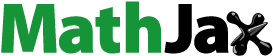
Abstract
Measles is a highly contagious disease that mainly affects children worldwide. Even though a reliable and effective vaccination is available, there were 140,000 measles deaths worldwide in 2018, and most of them were children under the age five years. In this paper, we comprehensively investigate a novel fractional SVEIR (Susceptible-Vaccinated-Exposed-Infected-Recovered) model of the measles epidemic powered by nonlinear fractional differential equations to understand the epidemic’s dynamical behaviour. We use a non-singular Atangana-Baleanu fractional derivative to analyze the proposed model, taking advantage of non-locality. The existence, uniqueness, positivity and boundedness of the solutions are shown via concepts of fixed point theory, and we also perform the Ulam-Hyers stability of the considered model. The parameter sensitivity is discussed in the context of the variance with each parameter using 3-D graphics based on the basic reproduction number. Moreover, with the Atangana-Toufik numerical scheme, numerical findings are depicted for different fractional-order values. The presented approach produce results that are efficiently consistent and in excellent agreement with the theoretical results.
1. Introduction
Measles is also named as morbilli or rubeola, is an infectious disease resulted by the Morbillivirus, a species of the Paramyxoviridae family. It specifically targets children under five and has a significant fatality rate. The measles virus is vaccine-preventable, yet the World Health Organization (WHO) still considers this disease to be a public health issue (Budigan Ni et al., Citation2023; Center of Disease Control and Prevention, n.d; Gambrell, Sundaram, & Bednarczyk, Citation2022). Disease reported the lives of over 110,000 persons in 2017 especially young children (James Peter, Ojo, Viriyapong, & Abiodun Oguntolu, Citation2022). presents the ten states that the disease outbreak has most profoundly influenced. The number of confirmed cases of measles in 2019 was, interestingly, the most in the previous two decades. Meantime, measles outbreaks have been highlighted in 2019 in many nations, including Angola, Cameroon, Sudan, Nigeria, Chad, Congo, Madagascar, and South Sudan in Africa, Kazakhstan in Central Asia, the Philippines and Thailand in Southeast Asia, and Ukraine in Eastern Europe (Callister, Citation2019). In 182 countries, there were 364,811 cases reported in the first quarter of 2019, as stated in the WHO statistics. Moreover, massive increase in measles cases were seen in the Western Pacific, European and African zones (Jost, Luzi, Metzler, Miran, & Mutsch, Citation2015).
Table 1. List of top five nations with worldwide measles outbreaks Equation(7)(7)
(7) .
The first flu-like measles symptoms occur 7 to 14 days after the first virus contacts the human host. Within two to five days of the initial symptoms, skin rashes and Koplik spots within the mouth of the influenced person happen, and the rashes then diffuse to the rest of the body. This viral infection spreads with the signs of high fever, rash development, cough, a runny nose, red and watery eyes. Approximately one-third of all diagnosed cases are expected to have significant consequences, such as pneumonia, acute encephalitis, and common problems like diarrhoea and skin infections (Battegay, Itin, & Itin, Citation2012; Gould, Citation2015). Furthermore, respiratory droplets caused by sneezing and coughing, direct communication with contaminated noses or throat secretions and close physical contact spread the measles virus from person to person (Angelo et al., Citation2019). Policies corresponding to vaccination play an essential role in preventing the human host from disease. Many vaccinations have been made to protect hosts from widespread infections in certain areas (Ilesanmi, Adeyinka, & Olakunde, Citation2022; Vojtek, Larson, Plotkin, & Van Damme, Citation2022). It is accurate to say that some of these vaccinations are expensive, some have adverse effects, and none are entirely adequate. It is also claimed that the development of a low-cost, beneficial, and safe vaccine, coupled with faster immunization programs has led to an 80% reduction in measles-related moralities, particularly in industrialized nations (Fisker et al., Citation2022).
To develop practical mitigation actions to eliminate and prevent measles, policymakers conducted a wide range of studies. Quantitative methodologies are required to assess the cost-effectiveness and effect of these activities in order to enhance them in the future (Ain & Wang, Citation2023; Pokharel, Adhikari, Gautam, Uprety, & Vaidya, Citation2022). Mathematical modelling has greatly aided in visualizing, studying, and comprehending the transmission mechanism of several diseases (Khan & Atangana, Citation2022; Raza, Arshed, Bakar, Shahzad, & Inc, Citation2023). Moreover, these models enable efficient control policies for the determent of future infection. The mathematical analysis of these models provides vital findings of infection and estimates future consequences that are hard to quantify under other conditions (Abbasi, Zamani, Mehra, Shafieirad, & Ibeas, Citation2020; Khan, Ullah, Ali, & Zaman, Citation2019; Tyagi, Gupta, Abbas, Das, & Riadh, Citation2021). Furthermore, for some recent and interesting results related to nonlinear fractional boundary value problems, fractional integro-differential equations, tuberculosis model using different fractional derivatives, etc. see (Batool, Talib, Riaz & Tunç, Citation2022; Bohner, Tunç & Tunç, Citation2021; Graef, Tunç & Şevli Citation2021; Tunç, Tunç &Yao, 2021; Tunç & Tunç, Citation2023; Zafar, Zaib, Hussain, Tunç & Javeed, Citation2022). Numerous epidemic models have been constructed to better explain the biological mechanism of measles disease outbreak (Ochoche & Gweryina, Citation2014; Sowole, Ibrahim, Sangare, & Lukman, Citation2020). It is also used to analyze the effectiveness of health care initiatives and indicate the ideal plan of action for fighting measles, including how to utilize the vaccination efficiently. The literature (Liu, Ikram, Khan, & Din, Citation2022; Memon, Qureshi, & Memon, Citation2020) uses various measles models for real-world data to forecast disease transmission and control.
The fact that fractional order models (FOMs) provide more proficient, in-depth, reliable, and valuable information concerning the dynamics of many diseases than traditional models is evident. Its hereditary features and memory characterization make it special to traditional models (Ain, Khan, Abdeljawad, Gómez-Aguilar, & Riaz, Citation2024; Ain et al., Citation2022; Alzahrani & Khan, Citation2020; Baishya, Achar, Veeresha, & Prakasha, Citation2021; Raza, Bakar, Khan, & Tunç, Citation2022; Singh, Kumar, Hammouch, & Atangana, Citation2018; Tao, Anjum, & Yang, Citation2023). FOMs make it easier to investigate and illustrate the dynamics between two non-local locations. Several concepts and theories have been presented and established regarding fractional order derivatives (Uchaikin, Citation2013; Yang, Citation2019). In Ref. (Samko, Kilbas, & Marichev, Citation1993) the essential concept and principle of the fractional calculus are presented. Fractional derivatives have been proven to be an excellent tool for simulating real-world issues in various fields, including engineering, physics, economics, and biology. FOMs offer more reliable, accurate, and consistent insights into the dynamics of disease resulting from a biological system (Anjum, Ain, & Li, Citation2021; Anjum, He, & He, Citation2021; Butt, Ahmad, Rafiq, & Baleanu, Citation2022; Conlan, Rohani, Lloyd, Keeling, & Grenfell, Citation2010; Farman, Saleem, Ahmad, & Ahmad, Citation2018; Qureshi & Jan, Citation2021). The researchers in Ref. (Butt, Ahmad Saqib, Alshomrani, Bakar, & Inc, Citation2024) discuss the dynamic characteristics of the fractional cervical cancer system, along with the sensitivity of the basic reproduction number. To compare the outcomes of the suggested model with the integer-order model, Abboubakkar et al. Abboubakar, Fandio, Sofack, and Ekobena Fouda, (Citation2022) designed a mathematical model for measles employing the Caputo derivative. The study in Ref. (Nabti & Ghanbari, Citation2021) examines an SVEIR measles fractional framework, demonstrating the existence and uniqueness of the measles fractional model while dividing the population into five subcategories. Ahmad et al. (Citation2024) have utilized vaccination impact as a control strategy for the dynamics of COVID-19. In their work to prevent Hepatitis disease, Ain and Chu (Citation2004) studied the impact of vaccines in the Hepatitis model.
In this study, we employ a fractional system to elucidate the dynamics of measles transmission in the presence of vaccination, serving as the conceptual foundation for the preceding discussion. We also evaluated and assessed the effect of different parameters of this model on the results of the basic reproduction number in order to identify the most important aspects of disease control and prevention. Also noteworthy is that the measles model presented here is a newly developed model that has been examined for the first time in the context of the current research using the ABC fractional operator. Moreover, some new research studies either overlook the significance of sensitivity analysis of or do so by analyzing local forward sensitivity indices to figure out the critical epidemiological model parameters that affect
For the numerical solution, we use the Atangana-Toufik method (ATM) from the literature (Toufik & Atangana, Citation2017).
The remaining article is further structured into the following sections: Section 2 presented some fundamental results after developing a dynamical system for measles spread within the environment of both traditional and fractional derivatives. In Section 3, we investigated the suggested model for the qualitative analysis, including the existence, uniqueness, and positivity of the solution for the proposed model. The dynamical aspect of the presented model, such as stability analysis, and the fundamental reproduction number is examined and computed. In Section 4, a sensitivity analysis of
is performed. To understand the dynamics of the suggested model with the ABC derivative operator, a numerical approach with illustrations is introduced in Section 5. Section 6 summarizes the stated research work and offers future prospects.
2. Measles model with the ABC derivative
We consider a mathematical model with five categories for the spread of measles disease in a particular region based primarily on individuals status. Following are the epidemiological classes into which the compartments are classified. Susceptible humans who are at risk or susceptible to acquiring measles, vaccinated humans
who have received a measles vaccination, exposed humans
who have been revealed symptoms of measles, infected humans
who have measles and are transmittable, and recovered humans
who have measles and have been naturally healed. For those in this class, the body’s immunity becomes permanently resilient to the ailment after the cure, preventing further infection and recurrence. It is also assumed that there is no infection after the recovery and the total population will remain constant at any time. provide a pictorial illustration of the model.
Therefore, the following system describes the dynamics of measles transmission in the human population can be expressed as:
(1)
(1)
The system described in Equation(1)(1)
(1) has already been studied in Ref. (Peter, Qureshi, Ojo, Viriyapong, & Soomro, Citation2022). Besides that, this model does not account for the memory effects, which are found in several biological models. To expedite the reduction of measles prevalence, this methodology aims to enhance both diagnosis and therapy for individuals exposed to the disease. Therefore, we modify the framework by replacing the traditional derivative with the recently proposed ABC fractional derivative, permitting the model to account for memory characteristics. Let us first construct the fractional illustration of the considered system by using the ABC operator:
(2)
(2)
with the following initial constraints
where
and
signifies the ABC derivative of order
2.1. Fundamental results
In this section of the study, we will present some fundamental concepts that will be useful for our computations in the remaining sections.
Definition 1.
The following expression (Butt et al., Citation2024) defines the fractional derivative of Riemann-Liouville with order and
as:
(3)
(3)
Definition 2.
The Caputo fractional derivative of order and
is stated in the following way (Butt et al., Citation2024):
(4)
(4)
Definition 3.
(Butt et al., Citation2022) A mapping with the constraint that
then the ABC derivative of order
is defined as following:
(5)
(5)
where
is a normalization constant with the property that
and
is a ML operator, and defined by
Definition 4.
(Din, Li, Khan, Khan, & Liu, Citation2022) The non-integer integration of a mapping taking
in the sense of ABC derivative is defined as:
(6)
(6)
Definition 5.
(Butt et al., Citation2022) The Laplace transform (LT) in the ABC sense for a mapping can be stated as:
(7)
(7)
Theorem 1.
(Din et al., Citation2022) The following fractional order problem(8)
(8) has a unique solution
(9)
(9)
Theorem 2.
(Zafar et al., Citation2022) Consider a convex subset of
and also assume that
with
is contraction,
is a continuous and compact set,
3. Qualitative analysis
In this part, we employed Banach fixed point theorems to establish the existence and uniqueness of the given system, in addition to the Ulam-Hyers stability of the analyzed model under the ABC derivative. Also, we compute the equilibrium states and fundamental reproduction number for the suggested system.
3.1. Existence and uniqueness of solutions for the measles model
In order to examine the existence of the solution of the non-integer model Equation(2)(2)
(2) , let us consider the model in the below form:
(10)
(10)
where
(11)
(11)
The following model will be used for the communication of Equation(2)(2)
(2) as:
(12)
(12)
Using EquationEquation (5)(5)
(5) , the above system becomes
(13)
(13)
where
(14)
(14)
and
(15)
(15)
Now, for the qualitative inspection, the following assumptions and
must be fulfilled:
(
):
is continuous mapping from
to
and there exists two constants
such that
(
): there exists a number
such that
(17)
(17)
Theorem 3.
(Din et al., Citation2022) Assuming that and
are true, then the proposed model Equation(2)
(2)
(2) has a solution, given that
(18)
(18)
Proof.
We transform Equation(12)(12)
(12) into a fixed point problem, that is
using the operator
expressed as:
(19)
(19)
Let
(20)
(20)
is a close, convex, bounded subset with
where
(21)
(21)
Define the operators
such that
(22)
(22)
(23)
(23)
We now divide the proof in the way described below:
Step Equation(1)
(1)
(1) :
for all
In fact, we have
(24)
(24) Therefore, we have
(25)
(25)
Step Equation(2)
(2)
(2) :
is contraction. Let
Then via (
), we get
(26)
(26)
Step Equation(3)
(3)
(3) :
is relatively compact.
Case 1:
is a continuous function. Since
is continuous, then
is also continuous.
Case 2:
is uniformly bounded on
Consider
then, we get
(27)
(27)
Therefore is uniformly bounded on
Case 3:
is equicontinuous. Suppose that
and
Then
From EquationEquation (28)(28)
(28) , it follows that
(29)
(29)
According to the theorem of Arzela-Ascoli, we reveal that is entirely continuous. Since, the system Equation(12)
(12)
(12) possesses at least one solution, then the suggested system has a unique solution. ▪
Theorem 4.
(Din et al., Citation2022) Given that assumption () is true, the system Equation(12)
(12)
(12) has a unique solution, implying that Equation(2)
(2)
(2) also has a unique solution if
Proof.
Suppose that be an operator defined
by
(30)
(30)
Let then
(31)
(31)
where
The operator is the contraction from EquationEquation (31)
(31)
(31) . As a result, EquationEquation (12)
(12)
(12) has a unique solution which inferred that the investigated model Equation(2)
(2)
(2) also has a unique solution. ▪
3.2. Ulam-Hyers stability
To discuss the stability of the suggested system by performing a slight variation and only satisfying,
thus
Lemma 1.
(Zafar et al., Citation2022) The following transformed problem’s
(32)
(32) solution satisfies
(33)
(33) where
(34)
(34)
Proof.
The proof of the Lemma 1 is straightforward, so we omit it. ▪
Theorem 5.
(Zafar et al., Citation2022) The analytical solution for the proposed system is UH stable if , and consequently the solution of the system Equation(12)
(12)
(12) is UH stable with the assumption (
) and EquationEquation (32)
(32)
(32) .
Proof.
Suppose that and
denote the unique solutions of Equation(12)
(12)
(12) , then
(35)
(35)
From EquationEquation (35)(35)
(35) , we get
(36)
(36)
Thus, we deduced that the solution of EquationEquation (12)(12)
(12) is UH stable and hence generalized UH stable utilizing
implying that the presented initial value problem solution is Ulam-Hyers stable and also generalized Ulam-Hyers stable. ▪
Assume that the following assumptions
Lemma 2.
(Zafar et al.,Citation2022) The following equation will satisfy EquationEquation (32)(32)
(32) as:
(37)
(37)
Proof.
The proof of the Lemma 2 is straightforward, so we omit it. ▪
Theorem 6.
(Zafar et al., Citation2022) According to Lemma 2, the solution for the suggested system is Ulam-Hyers-Rassias (UHR) stable, and as a result, generalized UHR stable.
Proof.
Suppose that and
denote the unique solutions of Equation(12)
(12)
(12) , then
(38)
(38)
From EquationEquation (38)(38)
(38) , we yield
(39)
(39)
Hence the solution of EquationEquation (12)(12)
(12) is UHR stable and consequently generalized UHR stable. ▪
3.3. Positivity of the solution
Theorem 7.
(Peter et al., Citation2022) The closed set(40)
(40) is positively invariant with respect to the model Equation(2)
(2)
(2) .
Proof.
Combining each of the governing equations of the system Equation(2)(2)
(2) yields
which can be written as:
(41)
(41)
Using the Laplace transform on Equation(41)(41)
(41) , we get
(42)
(42)
The nature of the Mittag-Leffler function is asymptotic (Jost et al., Citation2015). Therefore, we have
as
Consequently, the system Equation(2)
(2)
(2) has the solution in
Thus the system is positively invariant. ▪
3.4. Equilibrium points and reproduction number of the system
The suggested fractional model Equation(2)(2)
(2) admits two equilibrium states: measles-free and measles-endemic equilibrium states. The measles-free equilibrium is shown by
which exists only when there is no disease in the host population. For this point, every measles suffered class will be zero. Therefore, the measles-free equilibrium state
is given by:
(43)
(43)
Measles-endemic equilibrium state is represented by exists only when the disease is still present in the population. Using the following system of equations:
(44)
(44)
the measles-endemic equilibrium point
is obtained, where
For the basic reproduction number (), the concept of the next-generation matrix method is applied (Ahmad et al., Citation2024; Butt et al., Citation2023; Citation2024). Therefore, the computed value of the threshold parameter for the proposed fractional model is given by:
(45)
(45)
4. Sensitivity analysis of 

Making decisions about effectively managing a disease necessitates careful consideration of the sensitivity analysis concept. The sensitivity analysis enables us to examine how variables fluctuate when the parameters in are altered. It highlights the model’s most sensitive and impactful parameters and their effects on
Definition 6.
(Zafar et al., Citation2022) The normalized forward sensitivity index () of the basic reproduction number
that depends on a parameter
is provided below as:
(46)
(46)
In order to investigate the sensitivity of we compute its derivatives as follows:
The normalized sensitivity indices of the involved parameters are obtained as:
and illustrate the positive impact of
and
on the threshold parameter
presenting that an increase in the parameter values would lead to a rise in
It is clear from the calculated sensitivity indices that a 10
rise in the natality rate, effective contact rate, rate at which exposed humans become infected, and vaccine wane rate occurs to raise the value of
by 10
10
10
and 4.8
correspondingly, and can ultimately leading to a disease outbreak. Across the other perspective, natural recovery rate, natural death rate, death rate of infected humans due to measles, rate of vaccination of susceptible humans, and rate of exposed humans who have gone through screening and treatment suggests that raising their values by 10
will bring down the value of
by 4.3
10
5.6
4.8
and 9.9
respectively.
Figures (3–5) present the dynamics of reproduction numbers across various biological factors using 2D and 3D graphics. In , is calculated as
and
increase, while all other parameters remain constant. shows a 2D contour plot of
versus
indicating that an increase in
results in an increase in
In ,
is computed with
and
increasing. The 2D contour diagram of
versus
in illustrates that an increase in
leads to an increase in
calculates
assuming
and
are increasing, while the 2D contour graph of
versus
in shows that an increase in
results in an increase in
In the scenario where decreases or
increases, computes
In contour maps of
versus
confirm that a decrease in
leads to an increase in
calculates
assuming
is increasing and
is decreasing. The 2D contour figure of
versus
in shows that an increase in
causes a reduction in
computes
assuming
and
are increasing, while the 2D contour plot of
versus
in illustrates how an increase in
leads to an increase in
Finally, when decreases or
increases, determines
calculates
with
and
increasing, and displays similar performance trends.
5. Numerical analysis
This section introduces the Atangana-Toufik approach, a productive approximation method for the simulations of the considered system Equation(2)(2)
(2) . Remember that this strategy has previously been studied for fractional models (Butt et al., Citation2022; Toufik & Atangana, Citation2017). To apply this algorithm to system Equation(2)
(2)
(2) in the sense of ABC derivative, let us assume a uniform mesh on the interval [0, T] with the nodes marked 0, 1, 2, ., Nh, where Nh is a positive integer and
is the temporal step size. We now implement the Theorem 1 to each equation of the system Equation(2)
(2)
(2) to get the numerical solutions of the proposed system, we obtain the following results:
(47)
(47)
At a given point
then the EquationEquation (47)
(47)
(47) becomes
The above equation can written as:
(48)
(48)
Applying two-step Lagrange polynomial interpolation on the function in the interval
Therefore, we obtain
(49)
(49)
where
and
The above integrals substituted into the EquationEquation (49)(49)
(49) , then we have the solution
as follows:
(50)
(50)
Similarly, for the other state variables, we evaluate the following schemes:
(51)
(51)
(52)
(52)
(53)
(53)
(54)
(54)
We perform some numerical simulations for the system Equation(2)(2)
(2) employing the values of the parameters from as in a biologically feasible aspect to interpret our acquired results. The initial population of
and
are picked as 60309980, 0, 0, 76, and 0, respectively. The time varies between 0 and 25 months for the simulations. Population behaviour of the fractional measles model at the different fractional parameter values is depicted in . Effect of fractional order values
on the solution behaviour of
and
is described in . It is remarkable that when
is equal to 1, then the dynamics of the proposed model achieved its integer order case. To assess the impact of varying fractional parameters on reducing measles prevalence in the general population, we analyze the effects of these parameters on individual subpopulations, as depicted in . The results of the measles fractional model under various scenarios of fractional parameters are shown in . Over time, the fractional parameter values increase, leading to a decrease in the subpopulations of susceptible, vaccinated, exposed, and infected individuals. Conversely, an increase in the fractional parameter values over time results in an increase in the number of recovered individuals. These response curves illustrate the stability and asymptotic behaviour of the measles fractional system.
Figure 6. Population behaviour of the measles fractional model at the different fractional parameter values.
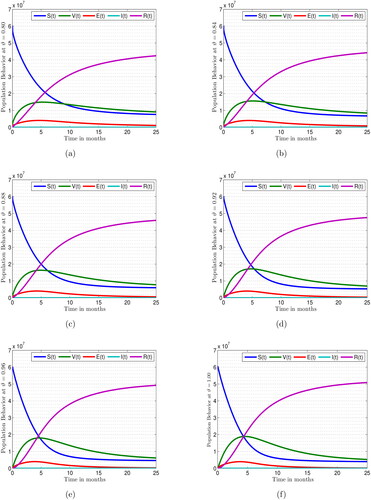
Figure 7. Simulations for the suggested model Equation(2)(2)
(2) using Atangan-Toufik scheme for the different values of fractional order
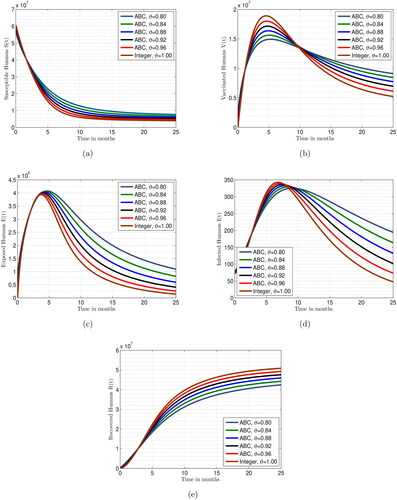
Table 2. Interpretation of involved symbols and their numeric values Equation(47)(47)
(47) .
Table 3. Sensitivity indices of against the parameters.
It seems that a larger fractional value leads to an improved outcome, as evidenced by the dynamics of the system approaching equilibrium in when the fractional order is reduced from 1 to 0.80 or even lower. Significant responses are observed in the compartments of the integrated model. Our numerical technique produces results as the time increment approaches a stable point, which represents the actual solution to the framework being studied. Each solution has a steady-state boundary. These graphs demonstrate how the model responds to decreasing fractional values, indicating that increasing the fractional value may enhance the solution’s accuracy.
This research illustrates that the long-lasting memory effect of the model can be effectively represented by fractional derivatives, which decrease as the fractional order approaches 1. The fractional differential operator captures an inherent effect, adding realism and accuracy to the proposed measles epidemic model. This framework considers long-term interdependencies, non-local consequences, durability, and recurrence, providing insights into measles transmission dynamics and guiding public health measures to prevent its spread. Decision-makers can utilize data relevant to the changing conditions of infected patients, as demonstrated by simulations. This analysis offers recommendations for practical measures to halt the spread of measles and anticipates advancements in this field.
6. Conclusion
The importance of epidemiological models in simulating disease transmission dynamics, fitting them to actual data, and recommending more effective control measures based on analysis cannot be overstated. To investigate the significance of memory in the system with the measles spread phenomenon, we have formulated an epidemiological framework for the spread of measles transmission with vaccination in a fractional context employing the ABC derivative. The primitive characteristics of the system, such as existence, uniqueness, boundedness, and positivity of the solution to the suggested fractional model, have been demonstrated using fundamental fractional calculus and fixed point theory. We have evaluated the system for equilibrium points and have used a next-generation matrix approach to obtain the threshold parameter for the considered model. To analyze the significance of various input components in we have conducted an analysis of the sensitivity of
using the partial rank correlation coefficient (PRCC) methodology. This analysis reveals that
and
are the most sensitive factors. To control the spread of measles, we recommend minimizing or controlling these rates. Furthermore, a numerical approach for the stated fractional operator has been provided to depict the solution behaviour of the model. We have found that the complicated behaviour of the measles model can be addressed more accurately and effectively by the fractional-order model. The numerical results indicate that increasing the fractional parameter from 0.80 to 1 leads to an increase in the susceptibility and recovery of the measles population, while the infection rate in the population decreases over time. With these findings, we intend to actively engage with public health officials and clinicians to contribute to more beneficial epidemiological studies aimed at combating the epidemic. Future research will explore the transmission behaviour of measles using a newly designed fractal-fractional operator.
Acknowledgement
This work was supported by the Ministry of Education, Youth and Sports of the Czech Republic through the e-INFRA CZ (ID:90254).
Disclosure statement
No potential conflict of interest was reported by the author(s).
References
- Abbasi, Z., Zamani, I., Mehra, A. H. A., Shafieirad, M., & Ibeas, A. (2020). Optimal control design of impulsive SQEIAR epidemic models with application to COVID-19. Chaos, Solitons, and Fractals, 139, 110054. doi:10.1016/j.chaos.2020.110054
- Abboubakar, H., Fandio, R., Sofack, B. S., & Ekobena Fouda, H. P. (2022). Fractional dynamics of a measles epidemic model. Axioms, 11(8), 363. doi:10.3390/axioms11080363
- Ahmad, W., Rafiq, M., Butt, A. I. K., Ahmad, N., Ismaeel, T., Malik, S., … Asif, Z. (2024). Analytical and numerical explorations of optimal control techniques for the bi-modal dynamics of Covid-19. Nonlinear Dynamics, 112(5), 3977–4006. doi:10.1007/s11071-023-09234-8
- Ain, Q. T., Anjum, N., Din, A., Zeb, A., Djilali, S., & Khan, Z. A. (2022). On the analysis of Caputo fractional order dynamics of Middle East Lungs Coronavirus (MERS-CoV) model. Alexandria Engineering Journal, 61(7), 5123–5131. doi:10.1016/j.aej.2021.10.016
- Ain, Q. T., & Chu, Y. M. (2004). On fractal fractional hepatitis B epidemic model with modified vacci. New England Journal of Medicine, 351(27), 2832–2838.
- Ain, Q. T., Khan, A., Abdeljawad, T., Gómez-Aguilar, J. F., & Riaz, S. (2024). Dynamical study of varicella-zoster virus model in sense of Mittag-Leffler kernel. International Journal of Biomathematics, 17(03), 2350027. doi:10.1142/S1793524523500274
- Ain, Q. T., & Wang, J. (2023). A stochastic analysis of co-infection model in a finite carrying capacity population. International Journal of Biomathematics, 2350083. doi:10.1142/S1793524523500833
- Alzahrani, E. O., & Khan, M. A. (2020). Comparison of numerical techniques for the solution of a fractional epidemic model. The European Physical Journal Plus, 135(1), 110. doi:10.1140/epjp/s13360-020-00183-4
- Angelo, K. M., Gastañaduy, P. A., Walker, A. T., Patel, M., Reef, S., Lee, C. V., & Nemhauser, J. (2019). Spread of measles in Europe and implications for US travelers. Pediatrics, 144(1) doi:10.1542/peds.2019-0414
- Anjum, N., Ain, Q. T., & Li, X. X. (2021). Two-scale mathematical model for tsunami wave. GEM-International Journal on Geomathematics, 12(1), 10.
- Anjum, N., He, C. H., & He, J. H. (2021). Two-scale fractal theory for the population dynamics. Fractals, 29(07), 2150182. doi:10.1142/S0218348X21501826
- Baishya, C., Achar, S. J., Veeresha, P., & Prakasha, D. G. (2021). Dynamics of a fractional epidemiological model with disease infection in both the populations. Chaos (Woodbury, N.Y.), 31(4), 043130. doi:10.1063/5.0028905
- Batool, A., Talib, I., Riaz, M. B., & Tunç, C. (2022). Extension of lower and upper solutions approach for generalized nonlinear fractional boundary value problems. Arab Journal of Basic and Applied Sciences, 29(1), 249–256. doi:10.1080/25765299.2022.2112646
- Battegay, R., Itin, C., & Itin, P. (2012). Dermatological signs and symptoms of measles: A prospective case series and comparison with the literature. Dermatology (Basel, Switzerland), 224(1), 1–4. doi:10.1159/000335091
- Bohner, M., Tunç, O., & Tunç, C. (2021). Qualitative analysis of caputo fractional ıntegro-differential equations with constant delays. Computational and Applied Mathematics, 40(6), 2021–2214. doi:10.1007/s40314-021-01595-3
- Budigan Ni, H., de Broucker, G., Patenaude, B. N., Dudley, M. Z., Hampton, L. M., & Salmon, D. A. (2023). Economic impact of vaccine safety incident in Ukraine: The economic case for safety system investment. Vaccine, 41(1), 219–225.
- Butt, A. R., Ahmad Saqib, A., Alshomrani, A. S., Bakar, A., & Inc, M. (2024). Dynamical analysis of a nonlinear fractional cervical cancer epidemic model with the nonstandard finite difference method. Ain Shams Engineering Journal, 15(3), 102479. doi:10.1016/j.asej.2023.102479
- Butt, A. I. K., Ahmad, W., Rafiq, M., & Baleanu, D. (2022). Numerical analysis of Atangana-Baleanu fractional model to understand the propagation of a novel corona virus pandemic. Alexandria Engineering Journal, 61(9), 7007–7027. doi:10.1016/j.aej.2021.12.042
- Butt, A. R., Saqib, A. A., Bakar, A., Ozsahin, D. U., Ahmad, H., & Almohsen, B. (2023). Investigating the fractional dynamics and sensitivity of an epidemic model with nonlinear convex rate. Results in Physics, 54, 107089. doi:10.1016/j.rinp.2023.107089
- Callister, L. C. (2019). Global Measles Outbreak. MCN. The American Journal of Maternal Child Nursing, 44(4), 237. doi:10.1097/NMC.0000000000000542
- Center of Disease Control and Prevention. (2023). Global Measles Outbreaks. Available online: https://www.cdc.gov/globalhealth/ measles/data/global-measles-outbreaks.html. (accessed on 19 January 2023).
- Center of Disease Control and Prevention. (n.d.). Global Measles Outbreaks. Available online: https://www.cdc.gov/measles/cases-outbreaks.
- Conlan, A. J. K., Rohani, P., Lloyd, A. L., Keeling, M., & Grenfell, B. T. (2010). Resolving the impact of waiting time distributions on the persistence of measles. Journal of the Royal Society, Interface, 7(45), 623–640. doi:10.1098/rsif.2009.0284
- Din, A., Li, Y., Khan, F. M., Khan, Z. U., & Liu, P. (2022). On Analysis of fractional order mathematical model of Hepatitis B using Atangana-Baleanu Caputo (ABC) derivative. Fractals, 30(01), 2240017. doi:10.1142/S0218348X22400175
- Farman, M., Saleem, M. U., Ahmad, A., & Ahmad, M. O. (2018). Analysis and numerical solution of SEIR epidemic model of measles with non-integer time fractional derivatives by using Laplace Adomian Decomposition Method. Ain Shams Engineering Journal, 9(4), 3391–3397. doi:10.1016/j.asej.2017.11.010
- Fisker, A. B., Martins, J. S. D., Jensen, A. M., Martins, C., Aaby, P., & Thysen, S. M. (2022). Health effects of utilising hospital contacts to provide measles vaccination to children 9-59 months-a randomised controlled trial in Guinea-Bissau. Trials, 23(1), 349. doi:10.1186/s13063-022-06291-z
- Gambrell, A., Sundaram, M., & Bednarczyk, R. A. (2022). Estimating the number of US children susceptible to measles resulting from COVID-19-related vaccination coverage declines. Vaccine, 40(32), 4574–4579. doi:10.1016/j.vaccine.2022.06.033
- Gould, D. (2015). Measles: Symptoms, diagnosis, management and prevention. Primary Health Care, 25(1), 34–40. doi:10.7748/phc.25.1.34.e908
- Graef, J. R., Tunç, C., & Şevli, H. (2021). Razumikhin qualitative analyses of Volterra integro-fractional delay differential equation with Caputo derivatives. Communications in Nonlinear Science and Numerical Simulation, 103(2021), 106037. doi:10.1016/j.cnsns.2021.106037
- Ilesanmi, M. M., Adeyinka, D. A., & Olakunde, B. O. (2022). Sustainable Development Goals and childhood measles vaccination in Ekiti State, Nigeria: Results from spatial and interrupted time series analyses. Vaccine, 40(28), 3861–3868. doi:10.1016/j.vaccine.2022.05.037
- James Peter, O., Ojo, M. M., Viriyapong, R., & Abiodun Oguntolu, F. (2022). Mathematical model of measles transmission dynamics using real data from Nigeria. Journal of Difference Equations and Applications, 28(6), 753–770. doi:10.1080/10236198.2022.2079411
- Jost, M., Luzi, D., Metzler, S., Miran, B., & Mutsch, M. (2015). Measles associated with international travel in the region of the Americas, Australia and Europe, 2001-2013: A systematic review. Travel Medicine and Infectious Disease, 13(1), 10–18. doi:10.1016/j.tmaid.2014.10.022
- Khan, M. A., & Atangana, A. (2022). Mathematical modeling and analysis of COVID-19: A study of new variant Omicron. Physica A, 599, 127452. doi:10.1016/j.physa.2022.127452
- Khan, T., Ullah, Z., Ali, N., & Zaman, G. (2019). Modeling and control of the hepatitis B virus spreading using an epidemic model. Chaos, Solitons, Fractals, 124, 1–9. doi:10.1016/j.chaos.2019.04.033
- Liu, P., Ikram, R., Khan, A., & Din, A. (2022). The measles epidemic model assessment under real statistics: An application of stochastic optimal control theory. Computer Methods in Biomechanics and Biomedical Engineering, 26(2), 138–159. doi:10.1080/10255842.2022.2050222
- Memon, Z., Qureshi, S., & Memon, B. R. (2020). Mathematical analysis for a new nonlinear measles epidemiological system using real incidence data from Pakistan. European Physical Journal plus, 135(4), 378. doi:10.1140/epjp/s13360-020-00392-x
- Nabti, A., & Ghanbari, B. (2021). Global stability analysis of a fractional SVEIR epidemic model. Mathematical Methods in the Applied Sciences, 44(11), 8577–8597. doi:10.1002/mma.7285
- Ochoche, J. M., & Gweryina, R. I. (2014). A mathematical model of measles with vaccination and two phases of infectiousness. IOSR Journal of Mathematics, 10(1), 95–105. doi:10.9790/5728-101495105
- Peter, O. J., Qureshi, S., Ojo, M. M., Viriyapong, R., & Soomro, A. (2022). Mathematical dynamics of measles transmission with real data from Pakistan. Modeling Earth Systems and Environment, 9(2), 1545–1558. doi:10.1007/s40808-022-01564-7
- Pokharel, A., Adhikari, K., Gautam, R., Uprety, K. N., & Vaidya, N. K. (2022). Modeling transmission dynamics of measles in Nepal and its control with monitored vaccination program. Mathematical Biosciences and Engineering: MBE, 19(8), 8554–8579. doi:10.3934/mbe.2022397
- Qureshi, S., & Jan, R. (2021). Modeling of measles epidemic with optimized fractional order under Caputo differential operator. Chaos, Solitons, Fractals, 145, 110766. doi:10.1016/j.chaos.2021.110766
- Raza, N., Arshed, S., Bakar, A., Shahzad, A., & Inc, M. (2023). A numerical efficient splitting method for the solution of HIV time periodic reaction-diffusion model having spatial heterogeneity. Physica A: Statistical Mechanics and Its Applications, 609, 128385. doi:10.1016/j.physa.2022.128385
- Raza, N., Bakar, A., Khan, A., & Tunç, C. (2022). Numerical simulations of the fractional-order SIQ mathematical model of Corona virus disease using the nonstandard finite difference scheme. Malaysian Journal of Mathematical Sciences, 16(3), 391–411. doi:10.47836/mjms.16.3.01
- Samko, S. G., Kilbas, A. A., & Marichev, O. I. (1993). Fractional integrals and derivatives (Vol. 1). Yverdon, Yverdon-les-Bains, Switzerland: Gordon and Breach Science Publishers.
- Singh, J., Kumar, D., Hammouch, Z., & Atangana, A. (2018). A fractional epidemiological model for computer viruses pertaining to a new fractional derivative. Applied Mathematics and Computation, 316, 504–515. doi:10.1016/j.amc.2017.08.048
- Sowole, S. O., Ibrahim, A., Sangare, D., & Lukman, A. O. (2020). Mathematical model for measles disease with control on the susceptible and exposed compartments. Open Journal of Mathematical Analysis, 4(1), 60–75. doi:10.30538/psrp-oma2020.0053
- Tao, H., Anjum, N., & Yang, Y. J. (2023). The Aboodh transformation-based homotopy perturbation method: New hope for fractional calculus. Frontiers in Physics, 11, 1168795. doi:10.3389/fphy.2023.1168795
- Toufik, M., & Atangana, A. (2017). New numerical approximation of fractional derivative with non-local and non-singular kernel: Application to chaotic models. The European Physical Journal Plus, 132(10), 1–16. doi:10.1140/epjp/i2017-11717-0
- Tunç, C., Tunç, O., & Yao, J.-C. (2022). On the new qualitative results in integro-differential equations with Caputo fractional derivative and multiple kernels and delays. Journal of Nonlinear Convex Analysis, 23(11), 2577–2591.
- Tunç, O., & Tunç, C. (2023). Solution estimates to Caputo proportional fractional derivative delay integro –differential equations. Revista de la Real Academia de Ciencias Exactas, Fisicas y Naturales – Serie A: Matematicas 117(1). doi:10.1007/s13398-022-01345-y
- Tyagi, S., Gupta, S., Abbas, S., Das, K. P., & Riadh, B. (2021). Analysis of infectious disease transmission and prediction through SEIQR epidemic model. Nonautonomous Dynamical Systems, 8(1), 75–86. doi:10.1515/msds-2020-0126
- Uchaikin, V. V. (2013). Fractional derivatives for physicists and engineers (Vol. 2). Berlin: Springer
- Vojtek, I., Larson, H., Plotkin, S., & Van Damme, P. (2022). Evolving measles status and immunization policy development in six European countries. Human Vaccines & Immunotherapeutics, 18(1), 2031776. doi:10.1080/21645515.2022.2031776
- Yang, X. J. (2019). General fractional derivatives: Theory, methods and applications. Chapman and Hall/CRC.
- Zafar, Z. U. A., Zaib, S., Hussain, M. T., Tunç, C., & Javeed, S. (2022). Analysis and numerical simulation of tuberculosis model using different fractional derivatives. Chaos, Solitons and Fractals, 160, 112202. doi:10.1016/j.chaos.2022.112202
- Zafar, Z. U. L. A., Yusuf, A., Musa, S. S., Qureshi, S., Alshomrani, A. S., & Baleanu, D. (2022). Impact of public health awareness programs on COVID-19 dynamics: A fractional modeling approach. Fractals, 31(10) doi:10.1142/S0218348X23400054