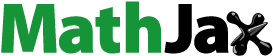
ABSTRACT
Brazil is one of the largest suppliers of commodities in the world, partly due to the agricultural expansion in the Cerrado biome that began in the 1970s. However, as areas with better soil and climate for agriculture become scarce, farmers advanced to marginal lands, where precipitation is less reliable for rainfed crops. The overall goal of this paper is to investigate the implications of occupying drier areas in the Cerrado biome, which are likely to become more so in the future. We estimated the effect of temperature and precipitation on soybean yields (kg/ha) using panel data regression at the municipal level, from 1980 to 2016, and at the farm-level in a subset region known as Matopiba. Our analysis estimated a reduction of 6% in soybean yield for each 1°C increase in temperature. According to interviewed farmers, the consequences of the recent droughts include land concentration and increased indebtedness. Based on historical observations, agricultural technologies do not provide adaptation to extreme heat and created a dependency on capital availability for investments in irrigation, raising questions about the future sustainability of this capital-intensive agricultural system if breakthroughs in adaptation do not occur.
1. Introduction
Brazil is one of the largest suppliers of commodities in the world, partly due to the agricultural expansion in the Brazilian savannas (also known as Cerrado) since 1970, made possible by the green revolution (Brum, Citation1988). The Cerrado is also a hotspot for environmental conservation (Mittermeier et al., Citation2011) and the fastest-growing agricultural region in the world (Graesser et al., Citation2015). The recent agricultural expansion in the Cerrado occurred mainly for soybean farming and accounts for 50% of the total planted area in 2018 (IBGE, Citation2018). However, as areas with better soil for agriculture become scarce, farmers advance to the ecotone between the savanna and the caatinga, a semi-arid environment where precipitation is less reliable. This has exposed farmers to increasing risks of financial hardships (Brito et al., Citation2018; IPCC, Citation2020) due to agribusinesses’ large capital investments. For instance, droughts in 2015/2016 reduced the average productivity by 33% in the Cerrado biome (IBGE, 2018). Thus, as projections of higher temperatures due to climate change in the region come to fruition (IPCC, Citation2020), the expected financial gains may become unrealistic for the coming decades.
The impacts of weather extreme events are unequal across countries, with several studies pointing out stronger effects on agriculture in the tropics (Burke et al., Citation2015; Lobell et al., Citation2011 Pires et al., Citation2016;). For example, the El Niño Southern Oscillation (ENSO) is correlated with a shortened rainfall season in the Amazon and Cerrado biomes (Pires et al., Citation2016) that usually results in crop losses (Anderson, Seager, Baethgen, & Cane, Citation2017). To mitigate these negative impacts, farmers in the Cerrado are increasingly relying on irrigation but studies suggest that both surface and groundwater are being overdrawn (Richey et al., Citation2015, La), calling into question the future sustainability of this farming strategy. This study adds to the literature on sustainable food production systems, a research priority worldwide (Gomez-Zavaglia et al., Citation2020).
We adopt a multi-scalar approach ranging from biome-level secondary data analysis to survey data about local farmers’ climate mitigation/adaptation practices in face of weather oscillation. Two questions drive the analytical framework: (1) Have higher temperatures and lower precipitation regimes affected agricultural productivity in the Cerrado? and (2) Are farmers’ perceptions of weather fluctuations and their responses (i.e. agricultural practices to mitigate droughts impact) consistent with the effects of increasing temperature and precipitation changes? The hypotheses are that: (i) higher temperatures and lower precipitation are associated with lower agricultural productivity in the Cerrado and (ii) farmers have an unrealistic perception of adaptation, partly because agricultural technologies and public policies provide short-term financial relief but not a long-term solution.
This paper is organized as follows. After this introduction, we briefly describe the Brazilian Cerrado and the Matopiba subregion, in particular, the focus of our analyses. This is followed by an explanation of the datasets used and the statistical methods employed. We finalize the paper by discussing the results within the broader context of adaptation to climate change and the future sustainability of these capital-intensive systems that increasingly rely on dwindling water resources.
1.1. Study area
The research focused on the Cerrado biome, the second largest biome in South America after Amazonia (). The Cerrado is characterized by pronounced dry and wet seasons. Rainfed agriculture is practiced from September to April, and this rainy period concentrates 80% of the total annual precipitation that ranges from 900 mm at the semi-arid caatinga ecotone to the east to 2000 mm in the Amazonian ecotone to the west (Embrapa, Citation2020). The soils are well developed but fertility is low, and acidity is high with pH ranging from 4.3 to 5.5 (Yamada, Citation2006). Despite the agronomic limitations, since 1960, this biome underwent an intensive occupation process and now accounts for more than half of the grain production in Brazil (Brum, Citation1988; Graesser et al., Citation2015; IBGE, 2018).
Figure 1. Map of the study area and analysis (in three scales. Cerrado, Matopiba, and farms). Source. Elaborated with data from fieldwork, Mapbiomas (Souza et al., Citation2020), IBGE (Citation2010, Citation2017) and Embrapa/GITE (Citation2014).

Two factors explain the modernization and expansion of Brazilian agriculture: the 1960/1970s public policies aimed at developing the country’s hinterlands and the development of technologies for managing soil fertilization in the tropics that allowed soy expansion in the savannas of the Cerrado biome. The main technologies that enabled annual crops in the Cerrado included better-adapted seeds (more recently developed seeds include genetically modified organisms), combined with acidity correction using limestone, and chemical fertilizers such as phosphorus, potassium, and nitrogen (Embrapa, Citation2020). Management techniques were also developed and are now widely adopted by farmers, such as minimum tillage. In order to advance these technologies, the government invested heavily in agricultural research for tropical climate, through the creation of the Brazilian Agricultural Research Company (Embrapa, in Portuguese acronym). As a result, crop productivity increased from ∼1,300 to 3,000 kg/ha since 1980 (IBGE, 2018). The introduction and expansion of soybean cultivation in the Cerrado symbolizes the agricultural revolution of the 1970s and the power of capital in ‘creating natural conditions’ for farming (Brum, Citation1988). The large-scale adoption of these technologies launched Brazil as a major supplier of food in the international market.
With most of the Cerrado closer to São Paulo occupied by the early 1990s, farmland expansion advanced to the transition areas between Cerrado and Amazonia, such as Mato Grosso state and more recently to the Matopiba (an acronym for the states of Maranhão, Tocantins, Piauí, and Bahia) (). The Matopiba region is a transition area between the Cerrado and the Amazon to the west and the Caatinga to the east, encompassing 73 million hectares. Since 2005, the Cerrado vegetation has been highly deforested, mainly for soy production. At the border with the Caatinga semi-arid biome, the main advantage for agriculture is the proximity to ports and the good road network, a locational advantage for an export commodity. Due to a relatively long dry period that extends from 4 to 6 months, more than 180,450 hectares are now irrigated in the Matopiba region, mostly by centre pivot systems that rely both on surface and groundwater (ANA & Embrapa, Citation2019). This dependence on irrigation is often associated with local conflicts and water shortages (Maneta et al., Citation2009; Pousa et al., Citation2019). Because agriculture in this region has periodic droughts related to the ENSO phenomenon, farmers, governments, and related stakeholders must consider adaptations to climate change since models predict higher temperatures and reduced rainfall amounts for the region (Cunha et al., Citation2019; IPCC, Citation2020; Pires et al., Citation2016). Therefore, it is worth assessing whether this continuous expansion over marginal lands will lead to a scenario of increased risk for this capital-intensive type of agriculture.
2. Methods
The research uses a multi-scale approach, comparing Cerrado municipal-level data with farm-level data in the Matopiba subregion. To study how weather variation affects productivity and what the farmers’ responses to weather extreme events are, we combined statistical analyses and interviews with stakeholders into two building blocks: (1) panel data analyses to assess the relationship between soy productivity (kg/ha) and climate variables (temperature and precipitation) from 1980 to 2018, in Cerrado municipalities and (2) data analysis of 90 interviews with farmers in the Matopiba region, on agricultural practices, economic performance, and perception about changes in temperature and precipitation.
2.1. Data
2.1.1. Climate variables and yield by the municipality
The climatic data were obtained through Google Earth Engine (GEE). The platform houses temperature and precipitation data from Copernicus Climate Change Service (Citation2017). The original monthly temperature data are in Kelvin (air temperature at 2 m height) and were converted to Celsius, and the precipitation data are in millimeters (mm) (). For both variables, we calculated averages for the period that includes the harvest season for the main crop studied (soybeans), which occurs from November to May. No difference in the results was found when we included the variables for the whole year that incorporate the off-season period. Therefore, we show results using only the data for the plant growth period, according to other studies (Schlenker & Roberts, Citation2009). Average values per municipality polygon were calculated by standard zonal statistical function in GEE. The municipalities’ polygons, soybean productivity (kilogram/hectare), and area planted values were downloaded from the Brazilian Institute of Geography and Statistics website (IBGE, 2017; 2018). In the Cerrado, the polygons of analysis are composed of 1388 municipalities, of which 322 (23%) are in the Matopiba. The period considered is from 1980 to 2018, resulting in a total of 54,171 pooled observations for the Cerrado and 12,558 (23%) for the Matopiba subregion. The lack of data for some years and particularly for some municipalities early in the time series, forced those observations to be dropped from certain analyses (see the final n in the results). summarizes the data set, source, and procedures for each variable at the municipality-level.
Table 1. Descriptive statistics and source of variables of interest in the Cerrado municipalities, from 1980 to 2018.
2.1.2. Farmer’s survey
A questionnaire was administered to 90 farmers throughout 2017, and the data collected covered a period from 2009 to 2016. We adopted a randomly stratified sampling strategy to have a representative group according to (i) the size of the farms reported in the Rural Environmental Register (CAR in the Portuguese acronym), a georeferenced digital registry of all private properties in Brazil that is available for download (MMA, n.d.); (ii) the farms of different sizes in the four states of Matopiba region; and (iii) the classes of edaphoclimatic conditions (i.e. precipitation and temperature). illustrates the location of the sampled properties. The farms had an average of 7383 hectares, with a total of 657,078 hectares, the planted area ranged from 80 to 31,000 hectares. The semi-structured questionnaire had the following broad questions (see Appendix B for details):
Background information, such as location (latitude and longitude coordinates), size of farm and agricultural area, and date of initial production of the area.
Agricultural practices such as the use of irrigation, crop-pasture integration (for minimum tillage purpose in most cases), and period of planting.
Production (in total kg), area planted (hectares), and crops planted.
Perception about changes in temperature and precipitation, and mitigation strategies, if any.
summarizes the main variables collected for the farm-level panel regressions. The regression analyses do not include the influence of agricultural markets, such as prices of inputs and outputs on farmers’ decisions, because these affect all farmers similarly (despite minor differences in transportation costs) and therefore do not substantially vary across municipalities. Other variables include the agricultural practices reported by farmers, such as double-cropping, changes in harvest calendar, irrigation, and minimum tillage practices.
Table 2. Data summary for variables of interest in the farms surveyed, from 2009 to 2016.
2.2. Statistical analysis
We estimated the long-term effect of temperature (°C) and precipitation (mm) on soybean productivity (kg/ha) using panel data regression at the municipal level within the Cerrado biome, from 1980 to 2018. Using a multi-scalar dataset, we also compared the variables and results with farm-level regressions in the subset region of Matopiba (2009–2016). We used different panel regression model specifications that consider variations across time and between spaces (municipalities or farms). The fixed effects (FE) model controls for potential biases that may arise from omitted time-invariant variables (e.g. soil quality). Meanwhile, the random effects (RE) models allow for time-invariant variables to be explicitly included in the analyses. We also compare the results from FE and RE with pooled OLS.
We followed the literature in adding quadratic terms for temperature and precipitation (Burke et al., Citation2015; Lobell et al., Citation2011; Schlenker & Roberts, Citation2009) to allow for non-linear effects between temperature and precipitation on yield; typically, soybean and other crops worldwide are hampered by extreme conditions of heat and precipitation. The quadratic coefficients allow for the estimation of turning points when temperature and precipitation begin to negatively affect yields. Irrigation was also included as a control variable in some of the specifications, in order to compare its effects on the magnitude of the temperature and precipitation coefficients and to estimate the impact of adaptation. Irrigation data are available only every 5 years and within a shorter timeframe, yielding a much smaller number of observations (see ). In all models, we log transformed the dependent variable. This log-level model estimates the percentage changes in productivity for each unit variation in the regressors.
To avoid spurious correlation, for each municipality, we detrended yield and isolated the linear growth trend resulting from technological innovation through time (Figure A1). If ignored, the trend aspect exaggerates the explanatory power of the regressors and may lead to erroneous conclusions because the trend (positive or negative) of values over time is not entirely caused by the independent variable. Following Wooldridge (Citation2013), we included ‘year’ as a detrending variable in all the models. An alternative detrending method can be applied by using the residuals of yield regressed on t as a dependent variable. We tested and obtained similar results in this second approach (see Appendix A); however, in order to facilitate the presentation, we include only the results of the former detrending method. Therefore, the results can be interpreted as the effect of temperature and precipitation on the deviation from the historical trend in soybean yield.
The regression model for the municipality-level analysis, from 1980 to 2018, is
Where:
= log of soy productivity in each municipality i in year t.α = the intercept.
= the municipality fixed effect, for each i.
T = average temperature for the harvest period (Celsius).
P = annual total precipitation (millimeters).
I = irrigated area (hectares).
year = detrending variable to isolate the linear growth of yield resulting from technology.
s = proportion of clay and sandy soils in municipality i to control for soil quality in the random effects estimation.
are the respective coefficients.T2 and P2 are the quadratic terms for T and P.
are the respective coefficients of the quadratic terms.
e = error term.
The farm-level data were not detrended for two reasons: (i) the analysis was short term (2009–2016), so we assumed that there were no major technological improvements during that time span and (ii) with data at the farm level, we were able to control for agricultural practices reported by farmers.
To help to interpret the results of the municipality-level analysis, we combined the modelling results with the farmers’ perspective on the causes and consequences of productivity loss. Therefore, we contextualized and validated the results with qualitative information, describing farmers’ adaptation strategies and socioeconomic consequences of extreme weather events in the Matopiba. As suggested by the literature (Vayda, Citation1983), this approach allows for the progressive contextualization of the results within socioeconomic processes that frame the local realities.
3. Results
3.1. Productivity and temperature/precipitation variability
3.1.1. Municipal-level analysis
With an upward trend, temperature (T) increased by approximately 1°C in the Cerrado municipalities () for the 1980–2018 period, and the regressions indicate that higher temperatures are associated with lower productivities ( and ). The temperature inflection point, beyond which yield declines, was around 21°C in those models without irrigation (models 1.4–1.6, ). This estimated temperature threshold for soybean is consistent with the literature (Burke et al., Citation2015; Schlenker & Roberts, Citation2009) and indicates that future unsuitable areas for agriculture will be in the northern portion of the Cerrado according to future climate projection. The level-quadratic coefficients for temperature were significant in all models, for all scales of analysis. All models and a joint hypothesis test suggest a non-linear relationship between temperature and productivity for any scale of analysis (municipality and farm level) in the absence of irrigation (, Figure A2).
Figure 2. Boxplot of the average annual temperature and total annual precipitation in the municipal polygons of the Cerrado and Matopiba subset, from 1980 to 2018. Source. Elaborated by authors with ERA5 Copernicus data.

Table 3. Panel model results to Cerrado municipalities from 1980 to 2018.
Table 4. Panel model results to Matopiba municipalities from 1980 to 2018.
Table 5. Panel model results to Matopiba farms from 2009 to 2016.
Average precipitation (P) has a slightly decreasing trend for the 1980–2018 period, and its effects indicate covariance with temperature. In the regressions, FE models show a negative effect of precipitation on yield for the level term whereas OLS and RE show positive effects. All models agree though that precipitation effects are very small, be it positive or negative (see partial effects in the tables below). Precipitation had the same non-linear relationship with productivity (also confirmed by a joint hypothesis test on the level and squared terms; all F(2,1087) statistics above 10) with rainfall inflection point ranging from 1,236 to 1,764 mm (, Figure A2), in accord with other studies (Spangler et al., Citation2017). Nevertheless, only 15% of the observations had precipitation above 1,764 mm yr−1, indicating that high precipitation is not detrimental to yield in the Cerrado in general, as opposed to Amazonia where studies have shown a stronger negative effect of precipitation (Chomitz & Thomas, Citation2001). According to the detrend variable (year), productivity increases by an average of 2% per year in all models.
In terms of partial effects, for the regressions without irrigation, a 1°C increase in temperature reduces productivity by 1–6% in the Cerrado municipalities, meanwhile a 100-mm increase in precipitation is associated with a 0.2% to 1% change in yield (). The Lagrange Multiplier (LM) test indicates the FE as the most consistent model, and also the preferred estimator of partial effects because the method controls for unobserved time-constant characteristics within each municipality that might be associated with soy productivity (e.g. soil type, terrain). This means that we are more confident in models 1.2 and 1.5 to estimate the effect of temperature and precipitation on productivity at the municipal level. The underreporting of irrigated areas and information gaps in the time series may also explain the non-significance of the variables in the models with irrigation and quadratic effect (models 1.10–1.12, and models 2.10–2.12).
For the Matopiba subset, the FE model estimates a higher partial effect of temperature and a significant effect of precipitation on yield across time (). However, the LM test indicates the OLS as the preferred specification, and its partial effects are consistent with the previous results for the Cerrado as a whole. The higher sensitivity of the Matopiba can be explained by the higher average temperature (5°C increase for the 1980–2018 period, in the (A,B)), and proximity to the semi-arid environment of the Caatinga as depicted in . In the eastern part of the Cerrado (including Matopiba), the temperature increased by more than one standard deviation for the entire period of 1980–2018. Continued increase in temperature above one standard deviation ((A)) is a bad omen for farmers in the region and might indicate an uncertain future due to the relationship between temperature and yields. For instance, (B) indicates that most of the productivity loss during 2015 occurred in the municipalities within the Matopiba.
Figure 3. Trend temperature increase divided by the standard deviation from 1980 to 2018 (A); and soy productivity loss/gain from 2015 to 2016 to the Cerrado municipalities during a severe drought (B). Source. Elaborated by the authors with data from IBGE (2010; 2018), Copernicus Climate Change Service (Citation2017).

3.1.2. Farm-level analysis
The regressions for the surveyed farms follow the same patterns observed at the municipality level, with the FE as the preferred estimator. For example, the non-linearity of precipitation was statistically significant but very small, and the negative effect of temperature on yield was substantial in all models (). Also, agricultural practices did not change the negative relationship between increased temperatures and productivity, and the practice of double-cropping was the only significant variable correlated with productivity (). However, the impact of droughts on productivity is uneven and varies according to the farmers’ property size, natural conditions (e.g. soil), and response in terms of agricultural practices adopted, as illustrated by the bimodal distribution shape in . Therefore, we must interpret the RE and FE with caution because the addition of agricultural practices in response to unobserved shocks is more likely and this would violate the strict exogeneity assumption required to obtain consistent estimators (Wooldridge, Citation2013). Such variables are probably correlated with the error term because farmers react to shocks in the past by incorporating new technologies or by changing agricultural practices. Nonetheless, the FE estimator captured the average impact of the 2015/2016 drought on crop failure, or a ∼30% yield reduction for each 1°C increase. Moreover, in normal years the distribution of temperature and productivity is similar between Matopiba municipalities and the surveyed farms ( and ), implying some mesoscale weather patterns.
Figure 4. Climate variables density distribution for properties owned by interviewed farmers in the Matopiba (2009–2016). The extreme drought refers to the 2015/2016 harvest calendar. Source. Elaborated by authors with fieldwork data (productivity), and ERA5 Copernicus (precipitation and temperature).

3.2. Farmer’s perception on climate variables and barriers for sustainable production under climate change
In this section, we use information from our interviews with farmers to describe their perceptions about climate change, weather variability, and agricultural production, and to contextualize the regression results. According to them, year-to-year weather oscillation (meaning temperature and rainfall fluctuations for them) is the main barrier to increasing production (33% of respondents) (), mainly due to its influence in the form of droughts and associated higher temperatures. Also, most farmers recognize that temperature is increasing in the region and that droughts are linked to El Niño occurrences. However, they claim that changes in temperature and rainfall pattern are cyclical fluctuations and not permanent or long-term trend (). Most farmers reported that a shorter rainy season is of greater concern for agriculture. Only 4% of respondents stated that there was no change in the rainfall pattern and 15% did not perceive changes in temperature. Some interviewees mentioned global warming as a culprit for those changes, but they doubt its anthropogenic origin, or that regional vegetation loss is related to any substantial reduction in evapotranspiration and rainfall. Concurrently, 77% of farmers agreed that native vegetation has benefits for the ecological equilibrium, conservation of natural springs, water resources, and humidity. The main adaptation strategies adopted by these landowners to unusual weather are calendar adjustments to delay or anticipate soy harvest (29%), minimum or no-tillage techniques such as crop-pasture integration (23%), rotation of crops (17%), or switch to different crops and varieties of seeds more adapted to harsher conditions (6.5%) ().
Figure 5. Answers to the question ‘what is the main barrier to increase production?’, in percentage. Source. Fieldwork (n = 90, N = 127).

Figure 6. Answers to the question ‘what is the causes of changes in temperature and rainfall patterns?’, in percentage. Source. Fieldwork (n = 90, N = 105).

Figure 7. Answers to the question ‘what is your adaptation strategy to changes in temperature and precipitation?’, in percentage. Source. Fieldwork (n = 90).

Farmers also mentioned political and economic factors that currently hinder sustained gains in production, such as credit access (17%), transportation logistics (15%), technical assistance, and labour capacity (both with 9%), shown in . Most of the properties surveyed were in financial recovery after losses incurred during the extreme drought of 2015/2016 when precipitation was 25% below the 2009–2016 average, and the only planting season with rainfall below 1,000 mm in those farms. Most farmers depend on annual credit to maintain crops (83% have loans) and the harvest losses reduced their collateral for new bank loans. However, according to the interviews, small producers have greater difficulty in accessing credit after extreme weather events due to the lack of financial solvency caused by the loss of production and the high capital requirements of soybean production. The impacts on farmers are uneven and depend on their geographic location and size of operation. For instance, in drier regions (i.e. Piaui and Bahia), smallholders planned to abandon farming altogether or shift to different crops after the 2016 drought and related indebtedness, while large producers saw the drought as an opportunity and expressed interest in expanding activities as conditions improved in 2017. The interviews with indebted rural producers indicate that general production statistics do not capture the current dynamics of land purchase and sale due to drought shocks, which seems to suggest more land concentration.
4. Discussion
The trend of increasing temperatures will continue for the next decades according to climate change models (Brasil, Citation2020; IPCC, Citation2020), extending the drought periods and affecting the demand for irrigated agriculture in the Cerrado (Latrubesse et al., Citation2019; Pires et al., Citation2016). The impact of droughts has increased in recent decades, including wildfires, water deficit, and reduction in crop productivity (Cunha et al., Citation2019). Evidence so far points to increasing edaphoclimatic restrictions in the semi-arid ecotone, where farmers and local communities seem to be more vulnerable to drought shocks (Costa et al., Citation2020), and weather oscillations lead to crop reduction and land abandonment (Cohn et al., Citation2016; Spera et al., Citation2014, Citation2016). Although short-term weather fluctuations and deviations from the normal are expected, a growing number of studies relate those fluctuations as consequences of long-term changes in the climate (Anderson et al., Citation2017; IPCC, Citation2020; Pires et al., Citation2016). For the future, the most conservative downscaled climate model from the National Institute for Space Research of Brazil (INPE, in Portuguese acronym) indicates an increase in the average annual temperature from 26°C to 28°C, for the period of 2016–2046, in the Matopiba region. This forthcoming scenario will make this region more like the caatinga, the semi-arid environment, and will reduce the frequency of rainfall and water availability for plants in the region.
The combined impact of climate change, land use change, and increased demand for water in the form of irrigation create negative feedback on the hydrologic system (Rodrigues et al., Citation2022). A monocrop landscape will reduce evapotranspiration, increase surface temperatures, and ultimately reduce precipitation. Without technological breakthroughs that reduce water dependency, the current system is unsustainable. According to interviewed farmers, adaptations to and consequences of the extreme drought in 2015/2016 included switching to different crops, land concentration, and capital dependency. As suggested by previous studies, climate change adaptation strategies may include changes in the farming system and even land abandonment (Deschênes & Greenstone, Citation2007; Laue & Arima, Citation2016), with social costs and consequences reverberating throughout the commodities supply chain (Burke et al., Citation2015). Our results also indicated a strong effect of weather extreme events in smaller planted areas (e.g. smallholders), and a non-significant effect of short-term adaptation strategies when the reduction in precipitation is substantial, such as the drought in 2015/2016. Although it is not possible to attribute these effects to climate change, the results serve as a reference for the potential impact of higher temperatures on soybean yields under a new climate regime that is expected in the near future.
Farmers’ perception that climate change is not a threat to agriculture is aligned with the common belief that capital and technology will overcome barriers to production (in the form of new seeds and irrigation). As consequence, farmers, governments, and traders continue to induce soybean expansion in the Matopiba, a region with little information on the risks posed by climate change (Costa et al., Citation2020; Garrett et al., Citation2013; Richards & Arima, Citation2018; Rueda et al., Citation2017). Moreover, the technologies developed during the Cerrado conquest (e.g. adapted seeds ans heavy liming) have shown limited historical adaptation potential to extreme heat (Schlenker & Roberts, Citation2009) and create dependency on capital availability in the form of expensive investments in irrigation. Despite the statistically non-significant effect of central pivots in maintaining long-term yield (), this seems to be the main climatic adaptation in the recent history of the Cerrado. From 2000 to 2017, the area irrigated by central pivots in the Cerrado increased from 433,107–1,222,409 hectares (ANA & Embrapa, Citation2019). The combination of rainfall shortage and expansion of irrigated area may affect the practice of double-cropping, which is the most important agricultural practice that explains higher productivity in our model (). Dependence on capital has two other consequences, the increase in the scale of operations and the concentration of land due to the low capacity of small farms to withstand frequent climatic shocks without going bankrupt. Ultimately, without proper technical-scientific assessment and land management policies, regions like the Matopiba may become the Brazilian version of the United States’ Dust Bowl, with prolonged periods of inadequate environmental conditions, inconsistent with initial expectations of financial gain.
Our estimates of crop loss due to increasing temperatures are consistent with the literature, but there is an important caveat about our inability to account for the concentration of CO2 in the atmosphere and its fertilization effects on soybean yields. Some authors claim that the reduction in productivity due to warmer environments can be partly compensated by CO2 fertilization, e.g. Lobell et al. (Citation2011) considers a value of ∼3%. However, the magnitude of the CO2 effect is controversial in the literature. For example, Ainsworth et al. (Citation2008) and Long et al. (Citation2005) claim that labouratory and field studies overestimated the positive effect of CO2 on crop yields. Moreover, the CO2 in the atmosphere can benefit invasive weed species as much as the target crop, affecting productivity and leading to higher production costs in the form of herbicide application (Ainsworth et al., Citation2008). In a methodological note, Schlenker and Roberts (Citation2009) suggested that the effects of CO2 cannot be accounted for in regression analysis because it is impossible to separate CO2 concentration from technological change. In all likelihood, this fertilization effect is controlled for by the inclusion of the year variable in our models, since CO2 concentrations have been growing steadily.
Lastly, global warming is expected to affect crops productivity across the world, mainly in tropical regions due to water stress, with a potential reduction in food supply and impact on food prices (Burke et al., Citation2015; Lobell et al., Citation2011; Pires et al., Citation2016; Zhao et al., Citation2017). Therefore, to achieve sustainable agricultural production we must address climate adaptation/mitigation (Vermeulen et al., Citation2012). The general goal of achieving a stable and secure supply of food while reducing risks of crop losses from climate may be beyond the regional scale, as they require deep changes in the food system itself, including consumption choices (e.g. less beef), international trade, development and transfer of technology, public policies, and capital flows (iPES, Citation2015; Movilla-Pateiro et al., Citation2021). At the local level, reduction of land clearing by agribusinesses is necessary to avoid compromising the microclimate and the loss of soil due to erosion (Coe et al., Citation2011; Leite-Filho et al., Citation2021; Spera et al., Citation2016). Our interviewed farmers indicate that it is easier to encourage practices that overlap climate and market risks, such as no-till practices and crop-livestock integration. However, it is questionable if it is even possible to develop sustainable agriculture systems that rely solely on capital and technology l (Béné et al., Citation2019), while ignoring the socio-environmental consequences of indebtedness, land concentration, and dwindling water resources.
5. Conclusion
The Cerrado is the biome with the largest agricultural production in Latin America, mainly soybeans for export. In recent decades, however, farmlands have expanded over more arid environment without adequate precipitation for rainfed agriculture. Our study estimated that every 1°C increase in temperature was associated with a reduction of 1–6% in soybean yield for the Cerrado biome since 1980, despite technological advances and irrigation growth. During the 2015 drought, productivity was 33% below the average observed in the previous year, with a greater impact in the ecotone between the Cerrado and the semi-arid Caatinga biome (Matopiba region). Moreover, we estimated that average annual temperatures above ∼21°C are harmful to soybean yields. Hence, these results combined with higher temperature projections, indicate the necessity to assess the risks and expectations of gains from farmland expansion in regions vulnerable to droughts.
Although the statistical results show that higher temperatures affect yields negatively, farmers do not perceive climate change as a threat to their livelihoods and they seem more concerned about short-term weather fluctuations. Our findings indicate that higher temperatures represent uncertainties for long-term financial gains in marginal agricultural expansion areas such as the Matopiba. Therefore, private and public actors must better assess the risks of agricultural expansion and take measures to mitigate or adapt land use activities to extreme weather events, particularly higher temperatures and droughts. Moreover, the socioeconomic implications of ENSO events within the context of climate change, although not fully understood, demand better territorial planning of farmland growth and related incentives. For instance, state and federal governments should re-evaluate plans that encourage agricultural expansion in climatic risky areas and create mechanisms to strengthen socio-environmental resilience and food security through local supply chains and crop diversification, with emphasis towards smaller landholders.
Supplemental Material
Download PDF (967.3 KB)Acknowledgement
The authors would like to thank Dr. Ken Young and Dr. Tim Beach for helpful comments on the previous versions of this paper and also the farmers and farm managers who friendly answered the survey.
Disclosure statement
No potential conflict of interest was reported by the authors.
Additional information
Funding
References
- Ainsworth, E. A., Leakey, A. D., Ort, D. R., & Long, S. P. (2008). FACE-ing the facts: Inconsistencies and interdependence among field, chamber and modeling studies of elevated [CO2] impacts on crop yield and food supply. New Phytologist, 179(1), 5–9. https://doi.org/10.1111/j.1469-8137.2008.02500.x
- ANA & Embrapa. (2019). Levantamento da Agricultura Irrigada por Pivôs Centrais no Brasil (1985-2017). Second edition. Available at: <https://www.snirh.gov.br>.
- Anderson, W., Seager, R., Baethgen, W., & Cane, M. (2017, May 28). Crop production variability in north and South America forced by life-cycles of the El nino southern oscillation. Agricultural and Forest Meteorology, 239, 151–165. https://doi.org/10.1016/j.agrformet.2017.03.008
- Béné, C., Oosterveer, P., Lamotte, L., Brouwer, I. D., de Haan, S., Prager, S. D., … Khoury, C. K. (2019). When food systems meet sustainability–current narratives and implications for actions. World Development, 113, 116–130. https://doi.org/10.1016/j.worlddev.2018.08.011
- Brasil. (2020). Projeções climáticas no Brasil. Ministério Da Ciência, Tecnologia e Inovação (MCTI); Instituto Nacional de Pesquisas Espaciais (INPE). Available at: <http://pclima.inpe.br >.
- Brito, S. S. B., Cunha, A. P. M. A., Cunningham, C. C., Alvalá, R. C., Marengo, J. A., & Carvalho, M. A. (2018). Frequency, duration and severity of drought in the semiarid northeast Brazil region. International Journal of Climatology, 38(2), 517–529. https://doi.org/10.1002/joc.5225.
- Brum, A. J. (1988). Modernização da agricultura : trigo e soja / Argemiro J. Brum. FIDENE, UNIJUI.
- Burke, M., Hsiang, S. M., & Miguel, E. (2015). Global non-linear effect of temperature on economic production. Nature, 527(7577), 235–239. https://doi.org/10.1038/nature15725.
- Chomitz, K., & Thomas, T. S. (2001). Geographic Patterns of Land Use and Land Intensity in the Brazilian Amazon. The World Bank Working Papers, No. 46. Washington, D.C.
- Coe, M. T., Latrubesse, E. M., Ferreira, M. E., & Amsler, M. L. (2011). The effects of deforestation and climate variability on the streamflow of the araguaia river, Brazil. Biogeochemistry, 105, 119–131. https://doi.org/10.1007/s10533-011-9582-2.
- Cohn, A. S., VanWey, L. K., Spera, S. A., & Mustard, J. F. (2016). Cropping frequency and area response to climate variability can exceed yield response. Nature Climate Change, 6(6), 601–604. https://doi.org/10.1038/nclimate2934.
- Copernicus Climate Change Service (C3S). (2017). ERA5: Fifth generation of ECMWF atmospheric re-analyses of the global climate. Copernicus Climate Change Service Climate Data Store (CDS), (date of access), https://cds.climate.copernicus.eu/cdsapp#!/home.
- Costa, L. d. A. N. d., Sant’Anna, A. A., & Young, C. E. F. (2020). Barren Lives: Drought shocks and agricultural vulnerability in the Brazilian semi-arid. LACEA Working Paper Series.
- Cunha, A. P. M. A., Zeri, M., Leal, K. D., Costa, L., Cuartas, L. A., Marengo, J. A., Tomasella, J., Vieira, R. M., Barbosa, A. A., Cunningham, C., Cal Garcia, J. V., Broedel, E., Alvalá, R., & Ribeiro-Neto, G. (2019). Extreme drought events over Brazil from 2011 to 2019. Atmosphere, 10(11), 642–662. https://doi.org/10.3390/atmos10110642.
- Deschênes, O., & Greenstone, M. (2007). The economic impacts of climate change: Evidence from agricultural output and random fluctuations in weather. American Economic Review, 97(1), 354–385. https://doi.org/10.1257/aer.97.1.354.
- Embrapa. (2020). Dinâmica agrícola no cerrado: análises e projeções. Édson Luis Bolfe, Edson Eyji Sano, Silvia Kanadani Campos (editors)– Brasília, DF: Embrapa, 2020.
- Embrapa/GITE (2014). Matopiba geoweb: Divisões político-administrativas das Unidades Federativas do Brasil, Microrregiões e Municipios que compõem a região do MATOPIBA. Embrapa’s webpage. Available at: <http://mapas.cnpm.embrapa.br/matopiba2015/>.
- Garrett, R. D., Lambin, E. F., & Naylor, R. L. (2013). Land institutions and supply chain configurations as determinants of soybean planted area and yields in Brazil. Land use Policy, 31, 385–396. https://doi.org/10.1016/j.landusepol.2012.08.002
- Gomez-Zavaglia, A., Mejuto, J. C., & Simal-Gandara, J. (2020). Mitigation of emerging implications of climate change on food production systems. Food Research International, 134, 109256. https://doi.org/10.1016/j.foodres.2020.109256
- Graesser, J., Aide, T. M., Grau, H. R., & Ramankutty, N. (2015). Cropland/pastureland dynamics and the slowdown of deforestation in Latin America. Environmental Research Letters, https://doi.org/10.1088/1748-9326/10/3/034017
- Hengl, T. (2018). Sand and clay content in%(kg/kg) at 6 standard depths (0, 10, 30, 60, 100 and 200 cm) at 250 m resolution (Version v0.2). Data set available at: 105281/zenodo.1476854 and https://doi.org/10.5281/zenodo.1476851.
- IBGE. (2010). Polígonos dos biomas brasileiros. Available at: <http://www.metadados.inde.gov.br/geonetwork/srv/por/metadata.show?id = 75177&currTab = simple >.
- IBGE. (2017). Polígonos dos municípios brasileiros. Available at: <https://www.ibge.gov.br/geociencias/organizacao-do-territorio/15774-malhas.html? = &t = downloads >.
- IBGE. (2018). Produção Agrícola e Pecuária Municipal. Available at: <https://sidra.ibge.gov.br/>.
- International Panel of Experts on Sustainable Food Systems (iPES. (2015). The New science of sustainable food systems: Overcoming barriers to food systems reform. International Panel of Experts on Sustainable Food Systems.
- IPCC. (2020). Climate Change and Land - Summary for policy makers. In Climate Change and Land: an IPCC special report on climate change, desertification, land degradation, sustainable land management, food security, and greenhouse gas fluxes in terrestrial ecosystems.
- Latrubesse, E. M., Arima, E., Ferreira, M. E., Nogueira, S. H., Wittmann, F., Dias, M. S., Dagosta, F. C. P., & Bayer, M. (2019). Fostering water resource governance and conservation in the Brazilian cerrado biome. Conservation Science and Practice, https://doi.org/10.1111/csp2.77
- Laue, J. E., & Arima, E. Y. (2016). Spatially explicit models of land abandonment in the Amazon. Journal of Land Use Science, https://doi.org/10.1080/1747423X.2014.993341
- Leite-Filho, A. T., Soares-Filho, B. S., Davis, J. L., Abrahão, G. M., & Börner, J. (2021). Deforestation reduces rainfall and agricultural revenues in the Brazilian Amazon. Nat. Commun, 12(1), 2591. https://doi.org/10.1038/s41467-021-22840-7
- Lobell, D. B., Schlenker, W., & Costa-Roberts, J. (2011). Climate trends and global crop production since 1980. Science, 333(6042), 616–620. https://doi.org/10.1126/science.1204531. 29 Jul 2011
- Long, S. P., Ainsworth, E. A., Leakey, A. D., & Morgan, P. B. (2005). Global food insecurity. Treatment of major food crops with elevated carbon dioxide or ozone under large-scale fully open-air conditions suggests recent models may have overestimated future yields. Philosophical Transactions of the Royal Society B: Biological Sciences, 360(1463), 2011–2020. https://doi.org/10.1098/rstb.2005.1749
- Maneta, M. P., Torres, M., Wallender, W. W., Vosti, S., Kirby, M., Bassoi, L. H., & Rodrigues, L. N. (2009). Water demand and flows in the São francisco river basin (Brazil) with increased irrigation. Agricultural Water Management, 96(8), https://doi.org/10.1016/j.agwat.2009.03.008
- Ministério do Meio Ambiente (MMA). (n.d). Sistema de Cadastro Ambiental Rural (CAR). Database from Serviço Florestal Brasileiro. Available at: <https://www.car.gov.br/publico/imoveis/index >. Access: Oct 27, 2020.
- Mittermeier, R. A., Turner, W. R., Larsen, F. W., Brooks, T. M., & Gascon, C. (2011). Global biodiversity conservation: The critical role of hotspots. In Biodiversity hotspots (pp. 3–22). Springer.
- Movilla-Pateiro, L., Mahou-Lago, X. M., Doval, M. I., & Simal-Gandara, J. (2021). Toward a sustainable metric and indicators for the goal of sustainability in agricultural and food production. Critical Reviews in Food Science and Nutrition, 61(7), 1108–1129. https://doi.org/10.1080/10408398.2020.1754161
- Pires, G. F., Abrahão, G. M., Brumatti, L. M., Oliveira, L. J. C., Costa, M. H., Liddicoat, S., Kato, E., & Ladle, R. J. (2016). Increased climate risk in Brazilian double cropping agriculture systems: Implications for land use in northern Brazil. Agricultural and Forest Meteorology, 228–229. https://doi.org/10.1016/j.agrformet.2016.07.005
- Pousa, R., Costa, M. H., Pimenta, F. M., Fontes, V. C., & Castro, M. (2019). Climate change and intense irrigation growth in western bahia, Brazil: The urgent need for hydroclimatic monitoring. Water (Switzerland), 11(5), https://doi.org/10.3390/w11050933
- Richards, P., & Arima, E. (2018). Capital surpluses in the farming sector and agricultural expansion in Brazil. Environmental Research Letters, https://doi.org/10.1088/1748-9326/aace8e
- Richey, A. S., Thomas, B. F., Lo, M. H., Reager, J. T., Famiglietti, J. S., Voss, K., & Rodell, M. (2015). Quantifying renewable groundwater stress with GRACE. Water Resources Research, 51(7), 5217–5238. https://doi.org/10.1002/2015WR017349
- Rodrigues, A. A., Macedo, M. N., Silvério, D. V., Maracahipes, L., Coe, M. T., Brando, P. M., & Bustamante, M. M. (2022). Cerrado deforestation threatens regional climate and water availability for agriculture and ecosystems. Global Change Biology, 28(22), 6807–6822.
- Rueda, X., Garrett, R. D., & Lambin, E. F. (2017). Corporate investments in supply chain sustainability: Selecting instruments in the agri-food industry. Journal of Cleaner Production, 142, 2480–2492. https://doi.org/10.1016/j.jclepro.2016.11.026
- Schlenker, W., & Roberts, M. J. (2009). Nonlinear temperature effects indicate severe damages to U.S. Crop yields under climate change. Proceedings of the National Academy of Sciences of the United States of America, 106(37), https://doi.org/10.1073/pnas.0906865106
- Souza, C. M., Z. Shimbo, J., Rosa, M. R., Parente, L. L., A. Alencar, A., Rudorff, B. F. T., Hasenack, H., Matsumoto, M., G. Ferreira, L., Souza-Filho, P. W. M., de Oliveira, S. W., Rocha, W. F., Fonseca, A. V., Marques, C. B., Diniz, C. G., Costa, D., Monteiro, D., Rosa, E. R., Vélez-Martin, E., … Azevedo, T. (2020). Reconstructing three decades of land use and land cover changes in brazilian biomes with landsat archive and earth engine. Remote Sensing, 12(17), 2735. http://doi.org/10.3390/rs12172735
- Spangler, K. R., Lynch, A. H., & Spera, S. A. (2017). Precipitation drivers of cropping frequency in the Brazilian cerrado: Evidence and implications for decision-making. Weather, Climate, and Society, 9(2), 201–213. Retrieved Jun 18, 2021, from https://journals.ametsoc.org/view/journals/wcas/9/2/wcas-d-16-0024_1.xml. https://doi.org/10.1175/WCAS-D-16-0024.1
- Spera, S. a., Cohn, A. S., VanWey, L. K., Mustard, J. F., Rudorff, B. F., Risso, J., & Adami, M. (2014). Recent cropping frequency, expansion, and abandonment in mato grosso, Brazil had selective land characteristics. Environmental Research Letters, 9(6), 64010. https://doi.org/10.1088/1748-9326/9/6/064010
- Spera, S. A., Galford, G. L., Coe, M. T., Macedo, M. N., & Mustard, J. F. (2016). Land-use change affects water recycling in Brazil’s last agricultural frontier. Global Change Biology, https://doi.org/10.1111/gcb.13298
- Vayda, A. P. (1983). Progressive contextualization: Methods for research in human ecology. Human Ecology, 11(3), 265–281. https://doi.org/10.1007/BF00891376
- Vermeulen, S. J., Campbell, B. M., & Ingram, J. S. (2012). Climate change and food systems. Annual Review of Environment and Resources, 37(1), 195–222. https://doi.org/10.1146/annurev-environ-020411-130608
- Wooldridge, J. M. (2013). Introductory econometrics: A modern approach (5th ed.). South-Western, Cengage Learning.
- Yamada, T. (2006). The cerrado of Brazil: A success story of production on acid soils. Soil Science & Plant Nutrition, 51(5), 617–620. https://doi.org/10.1111/j.1747-0765.2005.tb00076.x
- Zhao, C., Liu, B., Piao, S., Wang, X., Lobell, D. B., ... & Asseng, S. (2017). Temperature increase reduces global yields of major crops in four independent estimates. Proceedings of the National Academy of Sciences, 114(35), 9326–9331. https://doi.org/10.1073/pnas.1701762114