Abstract
Obesity is an alarming public health problem. Tailored nutritional therapy is advisable since emerging evidence on complex cross-talks among multifactorial agents. In this picture, the gut microbiota is highly individualized and intricately dependent on dietary patterns, with implications for obesity management. Most of the papers on the topic are observational and often conflicting. This review aimed to systematically organize the body of evidence on microbiota deriving from dietary trials in adult obesity giving the most certain phylogenetic, and metabolomic signatures in relation to both the host metabolism and phenotype changes published until now. We retrieved 18 randomized control trials on 1385 subjects with obesity who underwent several dietary interventions, including standard diet and healthy dietary regimens. Some phyla and species were more related to diets rich in fibers and others to healthy diets. Weight loss, metabolism improvements, inflammatory markers decrease were specifically related to different microorganisms or functions. The Prevotella/Bacteroides ratio was one of the most reported predictors. People with the burden of obesity comorbidities had the most significant taxonomic changes in parallel with a general improvement. These data emphasize the possibility of using symbiotic approaches involving tailored diets, microbiota characteristics, and maybe drugs to treat obesity and metabolic disorders. We encourage Authors to search for specific phylogenetic associations beyond a too generally reported Firmicutes/Bacteroides ratio.
Introduction
Obesity is an alarming public health problem, rapidly growing both in developed and developing countries, enough to be considered as an epidemic: in the US, more than two-thirds of the adults are classified as overweight, more than one-third are classified as obese, with a prevalence that is expected to increase further in the closer future (Ng et al. Citation2014). Personal, social, and economic costs of obesity comorbidities (i.e., cardiovascular diseases, cancer, diabetes, and other chronic conditions) represent a considerable issue (Tremmel et al. Citation2017); thus, the identification of consistently effective and, possibly, as more personalized as possible weight-reduction therapies are desirable.
The Mediterranean diet (MD) is recognized as one of the healthiest dietary regimens since it results in a reduced incidence of insulin resistance, hypertension, cardiovascular diseases (CVD), type 2 diabetes mellitus (T2DM), and metabolic syndrome (Estruch et al. Citation2018). The traditional MD is characterized by the consumption of a high intake of extra-virgin olive oil, fruits, cereals, nuts, legumes, and vegetables; moderate to low intake of fish and seafood, eggs, white meat, and dairy products; low intake of red and processed meats, and sweets. The MD exerts its beneficial effects through the synergistic mechanisms of several bioactive food components, which include unsaturated fatty acids, phytosterols, polyphenols, complex carbohydrates, fibers, vegetable protein, and non-sodium minerals. The PREDIMED multi-center randomized trial on 7,447 participants with high CVD risks receiving an energy-unrestricted MD supplemented with extra-virgin olive oil or nuts demonstrated decreased incidences of cardiovascular events compared to control diet (Salas-Salvadò et al., 2008; Babio et al. Citation2014; Estruch et al. Citation2018).
Other dietary patterns demonstrated to have a role in decreasing weight and cardiovascular risks are low-carbohydrate (low-carb) and low-fat diets. The basic principle of the low-carb diet is to restrict the total daily calories from carbohydrate intake to less than 45% of daily calories. Similarly, a low-fat diet is characterized by the reduction of total calories coming from fat to less than 20% of daily calories, with the acquisition of the remaining ones through the consumption of vegetables, fruits, and lean protein sources (Pace and Crowe Citation2016). According to a recent meta-analysis of randomized clinical trials (Mansoor et al. Citation2016), participants on low-carb diets experienced a greater reduction in body weight compared with participants on low-fat diets, but, at the same time, a greater increase in HDL-cholesterol and LDL-cholesterol, demonstrating an opposite change in two important cardiovascular risk factors. Moreover, the Primary care-led weight management for remission of T2DM (DiRECT) trial demonstrated that 825–853 kcal/day formula diet for 3–5 months (59% carbohydrate, 13% fat, 26% protein, 2% fiber) resulted in diabetes remission in almost half of participants (Lean et al. Citation2018).
Thus, the importance of tailored obesity nutritional therapy is clear. To date, different dietary interventions can lead to weight loss, although a substantial variability in diet success outcomes is visible (Johnston et al. Citation2014).
The emergence of the gut microbiota as a key regulator of health and disease has further complicated this issue. Since a mutualistic relation exists between diet and gut microbiota, dietary factors are among the most potent modulators of microbiota composition and function. Intestinal microbial communities in turn influence the absorption, metabolism, and storage of ingested nutrients, with potentially profound effects on the host physiology. Gut microbiota is highly individualized and intricately dependent on the quantity and quality of nutrients extracted from our diets, with direct implications for obesity (Ley et al. Citation2006; Turnbaugh et al. Citation2006; David et al. Citation2014). Microbial metabolites and proteins are known to communicate with the host influencing appetite control (Cani and Knauf Citation2016). Data collected over the past decade have identified the gut microbiota as an important factor defining interindividual variation in disease risk and dietary response. In spite of this, the current knowledge of the role of diet on microbiome-mediated health outcomes in humans mainly relies on observational studies in which confounding factors affect the conclusions (Grosso et al. Citation2017). Intervention studies to address the causal effects of diet on microbiome functions are still scarce or have been performed in animal models. Despite their cost and complexity, randomized controlled trials (RCTs) are the gold standard for evidence-based medicine and are an appropriate tool for identifying a causal relationship of a specific nutrient or diet on a health outcome in humans (Blumberg et al. Citation2010).
The aim of our systematic review (SR) is to explore the effects of MD diet, low-carb, and low-fat diet, and other standardized dietary habits on metabolic outcomes in subjects with overweight and obesity in relation to changes in the gut microbiota composition or function.
Methods
Literature search
We conducted a systematic review of randomized controlled trials (RCTs) on the effects of dietary habits in humans. To achieve this goal, the study protocol was organized according to PRISMA-P guidelines (Shamseer et al. Citation2015), and the resulting report was written according to the Preferred Reporting Items for Systematic Reviews and Meta-Analyses (PRISMA) (Moher et al. Citation2015).
PICO methodology (Population: obese and overweight adult subjects; Intervention: MD diets or low-carb diet or low-fat diet, or other standardized healthy dietary habits; Comparison: other dietary patterns; Outcomes: metabolic parameters and gut microbiota composition or function) to develop a search strategy based on medical subject headings (MeSH) and keywords were used.
The Cochrane Central Register of Controlled Trials, PubMed, and EMBASE databases (until December 2020) were systematically used. The reference lists of identified studies and key review articles, including previously published reviews, were also searched for all randomized clinical trials assessing the effects of MD, low-carb diet, or low-fat diet on metabolic outcomes together with gut microbiota composition. No country restrictions were imposed.
The search terms used included “diet” and “microbiota” and “metabolic” and “human” and “adult”. The search strategy used both keywords and MeSH terms. No further limitations were made so the search terms would be as sensitive as possible. The research was restricted to RTCs only. In addition, we checked the references of eligible articles for further papers that were not captured by our search strategy, and we corresponded with authors when the relevant information was missing in the paper. Only articles in English were considered. The review has been registered on PROSPERO (CRD42022301419).
Outcome measures
The primary outcome measures were as follows:
body weight, body weight excess (e.g., overweight, obesity), body mass index (BMI), metabolic syndrome (MetS), arterial hypertension, lipid profile, glucose levels, insulin resistance
gut microbiota composition (16S rDNA, metagenomics, metaproteomics, shotgun metagenomics), gut microbiota function (short-chain fatty acids, lactic acid, end-metabolic products, metabolomics).
Included studies had to report at least results on gut microbiota composition.
Inclusion and exclusion criteria
For inclusion, studies were required to (i) include overweight or obese adult subjects; (ii) be published in peer-reviewed journals in the English language; (iii) be a randomized interventional study.
We excluded studies on subjects affected by diabetes mellitus.
Identification of relevant studies
Potentially relevant papers were selected by reading the titles and abstracts. If abstracts were not available or did not provide enough results the entire article was retrieved and screened to determine whether it met the inclusion criteria.
Data extraction and synthesis
A form was generated to register whether individual studies met eligibility criteria and to collect data regarding the study design and methodological quality. Four authors (MC, SP, AC, and EA) independently reviewed the titles and the abstracts to identify studies to be included in the review. Any disagreement was discussed with the other three reviewers (FP, MM, EB). Finally, the full texts of articles that passed the screening and eligibility steps were retrieved and read by the same reviewers and all the other authors.
Any difference in opinion about the studies was resolved by discussion between all the investigators. The following data were extracted: author, date of publication, study design, characteristics of the participants, type of diet intervention, assessment methodology and reliability and validity of dietary measures, length of follow-up, methods of evaluation of gut microbiota, clinical outcomes, and changes in gut microbiota composition or function. This information is summarized in .
Table 1. Characteristics of included studies.
Quality assessment
The methodological quality of the included studies was assessed according to the Cochrane’s revised risk of bias tool RoB 2 (Sterne et al. Citation2019). Two authors independently evaluated the following domains for risk of bias: (1) randomization process; (2) deviations from intended interventions; (3) missing outcome data; (4) measurement of the outcome; (5) selection of the reported result. For each domain, there are three categories for risk of bias judgements: low risk, high risk, and some concerns (Sterne et al. Citation2019). The study was classified as having a low risk of bias if all domains were judged as at low risk of bias and as having a high risk of bias if it was at high risk of bias in any one domain or if it had some concerns in multiple domains. Otherwise, the study was considered as having some concerns (Sterne et al. Citation2019). Consequently, for visualizing risk-of-bias assessments for the selected studies, two figures were generated using the web app robvis (McGuinness and Higgins Citation2021). Disagreements were resolved by discussion with a third reviewer.
Results
Cumulatively, our search identified a total of 727 potentially eligible studies. After duplicates removal, there were 355 residual records. Of these, 319 were excluded as judged irrelevant according to titles. The remaining 36 records were screened by reading abstracts and 7 further were excluded because including diabetic patients (n = 3) or normal-weight subjects (n = 4) in the study population. Thus, 29 articles were retrieved and underwent full-text assessment. Among these, 7 were excluded for reasons related to the randomization process (n = 2) or to the intervention dietary pattern (n = 2), or because of being post-hoc analysis of RCTs conducted irrespective of the randomized dietary intervention (n = 3). The selection process is summarized in .
Study characteristics
The 22 articles included in SR were publications of results from 18 RCTs. All RCTs had been deposited in the ClinicalTrial.gov registry except 2 which had been set up before clinical trial registration was required by law regardless of intervention type (Russell et al. Citation2011; Walker et al. Citation2011; Salonen et al. Citation2014).
Articles included collectively encompassed 1385 subjects who completed the studies, 48.7% of whom were women (N = 675). These numbers may be overestimated as some studies were conducted in a subset of subjects recruited in a larger parental trial and may have included the same participants while 2 studies certainly involved the same population (Salonen et al. Citation2014; Walker et al. Citation2011). However, since the outcome differs in different papers, we decided to consider data from all reports.
At baseline, subjects’ age ranged from a mean of 27.6 ± 5.9 years to a mean of 63.72 ± 1.70 years. The maximum BMI was 39.37 ± 1. Kg/m2 and 338 patients (24.3%) were affected by metabolic syndrome. Subjects came from 9 countries (Spain, Denmark, USA, Italy, United Kingdom, Finland, France, Netherlands, Canada). Characteristics of included studies are detailed in .
Risk of bias within studies
The risk of bias assessment for the selected studies is presented in and . Most studies (68.2%) (Russell et al. Citation2011; Walker et al. Citation2011; Christensen et al. Citation2013; Fava et al. Citation2013; Lappi et al. Citation2013; Salonen et al. Citation2014; Haro et al. Citation2016a, Citation2016b, Citation2017; Vuholm et al. Citation2017; Bendtsen et al., 2018; Kopf et al. Citation2018; Fragiadakis et al. Citation2020; Grembi et al. Citation2020; Johnstone et al. Citation2020) were judged to have some concerns in at least one domain, especially in bias arising from the randomization process (45.5%) (Russell et al. Citation2011; Walker et al. Citation2011; Christensen et al. Citation2013; Fava et al. Citation2013; Salonen et al. Citation2014; Haro et al. Citation2016b, Citation2017; Vuholm et al. Citation2017; Kopf et al. Citation2018; Johnstone et al. Citation2020) and in the selection of the reported result (54.5%) (Russell et al. Citation2011; Walker et al. Citation2011; Christensen et al. Citation2013; Fava et al. Citation2013; Lappi et al. Citation2013; Salonen et al. Citation2014; Haro et al. Citation2016a, Citation2016b, Citation2017; Bendtsen et al. 2018; Fragiadakis et al. Citation2020; Grembi et al. Citation2020), mainly due to lack of information regarding allocation concealment or unavailable protocol study, respectively. Only 2 RCTs (9%) (Haro et al. Citation2016b; Lappi et al. Citation2013) were classified as having a high risk of bias: one probably didn’t conceal allocation adequately (Lappi et al. Citation2013), while the other provided no information about the extent of missing outcome data (Haro et al. Citation2016b). All trials (Russell et al. Citation2011; Walker et al. Citation2011; Christensen et al. Citation2013; Fava et al. Citation2013; Lappi et al. Citation2013; Salonen et al. Citation2014; Vitaglione et al. 2015; Haro et al. Citation2016a, Citation2016b, Citation2017; Beaumont et al. Citation2017; Vuholm et al. Citation2017; Bendtsen et al. 2018; Hjorth et al. Citation2018; Kopf et al. Citation2018; Schutte et al. Citation2018; Mayengbam et al. Citation2019; Roager et al. Citation2019; Fragiadakis et al. Citation2020; Grembi et al. Citation2020; Johnstone et al. Citation2020; Meslier et al. Citation2020) were considered at low risk both for bias due to deviations from intended interventions and bias in the measurement of the outcome.
Clinical results
Intervention diets are detailed in . In particular, 3 studies evaluated the effects of the MD and low-fat diet (N = 365) (Haro et al. Citation2016a, Citation2016b, Citation2017), 1 of MD only (N = 82) (Meslier et al. Citation2020), 1 of low dairy versus high dairy diet (N = 52) (Bendtsen et al., 2018), 1 of New Nordic Diet (N = 62) (Hjorth et al. Citation2018), 2 of low-carb or low-fat diet (N = 171) (Fragiadakis et al. Citation2020; Grembi et al. Citation2020); the other studies considered different standardized healthy dietary patterns (N = 653). The control diets were specified in 8 studies only (average Danish diet, western diet, limited fruit and vegetable intake, Finnic diet, Netherland diet, UK diet) (N = 451) (Lappi et al. Citation2013; Vitaglione et al., Citation2015; Vuholm et al., Citation2017; Hjorth et al. Citation2018; Kopf et al. Citation2018; Schutte et al. Citation2018; Johnstone et al. Citation2020; Meslier et al. Citation2020).
Table 2. Diets compositions of the included studies.
No intervention on physical activity was considered. One study estimated physical activity by International Physical Activity Questionnaire (Vitaglione et al., Citation2015).
Most studies recorded data about adherence to diet by food frequency questionnaires (Lappi et al. Citation2013; Haro et al. Citation2016a, Citation2016b, Citation2017; Kopf et al. Citation2018), some on a recall-based methodology (Vitaglione et al., 2015; Grembi et al. Citation2020) or by food diaries (Fava et al. Citation2013; Vitaglione et al., 2015; Beaumont et al. Citation2017; Vuholm et al. Citation2017; Bendtsen et al., 2018; Kopf et al. Citation2018; Schutte et al. Citation2018; Meslier et al. Citation2020). Three studies evaluated the fasting concentrations of plasma alkylresorcinols, phenolic lipids, used as biomarkers for people who eat wholegrains wheat and rye rather than refined cereals (Vuholm et al. Citation2017; Schutte et al. Citation2018; Roager et al. Citation2019).
Most, but not all studies, evaluated clinical changes related to anthropometric or metabolic parameters during the intervention diet (Christensen et al. Citation2013 Fava et al. Citation2013; Lappi et al. Citation2013; Salonen et al. Citation2014; Vitaglione et al., 2015; Haro et al. Citation2016a, Citation2017; Beaumont et al. Citation2017; Bendtsen et al., 2018; Vuholm et al. Citation2017; Hjorth et al. Citation2018; Kopf et al. Citation2018; Schutte et al. Citation2018; Fragiadakis et al. Citation2020; Grembi et al. Citation2020; Johnstone et al. Citation2020; Meslier et al. Citation2020). Two studies considered the results according to the Prevotella/Bacteroides (P/B) ratio (Hjorth et al. Citation2018; Grembi et al. Citation2020). One study evaluated the P/B ratio as a prognostic marker for successful body fat loss on two diets differing greatly in dietary fiber and whole-grain content (New Nordic Diet and Average Danish Diet). For this purpose, fecal samples were collected at baseline and the relative abundance of Prevotella spp. and Bacteroides spp. was determined. This resulted in a clear bimodal separation of subjects based on the log Prevotella spp. to Bacteroides spp. ratio, allowing designation of low P/B (<0.01) or high P/B (>0.01) ratio subjects (Hjorth et al. Citation2018). Similarly, another study investigating the correlation between pre-diet P/B ratio and weight loss success on low-carb or low-fat diet classified subjects as having high or low P/B ratio, although using a lower P/B ratio cutoff value (0.003) due to the lower prevalence and quantity of Prevotella spp. in fecal samples (Grembi et al. Citation2020).
Standard diet regimens
MD and low-fat diet reduced triglycerides levels in male obese subjects affected by metabolic syndrome (Haro et al. Citation2017). Moreover, the two dietary patterns were associated with an improvement in insulin sensitivity index measured from OGTT (Haro et al. Citation2016a).
An isocaloric MD (Meslier et al. Citation2020) reduced total plasma cholesterol and HDL-cholesterol if compared to the Western diet. A low-carb diet and low-fat diet showed similar effects on reduction in BMI, body fat, waist circumference, blood pressure, insulin, glucose levels, and resting energy expenditure. A low-carb diet compared to a low-fat diet caused an increase in LDL and HDL levels and a reduction in triglycerides and in respiratory exchange ratio (Fragiadakis et al. Citation2020).
Hypocaloric (daily 500-kcal of energy deficit compared with the estimated energy requirement of each subject) high-dairy and low-dairy diets were associated with a reduction in body weight, fat mass, hip, and waist circumference but a low-dairy diet resulted in greater loss of hip circumference (Bendtsen et al., 2018).
Other or healthy dietary regimens
Dietary interventions promoting a high intake of whole grains, fruits, and vegetables showed no difference in BMI, while whole grains diet reduced TNFα, or IL6 and C-reactive protein (Vitaglione et al., 2015; Kopf et al. Citation2018; Roager et al. Citation2019) compared to western diet (Kopf et al. Citation2018) or selected refined wheat products (Vitaglione et al., 2015), and fruits and vegetable diet reduced IL-6 compared to western diet (Kopf et al. Citation2018).
A whole-grain diet, compared to a refined grain diet, reduced body weight, fat-free mass and showed a tendency in reduction of fat mass (p = 0.057), reduced sagittal abdomen diameter, and a similar tendency was observed for waist circumference (Roager et al. Citation2019). Moreover, whole-grain wheat diet compared to refined wheat diet was associated with a reduction in liver steatosis measured with proton magnetic resonance spectroscopy (1H-MRS) was used to quantify lipid content in the liver (3-Tesla whole-body MRI scanner) (Schutte et al. Citation2018).
Rye bread diet, compared to refined white wheat bread, in subjects with metabolic syndrome had similar effects on body weight, markers of glucose metabolism, and inflammation but a decrease in high sensitivity C-reactive protein concentration was recorded (Lappi et al. Citation2013).
High MUFA/low glycemic index (GI) diet (HM/HG: total fat 38%, saturated fat 10%, MUFA 20%, PUFA 6%, CHO 45%, GI 53%), high carbohydrate/high GI diet (HC/HG: total fat 28%, SFA 10%, MUFA 11%, PUFA 6%, CHO 55%, GI 51%) and high carbohydrate/low GI diet (total fat 28%, saturated fat 10%, MUFA 11%, PUFA 6%, CHO 55%, GI 51%) reduced plasma total and LDL cholesterol compared to the starting high saturated fat diet- high GI diet (HS/HG: total fat 38%, high saturated fat 18%, MUFA 12%, PUFA 6%, CHO 45%, GI 64%). HDL cholesterol was slightly decreased after a high carbohydrate/high GI diet compared to a high saturated fat/HG diet. The concentration of NEFA increased after intervention with high carbohydrate/high GI compared to high saturated fat/high GI diet, while it decreased after intervention with high carbohydrate/low GI diet. Fasting plasma glucose concentrations were significantly lower after intervention with both high carbohydrate diets and high carbohydrate/high GI also decreased plasma insulin concentrations compared to baseline with high saturated fat diet- high GI diet (Fava et al. Citation2013).
Microbiome results
Microbiota changes in ecology and function are detailed in and in . Twenty studies sequenced 16S rDNA and the last two studies used the FISH technique (Russell et al. Citation2011; Fava et al. Citation2013). Among studies using 16S rDNA, two of them also performed a shotgun metagenomic sequencing analysis (Roager et al. Citation2019; Meslier et al. Citation2020), and the other two a quantitative real-time PCR (Walker et al. Citation2011; Christensen et al. Citation2013). Thirteen studies evaluated fecal and/or blood metabolic end-products of microbiota (Russell et al. Citation2011; Haro et al. Citation2016a; Fava et al. Citation2013; Salonen et al. Citation2014; Vitaglione et al., 2015; Beaumont et al. Citation2017; Vuholm et al. Citation2017; Bendtsen et al. 2018; Kopf et al. Citation2018; Mayengbam et al. Citation2019; Roager et al. Citation2019; Meslier et al. Citation2020; Johnstone et al. Citation2020).
Figure 4. Microbiota main changes in individuals with obesity following classical or healthy dietary regimens in comparison to several control diets. MD: Mediterranean Diet, compared to low-fat high carbohydrate diet in 1Haro et al. (Citation2016a, Citation2016b, Citation2017) and to a habitual western diet in 2Meslier et al. (Citation2020). LFHC: Low-fat high-carbohydrate diet shows changes in comparison to MD (1Haro et al. Citation2016a, Citation2016b, Citation2017; P. diastonis only in the non-MetS group; Streptococcus and Clostridium in MetS-OB) and in microbiome plasticity (3Fragiadakis et al. Citation2020; 4Grembi et al. Citation2020). HFD: High fat diet; F. prausnitzii increased in high saturated fat diet with a high glycemic index as opposed to baseline (5Fava et al. Citation2013). HPD: high protein diet presents variations in Roseburia/Eubacterium rectale as proportion of total bacteria and decreased of Bacteroidetes versus MD (6Russell et al. Citation2011; 7Beaumont et al. Citation2017). *Others: dietary interventions with resistant-starch weight maintenance diet (RS-WM) versus control weight maintenance diet (C-WM diet) (8Johnstone et al. Citation2020); whole grain (WG) and fruits and vegetables (FV) interventions versus baseline (9Kopf et al. Citation2018). WG diet was also studied against refined grain (RG) consumption (10Roager et al. Citation2019; 12Vitaglione et al. Citation2015), with correlation in women (13Vuholm et al. Citation2017); resistant starch (RS) diet, non-starch polysaccharides (NSPs) diet and weight loss (WL) diet versus Run-in maintenance diet (protein/carbohydrate/fat % as 13:52:35 of metabolizable energy) (11Salonen et al. Citation2014, 14 Walker et al. Citation2011). Created with BioRender.com.
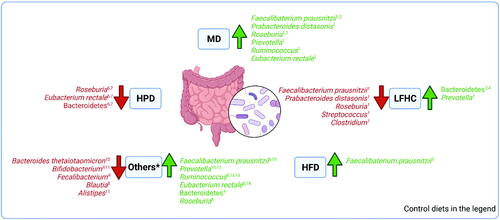
Table 3. Results of the included studies.
Microbiota composition
Standard diet regimens
The four studies which analyzed MD reported several species or genera that increased or decreased after the dietary intervention (Haro et al. Citation2016a, Citation2016b, Citation2017; Meslier et al. Citation2020). Increases in Faecalibacterium prausnitzii, Parabacteroides distasonis, and Roseburia, Prevotella, Rumninococcus, and Eubacterium rectale were the most frequently reported changes in comparison to a low-fat high-carbohydrate diet. Changes after the MD were influenced by the metabolic phenotype being more pronounced in subjects more compromised at baseline or showing a greater improvement in insulin resistance during the intervention.
Furthermore, studies with low-fat high-carbohydrate diets or low dairy products generally observed decreases in some species or genera, including some reported above as Faecalibacterium prausnitzii, Parabacteroides distasonis, and Roseburia, (Haro et al. Citation2016a, Citation2016b, Citation2017; Bendtsen et al., 2018; Fragiadakis et al. Citation2020; Grembi et al. Citation2020). Firmicutes/Bacteroidetes ratio, Streptococcus and Clostridium genera decreased, and Prevotella and generally Bacteroidetes increased, as microbiome plasticity. Changes were again more prevalent in subjects with a worse metabolic phenotype at baseline. A high saturated fat diet with a high glycemic index reported an increase in F. prausnitzii, meanwhile, high MUFA diets (with low or high glycemic index) reported a decrease in total bacteria with respect to diets with high carbohydrate with a high glycemic index or high saturated fat diet- high glycemic index (Fava et al. Citation2013). Some changes were indistinguishable after one year, suggesting resilience and an “obesity memory” (Fragiadakis et al. Citation2020).
Not standard or healthy dietary regimens
Most of the other dietary regimens described interventions with fibers, resistant starch, whole wheat, vegetables, and fruits with respect to conventional weight-loss diets or diets rich in foods with refined carbohydrates. The diet compositions, mainly regarding the glycemic index, fats, and type of fibers influenced the microbiome composition with more numerous genera and species described. Increases in Bacteroidetes, Ruminococcus bromii, F. prausnitzii, Roseburia, Prevotella, Eubacterium rectale, and Oscillobacter were the most frequently reported changes in comparison to control diets. Furthermore, decreases in Bacteroides, in particular thetaiotaomicron, Fecalibacterium, Blautia, Alistipes, and Bifidobacterium were also reported (Walker et al. Citation2011; Christensen et al. Citation2013; Lappi et al. Citation2013; Salonen et al. Citation2014; Vitaglione et al., 2015; Vuholm et al. Citation2017; Kopf et al. Citation2018; Schutte et al. Citation2018; Mayengbam et al. Citation2019; Roager et al. Citation2019; Johnstone et al. Citation2020). Fewer modifications than dietary models described above were described with high-protein diets that reported decreases in Roseburia, Eubacterium rectale, and Bacteroidetes (Russell et al. Citation2011; Beaumont et al. Citation2017).
Microbiota metabolic end-products
Standard diet regimens
The MD did not modify stool SCFAs in any study (Fava et al. Citation2013; Haro et al. Citation2016a, Citation2016b, Citation2017; Meslier et al. Citation2020), except for a control diet rich in high-saturated fats in which SCFAs increased (Fava et al. Citation2013). Diversely, a reduction in stool content of BCFAs (valerate, isovalerate, isobutyrate, and 2- methyl butyrate) and biliary acids (deoxycholic and lithocholic acid) and an increase in urinary urolithin glucuronides were reported, in relation with changes in the intake of some foods (decrease in meat and increase in nuts consumption) (Meslier et al. Citation2020). Serum metabolites expressed a shift of energy production from beta-oxidation to glycolysis in the previous models (Meslier et al. Citation2020) or also amino acid, and sphingolipid metabolism if a low-fat high carbohydrate diet is included (Haro et al. Citation2016a). The latter metabolites were mainly related with 3 bacterial genera and 2 bacterial species (Roseburia; Prevotella; Oscillospira; Faecalibacterium prausnitzii; Bacteroides uniformis) (Haro et al. Citation2016a).
Not standard or healthy dietary regimens
Fecal SCFA decreased in some (Salonen et al. Citation2014; Vuholm et al. Citation2017; Mayengbam et al. Citation2019) but not all the studies (Kopf et al. Citation2018; Roager et al. Citation2019; Johnstone et al. Citation2020) on the role of whole grain/resistant starch/fibers in comparison to other dietary models. Pea-derived fibers decreased fecal primary, secondary, and total bile acids (Mayengbam et al. Citation2019). Consumption of whole-grain was associated with high levels of fecal ferulic acid and serum dihydro-ferulic acid (DHFA) that were mostly related to an increase in Bacteroidetes abundance in one study (Vitaglione et al., 2015), and several urinary biomarkers of whole-grain intake, including phenolic metabolites (Roager et al. Citation2019). Although the microbial variations were negligible, diets rich in protein demonstrated a shift in bacterial metabolism toward amino acid degradation with different metabolite profiles according to the protein source (Russell et al. Citation2011; Beaumont et al. Citation2017). The butyrate concentration in stools was directly related to the content of carbohydrates (Russell et al. Citation2011).
Microbiota relation with host metabolism
A decrease in Prevotella copri was associated with an improvement in insulin resistance in subjects on the MD (Meslier et al. Citation2020) and a decrease in Holdemania with amelioration in glucose levels during an oral glucose tolerance test on a pea fiber diet (Mayengbam et al. Citation2019). Furthermore, the highest reduction in fecal biliary acids was related to a reduced relative abundance of Bilophila wadsworthia (Meslier et al. Citation2020). Taxonomic changes after MD reported in the previous paragraphs were more pronounced in subjects more metabolically compromised at baseline (Haro et al. Citation2016b, Citation2017). Weight was negatively associated with genera Lachnospira and Oscillospira, whereas Faecalibacterium, Clostridiales, and Clostridium were positively associated with it (Fava et al. Citation2013; Mayengbam et al. Citation2019). Furthermore, Bacteroides spp., and mainly Prevotella abundance, were associated with a decrease in body weight, BMI, waist circumference, fat mass percentage, and trunk fat percentage in several dietary models including a diet rich in fiber and whole grain or with high carbohydrate/high glycemic index (Christensen et al. Citation2013; Fava et al. Citation2013).
Healthy diet regimens resulted in improvements in inflammatory biomarkers related to some taxa. Individuals with higher Firmicutes and lower Bacteroidetes showed a greater decrease in lipopolysaccharides binding protein during the study (Kopf et al. Citation2018). Reduced TNF-α was associated with an increased abundance of Bacteroides and Lactobacillus (Vitaglione et al. Citation2015), meanwhile Erysipelatoclostridium ramosum positively with IL-6 (Roager et al. Citation2019).
Studies stratifying analysis according to prevotella/bacteroides ratio
High P/B ratio (> 0.01) subjects after 26-week of New Nordic Diet (very high in dietary fiber, whole grain, fruit and vegetables; dietary fiber 43.3 g/MJ, protein 18.1%, fat 30.4%, carbohydrates 46.6%) compared to Average Danish Diet (dietary fiber 28.6 g/MJ, protein 16.4%, fat 33.8%, carbohydrates 45.3%), showed a 3.15 kg and a 3.49 kg larger body fat and body weight loss respectively, and a 4.75 cm reduction in waist circumference, whereas no difference in such parameters was observed between NND and ADD among individuals with low P/B ratio (<0.01). A 2.27 kg body fat difference in responsiveness to the diets was found between the P/B groups, which came from a difference in response to NND. Similar differences in responsiveness to the diets were found for waist circumference (3.95 cm) and were borderline significant for body weight (2.33 kg) (Hjorth et al. Citation2018). During the 1-year follow-up period, subjects with the high P/B ratio changing from ADD to being recommended NND maintained their weight (−1.23 kg; NS), whereas subjects with the low P/B ratio changing from ADD to being recommended NND regained 2.76 kg (Hjorth et al. Citation2018).
Considering high P/B (>0.003) and low P/B (<0.003) ratio subjects on a low carb-diet compared to a low-fat diet (reduction of daily intake of carbohydrate or fat to 20 g/day, followed by slow increments of 5-15 g/day, according to individuals’ satisfaction with their daily intake and weight loss progress, with the ultimate objective of finding the lowest level of carbohydrate or fat they could achieve and conceivably maintain), high P/B ratio subjects on the low-carb diet had more weight loss than those with low P/B ratio, although this finding was not confirmed in a validation cohort. No association was found between weight loss and P/B ratio for subjects on the low-fat diet (Grembi et al. Citation2020).
Discussion
Recent literature strongly suggests that dietary approaches in obesity should be tailored to patients, and microbiota composition and function could be markers to select the best regimen. However, microbiota findings are still quite confusing. For the first time, we have tried to systematically review the dietary interventions with standard or healthy dietary patterns in controlled trials in adult obesity with a focus also on the microbiota.
First, standard diet regimens, including low-carbohydrate, low-fat, or MD resulted in decreasing weight and BMI, whereas other healthy regimens related to the promotion of whole grain, fruits, and vegetables could be related or not to a weight decrease (Johnstone et al. Citation2020; Fava et al. Citation2013; Lappi et al. Citation2013; Bendtsen et al. 2018; Hjorth et al. Citation2018; Kopf et al. Citation2018, Roager et al. Citation2019; Fragiadakis et al. Citation2020; Grembi et al. Citation2020). However, the latter interventions were clearly followed by a better phenotype, by decreasing inflammation, and improving glucose metabolism, lipid profile, and metabolic syndrome (Fava et al. Citation2013; Lappi et al. Citation2013; Vitaglione et al., Citation2015; Kopf et al. Citation2018; Schutte et al. Citation2018; Roager et al. Citation2019) similarly to the standard regimens (Haro et al. Citation2016a, Citation2017; Bendtsen et al. 2018; Meslier et al. Citation2020; Fragiadakis et al. Citation2020).
Second, the diet regimens clearly modified the microbiota signature in a dependent fashion mainly related to the macronutrient composition. Percentage in carbohydrates, fats, and fibers seem to be the master regulators of the microbiome composition, richness, and function, at least in a relatively short-term period, whereas increasing protein influenced relatively few species and genera. Bacteroides, and Prevotella sp, belonging from Bacteroidetes phylum, were among the species most frequently changed in their relative abundance, mainly when the dietary regimens were rich in fibers, as in the Mediterranean and Nordic diet, or because fruits, vegetables, and whole cereals were promoted (Russell et al. Citation2011; Christensen et al. Citation2013; Fava et al. Citation2013; Lappi et al. Citation2013; Vitaglione et al. Citation2015; Haro et al. Citation2016a, Citation2016b, Citation2017; Kopf et al. Citation2018; Mayengbam et al. Citation2019; Roager et al. Citation2019; Fragiadakis et al. Citation2020; Meslier et al. Citation2020). These results indicated a metabolic shift that included important changes in carbohydrate metabolism. Subjects with a higher abundance of Bacteroides appeared to have lower fiber- degrading potential than the Prevotella abundant subjects (Christensen et al. Citation2013; Vuholm et al. Citation2017; Hjorth et al. Citation2018). In line with this, in cross-sectional studies vegans and vegetarians had an abundance of Prevotellaceae, Ovo-Lacto vegetarians were characterized by the presence of Prevotella micans, Bacteroides vulgatus, and Faecalibacterium prausnitzii, and they presented the highest abundance of microbial carbohydrate- and protein-hydrolyzing enzymes (Ferrocino et al. Citation2015; De Angelis et al. Citation2020). The distinction in Prevotella abundance might help explain metabolic differences in response to a fiber-rich, whole-grain diet among people with metabolic diseases, and should be one of the first microbiota signatures to exploit large clinical trials on tailored nutrition. Furthermore, they are in line with the involvement of the Firmicutes/Bacteroidetes ratio in the obesity phenotype, likely related to usual diet composition, among the pathophysiological mechanisms (Ley et al. Citation2006; Cani Citation2019). However, by the analyzing data deriving from of all the papers we summarized, it appears clear that this ratio is too general to be considered a hallmark of obesity. Host’s characteristics and dietary interventions have a strong influence, frequently ignored in observational studies on obesity, and signatures of obesity are not consistent when the data are analyzed with consistent methods (Walters, Xu, and Knight Citation2014; Sze and Schloss Citation2016; Magne et al. Citation2020; Manor et al. Citation2020). Herein, most of the studies are burdened by confounding factors masked by the genetic traits for niche competitiveness between Firmicutes and Bacteroidetes sp. as determined by advanced phylogenetic assessments though shotgun sequencing (Bradley, Nayfach, and Pollard Citation2018).
In agreement with these considerations, moving from generalized categories, to species and genus levels, Parabacteroides distasonis, Roseburia sp., Rumninococcus sp., and Eubacterium rectale showed also a relative increased abundance after the MD or diets rich in fibers (Walker et al. Citation2011; Russell et al. Citation2011; Salonen et al. Citation2014; Haro et al. Citation2016a, Citation2016b, Citation2017; Vuholm et al. Citation2017; Kopf et al. Citation2018; Meslier et al. Citation2020; Johnstone et al. Citation2020). Similarly to Prevotella, Roseburia, Rumnicoccus, and Eubacterium rectale, belonging of the phylum Firmicutes, and Parabacteroides distasonis, belonging from Bacteroides, are known to ferment complex polysaccharides entering the colon to butyrate or succinate and acetate as a terminal product (Hillman et al. Citation2020; Ezeji et al. Citation2021; Steimle et al. Citation2021), and have demonstrated decreased in low-carb diets dependently by the carbohydrate total amount in cross-sectional studies (Duncan et al. Citation2007; Russell et al. Citation2011). Furthermore, Roseburia was strongly associated with a high Alternate Healthy Eating Index and Mediterranean Diet Score and a high intake of fruits, vegetables, legumes, and whole grains in the Cooperative Health Research in the Region of Augsburg (KORA) FF4 study (Breuninger et al. Citation2021). All these species seem to be a master signature of healthy diets, although their abundance could be modulated by the preexistent microbiota as well as mechanisms of resilience to return to an obesity microbiota phenotype (Xu and Knight Citation2015; Fragiadakis et al. Citation2020).
Interestingly, Faecalibacterium prausnitzii increased both after diets rich in fibers, including the MD (Lappi et al. Citation2013; Ferrocino et al. Citation2015; Haro et al. Citation2017; Roager et al. Citation2019; De Angelis et al. Citation2020; Meslier et al. Citation2020; Johnstone et al. Citation2020), and diets high in fats (Fava et al. Citation2013). F. prausnitzii, belonging to the Ruminococcaceae family (Clostridium cluster, Firmicutes), is one of the most abundant commensal bacteria, and was found to be a characteristic species also in subjects who followed a vegetarian diet (Ferrocino et al. Citation2015; De Angelis et al. Citation2020), in line with our results on fibers, and has recently been shown having the probiotic ability to produce vitamin B12 and hydrolyze lactulose and galacto-oligosaccharides increasing the content of fructose into the gut to support colonic epithelium growth (Cecchini et al. Citation2013; Fagundes et al. Citation2021). It has also been recognized as one of the most abundant butyrate producers in human feces (Cecchini et al. Citation2013). Since no more data than those in the papers we reviewed explain a role or modulation in relation to the dietary fat content, cross-feeding interactions with other species could be hypothesized (Kim Citation2020).
Although most of the reviews discussed the role of changes in SCFA production to drive some bacterial metabolic effects, we failed to observe a significant change in their quantity or balance in studies on the MD despite the fibers (Fava et al. Citation2013; Haro et al. Citation2016a, Citation2016b, Citation2017; Meslier et al. Citation2020). These findings suggest that the modulation of gut microbiota composition we discussed before may have a neutral effect. Phylogenetic changes may be functionally compensated for main metabolic end-products because other primary sources as amino acids could be used for SCFA production. On the other hand, SCFA decreased more often after dietary models were rich in fibers (Salonen et al. Citation2014; Vuholm et al. Citation2017; Mayengbam et al. Citation2019). Since fibers are known to increase SCFA production (Cummings and Macfarlane Citation1991), these findings in certain studies could result from a threshold effect of fiber intake or specific fiber subgroups that could differently modulate higher fermentation in the proximal colon, reduced fermentation in the distal one, or changes in the transit rate time (Govers et al. Citation1999). Unfortunately, the papers did not contain enough details to be conclusive on this point.
Two out of three studies evaluating bile acids reported a decrease in the content of both primary and secondary forms in stools after diet or foods rich in fibers (Haro et al. Citation2016b; Mayengbam et al. Citation2019; Meslier et al. Citation2020). Bile acids are synthesized from cholesterol and excreted through the stools. These findings in patients with obesity are in line with the demonstration that mixed fibers diluted bile acids by increasing fecal mass and transit time (Woodbury and Kern Jr, Woodbury and Kern Citation1971), and contrasting data could result from the content of insoluble fibers in the patients’ diet. The decrease in bile acids could be also related to a reduction in meat consumption when fibers were increased (Draper et al. Citation2018).
In general, the concordant decreased concentrations of BCFAs and the better metabolism of some phenolic compounds (Russell et al. Citation2011; Vitaglione et al., 2015; Desai et al. Citation2016; Beaumont et al. Citation2017; Mayengbam et al. Citation2019; Roager et al. Citation2019; Johnstone et al. Citation2020; Meslier et al. Citation2020; Benítez-Páez et al. Citation2021) suggested that both the MD and the healthy regimens could improve the inflammatory state of subjects with obesity and that some effects of diets are driven by microbiota functions.
Interestingly, microbiota composition at baseline or after interventions was associated with clinical and metabolic characteristics of the host.
Weight and weight decrease (BMI, waist circumference, trunk fat depending on the studies) was strongly associated with Prevotella abundance, mainly in the context of a high fiber diet (Fava et al. Citation2013; Christensen et al. Citation2013; Desai et al. Citation2016; Hjorth et al. Citation2018, Citation2020; Grembi et al. Citation2020; Benítez-Páez et al. Citation2021). Hjorth and coworkers proposed that Prevotella, in particular Prevotella copri – dominant in high-P/B subjects - has a greater potential to ferment dietary fiber than the low P/B subjects instead who had high abundances of Bacteroides spp. Moreover, an inverse association of the Prevotella genus with android fat post-weight-loss in older individuals, and an increased abundance of P. copri in high-responders was observed. Furthermore, subjects with a high P/B ratio lost more weight also in a low-carb diet regimen and regained less weight in the follow-up than those with a low P/B abundance (Hjorth et al. Citation2018, Citation2020). These results clearly indicated a post-weight-loss metabolic shift that included important changes in carbohydrate metabolism. In agreement with these findings, as stated before, other authors reported that subjects associated with a higher percentage of Bacteroides appeared to have lower fiber-degrading potential than the higher abundant Prevotella subjects (Vieira-Silva et al. Citation2019). This distinction might help to explain weight and metabolic differences in response to a fiber-rich, whole-grain diet.
The mechanisms through which Prevotella sp. could act on weight balance are still a matter of debate. Prevotella abundant patients may produce higher levels of SCFAs, propionate and succinate metabolism, that contribute to greater weight loss (Hjorth et al. Citation2020). Propionate is formed almost completely through the succinate pathway, characteristic of Bacteroidetes, including Prevotella (Reichardt et al. Citation2014; Precup and Vodnar Citation2019) and has been shown to be metabolized mainly in the liver. Its implication in reducing serum cholesterol and decreasing hepatic lipogenesis, acting on anti-inflammatory pathways, and preventing weight gain in overweight adult humans has been hypothesized based on consistent data in rodents (Chambers, Morrison, and Frost Citation2015). Furthermore, the potential role of propionate in enhancing satiety (Arora and Sharma Citation2011) is of increasing interest. Enhancing propionate production in the colon through dietary intervention with non-digestible carbohydrates is an attractive approach for increasing satiety and maintaining health and weight; however, in-depth knowledge of propionate producers within the gut microbiota is required for the development of tailored dietary strategies (Reichardt et al. Citation2014). Furthermore, very recently, a short intervention trial giving arabinoxylan-oligosaccharides to subjects with overweight has demonstrated that in individuals with low P/B ratio the prevalence of Bacteroides cellulosilyticus was the main predictor of body weight gain during the fiber rich diet and had an impact on host metabolism, mainly cholesterol levels, without changes in SCFAs (Christensen et al. Citation2020). Indeed, information beyond genus level could help in explaining mechanisms.
Weight was also negatively associated with genera Lachnospira and Oscillospira, whereas Faecalibacterium, Clostridiales, and Clostridium were positively associated with it in our results (Fava et al. Citation2013; Mayengbam et al. Citation2019). Although the studies we selected focused on these bacterial species, other components of the microbiota resulted to be associated with bodyweight loss in observational studies.
Dhakal and coworkers recently observed that high-responder subjects to losing weight with retail programs had a different abundance of Bacteroides eggerthii, Akkermansia muciniphila, Turicibacter sp., and Christensenella sp. (Dhakal, McCormack, and Dey Citation2020). Moreover, other species such as Lactobacillus and Bifidobacterium are currently used as probiotics and consumed for health promotion, including weight loss, although the evidence is still anecdotic or conflicting (Guazzelli Marques et al. Citation2020; Vallianou et al. Citation2020; Solito et al. Citation2021). All these findings suggest that microbiota composition is related to weight, but specific dietary interventions seem to modulate more frequently some phyla and, more importantly, species in obesity. Further studies are needed in populations with different microbiota signatures as well as studies with shotgun sequencing techniques to provide further insights and obtain consensus signals supporting a coherent and noteworthy association between diet, gut microbiota and health (Walters, Xu, and Knight Citation2014; Sze and Schloss Citation2016; Bradley, Nayfach, and Pollard Citation2018; Magne et al. Citation2020; Manor et al. Citation2020).
In contrast with data on weight, an improvement in insulin resistance in subjects on the MD was related to a decrease in Prevotella copri (Meslier et al. Citation2020) in line with other authors that reported a detrimental role of it on glucose metabolism and insulin resistance (Pedersen et al. Citation2016). On the other hand, a Swedish, high-fiber, barley-kernel intervention was found to improve glucose metabolisms among subjects with high Prevotella abundances, and P. copri was highly abundant in the metagenome of responders, probably because it promotes glycogen storage into the liver (Kovatcheva-Datchary et al. Citation2015), and similar findings have been reported recently in the PREDICT-1 study (Asnicar et al. Citation2021). These differences could be linked to the nature of the studies (randomized vs observational), the selected cohorts, and the diet-driven selection on P. copri machinery. It has been shown that P. copri strains related to an omnivorous diet have a high prevalence of genes involved in the branched-chain amino acid synthesis, a risk factor for glucose intolerance, and type 2 diabetes mellitus (Tett et al. Citation2021; Beaumont and Blachier Citation2020). This hypothesis is in line with the better metabolism of some phenolic compounds after a MD discussed before (Russell et al. Citation2011; Vitaglione et al., 2015; Beaumont et al. Citation2017; Mayengbam et al. Citation2019; Roager et al. Citation2019; Johnstone et al. Citation2020; Meslier et al. Citation2020). To help in clarifying all these results, a recent study has shown that P. copri is not a monotypic species but encompasses four distinct clades (A, B, C, and D) with functional diversity in carbohydrate metabolism (Fehlner-Peach et al. Citation2019; Tett et al. Citation2019). Since western diet demises its abundance, P. copri seems to confer a host metabolic benefit under a fiber-rich diet, but not if fats are abundant (Pedersen et al. Citation2016; Fehlner-Peach et al. Citation2019; Tett et al. Citation2019). These data shed light on the pivotal role also of the source and subtype of macronutrient substrates and considering them in studies will contribute to pitch microbiota-diseases signatures (Fehlner-Peach et al. Citation2019; Tett et al. Citation2019), as also discussed before regarding B. cellulosilyticus (Christensen et al. Citation2020). On the other hand, the apparently discrepant results about Prevotella abundance on weight and P. copri on the glucose metabolism stress again the interpretative bias caused by different methodologies and the lack of correspondence with health of too generalist microbial categories.
A decrease in Holdemania was associated with amelioration in glucose levels during an oral glucose tolerance test on pea fiber diet (Mayengbam et al. Citation2019), in agreement with another report (Lippert et al. Citation2017). Holdemania, a Firmicutes belonging to the family Erysipelotrichaceae, is capable of fermenting sugars such as fructose, glucose, and sucrose but not complex carbohydrates including polyols or starch (Willems et al. Citation1997). It also contributes to the breakdown of the mucin layer (Raimondi et al. Citation2021). We can hypothesize that a diet rich in fibers favors species with metabolism for complex carbohydrates with a parallel drop of relative abundance of others as Holdemania that could contribute to the absorption and metabolism of sugars and leaky gut. Furthermore, generally healthy dietary patterns were associated with a reduction of inflammatory markers. These findings are all concordant with other research indicating that the modification of the microbiota in various diseases, in these cases diet-driven, can blunt inflammation and improve the metabolic profile, with a signature related to the disease and the intervention (Barber et al. Citation2021).
Last, subjects with the worse metabolic profile at the starting of the trials were those who presented the most significant taxonomic changes and parallel metabolic improvement (Haro et al. Citation2016b, Citation2017). This means that subjects with the worse phenotype are those characterized by a more pronounced reduction in the abundance of genera with saccharolytic activity, which likely leads to the production of energy from amino acids and, therefore, branch chain amino acids that impair glucose metabolism as discussed before. These results strengthen the importance of strongly promoting chances in lifestyle habits in subjects with obesity and related diseases.
Some limits exist regarding our data since populations and dietary intervention are heterogeneous, but the risk of bias was generally low. Most of the currently published papers are observational and often conflicting. Controlled clinical interventions are spare, but this review aimed to organize the body of evidence on microbiota deriving from dietary trials in adult obesity giving the most certain signatures that should lead to further studies.
In conclusion, our findings support the importance of tailored dietary approaches to improve host metabolism as well as microbiota composition and function in obesity. Some phyla and species are more strictly related to diets rich in fibers and others to healthy diets. Changes in weight and metabolism are related to different microorganisms or functions and should be approached with in-depth further trials. The Firmicutes/Bacteroidetes ratio, so cited in many observational studies on obesity, is frequently a wrong or misleading conception to provide any association with health status, due to lack of the evaluation of masked factors, first of all diet and niche competitiveness. We encourage researchers to use more specific metrics, focusing on genus and species levels, and ever consider diet. Deeply identifying associations among host lifestyle factors, health, and microbiota signatures at genus and species levels can enable to design the roadmap of personalized interventions and clinical trials. These data emphasize the possibility of using a symbiotic approach involving both tailored diet, microbiota changes, and maybe drugs to treat obesity and metabolic disorders.
Acknowledgements
The Authors should thank Daniele Spadaccini, and Sabrina Tini for their technical assistance.
Disclosure statement
The Authors have no conflict of interests that could have influenced the objective reports of the results.
Additional information
Funding
References
- Arora, T., and R. Sharma. 2011. Fermentation potential of the gut microbiome: Implications for energy homeostasis and weight management. Nutrition Reviews 69 (2):99–106. doi: 10.1111/j.1753-4887.2010.00365.x.
- Asnicar, F., S. E. Berry, A. M. Valdes, L. H. Nguyen, G. Piccinno, D. A. Drew, E. Leeming, R. Gibson, C. L. Roy, H. A. Khatib, et al. 2021. Microbiome connections with host metabolism and habitual diet from 1,098 deeply phenotyped individuals. Nature Medicine 27 (2):321–32. doi: 10.1038/s41591-020-01183-8.
- Babio, N., E. Toledo, R. Estruch, E. Ros, M. A. Martínez-González, O. Castañer, M. Bulló, D. Corella, F. Arós, E. Gómez-Gracia, et al. 2014. Mediterranean diets and metabolic syndrome status in the PREDIMED randomized trial. Canadian Medical Association Journal 186 (17):E649–57. doi: 10.1503/cmaj.140764.
- Barber, T. M., G. Valsamakis, G. Mastorakos, P. Hanson, I. Kyrou, H. S. Randeva, and M. O. Weickert. 2021. Dietary influences on the microbiota-gut-brain axis. International Journal of Molecular Sciences 22 (7):3502. doi: 10.3390/ijms22073502.
- Beaumont, M., and F. Blachier. 2020. Amino acids in intestinal physiology and health. Advances in Experimental Medicine and Biology 1265:1–20. doi: 10.1007/978-3-030-45328-2_1.
- Beaumont, M., K. J. Portune, N. Steuer, A. Lan, V. Cerrudo, M. Audebert, F. Dumont, G. Mancano, N. Khodorova, M. Andriamihaja, et al. 2017. Quantity and source of dietary protein influence metabolite production by gut microbiota and rectal mucosa gene expression: A randomized, parallel, double-blind trial in overweight humans. The American Journal of Clinical Nutrition 106 (4):1005–19. doi: 10.3945/ajcn.117.158816.
- Bendtsen, L. Q., T. Blaedel, J. B. Holm, J. K. Lorenzen, A. B. Mark, P. Kiilerich, K. Kristiansen, A. Astrup, and L. H. Larsen. 2018. High intake of dairy during energy restriction does not affect energy balance or the intestinal microflora compared with low dairy intake in overweight individuals in a randomized controlled trial. Applied Physiology, Nutrition, and Metabolism = Physiologie Appliquee, Nutrition et Metabolisme 43 (1):1–10. doi: 10.1139/apnm-2017-0234.
- Benítez-Páez, A., A. L. Hess, S. Krautbauer, G. Liebisch, L. Christensen, M. F. Hjorth, T. M. Larsen, and Y. Sanz, MyNewGut consortium. 2021. Sex, food, and the gut microbiota: Disparate response to caloric restriction diet with fiber supplementation in women and men. Molecular Nutrition & Food Research 65 (8):e2000996. doi: 10.1002/mnfr.202000996.
- Blumberg, J., R. P. Heaney, M. Huncharek, T. Scholl, M. Stampfer, R. Vieth, C. M. Weaver, and S. H. Zeisel. 2010. Evidence-based criteria in the nutritional context. Nutrition Reviews 68 (8):478–84. doi: 10.1111/j.1753-4887.2010.00307.x.
- Bradley, P. H., S. Nayfach, and K. S. Pollard. 2018. Phylogeny-corrected identification of microbial gene families relevant to human gut colonization. PLoS Computational Biology 14 (8):e1006242. doi: 10.1371/journal.pcbi.1006242.
- Breuninger, T. A., N. Wawro, J. Breuninger, S. Reitmeier, T. Clavel, J. Six-Merker, G. Pestoni, S. Rohrmann, W. Rathmann, A. Peters, et al. 2021. Associations between habitual diet, metabolic disease, and the gut microbiota using latent Dirichlet allocation. Microbiome 9 (1):61. doi: 10.1186/s40168-020-00969-9.
- Cani, P. D. 2019. Microbiota and metabolites in metabolic diseases. Nature Reviews. Endocrinology 15 (2):69–70. doi: 10.1038/s41574-018-0143-9.
- Cani, P. D., and C. Knauf. 2016. How gut microbes talk to organs: The role of endocrine and nervous routes. Molecular Metabolism 5 (9):743–52. doi: 10.1016/j.molmet.2016.05.011.
- Cecchini, D. A., E. Laville, S. Laguerre, P. Robe, M. Leclerc, J. Doré, B. Henrissat, M. Remaud-Siméon, P. Monsan, and G. Potocki-Véronèse. 2013. Functional metagenomics reveals novel pathways of prebiotic breakdown by human gut bacteria. PLoS One 8 (9):e72766. doi: 10.1371/journal.pone.0072766.
- Chambers, E. S., D. J. Morrison, and G. Frost. 2015. Control of appetite and energy intake by SCFA: What are the potential underlying mechanisms? The Proceedings of the Nutrition Society 74 (3):328–36. doi: 10.1017/S0029665114001657.
- Christensen, E. G., T. R. Licht, M. Kristensen, and M. I. Bahl. 2013. Bifidogenic effect of whole-grain wheat during a 12-week energy-restricted dietary intervention in postmenopausal women. European Journal of Clinical Nutrition 67 (12):1316–21. doi: 10.1038/ejcn.2013.207.
- Christensen, L., C. V. Sørensen, F. U. Wøhlk, L. Kjølbaek, A. Astrup, Y. Sanz, M. F. Hjorth, and A. Benítez-Páez. 2020. Microbial enterotypes beyond genus level: Bacteroides species as a predictive biomarker for weight change upon controlled intervention with arabinoxylan oligosaccharides in overweight subjects. Gut Microbes 12 (1):1847627. doi: 10.1080/19490976.2020.1847627.
- Cummings, J. H., and G. T. Macfarlane. 1991. The control and consequences of bacterial fermentation in the human colon. The Journal of Applied Bacteriology 70 (6):443–59. doi: 10.1111/j.1365-2672.1991.tb02739.x.
- David, L. A., C. F. Maurice, R. N. Carmody, D. B. Gootenberg, J. E. Button, B. E. Wolfe, A. V. Ling, A. S. Devlin, Y. Varma, M. A. Fischbach, et al. 2014. Diet rapidly and reproducibly alters the human gut microbiome. Nature 505 (7484):559–63. doi: 10.1038/nature12820.
- De Angelis, M., I. Ferrocino, F. M. Calabrese, F. De Filippis, N. Cavallo, S. Siragusa, S. Rampelli, R. D. Cagno, K. Rantsiou, L. Vannini, et al. 2020. Diet influences the functions of the human intestinal microbiome. Scientific Reports 10 (1):4247. doi: 10.1038/s41598-020-61192-y.
- Desai, M. S., A. M. Seekatz, N. M. Koropatkin, N. Kamada, C. A. Hickey, M. Wolter, N. A. Pudlo, S. Kitamoto, N. Terrapon, A. Muller, et al. 2016. A dietary fiber-deprived gut microbiota degrades the colonic mucus barrier and enhances pathogen susceptibility. Cell 167 (5):1339–53.e21. doi: 10.1016/j.cell.2016.10.043.
- Dhakal, S., L. McCormack, and M. Dey. 2020. Association of the gut microbiota with weight-loss response within a retail weight-management program. Microorganisms. 8 (8):1246. doi: 10.3390/microorganisms8081246.
- Draper, C. F., I. Vassallo, A. D. Cara, C. Milone, O. Comminetti, I. Monnard, J. P. Godin, M. Scherer, M. Su, W. Jia, et al. 2018. A 48-hour vegan diet challenge in healthy women and men induces a BRANCH-chain amino acid related health associated, metabolic signature. Molecular Nutrition & Food Research 62 (3) doi: 10.1002/mnfr.201700703.
- Duncan, S. H., A. Belenguer, G. Holtrop, A. M. Johnstone, H. J. Flint, and G. E. Lobley. 2007. Reduced dietary intake of carbohydrates by obese subjects results in decreased concentrations of butyrate and butyrate-producing bacteria in feces. Applied and Environmental Microbiology 73 (4):1073–8. doi: 10.1128/AEM.02340-06.
- Estruch, R., E. Ros, J. Salas-Salvadó, M. I. Covas, D. Corella, F. Arós, E. Gómez-Gracia, V. Ruiz-Gutiérrez, M. Fiol, J. Lapetra, et al. 2018. Primary prevention of cardiovascular disease with a mediterranean diet supplemented with extra-virgin olive oil or nuts. New England Journal of Medicine 378 (25):e34. doi: 10.1056/NEJMoa1800389.
- Ezeji, J. C., D. K. Sarikonda, A. Hopperton, H. L. Erkkila, D. E. Cohen, S. P. Martinez, F. Cominelli, T. Kuwahara, A. E. K. Dichosa, C. E. Good, et al. 2021. Parabacteroides distasonis: Intriguing aerotolerant gut anaerobe with emerging antimicrobial resistance and pathogenic and probiotic roles in human health. Gut Microbes 13 (1):1922241. doi: 10.1080/19490976.2021.1922241.
- Fagundes, R. R., A. R. Bourgonje, A. Saeed, A. Vich Vila, N. Plomp, T. Blokzijl, M. Sadaghian Sadabad, J. Z. H. von Martels, S. S. van Leeuwen, R. K. Weersma, et al. 2021. Inulin-grown Faecalibacterium prausnitzii cross-feeds fructose to the human intestinal epithelium. Gut Microbes 13 (1):1993582. doi: 10.1080/19490976.2021.1993582.
- Fava, F., R. Gitau, B. A. Griffin, G. R. Gibson, K. M. Tuohy, and J. A. Lovegrove. 2013. The type and quantity of dietary fat and carbohydrate alter faecal microbiome and short-chain fatty acid excretion in a metabolic syndrome ‘at-risk’ population. International Journal of Obesity 37 (2):216–23. doi: 10.1038/ijo.2012.33.
- Ferrocino, I., R. D. Cagno, M. De Angelis, S. Turroni, L. Vannini, E. Bancalari, K. Rantsiou, G. Cardinali, E. Neviani, and L. Cocolin. 2015. Fecal microbiota in healthy subjects following omnivore, vegetarian and vegan diets: Culturable populations and rRNA DGGE Profiling. PLoS One 10 (6):e0128669. doi: 10.1371/journal.pone.0128669.
- Fehlner-Peach, H., C. Magnabosco, V. Raghavan, J. U. Scher, A. Tett, L. M. Cox, C. Gottsegen, A. Watters, J. D. Wiltshire-Gordon, N. Segata, et al. 2019. Distinct polysaccharide utilization profiles of human intestinal prevotella copri isolates. Cell Host & Microbe 26 (5):680–90.e5. doi: 10.1016/j.chom.2019.10.013.
- Fragiadakis, G. K., H. C. Wastyk, J. L. Robinson, E. D. Sonnenburg, J. L. Sonnenburg, and C. D. Gardner. 2020. Long-term dietary intervention reveals resilience of the gut microbiota despite changes in diet and weight. The American Journal of Clinical Nutrition 111 (6):1127–36. doi: 10.1093/ajcn/nqaa046.
- Govers, M. J., N. J. Gannon, F. R. Dunshea, P. R. Gibson, and J. G. Muir. 1999. Wheat bran affects the site of fermentation of resistant starch and luminal indexes related to colon cancer risk: A study in pigs. Gut 45 (6):840–7. doi: 10.1136/gut.45.6.840.
- Grembi, J. A., L. H. Nguyen, T. D. Haggerty, C. D. Gardner, S. P. Holmes, and J. Parsonnet. 2020. Gut microbiota plasticity is correlated with sustained weight loss on a low-carb or low-fat dietary intervention. Scientific Reports 10 (1):1405. doi: 10.1038/s41598-020-58000-y.
- Grosso, G., A. Micek, J. Godos, A. Pajak, S. Sciacca, F. Galvano, and P. Boffetta. 2017. Health risk factors associated with meat, fruit and vegetable consumption in cohort studies: A comprehensive meta-analysis. PLoS One 12 (8):e0183787. doi: 10.1371/journal.pone.0183787.
- Guazzelli Marques, C., A. de Piano Ganen, A. Zaccaro de Barros, R. V. Thomatieli Dos Santos, and M. V. L. Dos Santos Quaresma. 2020. Weight loss probiotic supplementation effect in overweight and obesity subjects: A review. Clinical Nutrition (Edinburgh, Scotland) 39 (3):694–704. doi: 10.1016/j.clnu.2019.03.034.
- Haro, C., M. Montes-Borrego, O. A. Rangel-Zúñiga, J. F. Alcalá-Díaz, F. Gómez-Delgado, P. Pérez-Martínez, J. Delgado-Lista, G. M. Quintana-Navarro, F. J. Tinahones, B. B. Landa, et al. 2016a. Two healthy diets modulate gut microbial community improving insulin sensitivity in a human obese population. The Journal of Clinical Endocrinology and Metabolism 101 (1):233–42. doi: 10.1210/jc.2015-3351.
- Haro, C., S. Garcia-Carpintero, J. F. Alcala-Diaz, F. Gomez-Delgado, J. Delgado-Lista, P. Perez-Martinez, O. A. Rangel Zuñiga, G. M. Quintana-Navarro, B. B. Landa, J. C. Clemente, et al. 2016b. The gut microbial community in metabolic syndrome patients is modified by diet. Journal of Nutritional Biochemistry. 27:27–31. doi: 10.1016/j.jnutbio.2015.08.011.
- Haro, C., S. García-Carpintero, O. A. Rangel-Zúñiga, J. F. Alcalá-Díaz, B. B. Landa, J. C. Clemente, P. Pérez-Martínez, J. López-Miranda, F. Pérez-Jiménez, and A. Camargo. 2017. Consumption of two healthy dietary patterns restored microbiota dysbiosis in obese patients with metabolic dysfunction. Molecular Nutrition & Food Research. (12):61. doi: 10.1002/mnfr.201700300.
- Hillman, E. T., A. J. Kozik, C. A. Hooker, J. L. Burnett, Y. Heo, V. A. Kiesel, C. J. Nevins, J. Oshiro, M. M. Robins, and R. D. Thakkar. 2020. Comparative genomics of the genus Roseburia reveals divergent biosynthetic pathways that may influence colonic competition among species. Microb Genom 6 (7):mgen000399. doi: 10.1099/mgen.0.000399.
- Hjorth, M. F., H. M. Roager, T. M. Larsen, S. K. Poulsen, T. R. Licht, M. I. Bahl, Y. Zohar, and A. Astrup. 2018. Pre-treatment microbial Prevotella-to-Bacteroides ratio, determines body fat loss success during a 6-month randomized controlled diet intervention. International Journal of Obesity ). 42 (3):580–3. doi: 10.1038/ijo.2017.220.
- Hjorth, M. F., L. Christensen, T. M. Larsen, H. M. Roager, L. Krych, W. Kot, D. S. Nielsen, C. Ritz, and A. Astrup. 2020. Pretreatment Prevotella-to-Bacteroides ratio and salivary amylase gene copy number as prognostic markers for dietary weight loss. The American Journal of Clinical Nutrition 111 (5):1079–86. doi: 10.1093/ajcn/nqaa007.
- Johnston, B. C., S. Kanters, K. Bandayrel, P. Wu, F. Naji, R. A. Siemieniuk, G. D. Ball, J. W. Busse, K. Thorlund, G. Guyatt, et al. 2014. Comparison of weight loss among named diet programs in overweight and obese adults: A meta-analysis. JAMA 312 (9):923–33. doi: 10.1001/jama.2014.10397.
- Johnstone, A. M., J. Kelly, S. Ryan, R. Romero-Gonzalez, H. McKinnon, C. Fyfe, E. Naslund, R. Lopez-Nicolas, D. Bosscher, A. Bonnema, et al. 2020. Nondigestible carbohydrates affect metabolic health and gut microbiota in overweight adults after weight loss. The Journal of Nutrition 150 (7):1859–70. PMID: 32510158. doi: 10.1093/jn/nxaa124.
- Kim, H. S. 2020. Microbiota in Rosacea. American Journal of Clinical Dermatology 21 (Suppl 1):25–35. doi: 10.1007/s40257-020-00546-8.
- Kopf, J. C., M. J. Suhr, J. Clarke, S. I. Eyun, J. M. Riethoven, A. E. Ramer-Tait, and D. J. Rose. 2018. Role of whole grains versus fruits and vegetables in reducing subclinical inflammation and promoting gastrointestinal health in individuals affected by overweight and obesity: A randomized controlled trial. Nutrition Journal 17 (1):72. doi: 10.1186/s12937-018-0381-7.
- Kovatcheva-Datchary, P., A. Nilsson, R. Akrami, Y. S. Lee, F. De Vadder, T. Arora, A. Hallen, E. Martens, I. Björck, and F. Bäckhed. 2015. Dietary fiber-induced improvement in glucose metabolism is associated with increased abundance of Prevotella. Cell Metabolism 22 (6):971–82. doi: 10.1016/j.cmet.2015.10.001.
- Lappi, J., M. Kolehmainen, H. Mykkänen, and K. Poutanen. 2013. Do large intestinal events explain the protective effects of whole grain foods against type 2 diabetes? Critical Reviews in Food Science and Nutrition 53 (6):631–40. doi: 10.1080/10408398.2010.550388.
- Lean, M. E., W. S. Leslie, A. C. Barnes, N. Brosnahan, G. Thom, L. McCombie, C. Peters, S. S. Zhyzhneuskaya, A. Al-Mrabeh, K. G. Hollingsworth, et al. 2018. Primary care-led weight management for remission of type 2 diabetes (DiRECT): An open-label, cluster-randomised trial. The Lancet 391 (10120):541–51. doi: 10.1016/S0140-6736(17)33102-1.
- Ley, R. E., P. J. Turnbaugh, S. Klein, and J. I. Gordon. 2006. Microbial ecology: Human gut microbes associated with obesity. Nature 444 (7122):1022–3. doi: 10.1038/4441022a.
- Lippert, K., L. Kedenko, L. Antonielli, I. Kedenko, C. Gemeier, M. Leitner, A. Kautzky-Willer, B. Paulweber, and E. Hackl. 2017. Gut microbiota dysbiosis associated with glucose metabolism disorders and the metabolic syndrome in older adults. Beneficial Microbes 8 (4):545–56. doi: 10.3920/BM2016.0184.
- Magne, F., M. Gotteland, L. Gauthier, A. Zazueta, S. Pesoa, P. Navarrete, and R. Balamurugan R. 2020. The firmicutes/bacteroidetes ratio: A relevant marker of gut dysbiosis in obese patients? Nutrients 12 (5):1474. doi: 10.3390/nu12051474.
- Manor, O., C. L. Dai, S. A. Kornilov, B. Smith, N. D. Price, J. C. Lovejoy, S. M. Gibbons, and A. T. Magis. 2020. Health and disease markers correlate with gut microbiome composition across thousands of people. Nature Communications 11 (1):5206. doi: 10.1038/s41467-020-18871-1.
- Mansoor, N., K. J. Vinknes, M. B. Veierød, and K. Retterstøl. 2016. Effects of low-carbohydrate diets v. low-fat diets on body weight and cardiovascular risk factors: A meta-analysis of randomised controlled trials. British Journal of Nutrition. 115 (3):466–79. doi: 10.1017/S0007114515004699.
- Mayengbam, S., J. E. Lambert, J. A. Parnell, J. M. Tunnicliffe, A. C. Nicolucci, J. Han, T. Sturzenegger, J. Shearer, B. Mickiewicz, H. J. Vogel, et al. 2019. Impact of dietary fiber supplementation on modulating microbiota-host-metabolic axes in obesity. The Journal of Nutritional Biochemistry 64:228–36. doi: 10.1016/j.jnutbio.2018.11.003.
- McGuinness, L. A., and J. P. T. Higgins. 2021. Risk-of-bias VISualization (robvis): An R package and Shiny web app for visualizing risk-of-bias assessments. Research Synthesis Methods 12 (1):55–61. doi: 10.1002/jrsm.1411.
- Meslier, V., M. Laiola, H. M. Roager, F. De Filippis, H. Roume, B. Quinquis, R. Giacco, I. Mennella, R. Ferracane, N. Pons, et al. 2020. Mediterranean diet intervention in overweight and obese subjects lowers plasma cholesterol and causes changes in the gut microbiome and metabolome independently of energy intake. Gut 69 (7):1258–68. doi: 10.1136/gutjnl-2019-320438.
- Moher, D., L. Shamseer, M. Clarke, D. Ghersi, A. Liberati, M. Petticrew, P. Shekelle, and L. A. Stewart, PRISMA-P Group. 2015. Preferred reporting items for systematic review and meta-analysis protocols (PRISMA-P) 2015 statement. Systematic Reviews 4 (1):1. doi: 10.1186/2046-4053-4-1.
- Ng, M., T. Fleming, M. Robinson, B. Thomson, N. Graetz, C. Margono, E. C. Mullany, S. Biryukov, C. Abbafati, S. F. Abera, et al. 2014. Global, regional, and national prevalence of overweight and obesity in children and adults during 1980-2013: A systematic analysis for the Global Burden of Disease Study 2013. Lancet (London, England) 384 (9945):766–81. doi: 10.1016/S0140-6736(14)60460-8.
- Pace, L. A., and S. E. Crowe. 2016. Complex relationships between food, diet, and the microbiome. Gastroenterology Clinics of North America 45 (2):253–65. doi: 10.1016/j.gtc.2016.02.004.
- Pedersen, H. K., V. Gudmundsdottir, H. B. Nielsen, T. Hyotylainen, T. Nielsen, B. A. Jensen, K. Forslund, F. Hildebrand, E. Prifti, G. Falony, MetaHIT Consortium, et al. 2016. Human gut microbes impact host serum metabolome and insulin sensitivity. Nature 535 (7612):376–81. doi: 10.1038/nature18646.
- Precup, G., and D. C. Vodnar. 2019. Gut Prevotella as a possible biomarker of diet and its eubiotic versus dysbiotic roles: A comprehensive literature review. The British Journal of Nutrition 122 (2):131–40. doi: 10.1017/S0007114519000680.
- Raimondi, S., E. Musmeci, F. Candeliere, A. Amaretti, and M. Rossi. 2021. Identification of mucin degraders of the human gut microbiota. Scientific Reports 11 (1):11094. doi: 10.1038/s41598-021-90553-4.
- Reichardt, N., S. H. Duncan, P. Young, A. Belenguer, C. McWilliam Leitch, K. P. Scott, H. J. Flint, and P. Louis. 2014. Phylogenetic distribution of three pathways for propionate production within the human gut microbiota. The ISME Journal 8 (6):1323–35. doi: 10.1038/ismej.2014.14.
- Roager, H. M., J. K. Vogt, M. Kristensen, L. B. S. Hansen, S. Ibrügger, R. B. Maerkedahl, M. I. Bahl, M. V. Lind, R. L. Nielsen, H. Frøkiaer, et al. 2019. Whole grain-rich diet reduces body weight and systemic low-grade inflammation without inducing major changes of the gut microbiome: A randomised cross-over trial. Gut 68 (1):83–93. doi: 10.1136/gutjnl-2017-314786.
- Russell, W. R., S. W. Gratz, S. H. Duncan, G. Holtrop, J. Ince, L. Scobbie, G. Duncan, A. M. Johnstone, G. E. Lobley, R. J. Wallace, et al. 2011. High-protein, reduced-carbohydrate weight-loss diets promote metabolite profiles likely to be detrimental to colonic health. The American Journal of Clinical Nutrition 93 (5):1062–72. doi: 10.3945/ajcn.110.002188.
- Salas-Salvadó, J., J. Fernández-Ballart, E. Ros, M. A. Martínez-González, M. Fitó, R. Estruch, D. Corella, M. Fiol, E. Gómez-Gracia, F. Arós, PREDIMED Study Investigators, et al. 2008. Effect of a Mediterranean diet supplemented with nuts on metabolic syndrome status: One-year results of the PREDIMED randomized trial. Archives of Internal Medicine 168 (22):2449–58. doi: 10.1001/archinte.168.22.2449.
- Salonen, A., L. Lahti, J. Salojärvi, G. Holtrop, K. Korpela, S. H. Duncan, P. Date, F. Farquharson, A. M. Johnstone, G. E. Lobley, et al. 2014. Impact of diet and individual variation on intestinal microbiota composition and fermentation products in obese men. The ISME Journal 8 (11):2218–30. doi: 10.1038/ismej.2014.63.
- Schutte, S., D. Esser, F. P. M. Hoevenaars, G. Hooiveld, M. G. Priebe, R. J. Vonk, S. Wopereis, and L. A. Afman. 2018. A 12-wk whole-grain wheat intervention protects against hepatic fat: The Graandioos study, a randomized trial in overweight subjects. The American Journal of Clinical Nutrition 108 (6):1264–74. doi: 10.1093/ajcn/nqy204.
- Shamseer, L., D. Moher, M. Clarke, D. Ghersi, A. Liberati, M. Petticrew, P. Shekelle, and L. A. Stewart, PRISMA-P Group. 2015. Preferred reporting items for systematic review and meta-analysis protocols (PRISMA-P) 2015: Elaboration and explanation. BMJ (Clinical Research ed.) 350:g7647. doi: 10.1136/bmj.g7647.
- Solito, A., N. Bozzi Cionci, M. Calgaro, M. Caputo, L. Vannini, I. Hasballa, F. Archero, E. Giglione, R. Ricotti, G. E. Walker, et al. 2021. Supplementation with Bifidobacterium breve BR03 and B632 strains improved insulin sensitivity in children and adolescents with obesity in a cross-over, randomized double-blind placebo-controlled trial. Clinical Nutrition 40 (7):4585–94. doi: 10.1016/j.clnu.2021.06.002.
- Steimle, A., M. Neumann, E. T. Grant, J. D. Turner, and M. S. Desai. 2021. Concentrated raw fibers enhance the fiber-degrading capacity of a synthetic human gut microbiome. International Journal of Molecular Sciences 22 (13):6855. doi: 10.3390/ijms22136855.
- Sterne, J. A. C., J. Savović, M. J. Page, R. G. Elbers, N. S. Blencowe, I. Boutron, C. J. Cates, H. Y. Cheng, M. S. Corbett, S. M. Eldridge, et al. 2019. RoB 2: A revised tool for assessing risk of bias in randomised trials. BMJ (Clinical Research ed.) 366:l4898. doi: 10.1136/bmj.l4898.
- Sze, M. A., and P. D. Schloss. 2016. Looking for a signal in the noise: Revisiting obesity and the microbiome. mBio 7 (4):e01018–16. doi: 10.1128/mBio.01018-16.
- Tett, A., K. D. Huang, F. Asnicar, H. Fehlner-Peach, E. Pasolli, N. Karcher, F. Armanini, P. Manghi, K. Bonham, M. Zolfo, et al. 2019. The Prevotella copri complex comprises four distinct clades underrepresented in westernized populations. Cell Host & Microbe. 26 (5):666–79.e7. doi: 10.1016/j.chom.2019.08.018.
- Tett, A., E. Pasolli, G. Masetti, D. Ercolini, and N. Segata. 2021. Prevotella diversity, niches and interactions with the human host. Nature Reviews. Microbiology 19 (9):585–99. doi: 10.1038/s41579-021-00559-y.
- Tremmel, M., U. G. Gerdtham, P. M. Nilsson, and S. Saha. 2017. Economic burden of obesity: A systematic literature review. International Journal of Environmental Research and Public Health 14 (4):435. doi: 10.3390/ijerph14040435.
- Turnbaugh, P. J., R. E. Ley, M. A. Mahowald, V. Magrini, E. R. Mardis, and J. I. Gordon. 2006. An obesity-associated gut microbiome with increased capacity for energy harvest. Nature 444 (7122):1027–31. doi: 10.1038/nature05414.
- Vallianou, N., T. Stratigou, G. S. Christodoulatos, C. Tsigalou, and M. Dalamaga. 2020. Probiotics, prebiotics, synbiotics, postbiotics, and obesity: Current evidence, controversies, and perspectives. Current Obesity Reports 9 (3):179–92. doi: 10.1007/s13679-020-00379-w.
- Vieira-Silva, S., J. Sabino, M. Valles-Colomer, G. Falony, G. Kathagen, C. Caenepeel, I. Cleynen, S. van der Merwe, S. Vermeire, and J. Raes. 2019. Quantitative microbiome profiling disentangles inflammation- and bile duct obstruction-associated microbiota alterations across PSC/IBD diagnoses. Nature Microbiology 4 (11):1826–31. doi: 10.1038/s41564-019-0483-9.
- Vitaglione, P., I. Mennella, R. Ferracane, A. A. Rivellese, R. Giacco, D. Ercolini, S. M. Gibbons, A. La Storia, J. A. Gilbert, S. Jonnalagadda, et al. 2015. Whole-grain wheat consumption reduces inflammation in a randomized controlled trial on overweight and obese subjects with unhealthy dietary and lifestyle behaviors: Role of polyphenols bound to cereal dietary fiber. The American Journal of Clinical Nutrition 101 (2):251–61. doi: 10.3945/ajcn.114.088120.
- Vuholm, S., D. S. Nielsen, K. N. Iversen, J. Suhr, P. Westermann, L. Krych, J. R. Andersen, and M. Kristensen. 2017. Whole-grain rye and wheat affect some markers of gut health without altering the fecal microbiota in healthy overweight adults: A 6-week randomized trial. The Journal of Nutrition 147 (11):2067–75. doi: 10.3945/jn.117.250647.
- Walker, A. W., J. Ince, S. H. Duncan, L. M. Webster, G. Holtrop, X. Ze, D. Brown, M. D. Stares, P. Scott, A. Bergerat, et al. 2011. Dominant and diet-responsive groups of bacteria within the human colonic microbiota. The ISME Journal 5 (2):220–30. doi: 10.1038/ismej.2010.118.
- Walters, W. A., Z. Xu, and R. Knight. 2014. Meta-analyses of human gut microbes associated with obesity and IBD. FEBS Letters 588 (22):4223–33. doi: 10.1016/j.febslet.2014.09.039.
- Willems, A., W. E. Moore, N. Weiss, and M. D. Collins. 1997. Phenotypic and phylogenetic characterization of some Eubacterium-like isolates containing a novel type B wall murein from human feces: Description of Holdemania filiformis gen. nov., sp. nov. International Journal of Systematic Bacteriology 47 (4):1201–4. doi: 10.1099/00207713-47-4-1201.
- Woodbury, J. F., and F. Kern. Jr. 1971. Fecal excretion of bile acids: A new technique for studying bile acid kinetics in patients with ileal resection. Journal of Clinical Investigation 50 (12):2531–40. doi: 10.1172/JCI106753.
- Xu, Z., and R. Knight. 2015. Dietary effects on human gut microbiome diversity. The British Journal of Nutrition 113 Suppl (Suppl 0):S1–S5. doi: 10.1017/S0007114514004127.