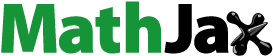
Abstract
Copper (Cu) nanoparticles (NPs) and polyvinyl alcohol (PVA) are utilized to enhance heat transfer (HT) which is used in the efficiency of solar energy systems. Copper nanoparticles have excellent thermal conductivity (TC) properties that enable them to conduct heat efficiently. In this arrangement, the gap between the two cylindrical channels is settled for the hybrid nanofluids (HNFs) flow in an inclined position that is favourable to sunlight. The nanomaterials consist of a mixture of PVA and Cu nanoparticles (NPs), to execute HNFs. Solar radiation is present on the hot side of the system. The porous gap between the two channels is considered variable which plays a crucial role in enhancing heat transfer and energy conversion. The varying permeability of the gap is adjusted to control the flow resistance and improve the stability of the system. It is observed that higher porosity allows for better convective heat transfer and reduced pressure drop. The transformed equations are solved through an artificial neural network (ANN) while the control volume finite element method (CVFEM) is also used to handle the governing equations. The Cu and PVA (HNF) improves solar radiation absorption and protects components, ultimately increasing the performance and efficiency of the systems.
1. Introduction
Nanofluids (NFs) are decisive in improving heat transfer (HT) in various applications, including solar systems. The process of creating nanofluids involves the dispersing of solid NPs (typically ranging from 1–100 nanometres) into conventional HT fluids, such as water or oil. Nanofluids are transferring heat more efficiently than conventional fluids due to the high thermal conductivity (TC) of nanoparticles (NPs).
Nanoparticles (NPs). dispersion in the base fluid improved the heat transfer (HT) rates, better heat dissipation, and reduced energy losses in solar systems (Baig et al., Citation2021; Manjunatha et al., Citation2019). Although nanofluids are well known, hybrid nanofluids (HNFs) were introduced about a decade ago. HNFs combine two categories of nanomaterials with regular fluids for more efficient HT. The stability and fabrication of nanofluids are determined largely by the chemical compositions of nanoscale materials and regular base fluids. The use of nanoliquid is required for a wide variety of applications, such as thermal engineering and manufacturing, microfluidics, nanofluidics, microelectronics, medical sciences, and many fields. However, nanoparticles have limitations to enhance the thermal efficiency based on their chemical and physical aspects. The researchers used two different kinds of materials in the same base fluid known as hybrid nanofluid (HNFs) to resolve this issue. HNFs have significant relevance in the solar system and heat transfer (HT) due to their unique properties and potential applications. A relative analysis of HNF progression condensed between two infinite and parallel plates as presented by Chu et al. (Said et al., Citation2022). They further demonstrated the impacts of magnetohydrodynamics and particles’ morphology. Dezfulizadeh et al. (Chu et al., Citation2022) analyzed the highly efficient behaviours of thermal shifts in a heat exchanger with the use of highly recommended HNF and MHD sources.
Gul et al. (Dezfulizadeh et al., Citation2021) observed that the Cu + Fe3O4 HNFs show pretty enhancement in the HT rate. Similarly, the performance of HNF using a mathematical model for channel flow can be seen in (Raza et al., Citation2019). Recently, Waini et al. (Gul et al., Citation2021) investigated the unsteady impingement of HNFs towards some permeable decelerating disk and concluded multiple solutions sequentially. The thermal transport rates through HNF considered with micropolarity, are observed by Gumber et al. (Waini et al., Citation2021) where they also studied the external ramifications of suction/injection along with radiative fluxes. Gul et al. (Gumber et al., Citation2022) examined the stagnation-point flow in the right-away vicinity of the rotating sphere. HNFs consisting of copper nanoparticles and PVA-based have been gaining attention in solar applications. Enhancing heat transfer and improving the efficiency of solar energy systems is achieved by using copper nanoparticles and polyvinyl alcohol (PVA).
Copper nanoparticles are capable of conducting heat efficiently due to their excellent thermal conductivity properties. The (HT) capability of the system is significantly enhanced when they are combined with HT fluids. Solar water heating systems or concentrated solar power systems require efficient HT from the solar collector to the working fluid, making this particularly useful.
Due to their high surface area to volume ratio, Cu nanoparticles are achieving better absorption and dispersion of solar radiation. Generally, the efficiency of the solar energy system is improved by the increased absorption of sunlight.
The properties of polyvinyl alcohol (PVA) are advantageous for solar energy applications. Being transparent to visible light and reflective to infrared radiation makes it suitable for use as a solar absorber material. By coating surfaces with PVA, solar radiation absorption is enhanced, leading to an increase in heating in solar thermal systems. In addition, PVA is utilized as an encapsulating material in solar panels to safeguard photovoltaic cells from moisture and other environmental influences while allowing light to enter the structure. The Cu and PVA-based hybrid nanofluids are mainly used in the enhancement of heat transfer as mentioned in (Aureen Albert et al., Citation2020; Gul et al., Citation2021; Shahzad et al., Citation2021).
Porous space is an efficient heat transfer source to use the nanofluid in terms of uniform temperature distribution. Optimal utilization of the captured solar energy is possible through porous space, which is particularly important for solar energy systems. The thermal performance of the system is improved by increasing the heat transfer efficiency in the presence of porous space (Chamkha, Citation1996; Chamkha, Citation1997; Haider et al., Citation2021; Saeed et al., Citation2021). However, the variable porous space concept is very rare but important for stable fluid distribution (Jarray et al., Citation2019). The variable porous space in hybrid nanofluid flow has significant importance in heat transfer enhancement and solar energy systems (Chamkha et al., Citation2002). The flow characteristics of the HNF are controlled by adjusting the porous space and are more prominent when the porous space is flexible (Haider et al., Citation2021). The variable porous structure's presence results in energy savings and improved efficiency by reducing pressure drops in the system.
In this regard, the work done by the researchers (Tlau & Ontela, Citation2022) to utilize the fluid flow according to the uniform fluid distribution for the enhancement of heat transfer (HT).
HT and solar systems have significant applications for HNF flow between the gap of two concentric cylinders as mentioned in (Tijani et al., Citation2023). The flow between concentric cylinders allows for increased surface area for heat transfer (HT), resulting in enhanced performance. The efficiency of solar thermal collectors is enhanced in solar systems by using HNF in the flow between concentric cylinders. The nanofluid's enhanced thermal properties make it easier to absorb solar radiation and transfer heat to the working fluid (Gouran et al., Citation2022). In application, HNFs have benefits such as improved stability and reduced corrosion, ensuring the system's long-term durability. The use of nanofluids in the gap between concentric cylinders is optimized through careful selection of nanoparticles, concentration, and flow conditions, to achieve the desired heat transfer efficiency and solar energy absorption (Peng et al., Citation2019; Sheikholeslami et al., Citation2017).
In the existing literature, the porous space is not used for the fluid flow between the gap of two cylinders. In the present analysis, the idea of porous and variable space is introduced to fill the research gap. After quite an extensive literature search, this paper is designated to discuss the attributes of hybrid nanofluid flow through a uniform gap between two concentric cylindrical structures. The newness of the study is pointed out as.
The two cylinders are placed in the inclined position that are favourable for solar radiations.
The medium between the two cylinders is kept porous and variable. In the existing literature, the porous medium used is usually constant. Therefore, this is the main extension of the proposed model.
The Cu and PVA materials are used in terms of hybrid nanomaterials. From the existing literature, it has been found that these materials are experimentally favourable for the solar system.
The CVFEM method (Dogonchi et al., Citation2019; Gul et al., Citation2023; Gul et al., Citation2023; Mohsenian et al., Citation2022; Mukhtar & Gul, Citation2023; Reddy, Citation2019) is used for the solution of the governing nonlinear partial differential equations.
The nonlinear ordinary differential equations are solved using the ANN (Ali et al., Citation2022; Alnahdi et al., Citation2024; Liu et al., Citation2021; Mishra et al., Citation2016; Öcal et al., Citation2021; Raja et al., Citation2022) strategy.
2. Materials and methods
water-based hybrid nano liquid is considered to flow through a gap of the concentric annulus. The characteristic radius of the inner cylinder is marked as while that of the outer one is taken
. Water is saturated with
nanocomposites. The geometry includes an inertial frame that involves a cylindrical coordinates system (
) axis is considered along axial reference while the radial line is denoted by the r−axis. Furthermore, the flow is assumed steady, laminar, incompressible, and solely induced by the inner cylinder, rotating with uniform angular velocity
. The velocity flow field is customized bi-directional while the temporal attributes are reported uni-directional, i.e. u = (vr,vϑ,0) along (
) directions, and T = T(r), respectively. Moreover, the flow is assumed to be sustained about radial line
, where i.e.
is treated constant and
. The solar radiations
emerging from the hot surface wall and transferred to the variable porous medium, along with the natural convection mode of heat transfer whose consequences are significantly incorporated. A physical demonstration of the expanse is shown in Figure .
In the view of above assumptions made and (Gouran et al., Citation2022; Haider et al., Citation2021; Tijani et al., Citation2023; Tlau & Ontela, Citation2022), the mathematical statements governing the hybrid nanofluid flow results as:
(1)
(1)
(2)
(2)
(3)
(3)
Physical conditions shall be adapted as follows:
(4)
(4)
Here, the subscripts used for the respective hybrid nano liquid and base liquid are and
, relatively. Also note that
, k and
are the absolute viscosity, conductivity, and thermal expansion coefficient, sequentially. Using the concept of Rossland's approximation, the radiative flux
is estimated like:
(5)
(5) where σ∗ represents the Stefan-Boltzman constant and κ∗ symbolizes the mean absorption coefficient. The thermophysical properties of prime interest are mentioned in Table , while their relations to mono and bi-hybrid nanofluid are given below.
(6)
(6)
Table 1. Materials’ thermal and physical properties.
3. Transformations
As implemented by (Gouran et al., Citation2022; Haider et al., Citation2021; Tijani et al., Citation2023; Tlau & Ontela, Citation2022), consider the following non-dimensional variables,
(7)
(7)
Under all the above considerations, equation. (1) is satisfied. However, equations. (2)−(4) transform into:
(8)
(8)
(9)
(9)
(10)
(10)
(11)
(11) whereas the dimensionless parameters are illustrated below.
(12)
(12)
The variable porous space terminology in Equation (9) is used from the existing literature (Chamkha et al., Citation2002; Haider et al., Citation2021; Tlau & Ontela, Citation2022).
Here and
, are used from the latest literature (Chamkha et al., Citation2002). The particle diameter
is affected by the empirical constants
, while ambient porosity is represented by
. The simulation purpose involves keeping the experimental values of embedding parameters
, fixed.
Relations for skin friction and Nusselt number are as follows.
(13)
(13)
With the help of Equation (13), Equation (14) revises so:
(14)
(14)
4. Solution methodologies
4.1. Control volume finite element method (CVFEM)
CVFEM is a hybrid approximation technique that implements the combined intuitions of control volume notion and finite element schemes. It divides a complex domain into some small, countable, and finite elements using the concept of discretization. The flow behaviour can be then specified by relatively simple equations. As far as accuracy, convergence, and stability are concerned, the finite element schemes are regarded as superior to other numerical techniques as mentioned in (Mohsenian et al., Citation2022; Mukhtar & Gul, Citation2023; Peng et al., Citation2019; Sheikholeslami et al., Citation2017).
CVFEM Limitations: CVFEM is computationally expensive, especially for large-scale simulations or intricate geometries. The method requires the discretization of the domain into control volumes and the solution of a set of governing equations for each control volume. This can lead to a large number of simultaneous equations, which increases the computational complexity of the method.
Similarly, CVFEM may not always provide the same accuracy as other numerical methods, such as finite element method (FEM) or finite difference method (FDM). The control volumes used in CVFEM are typically non-uniform and irregular, which can lead to interpolation errors and inaccuracies in the approximation of the solution.
4.2. Artificial neural networks (ANNs)
There are several frameworks for using (ANNs) to solve the differential equations that arise in the fluid flow models. The main steps are pointed out as.
Identify the variables and parameters that need to be predicted or solved in the first step.
The second step includes a dataset of input-output pairs, where the inputs are the variables and parameters related to the fluid flow model.
Train the ANN using the dataset. Divide the dataset into training and validation groups. The weights and biases of the ANN are modified iteratively with optimization algorithms such as gradient descent during training to minimize the difference between predicted outputs and actual outputs.
Evaluate the ANN model's performance by evaluating metrics such as mean squared error, root mean squared error or R-squared value. To achieve better performance, it may be necessary to adjust the model architecture or training parameters.
Evaluate the generalization capability of the trained ANN model against new, unseen data. Ensure accuracy and reliability by comparing the model's predictions with known solutions or experimental data.
If the model is not accurate or new data becomes available, refine it iteratively by repeating steps 2-5.
The development of the ANN model requires meticulous data optimization to ensure accurate predictions (Reddy, Citation2019). The ANN model training dataset has been thoughtfully partitioned to optimize the results. The testing phase takes 10%, validation takes another 10%, and the remaining 80% is used to train the ANN model to its maximum capability. The optimal number of neurons used in ANNs cannot be determined by a universal approach (Gul et al., Citation2023). This study proposed neural networks (NNs) to address the fluidic problem with a sigmoid activation function. Figure shows that the input values for the sigmoid activation function are within the range of 1 to 0. The Levenberg-Marquardt training algorithm, commonly known as LMTA, is a well-liked and widely used training method in the literature, with researchers frequently choosing it when designing ANN models (Dogonchi et al., Citation2019). The Hidden nodes are covered by the Tan-Sig function in this ANN model, while the output nodes are covered by the Purelin function (Gul et al., Citation2023). The following are the configurations for the transfer functions:
(15)
(15)
Analyzing their prediction performance is a critical next step after the development of ANN models. The R and MSE values (Ali et al., Citation2022) are computed using the formulas mentioned below.
(16)
(16)
(17)
(17)
The error between predicted and target data is an essential metric used to evaluate the effectiveness of ANN models, and it is used as the following relationship:
(18)
(18) The distribution of training, testing, and validation datasets in an Artificial Neural Network (ANN) is typically based on a ratio or percentage that is commonly used. One common distribution is 80% for training, 10% for testing, and 10% for validation. In this distribution, the training dataset consists of 80% of the total data available. It is used to train the ANN model by adjusting the weights and biases of the network based on the input data and expected output labels. The purpose of the training phase is to enable the network to learn patterns and relationships within the data. The testing dataset is used to evaluate the performance of the trained model. It represents 10% of the total data and is used to assess how well the model is generalized to unseen data. The testing phase assists in measuring the accuracy and performance of the ANN on data that it has not been exposed to during the training stage. The validation dataset also represents 10% of the total data and is used to fine-tune the model and prevent overfitting. Overfitting occurs when the ANN performs well on the training data but fails to generalize to new, unseen data. The validation dataset helps in optimizing the hyperparameters of the model by providing unbiased feedback on its performance. It is important to note that these percentages can vary depending on the specific problem, dataset size, and availability of data. The 80-10-10 distribution is a commonly used starting point but adjustable to suit the specific requirements of the problem at hand.
Limitation of ANN. ANNs are typically used for pattern recognition and prediction tasks, but ANNs do not provide insights into the causal relationships between variables. ANNs can make accurate predictions based on correlation, but they may not be useful for understanding and modeling causal relationships in complex systems. ANNs also struggle to generalize well to unseen or unfamiliar data.
5. Discussion
The role of an artificial neural network (ANN) in the study of the HNF flow between the gap of two cylinders is approached by the use of an autoencoder (AE), which is a specific type of neural network architecture. One of the main roles of an AE is to compress the input data. In the case of modelling the HNFs flow between the gap of two cylinders, various parameters affect the flow behaviour. An AE take these input parameters.
() and compress them into a lower-dimensional representation known as the latent space. This compression allows for better understanding and visualization of the flow behaviour. AE automatically learns and extracts relevant features from the input data. For the HNFs flow, there is a complex relationships and interactions between the parameters
that affect the flow behaviour. The AE can uncover these hidden features and represent them in the latent space. This helps in identifying important characteristics of the flow and understanding the physics behind it.
The geometry of the model problem is presented in Figure . All assumptions and flow structures are defined within the represented geometry Figure (a) while the grids are presented in Figure . (b). Here, the whole problem design and steps are presented and flow among the concentric cylinder is demonstrated for velocity and temperature profiles. The problem has been solved by (CVFEM) method. Figures are plotted using the ANNs and CVFEM strategies to find out the velocity and temperature distribution under the influence of various embedded parameters. Table shows the thermophysical properties of and water. The computations regarding ANNs strategy, Skin friction
, Nusselt number
for relating parameters are demonstrated in Tables . The hybrid nanofluids (HNFs) flow between two concentric cylinders is observed for the enhancement of heat transfer (HT) under the influence of solar radiations. The obtained results validation has been performed employing the deep neural network (DNN) strategy with the combination of the Bayesian regularization scheme (BRS) procedure. A detailed description of these strategies (DNN-BRS) is displayed in (Ali et al., Citation2022; Dogonchi et al., Citation2019; Gul et al., Citation2023; Gul et al., Citation2023; Reddy, Citation2019). The HNFs flow performance is also calculated through CVFEM and the validation through (DNN-BRS) is the main objective of this study. The DNN process was performed by introducing neurons into the primary and secondary hidden layers also applied to the known log-sigmoid function (LSF) and shown in Figure (a,b). The dataset is provided with the help of the Adam method, and this procedure is done by testing, training, and verification practices. The model problem is characterized by two parts including velocity field and temperature profile. The precision of the DNN-BRS solver is perceived by equating the standard and proposing solutions. Figure (a-f) illustrate how the parameters
, influence
field using Hybrid nanofluids in the presence of solar radiations. The thermal Grashof number improves the fluid velocity for its larger values as shown in Figure (a). Natural convection is essential for the inclined cylinders. The Grashof number can indicate the dominance of natural convection due to buoyancy forces, which is important for effective heat transfer. A higher Grashof number implies a greater contribution of buoyancy forces, leading to enhanced heat transfer. Physically, the Grashof number plays a role in determining the energy efficiency of solar energy systems utilizing hybrid nanofluids. By understanding the significance of the Grashof number, engineers and researchers can optimize system parameters to maximize energy conversion and output. This can involve considering factors such as the inclination angle of the cylinder and the choice of hybrid nanofluids with suitable thermophysical properties. The AE performance, MSE measures, DNNs-BRS strategy in terms of STs, the performance of the EHs, fitting curve, and performance of the Regression for the Grashof number (
) is shown in Figure (b to g). The AE performance is shown in Figure (b). The error approximations shown in this figure validate the obtained results. A neural network is trained to approximate a function by using mean squared error (MSE) as the loss function as shown in Figure (c). The MSE training activity for the parameter
in the proposed model for the heat transfer applications of the fluid flow between the two cylinders is precisely validated through calculation 1.07×
at epochs 221. The ‘num' (Mu) parameter in the gradient form under the influence of the convection parameter using a neural network in the case of hybrid nanofluids flow between two cylinders is displayed in Figure (d). The output in terms of the gradient is 9.956 ×
. In the artificial neural network, the error histogram (EH)is a crucial tool that provides crucial information about the distribution and magnitude of errors during the training phase. The ability to understand how well the network is learning and where the model may be struggling is provided for network designers and developers. The obtained results through EHs are obtained as 1.08 ×
, and shown in Figure (e). The evolution of ANNs is shaped by the fitness function, which drives optimization and ultimately determines the quality of solutions obtained. The fitness function is used to validate the proposed model of the fluid flow between the concentric cylinders through the DNNs-BRS strategy is displayed in Figure (f). The importance of regression results in terms of DNNs-BRS (Deep Neural Network-based Black-box Regression System) lies in the accuracy and reliability of the predictions made by the system. The regression results in terms of the DNNs-BRS for the model problem are shown in Figure (g). The obtained results validate the proposed model for the applications of heat transfer analysis through hybrid nanofluid flow among the concentric cylinders. The variable porous factor
has paramount significance upon the motion Cu + PVT + H2O that is been successfully plotted in (4(a)). The porous space acts as a medium for the flow of the nanofluid, facilitating the movement of the fluid through the gaps between the cylinders. The porous medium helps to distribute the nanofluid evenly across the cylinders’ surfaces, ensuring efficient heat transfer and maintaining a uniform temperature distribution. This is particularly important for solar energy systems, as it allows for optimal utilization of the captured solar energy. The small increase in the values of (
) controlling the fluid distribution and reducing the flow motion
. The AE figure for the porosity parameter
is shown in Figure (b). The MSE training activity for the parameter
in the proposed model for the heat transfer applications of the fluid flow between the two cylinders is precisely validate through calculation 1.523×
at epochs 224 is displayed in Figure (c). The ‘num' (Mu) parameter in the gradient form under the influence of variable porous space parameter using a neural network in the case of hybrid nanofluids flow between two cylinders is displayed in Figure (d). The output in terms of the gradient is 9.9218 ×
. The obtained result through EHs is 5.89 ×
, and shown in Figure (e). The fitness function is used to validate the proposed model of the fluid flow between the concentric cylinders in case of the variable porous space through the DNNs-BRS strategy is displayed in Figure (f). The regression results under the influence of the variable porous space parameter in terms of the DNNs-BRS for the model problem is shown in Figure (g).
Figure 3. (a-g): VS (a)
(b) AE performance, (c) MSE measures,(d) STs performance (e) EHs performance, (f) fitting curve, (g) performance of the Regression.

Figure 4. (a-g): VS (a)
(b) AE performance, (c) MSE measures,(d) STs performance (e) EHs performance, (f) fitting curve, (g) performance of the Regression.

Figure 5. (a-g): VS (a)
(b) AE performance, (c) MSE measures,(d) STs performance (e) EHs performance, (f) fitting curve, (g) performance of the Regression.
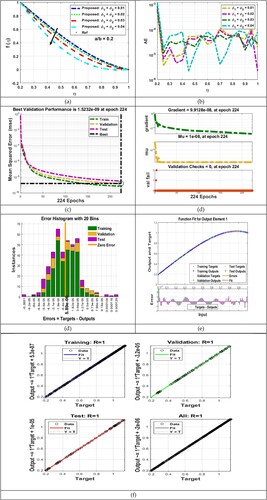
Figure 6. (a-g): VS (a)
(b) AE performance, (c) MSE measures,(d) STs performance (e) EHs performance, (f) fitting curve, (g) performance of the Regression.
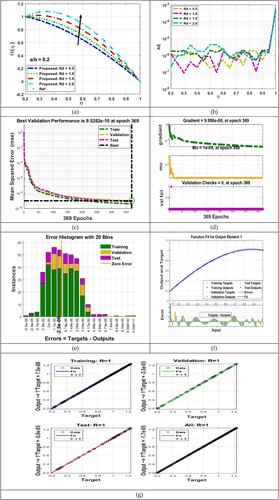
Figure 7. (a-g): VS (a)
(b) AE performance, (c) MSE measures,(d) STs performance (e) EHs performance, (f) fitting curve, (g) performance of the Regression.

Table 2. Outcomes of LMS-NNA for different scenarios of NCM-HMTMNFF-NNA.
Table 3. The skin friction outputs on the variations of the embedded parameters.
Table 4. The heat transfer rate versus .
The significance of (volume fraction) to
for Cu + PVT + H2O nanofluids is shown in Figure ((a)). The resistance produced due to the increasing values of
and consequently the fluid motion reduced. Physically, hybrid nanofluids are commonly used in solar thermal systems to transfer heat from solar collectors to heat storage systems or to directly heat fluids. The nanofluid viscosity rises as the volume fraction of nanoparticles increases. The solar system's energy capture efficiency can be improved by suppressing natural convection and reducing flow instabilities, which can lead to reduced convective heat losses in the fluid. The AE performance for the parameter
shown in Figure (b).
The MSE training activity for the parameter in the proposed model for the heat transfer applications of the fluid flow between the two cylinders is precisely validated through calculation 1.523×
at epochs 224 is displayed in Figure (c). The ‘num' (Mu) parameter in the gradient form under the influence of
using a neural network in the case of hybrid nanofluids flow between two cylinders is displayed in Figure (d). The output in terms of the gradient is 9.9218 ×
. The obtained result through EHs is 5.89 ×
, and shown in Figure (e) under the influence of
. The fitness function is used to validate the proposed model of the fluid flow between the concentric cylinders in the case of the variable porous space through the DNNs-BRS strategy is displayed in Figure (f). The regression results under the influence of the variable porous space parameter in terms of the DNNs-BRS for the model problem are shown in Figure (g). Figure (a-g)) frame the sundry outcomes in dimensional temperature opposite to varying values of model parameters Rd and
, sequentially, with Cu + PVA + H2O nanofluids. Figure ((a)) shows that increasing the radiation parameter improves the thermal profile and this impact is more effective for Cu + PVT + H2O nanofluid. The temperature field is significantly influenced by the solar radiation parameter when using hybrid nanofluids made of Copper and PVA materials. The nanofluids absorb more solar energy when the solar radiation parameter is elevated. As more energy is transferred to the system, the temperature of nanofluids increases. The high thermal conductivity of copper and PVA materials allows them to effectively absorb and transfer heat. This has the potential to be advantageous in applications like solar thermal systems or solar water heaters, where higher temperatures are needed for efficient heat transfer. Figure ((b)) shows the AE performance under the influence of
.
The MSE training activity for the parameter in the proposed model for the heat transfer applications of the fluid flow between the two cylinders is precisely validated through calculation 9.528×
at epochs 369 is displayed in Figure (c). The ‘num' (Mu) parameter in the gradient form under the influence of
using a neural network in the case of hybrid nanofluids flow between two cylinders is displayed in Figure (d). The output in terms of the gradient is 9.956 ×
. The obtained result through EHs is 2.5 ×
, and shown in Figure (e) under the influence of
. The fitness function is used to validate the proposed model of the fluid flow between the concentric cylinders in the case of the variable porous space through the DNNs-BRS strategy is displayed in Figure (f). The regression results under the influence of the variable porous space parameter in terms of the DNNs-BRS for the model problem are shown in Figure (g).
The impact of on
is estimated in Figure ((a)). For Cu + PVT + H2O nanofluids, the incorporation of
has a significant impact on the thermophysical aspects which are considered. After a comparison is made for the preceding nano additives, the thermo-physical features of nanofluid, knf and µnf, significantly improve. The double characteristic is explained by the dynamics of the volume fraction of nanoparticles for various nanofluids. Nanoparticles, such as Copper nanoparticles, have high thermal conductivity compared to the base fluid. Increasing the nanoparticle volume fraction in the hybrid nanofluid enhances the heat transfer by facilitating better thermal conduction throughout the fluid. This improved heat transfer ensures efficient energy absorption by the PVT material, leading to enhanced conversion efficiency and overall performance of the solar system. Physically, hybrid nanofluids for solar applications depend heavily on the nanoparticle volume fraction. It affects heat transfer, optical properties, stability, fluid properties, and cost-effectiveness. Optimizing the volume fraction can help maximize energy conversion efficiency and ensure the long-term performance and reliability of solar energy conversion systems. Figure (b) shows the AE performance for the error estimation under the influence of
on
. The MSE training activity for the parameter
in the proposed model for the heat transfer applications of the fluid flow between the two cylinders is precisely validated through calculation 1.523×
at epochs 224 is displayed in Figure (c). The ‘num' (Mu) parameter in the gradient form under the influence of
using a neural network in the case of hybrid nanofluids flow between two cylinders is displayed in Figure (d). The output in terms of the gradient is 9.921×
. The obtained result through EHs is 5.89 ×
, and shown in Figure (e) under the influence of
. The fitness function is used to validate the proposed model of the fluid flow between the concentric cylinders in the case of the variable porous space through the DNNs-BRS strategy is displayed in Figure (f). The regression results under the influence of the variable porous space parameter in terms of the DNNs-BRS for the model problem are shown in Figure (g). The heating and cooling capacity of Cu + PVA is enhanced as the value of
increases. Observing the overall patterns between the concentric cylinder, the flow distribution upshots in contour form as shown in Figure ((a)). The fluid flow is comparatively uniform in front of the solar radiations while the fluid flow in the opposite side of the radiations is affected by the porous space. Similarly, the highly porous space is dense and the flow of fluid becomes difficult, as shown in Figure ((b)). However, for higher thermal performance this medium is more prominent to keep the fluid hotter for a long time. The close view of the fluid flow in small porous and larger porous space are shown in Figure ((c)) and (8(d)). These are the faces of cylinders that are favourable for solar radiation. Again, the Hybrid nanofluid flow is uniform in the first case due to the small amount of porous space while the flow of fluid is reduced due to the higher resistivity of the porous space. Copper nanoparticles and PVA can be used to enhance heat transfer and efficiency of solar energy systems. Heat transfer fluids or coatings can significantly enhance the system's heat transfer capability. The efficiency of heat transfer from the solar collector to the working fluid is crucial for solar water heating systems or concentrated solar power systems, making this particularly useful. Due to their high surface area to volume ratio, copper nanoparticles can achieve better absorption and dispersion of solar radiation. The enhancement in heat transfer rate with the variation of particle volume fraction is shown in Figure (a). Also, it is observed that hybrid nanofluid is more prominent in the enhancement of heat transfer. The % %-wise enhancement in the heat transfer is shown in Figure (b). The output shows that hybrid nanofluid is favourable for heat transfer.
5.1. Analyzing skin friction and heat transfer.
Table contains computational values for different factors in ANN models. The table includes distinct computational values for MSE (training, validation, and testing), as well as performance, gradient, and mu, across different epochs (1000, 526, 430, 890, 1000, 1000) for the model problem.
Here, the dimensionless skin-friction coefficient Cf is plotted against different values of, in Table . The outputs demonstrate constructive mannerisms with increasing
flow rates at the inner-most surface wall, whereas contrary natures concerning momentum boundary layers are portrayed at the outer cylinder. Table summarizes the significant efforts of non-dimensional Nusselt number Nu vs variations in vigorous related parameters. Water shows pretty improvements in heat transfer rates under the incorporation of volume fraction of both mono/hybrid nanoparticles and installation of the variable porous medium and thermal radiation. However, it is notorious that these constructive impacts for the temporal conduction processes are enhanced when involving Cu + PVA + H2O hybrid nano liquid. Note that all the above discussions are acceptable in light of physical laws relating to fluid mechanics and thermodynamics (Table ).
Table 5. The parameters values range as per stability analysis.
The range of values for the parameters in water-based hybrid nanofluids of Cu and PVA nanomaterials flowing between two cylinders varies depending on many factors such as the desired flow conditions, stability of the system, and HNFs composition as shown in Table . However, here are some approximate ranges based on the stability analysis and existing literature. The Prandtl number is a dimensionless parameter that represents the ratio of momentum diffusivity to thermal diffusivity. For water, its Prandtl number is around 6.9. The addition of Cu and PVA nanomaterials may slightly alter the Prandtl number depending on their influences on the thermal and momentum diffusivities. Generally, the Prandtl number can range from 2 to 15 for HNFs. The porous space parameter represents the proportion of the porous region (such as a porous medium) in the flow domain. It is usually defined as the ratio of the porous region volume to the total volume of the flow domain. The range for this parameter varies widely depending on the specific computational setup. According to the stability of the system it ranges from 0 (no porous region) to 1 (the entire flow domain is porous). The radiation parameter represents the importance of radiation heat transfer in the system. For water-based nanofluids, the radiation heat transfer is typically not significant unless high temperatures or special conditions are involved. Therefore, the radiation parameter is usually assumed small. However, if radiation heat transfer is significant, the radiation parameter ranges from 0 to a higher value depending on the radiation properties of the HNFs and the temperatures involved. Similarly, the Grashof number and nanoparticle volume fraction range for the recent model based on the HNFs and stability analysis are (1 to 7), and (0.01 to 0.05) respectively. By comparing the present work with existing literature, similarities and differences are highlighted in Cu and PVA hybrid nanofluids (HNFs) flow between the gap of two cylinders shown in Table . A closed agreement has been obtained between the present work and existing literature (Gouran et al., Citation2022).
Table 6. Based on the common parameter of gap between two cylinders the current work is compared with (Gouran et al., Citation2022).
6. Conclusions
The main findings of this study are highlighted below. The hybrid nanofluids flow between the concentric cylinders is considered for the applications of heat transfer. The PDE solution is obtained through the CVFEM technique. The ANN method has been used to find the solution to the system of ODE. The dataset is numerically provided through the Adam method by using the procedure of training, testing, and verification with 14, 12, and 74 percentages. The correctness of the DNN-BRS solver is observed through the comparison of the reference and proposed solutions, which is performed in good agreement for each case of the mathematical model. The outcomes of the physical parameters are displayed here.
Validation of the obtained results through error analysis using the square residual error, testing, and training is achieved using the neural network strategy.
With increasing Da, the skin friction at the boundaries increases, though the pattern reverses for escalating Gr.
It has been observed that the variable porous space (Da) in hybrid nanofluid flow has significant significance in enhancing heat transfer and solar energy systems. Enhancing heat transfer rates, increasing thermal conductivity, allowing for heat storage, allowing for flow control, and reducing pressure drops are all benefits of the variable porous space.
The temperature field is significantly influenced by the solar radiation parameter (Rd) when using hybrid nanofluids made of Copper materials and PVA. The nanofluids absorb more solar energy when the solar radiation parameter is elevated.
The present analysis is compared with the existing literature and validated.
In conclusion, the use of copper nanoparticles and PVA materials in heat transfer and solar energy systems can enhance heat transfer efficiency. The Cu and PVA hybrid nanofluid improves solar radiation absorption and protects components, ultimately increasing the overall performance and efficiency of the systems.
The same model is extendable using the non-Newtonian fluids for the blood flow.
The same model is also a best choice to use for the tri-hybrid nanofluids flow for the heat transfer applications.
Electric field, Magnetic field, Couple stresses extensions are also lies in the future work.
Acknowledgment
The authors extend their appreciation to the Deanship of Research and Graduate Studies at University of Tabuk for funding this work through Research no. 0173-1444-S.
Disclosure statement
No potential conflict of interest was reported by the author(s).
Data availability statement
Data will be available on a reasonable request.
Additional information
Funding
References
- Ali, A., Ahammad, N. A., Tag-Eldin, E., Gamaoun, F., Daradkeh, Y. I., & Yassen, M. F. (2022). MHD Williamson nanofluid flow in the rheology of thermal radiation, Joule heating, and chemical reaction using the Levenberg–Marquardt neural network algorithm. Frontiers in Energy Research, 1175, 1–23.
- Alnahdi, A. S., Khan, A., Gul, T., & Ahmad, H. (2024). Stagnation point nanofluid flow in a variable darcy space subject to thermal convection using artificial neural network technique. Arabian Journal for Science and Engineering, 1–18.
- Aureen Albert, A., Harris Samuel, D. G., Parthasarathy, V., & Kiruthiga, K. (2020). A facile one pot synthesis of highly stable PVA–CuO hybrid nanofluid for heat transfer application. Chemical Engineering Communications, 207(3), 319–330. https://doi.org/10.1080/00986445.2019.1588731
- Baig, N., Kammakakam, I., & Falath, W. (2021). Nanomaterials: A review of synthesis methods, properties, recent progress, and challenges. Advanced Materials, 2(6), 1821–1871. https://doi.org/10.1039/D0MA00807A
- Chamkha, A. J. (1996). Non-Darcy hydromagnetic free convection from a cone and a wedge in porous media. International Communications in Heat and Mass Transfer, 23(6), 875–887. https://doi.org/10.1016/0735-1933(96)00070-X
- Chamkha, A. J. (1997). Non-Darcy fully developed mixed convection in a porous medium channel with heat generation/absorption and hydromagnetic effects. Numerical Heat Transfer, Part A Applications, 32(6), 653–675. https://doi.org/10.1080/10407789708913911
- Chamkha, A. J., Issa, C., & Khanafer, K. (2002). Natural convection from an inclined plate embedded in a variable porosity porous medium due to solar radiation. International Journal of Thermal Sciences, 41(1), 73–81. https://doi.org/10.1016/S1290-0729(01)01305-9
- Chu, Y. M., Bashir, S., Ramzan, M., & Malik, M. Y. (2022). Model−based comparative study of magnetohydrodynamics unsteady hybrid nanofluid flow between two infinite parallel plates with particle shape effects. Mathematical Methods in the Applied Sciences, 11568–11582.
- Dezfulizadeh, A., Aghaei, A., Hassani Joshaghani, A., & Najafizadeh, M. M. (2021). Exergy efficiency of a novel heat exchanger under MHD effects filled with water-based Cu–SiO2−MWCNT ternary hybrid nanofluid based on empirical data. Journal of Thermal Analysis and Calorimetry, 147(7), 4781–4804. https://doi.org/10.1007/s10973-021-10867-3
- Dogonchi, A. S., Chamkha, A. J., & Ganji, D. D. (2019). A numerical investigation of magneto-hydrodynamic natural convection of Cu–water nanofluid in a wavy cavity using CVFEM. Journal of Thermal Analysis and Calorimetry, 135(4), 2599–2611. https://doi.org/10.1007/s10973-018-7339-z
- Gouran, S., Mohsenian, S., & Ghasemi, S. (2022). Theoretical analysis on MHD nanofluid flow between two concentric cylinders using efficient computational techniques. Alexandria Engineering Journal, 61(4), 3237–3248. https://doi.org/10.1016/j.aej.2021.08.047
- Gul, T., Alharbi, S. O., Khan, I., Khan, M. S., & Alzahrani, S. (2023). Comparative analysis of the flow of the hybrid nanofluid stagnation point on the slippery surface by the CVFEM approach. Alexandria Engineering Journal, 76, 629–639. https://doi.org/10.1016/j.aej.2023.06.025
- Gul, T., Ali, B., Alghamdi, W., Nasir, S., Saeed, A., Kumam, P., & Jawad, M. (2021). Mixed convection stagnation point flow of the blood based hybrid nanofluid around a rotating sphere. Scientific Reports, 11(1), 1–15. https://doi.org/10.1038/s41598-020-79139-8
- Gul, T., Bilal, M., Alghamdi, W., Asjad, M. I., & Abdeljawad, T. (2021). Hybrid nanofluid flow within the conical gap between the cone and the surface of a rotating disk. Scientific Reports, 11(1), 1–19. https://doi.org/10.1038/s41598-020-79139-8
- Gul, T., Nasir, S., Berrouk, A. S., Raizah, Z., Alghamdi, W., Ali, I., & Bariq, A. (2023). Simulation of the water-based hybrid nanofluids flow through a porous cavity for the applications of the heat transfer. Scientific Reports, 13(1), 7009. https://doi.org/10.1038/s41598-023-33650-w
- Gumber, P., Yaseen, M., Rawat, S. K., & Kumar, M. (2022). Heat transfer in micropolar hybrid nanofluid flow past a vertical plate in the presence of thermal radiation and suction/injection effects. Partial Differential Equations in Applied Mathematics, 5, 100240. https://doi.org/10.1016/j.padiff.2021.100240
- Haider, F., Hayat, T., & Alsaedi, A. (2021). Flow of hybrid nanofluid through Darcy-Forchheimer porous space with variable characteristics. Alexandria Engineering Journal, 60(3), 3047–3056. https://doi.org/10.1016/j.aej.2021.01.021
- Jarray, A., Mehrez, Z., & El Cafsi, A. (2019). Mixed convection Ag-MgO/water hybrid nanofluid flow in a porous horizontal channel. The European Physical Journal Special Topics, 228(12), 2677–2693. https://doi.org/10.1140/epjst/e2019-900068-8
- Liu, T., Li, Y., Jing, Q., Xie, Y., & Zhang, D. (2021). Supervised learning method for the physical field reconstruction in a nanofluid heat transfer problem. International Journal of Heat and Mass Transfer, 165, 120684. https://doi.org/10.1016/j.ijheatmasstransfer.2020.120684
- Manjunatha, S., Kuttan, B. A., Jayanthi, S., Chamkha, A., & Gireesha, B. J. (2019). Heat transfer enhancement in the boundary layer flow of hybrid nanofluids due to variable viscosity and natural convection. Heliyon, 5(4), https://doi.org/10.1016/j.heliyon.2019.e01469
- Mishra, S. R., Baag, S., & Mohapatra, D. K. (2016). Chemical reaction and Soret effects on hydromagnetic micropolar fluid along a stretching sheet. Engineering Science and Technology, an International Journal, 19(4), 1919–1928. https://doi.org/10.1016/j.jestch.2016.07.016
- Mohsenian, S., Gouran, S., & Ghasemi, S. E. (2022). Evaluation of weighted residual methods for thermal radiation on nanofluid flow between two tubes in presence of magnetic field. Case Studies in Thermal Engineering, 32, 101867. https://doi.org/10.1016/j.csite.2022.101867
- Mukhtar, S., & Gul, T. (2023). Solar radiation and thermal convection of hybrid nanofluids for the optimization of solar collector. Mathematics, 11(5), 1175. https://doi.org/10.3390/math11051175
- Öcal, S., Gökçek, M., Çolak, A. B., & Korkanç, M. (2021). A comprehensive and comparative experimental analysis on thermal conductivity of TiO 2-CaCO 3/Water hybrid nanofluid: Proposing new correlation and artificial neural network optimization. Heat Transfer Research, 52(17), https://doi.org/10.1615/HeatTransRes.2021039444
- Peng, Y., Alsagri, A. S., Afrand, M., & Moradi, R. (2019). A numerical simulation for magnetohydrodynamic nanofluid flow and heat transfer in rotating horizontal annulus with thermal radiation. RSC Advances, 9(39), 22185–22197. https://doi.org/10.1039/C9RA03286J
- Raja, M. A. Z., Shoaib, M., Khan, Z., Zuhra, S., Saleel, C. A., Nisar, K. S., & Khan, I. (2022). Supervised neural networks learning algorithm for three dimensional hybrid nanofluid flow with radiative heat and mass fluxes. Ain Shams Engineering Journal, 13(2), 101573. https://doi.org/10.1016/j.asej.2021.08.015
- Raza, J., Mebarek-Oudina, F., & Chamkha, A. J. (2019). Magnetohydrodynamic flow of molybdenum disulfide nanofluid in a channel with shape effects. Multidiscipline Modeling in Materials and Structures, 15(4), 737–757. https://doi.org/10.1108/MMMS-07-2018-0133
- Reddy, J. N. (2019). Introduction to the finite element method. McGraw-Hill Education.
- Saeed, A., Jawad, M., Alghamdi, W., Nasir, S., Gul, T., & Kumam, P. (2021). Hybrid nanofluid flow through a spinning Darcy–Forchheimer porous space with thermal radiation. Scientific Reports, 11(1), 16708. https://doi.org/10.1038/s41598-021-95989-2
- Said, Z., Sundar, L. S., Tiwari, A. K., Ali, H. M., Sheikholeslami, M., Bellos, E., & Babar, H. (2022). Recent advances on the fundamental physical phenomena behind stability, dynamic motion, thermophysical properties, heat transport, applications, and challenges of nanofluids. Physics Reports, 946, 1–94.
- Shahzad, F., Jamshed, W., Nisar, K. S., Khashan, M. M., & Abdel-Aty, A. H. (2021). Computational analysis of Ohmic and viscous dissipation effects on MHD heat transfer flow of Cu-PVA Jeffrey nanofluid through a stretchable surface. Case Studies in Thermal Engineering, 26, 101148. https://doi.org/10.1016/j.csite.2021.101148
- Sheikholeslami, M., Nimafar, M., & Ganji, D. (2017). Nanofluid heat transfer between two pipes considering Brownian motion using AGM. Alexandria Engineering Journal, 56(2), 277–283. https://doi.org/10.1016/j.aej.2017.01.032
- Tijani, Y. O., Akolade, M. T., & Mohd Kasim, A. R. (2023). Transport features on bidirectional nanofluid flow with convective heating and variable darcy regime. Journal of Computational and Theoretical Transport, 1–20.
- Tlau, L., & Ontela, S. (2022). Entropy analysis of hybrid nanofluid flow in a porous medium with variable permeability considering isothermal/isoflux conditions. Chinese Journal of Physics, 80, 239–252. https://doi.org/10.1016/j.cjph.2022.10.001
- Waini, I., Ishak, A., & Pop, I. (2021). Multiple solutions of the unsteady hybrid nanofluid flow over a rotating disk with stability analysis. European Journal of Mechanics-B/Fluids, 94, 121–127. https://doi.org/10.1016/j.euromechflu.2022.02.011