jeetendra Prakash, Cathy R. Farnworth, Ritika Khurana, Srabashi Ray, Tek B. Sapkota, and Dil Bahadur Rahut. 2020. “Does Women’s Participation in Agricultural Technology Adoption Decisions Affect the Adoption of Climate-Smart Agriculture? Insights from Indo-Gangetic Plains of India.” Review of Development Economics 24 (3): 973–990. https://doi.org/10.1111/RODE.12670.
- Ayim, Claudia, Ayalew Kassahun, Chris Addison, and Bedir Tekinerdogan. 2022. “Adoption of ICT Innovations in the Agriculture Sector in Africa: A Review of the Literature.” Agriculture and Food Security 11 (1): 1–16. https://doi.org/10.1186/S40066-022-00364-7/TABLES/9.
- Balafoutis, Athanasios, Bert Beck, Spyros Fountas, Jurgen Vangeyte, Tamme van der Wal, Iria Soto, Manuel Gómez-Barbero, Andrew Barnes, and Vera Eory. 2017. “Precision Agriculture Technologies Positively Contributing to GHG Emissions Mitigation, Farm Productivity and Economics.” Sustainability 9 (8), https://doi.org/10.3390/SU9081339.
- Barakabitze, Alcardo A., Edvin J. Kitindi, Camilius Sanga, Ayubu Shabani, Joseph Philipo, and George Kibirige. 2015. “New Technologies for Disseminating and Communicating Agriculture Knowledge and Information: Challenges for Agricultural Research Institutes in Tanzania.” The Electronic Journal of Information Systems in Developing Countries 70 (1): 1–22. https://doi.org/10.1002/J.1681-4835.2015.TB00502.X.
- Berkowsky, Ronald W, Joseph Sharit, and Sara J Czaja. 2017. “Factors Predicting Decisions About Technology Adoption Among Older Adults.” Innovation in Aging 1 (3), https://doi.org/10.1093/GERONI/IGY002.
- Beza, Eskender, Pytrik Reidsma, P. Marijn Poortvliet, Melisew Misker Belay, Ben Sjors Bijen, and Lammert Kooistra. 2018. “Exploring Farmers’ Intentions to Adopt Mobile Short Message Service (SMS) for Citizen Science in Agriculture.” Computers and Electronics in Agriculture 151 (August): 295–310. https://doi.org/10.1016/j.compag.2018.06.015.
- Birner, Regina, Thomas Daum, and Carl Pray. 2021, March. “Who Drives the Digital Revolution in Agriculture? A Review of Supply-Side Trends, Players and Challenges.” Applied Economic Perspectives and Policy. https://doi.org/10.1002/AEPP.13145.
- Carrer, Marcelo José, Hildo Meirelles de Souza Filho, and Mário Otávio Batalha. 2017. ““Factors Influencing the Adoption of Farm Management Information Systems (FMIS) by Brazilian Citrus Farmers.” Computers and Electronics in Agriculture 138 (June): 11–19. https://doi.org/10.1016/J.COMPAG.2017.04.004.
- Chatterjee, Susmita, Sangita Dutta Gupta, and Parijat Upadhyay. 2020. “Technology Adoption and Entrepreneurial Orientation for Rural Women: Evidence from India.” Technological Forecasting and Social Change 160 (November), https://doi.org/10.1016/J.TECHFORE.2020.120236.
- Coggins, Sam, Mariette McCampbell, Akriti Sharma, Rama Sharma, Stephan M. Haefele, Emma Karki, Jack Hetherington, Jeremy Smith, and Brendan Brown. 2022. “How Have Smallholder Farmers Used Digital Extension Tools? Developer and User Voices from Sub-Saharan Africa, South Asia and Southeast Asia.” Global Food Security 32 (March), https://doi.org/10.1016/J.GFS.2021.100577.
- Cotter, Marc, Folkard Asch, Bayuh Belay Abera, Boshuwenda Andre Chuma, Kalimuthu Senthilkumar, Arisoa Rajaona, Ando Razafindrazaka, Kazuki Saito, and Sabine Stuerz. 2020. “Creating the Data Basis to Adapt Agricultural Decision Support Tools to New Environments, Land Management and Climate Change—A Case Study of the RiceAdvice App.” Journal of Agronomy and Crop Science 206 (4): 423–432. https://doi.org/10.1111/JAC.12421.
- Crastes dit Sourd, Romain. 2023. “A New Empirical Approach for Mitigating Exploding Implicit Prices in Mixed Multinomial Logit Models.” American Journal of Agricultural Economics, https://doi.org/10.1111/AJAE.12367.
- Dawkins, Marian Stamp. 2016. “Animal Welfare and Efficient Farming: Is Conflict Inevitable?” Animal Production Science 57 (2): 201–208. https://doi.org/10.1071/AN15383.
- Dollman, James, Kate M. Gunn, and Melissa Joan Hull. 2021. “Sociodemographic Predictors of Attitudes to Support Seeking from a Medical Doctor or Other Health Provider Among Rural Australians.” International Journal of Behavioral Medicine 28 (5): 616–626. https://doi.org/10.1007/S12529-021-09956-2.
- Ellison, Brenna, John C. Bernard, Michelle Paukett, and Ulrich C. Toensmeyer. 2016. “The Influence of Retail Outlet and FSMA Information on Consumer Perceptions of and Willingness to Pay for Organic Grape Tomatoes.” Journal of Economic Psychology 55 (August): 109–119. https://doi.org/10.1016/j.joep.2016.05.002.
- Fabregas, Raissa, Tomoko Harigaya, Michael Kremer, and Ravindra Ramrattan. 2022. “Digital Agricultural Extension for Development.” Introduction to Development Engineering, 187–219. https://doi.org/10.1007/978-3-030-86065-3_8.
- Faustin, Vidogbèna, Anselme A. Adégbidi, Stephen T. Garnett, Delphin O. Koudandé, Valentin Agbo, and Kerstin K. Zander. 2010. “Peace, Health or Fortune? Preferences for Chicken Traits in Rural Benin.” Ecological Economics 69 (9): 1848–1857. https://doi.org/10.1016/j.ecolecon.2010.04.027.
- Ferrari, Alessio, Manlio Bacco, Kirsten Gaber, Andreas Jedlitschka, Steffen Hess, Jouni Kaipainen, Panagiota Koltsida, Eleni Toli, and Gianluca Brunori. 2022. “Drivers, Barriers and Impacts of Digitalisation in Rural Areas from the Viewpoint of Experts.” Information and Software Technology 145 (May), https://doi.org/10.1016/J.INFSOF.2021.106816.
- Gichuki, Castro Ngumbu, and Milcah Mulu-Mutuku. 2018. “Determinants of Awareness and Adoption of Mobile Money Technologies: Evidence from Women Micro Entrepreneurs in Kenya.” Women’s Studies International Forum 67 (March): 18–22. https://doi.org/10.1016/J.WSIF.2017.11.013.
- Gow, Gordon, Ataharul Chowdhury, Jeet Ramjattan, and Wayne Ganpat. 2020. “Fostering Effective Use of ICT in Agricultural Extension: Participant Responses to an Inaugural Technology Stewardship Training Program in Trinidad.” The Journal of Agricultural Education and Extension 26 (4): 335–350. https://doi.org/10.1080/1389224X.2020.1718720.
- Grunert, K. G., W. I. Sonntag, V. Glanz-Chanos, and S. Forum. 2018. “Consumer Interest in Environmental Impact, Safety, Health and Animal Welfare Aspects of Modern Pig Production: Results of a Cross-National Choice Experiment.” Meat Science 137 (March): 123–129. https://doi.org/10.1016/J.MEATSCI.2017.11.022.
- Hay, Rachel, and Philip Pearce. 2014. “Technology Adoption by Rural Women in Queensland, Australia: Women Driving Technology from the Homestead for the Paddock.” Journal of Rural Studies 36 (October): 318–327. https://doi.org/10.1016/J.JRURSTUD.2014.10.002.
- Hoang, Hung Gia. 2020. “Determinants of the Adoption of Mobile Phones for Fruit Marketing by Vietnamese Farmers.” World Development Perspectives 17 (March): 1–8. https://doi.org/10.1016/J.WDP.2020.100178.
- Isgin, Tamer, Abdulbaki Bilgic, D. Lynn Forster, and Marvin T. Batte. 2008. “Using Count Data Models to Determine the Factors Affecting Farmers’ Quantity Decisions of Precision Farming Technology Adoption.” Computers and Electronics in Agriculture 62 (2): 231–242. https://doi.org/10.1016/J.COMPAG.2008.01.004.
- Karpouzoglou, Timothy, Zed Zulkafli, Sam Grainger, Art Dewulf, Wouter Buytaert, and David M. Hannah. 2016. “Environmental Virtual Observatories (EVOs): Prospects for Knowledge Co-Creation and Resilience in the Information Age.” Current Opinion in Environmental Sustainability 18 (February): 40–48. https://doi.org/10.1016/J.COSUST.2015.07.015.
- Khan, Nasir Abbas, Gao Qijie, Selamawit Fantahun Sertse, Md Nur Nabi, and Palwasha Khan. 2019. ““Farmers’ Use of Mobile Phone-Based Farm Advisory Services in Punjab, Pakistan.” Information Development 36 (3): 390–402. https://doi.org/10.1177/0266666919864126.
- Kieti, John, Timothy Mwololo Waema, Heike Baumüller, Elijah Bitange Ndemo, and Tonny Kerage Omwansa. 2022. “What Really Impedes the Scaling out of Digital Services for Agriculture? A Kenyan Users’ Perspective.” Smart Agricultural Technology 2 (December), https://doi.org/10.1016/J.ATECH.2022.100034.
- Klerkx, Laurens, Emma Jakku, and Pierre Labarthe. 2022. “A Review of Social Science on Digital Agriculture, Smart Farming and Agriculture 4.0: New Contributions and a Future Research Agenda.” NJAS: Wageningen Journal of Life Sciences 90–91 (1): 1–16. https://doi.org/10.1016/J.NJAS.2019.100315.
- Klerkx, Laurens, and David Rose. 2020. “Dealing with the Game-Changing Technologies of Agriculture 4.0: How Do We Manage Diversity and Responsibility in Food System Transition Pathways?” Global Food Security 24 (March), https://doi.org/10.1016/J.GFS.2019.100347.
- Krah, Kwabena, Hope Michelson, Emilie Perge, and Rohit Jindal. 2019. “Constraints to Adopting Soil Fertility Management Practices in Malawi: A Choice Experiment Approach.” World Development 124 (December), https://doi.org/10.1016/j.worlddev.2019.104651.
- Lancaster, Kelvin J. 1966. “A New Approach to Consumer Theory.” The Journal of Political Economy 74 (2): 132–157. https://doi.org/10.1086/259131
- Leeuwis, Cees, and Noelle Aarts. 2011. “Rethinking Communication in Innovation Processes: Creating Space for Change in Complex Systems.” The Journal of Agricultural Education and Extension 17 (1): 21–36. https://doi.org/10.1080/1389224X.2011.536344.
- Leng, Chenxin, Wanglin Ma, Jianjun Tang, and Zhongkun Zhu. 2020. “ICT Adoption and Income Diversification among Rural Households in China.” Applied Economics 52 (33): 3614–3628. https://doi.org/10.1080/00036846.2020.1715338.
- López-Becerra, Erasmo I., Narciso Arcas-Lario, and Francisco Alcon. 2016. “The Websites Adoption in the Spanish Agrifood Firms.” Spanish Journal of Agricultural Research 14 (4), https://doi.org/10.5424/SJAR/2016144-10113.
- Martey, Edward, Prince M. Etwire, Desmond Sunday Adogoba, and Theophilus Kwabla Tengey. 2021. “Farmers’ Preferences for Climate-Smart Cowpea Varieties: Implications for Crop Breeding Programmes.” Climate and Development, https://doi.org/10.1080/17565529.2021.1889949.
- McCampbell, Mariette, Julius Adewopo, Laurens Klerkx, and Cees Leeuwis. 2021. “Are Farmers Ready to Use Phone-Based Digital Tools for Agronomic Advice? Ex-Ante User Readiness Assessment Using the Case of Rwandan Banana Farmers.” The Journal of Agricultural Education and Extension 29 (1): 29–51. https://doi.org/10.1080/1389224X.2021.1984955.
- Miranda, Marília Queiroz, Josivania Silva Farias, Carolina de Araújo Schwartz, and Juliana Pascualote Lemos de Almeida. 2016. “Technology Adoption in Diffusion of Innovations Perspective: Introduction of an ERP System in a Non-Profit Organization.” Revista de Administração e Inovação 13 (1): 48–57. https://doi.org/10.1016/J.RAI.2016.02.002.
- Munthali, Nyamwaya, Cees Leeuwis, Annemarie van Paassen, Rico Lie, Richard Asare, Ron van Lammeren, and Marc Schut. 2022. “Innovation Intermediation in a Digital Age: Comparing Public and Private New-ICT Platforms for Agricultural Extension in Ghana.” NJAS: Wageningen Journal of Life Science 86–87 (1): 64–76. https://doi.org/10.1016/J.NJAS.2018.05.001.
- Nonvide, Gbetondji Melaine Armel, Daniel B. Sarpong, George T.M. Kwadzo, Henry Anim-Somuah, and Fulbert Amoussouga Gero. 2018. “Farmers’ Perceptions of Irrigation and Constraints on Rice Production in Benin: A Stakeholder-Consultation Approach.” International Journal of Water Resources Development 34 (6): 1001–1021. https://doi.org/10.1080/07900627.2017.1317631.
- Ogwuike, Philomena Chioma-Akalugo, Aminou Arouna, and Clinton Obinna Ogwuike. 2021, June. “Assessment of Rice Threshing Technology Characteristics for Enhanced Rice Sector Development in Senegal.” African Journal of Science Technology Innovation and Development, 1–10. https://doi.org/10.1080/20421338.2021.1924424.
- Ojo, T. O., A. A. Adetoro, A. A. Ogundeji, and J. A. Belle. 2021. “Quantifying the Determinants of Climate Change Adaptation Strategies and Farmers’ Access to Credit in South Africa.” Science of The Total Environment 792 (October): 148499. https://doi.org/10.1016/j.scitotenv.2021.148499.
- Oliveira, Antonio, Carlos Resende, André Pereira, Pedro Madureira, João Gonçalves, Ruben Moutinho, Filipe Soares, and Waldir Moreira. 2020. “IoT Sensing Platform as a Driver for Digital Farming in Rural Africa.” Sensors 20 (12), https://doi.org/10.3390/S20123511.
- Orser, Barbara, Allan Riding, and Yanhong Li. 2019. “Technology Adoption and Gender-Inclusive Entrepreneurship Education and Training.” International Journal of Gender and Entrepreneurship 11 (3): 273–298. https://doi.org/10.1108/IJGE-02-2019-0026/FULL/PDF.
- Owusu, Alex Barimah, Paul W.K. Yankson, and Stephen Frimpong. 2017. “Smallholder Farmers’ Knowledge of Mobile Telephone Use: Gender Perspectives and Implications for Agricultural Market Development.” Progress in Development Studies 18 (1): 36–51. https://doi.org/10.1177/1464993417735389.
- Paudel, Krishna P., Ashok K. Mishra, Mahesh Pandit, and Eduardo Segarra. 2021. “Event Dependence and Heterogeneity in the Adoption of Precision Farming Technologies: A Case of US Cotton Production.” Computers and Electronics in Agriculture 181 (February), https://doi.org/10.1016/J.COMPAG.2020.105979.
- Porciello, Jaron, Sam Coggins, Edward Mabaya, and Gabriella Otunba-Payne. 2022. “Digital Agriculture Services in Low- and Middle-Income Countries: A Systematic Scoping Review.” Global Food Security 34 (September), https://doi.org/10.1016/J.GFS.2022.100640.
- Roberts, Roland K., Burton C. English, James A. Larson, Rebecca L. Cochran, W. Robert Goodman, Sherry L. Larkin, Michele C. Marra, Steven W. Martin, W. Donald Shurley, and Jeanne M. Reeves. 2004. “Adoption of Site-Specific Information and Variable-Rate Technologies in Cotton Precision Farming.” Journal of Agricultural and Applied Economics 36 (1): 143–158. https://doi.org/10.1017/S107407080002191X.
- Rodríguez-Sabiote, Clemente, José Álvarez-Rodríguez, Daniel Álvarez-Ferrandiz, and Félix Zurita-Ortega. 2021. “Using Chi-Squared Automatic Interaction Detection Modelling to Identify Student Opinion Profiles Regarding Same-Sex Couples as a Family Structure.” Heliyon 7 (3), https://doi.org/10.1016/J.HELIYON.2021.E06469.
- Rodríguez, Jhonn Pablo, Ana Isabel Montoya-Munoz, Carlos Rodriguez-Pabon, Javier Hoyos, and Juan Carlos Corrales. 2021. “IoT-Agro: A Smart Farming System to Colombian Coffee Farms.” Computers and Electronics in Agriculture 190 (November), https://doi.org/10.1016/J.COMPAG.2021.106442.
- Shang, Linmei, Thomas Heckelei, Maria K. Gerullis, Jan Börner, and Sebastian Rasch. 2021. “Adoption and Diffusion of Digital Farming Technologies - Integrating Farm-Level Evidence and System Interaction.” Agricultural Systems 190 (May), https://doi.org/10.1016/J.AGSY.2021.103074.
- Smidt, Hermanus Jacobus. 2021. “Factors Affecting Digital Technology Adoption by Small-Scale Farmers in Agriculture Value Chains (AVCs) in South Africa.” Information Technology for Development, https://doi.org/10.1080/02681102.2021.1975256.
- Soullier, Guillaume, Matty Demont, Aminou Arouna, Frédéric Lançon, and Patricio Mendez del Villar. 2020. “The State of Rice Value Chain Upgrading in West Africa.” Global Food Security 25 (June), https://doi.org/10.1016/j.gfs.2020.100365.
- Streletskaya, Nadia A., Sara Maruyama, Susan Queisser, Sheri Cole, Alina N. Stelick, and Juyun Lim. 2023. “How Information Leads Consumers to Select Specialty Foods When Tasting Is Not an Option.” Food Quality and Preference 105 (January), https://doi.org/10.1016/J.FOODQUAL.2022.104769.
- Suvedi, Murari, Raju Ghimire, and Michael Kaplowitz. 2017. “Farmers’ Participation in Extension Programs and Technology Adoption in Rural Nepal: A Logistic Regression Analysis.” The Journal of Agriculture Education and Extension 23 (4): 351–371. https://doi.org/10.1080/1389224X.2017.1323653.
- Sylla, Ahmed Yves, Ramatu Mahama Al-Hassan, Irene Susana Egyir, and Henry Anim-Somuah. 2019. “Perceptions about Quality of Public and Private Agricultural Extension in Africa: Evidence from Farmers in Burkina Faso.” Cogent Food & Agriculture 5 (1), https://doi.org/10.1080/23311932.2019.1685861.
- Teece, David J. 2018. “Business Models and Dynamic Capabilities.” Long Range Planning 51 (1): 40–49. https://doi.org/10.1016/j.lrp.2017.06.007.
- Thurstone, L. L. 1927. “A Law of Comparative Judgment.” Psychological Review 34 (4): 273–286. https://doi.org/10.1037/h0070288.
- Van Campenhout, Bjorn, David J. Spielman, and Els Lecoutere. 2021. “Information and Communication Technologies to Provide Agricultural Advice to Smallholder Farmers: Experimental Evidence from Uganda.” American Journal of Agricultural Economics 103 (1): 317–337. https://doi.org/10.1002/AJAE.12089.
- Vercillo, S., T. Weis, and I. Luginaah. 2020. “A Bitter Pill: Smallholder Responses to the New Green Revolution Prescriptions in Northern Ghana.” International Journal of Sustainable Development & World Ecology 27 (6): 565–575. https://doi.org/10.1080/13504509.2020.1733702.
- Vial, Annemiek, Claudia van der Put, Geert Jan J.M, Marc Dinkgreve Stams, and Mark Assink. 2021. “Validation and Further Development of a Risk Assessment Instrument for Child Welfare.” Child Abuse & Neglect 117 (July), https://doi.org/10.1016/J.CHIABU.2021.105047.
- Voss, Rachel C., Tony Jansen, Bacary Mané, Carol Shennan, Rachel C. Voss, Tony Jansen, Bacary Mané, and Carol Shennan. 2021. “Encouraging Technology Adoption Using ICTs and Farm Trials in Senegal: Lessons for Gender Equity and Scaled Impact.” World Development 146 (C), https://doi.org/10.1016/J.WORLDDEV.2021.105620.
- Waldman, Kurt B., David L. Ortega, Robert B. Richardson, and Sieglinde S. Snapp. 2017. “Estimating Demand for Perennial Pigeon Pea in Malawi Using Choice Experiments.” Ecological Economics 131 (January): 222–230. https://doi.org/10.1016/j.ecolecon.2016.09.006.
- Walisinghe, Buddhini Ranjika, Shyama Ratnasiri, Nicholas Rohde, and Ross Guest. 2017. “Does Agricultural Extension Promote Technology Adoption in Sri Lanka.” International Journal of Social Economics 44 (12): 2173–2186. https://doi.org/10.1108/IJSE-10-2016-0275/FULL/XML.
- Weber, Sylvain. 2019. “A Step-by-Step Procedure to Implement Discrete Choice Experiments in Qualtrics.” Social Science Computer Review 39 (5): 903–921. https://doi.org/10.1177/0894439319885317.
- Wolfert, Sjaak, Lan Ge, Cor Verdouw, and Marc Jeroen Bogaardt. 2017. “Big Data in Smart Farming – A Review.” Agricultural Systems 153 (May): 69–80. https://doi.org/10.1016/J.AGSY.2017.01.023.
- Wuepper, David, Nikolaus Roleff, and Robert Finger. 2021. “Does It Matter Who Advises Farmers? Pest Management Choices with Public and Private Extension.” Food Policy 99 (February): 101995. https://doi.org/10.1016/J.FOODPOL.2020.101995.
- Zossou, Espérance, Kazuki Saito, Alidou Assouma-Imorou, Kokou Ahouanton, and Bitrus Dawi Tarfa. 2020. “Participatory Diagnostic for Scaling a Decision Support Tool for Rice Crop Management in Northern Nigeria.” Development in Practice: 1–16. https://doi.org/10.1080/09614524.2020.1770699.
Appendices
Appendix A1. Sample of the dataset of two rice farmers
Appendix A2. Cronbach’s alpha test
Appendix A3. Pairwise correlation test
Pairwise correlations Group 1
Pairwise correlations Group 2