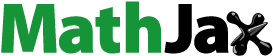
ABSTRACT
Utilizing survey information obtained from five irrigation schemes in southeastern Africa, we investigated the influence of agricultural innovation platforms (AIPs) and monitoring tools on a range of farm and household outcome indicators. Doubly robust estimation was used to measure the effects of these interventions, with a variety of other methods used for robustness checks. Involvement in AIP activities and using monitoring tools was found to be statistically associated with increased on-farm income together with an increased capacity to fund child education. Participation in AIPs also had a significant positive influence on off-farm income and reduced food shortages. Moreover, spillover effects were accounted for in the estimations and statistically significant positive effects were found regarding on-farm income for non-participants. These findings suggest that interventions with strong agricultural innovation system approaches in irrigation schemes in Africa could provide significant societal benefits.
1. Introduction
Internationally, Africa has been rated as having the smallest farming productivity score (Ariga, Mabaya, Waithaka, & Wanzala-Mlobela, Citation2019; World Bank, Citation2021), with an immense discrepancy between actual and potential levels of agricultural production (e.g. Ragasa & Chapoto, Citation2017). Irrigation is one instrument often put forward as a favoured policy mechanism to increase agricultural productivity and reduce poverty in Africa (Manero & Wheeler, Citation2022; Speelman et al., Citation2011).
Many suggest that countries in Africa have extensive opportunities, and need, to increase irrigation (e.g. You et al., Citation2011) given their current water resources (FAO, Citation2016); land availability (The Land Matrix, Citation2021); and increasing population (UNDESA, Citation2017). However, irrigation success has been shown to be exceptionally small in Africa (Kikuchi, Mano, Njagi, Merrey, & Otsuka, Citation2021). Mutiro and Lautze’s (Citation2015) historical in-depth investigation of irrigation schemes within southern Africa illustrated that only around six in ten schemes fulfilled minimal performance yardsticks. Kamwamba-Mtethiwa, Weatherhead, and Knox (Citation2016) found positive impact of irrigation in less than 60% of studies of small-scale pump irrigation. García-Bolaños et al. (Citation2011) examined the status of 22 irrigation schemes in Mauritania and concluded that many of these schemes were defective, with little actual irrigation production. Reasons for this include market problems, concerns about resource ownership, inadequate extension service, static cropping and irrigation arrangements, governance and the absence of local support (Bjornlund, van Rooyen, & Stirzaker, Citation2017; Hounkonnou et al., Citation2012; Röling, Citation2009; Wheeler et al., Citation2017). In addition, legacy issues from colonialism hamper development (Bjornlund, V et al., Citation2020; Jew, Whitfield, Dougill, Mkwambisi, & Steward, Citation2020).
The predominance of the traditional linear school of agricultural extension proposed that technical advances are meant to trickle down and be adopted on mass by farmers. Such an approach has not always been successful in Africa, nor in many other countries (Hounkonnou et al., Citation2012; Pamuk, Bulte, Adekunle, & Diagne, Citation2014; Wheeler et al., Citation2017). Hence, there has been increasing focus on other methods of agricultural extension such as ‘Agricultural Innovation Systems (AIS)’ (World Bank, Citation2006). In general, for an agricultural investment to be meaningful and viable, AIS aims to ensure that the needs of everyone within the sector are met and that they work in cooperation – from the initial concept to the broadcasting of improved methods (Sumberg, Citation2005; van Rooyen et al., Citation2017).
To date, a number of interventions compatible with AIS working guidelines (such as agricultural innovation platformsFootnote1 (AIP)) have been introduced across many African countries to drive farm productivity (Hounkonnou et al., Citation2012; Schut et al., Citation2018; Tahir et al., Citation2020). For example, a cocoa innovation platform was initiated in Ghana to overcome information related and other challenges of farm households (Quarmine et al., Citation2012). Likewise, a goat/livestock innovation platform was formed in Zimbabwe to improve the productivity of goat keepers via remunerative market links (van Rooyen & Tui, Citation2009).
Given that a series of programmes embedded with AIS principles have been operational for over a decade in Africa (Hounkonnou et al., Citation2012; Kaaria, Njuki, Abenakyo, Delve, & Sanginga, Citation2008; Mapila, Kirsten, & Meyer, Citation2012; Quarmine et al., Citation2012), there has been increasing attention on evaluating the success of these programmes (e.g. Pamuk & Van Rijn, Citation2019). The vast majority of African studies have concerned exclusively on the implementation and development of AIP working procedures and guidelines (e.g. van Rooyen et al., Citation2017). Consequently, there has been limited attempts to address the issue of causality of whether AIPs improved outcomes, or is it due to other factors that have not been controlled. The existing literature that has examined the effects of AIPs on farm household outcomes are often statistically inconclusive, or have not implemented robust methods to assess causality questions, although many of them have argued that innovation platforms have resulted in positive effects (e.g. Bjornlund, H et al., Citation2020; Kaaria, Njuki, Abenakyo, Delve, & Sanginga, Citation2008).
Since there remains many unknowns about the relationship between AIPs and associated farm outcomes, this study seeks to investigate a deeper understanding of the casual impact of various irrigation interventions (namely AIPs and soil moisture and nutrients monitoring tools) on various farm and household outcome indicators in southeastern Africa. In this study, soil moisture and nutrients monitoring tools includes ‘Wetting Front Detector’ and ‘Chameleon sensor’ technologies. Hereafter, they are referred to as ‘monitoring tools’. A doubly robust estimation technique is employed to investigate the contribution of these interventions on on-farm income, off-farm income, the ability to pay for child education and household food shortages in five irrigation schemes across three southeastern African countries. In addition, likely spillover impacts of AIPs and monitoring tools on non-participants and non-users are also investigated.
2. Agricultural innovation systems literature
The limitations of the technology supply push approach (Biggs, Citation2007; Hounkonnou et al., Citation2012; Röling, Citation2009; Wheeler et al., Citation2017) and the negligible adoption of agricultural technologies in Africa led to the need to find practical, local solutions, while considering local resources, customs and services. Hence, from around the last decade of the second Millennia, there has been increasing focus on AIS models (World Bank, Citation2006). The AIS model recognises that enhanced technologies and knowledge are the result of coordinated and iterative work by various agricultural entities. Overall, AIS strives to accommodate every component/sector within the agriculture jurisdiction (Hounkonnou et al., Citation2012; World Bank, Citation2006).
Under AIS guidelines, AIPs are crucial – to help ensure practical changes on the ground (Sanyang et al., Citation2016; Schut et al., Citation2018; Schut et al., Citation2019). Typically, an AIP involves an association of entitiesFootnote2, with expertise and resources, who work together to reduce agricultural barriers. Within an AIP, local farmers can take part in discussions, and raise concerns to influence decision-making. Practices and exercises of AIPs are routinely adjusted, using voluntary and flexible processes to reflect the general consensus. Facilitators play a vital role in the success of AIPs, their role is to progress negotiations between a diverse array of parties and harness resources (van Rooyen et al., Citation2017). Building trust among key agents, representation and embracing diversity are critical parts of the effectiveness and practicality of AIPs (Schut et al., Citation2018; van Rooyen et al., Citation2017). For instance, the meta-analysis of Schut et al. (Citation2018) depicted that AIPs that engaged all stakeholders and acted in accordance with their needs were shown to be more effective.
The literature evaluating AIPs is growing steadily, and the methods to assess impacts are also becoming more sophisticated, moving from descriptive statistics to treatment effect methods. However, a primary focus has been on the impact of the intervention on participating farmers only (Ahimbisibwe et al., Citation2020; Mapila, Kirsten, & Meyer, Citation2012; Siziba et al., Citation2013), with little attention paid to potential spillover impacts. Bisseleua et al. (Citation2018) examined the influences of multi-stakeholder processes on a range of household outcomes and showed evidence that taking part in these programmes had positively enhanced the efficiency of farm production and capital availability utilizing descriptive analysis and Tobit and stochastic frontier models. In the same way, Chilundo et al. (Citation2020), Mdemu et al. (Citation2020) and Parry et al. (Citation2020) used descriptive statistics and qualitative data to examine the potential benefits of AIPs and monitoring tools and found evidence that these initiatives resulted in a positive impact on productivity, income and water use. In a recent study, using descriptive statistics, Osorio-García et al. (Citation2020) presented the positive influences of AIPs on farming practices, stakeholders' interaction and climate change knowledge in Colombia.
On the other hand, in Malawi, Mapila, Kirsten, and Meyer (Citation2012) examined the casual links between enabling rural innovations and a number of key welfare indicators. They employed propensity score matching (PSM) and revealed that innovation programmes enabled cultivating households to attain more income, production and better farming methods. Ogunniyi et al. (Citation2017) also used PSM techniques to analyse the role of a growth enhancement support scheme in Nigeria and depicted that the intervention had a statistically significant positive income and production impact on intervention households. However, despite the fact that PSM is one of the classic intervention assessment toolkits used traditionally (Rosenbaum & Rubin, Citation1983), it is an imperfect method to manage biases connected with unknown influencing variables and specification errors (Imbens & Wooldridge, Citation2009).
Another investigation by Siziba et al. (Citation2013) concluded that innovation platforms had positively and significantly boosted agricultural production in three African countries, using instrumental variable technique. In Uganda, Ahimbisibwe et al. (Citation2020) used the endogenous switching regression method and illustrated that AIPs enhanced the consumption level of farmers. Pamuk, Bulte, Adekunle, and Diagne (Citation2014) studied the roles of AIPs, initiated in a number of African countries, using a rigorous panel data assessment technique. While they found that AIP interventions had positive payoffs in terms of the use of some agricultural practices (e.g. crop management), AIP had no observed statistically significant benefit for other practices (e.g. post-harvest management). By disaggregating the data into sub-samples, the same study also demonstrated that AIPs could have inconclusive results. Pamuk, Bulte, Adekunle, and Diagne (Citation2015) continued this evaluation through measuring the poverty curtailing contribution of AIPs but found inconclusive results. In general, the above intervention assessment toolkits are limited in capturing and analyzing the potential spillover effects from implemented AIP interventions on non-recipient farm households. Bearing in mind this gap, this study seeks to pay greater attention to understanding both causal and spillover impacts from the implementation of AIPs and monitoring tools on farm household outcomes in five irrigation schemes in southeastern Africa.
3. Data and research methodology
3.1. Survey overview and area
Farm-level information obtained from a face-to-face survey in 2017 from an Australian Centre for International Agricultural Research (ACIAR) sponsored project, dedicated to enhance irrigation outcomes in five schemes from three southeastern African countries: Mozambique, Tanzania and Zimbabwe, was employed for this investigation. The project commenced in 2013 and made two interventions within the targeted schemes: AIPs and monitoring tools. The underlying consideration was that barriers to the performance of irrigation schemes in Africa are multi-faceted, implying that efforts concentrating solely on one aspect to resolve them would be unlikely to succeed (Bjornlund, H et al., Citation2020). The research team obtained ethics approval by the Human Research Ethics Committee at the Australian National University (Human Ethics Protocol 2017/263). All households had provided informed consent before participation.
The monitoring tools include the ‘Wetting Front Detector’, which tracks the nitrate content of soil water at different depth of the root zone The other tool was the ‘Chameleon sensor’ device, which was essentially designed to allow smallholders to track the level of soil moisture at various depths. The innovation was designed to rate soil moisture in a clear and easy way: a red, green and blue light represent dry, moist and wet soil respectively – rather than building in too much complexity. Combined the two tools supported farmers to understand the extent of soil moisture and nutrients available in the soil for enhanced irrigation scheduling decision making. Subsequently, farmers are able to easily gauge soil moisture and take appropriate action – making better use of factors of production, in particular water and fertilizer (Stirzaker et al., Citation2017).
3.1.1. AIP details
AIPs were deployed in each scheme from 2013 onwards to identify productivity barriers and seek practical solutions. AIPs comprised of all stakeholders involved with the schemes: farmers and their organizations; government departments; civic leaders; development agencies; transport; input suppliers; output buyers and advisory services (van Rooyen et al., Citation2017). Members were nominated on the basis of: (1) experience in identifying productivity barriers; (2) expertise to put forward relevant ideas to overcome impediments; (3) willingness and expertise to play a part in overcoming the barriers; and (4) ability to distribute ideas, information and experiences over the course of time (Bjornlund, H et al., Citation2020).
The strategies adopted by the project regarding AIP participants varied across the three countries, according to each study area's existing situation. The scheme in Mozambique had a small number of farmers; so that all farmers attended meetings and became involved in AIP deliberations (). The schemes in Tanzania and Zimbabwe had more farming households hence only a small fraction of farmers participated in meetings while all farmers were invited to attend and benefit from the AIP initiatives/interventions. AIP initiatives/activities included workshops, focus group discussions, field visits, demonstration programmes, study and market visits, among many others (Bjornlund, H et al., Citation2020). In Tanzania, irrigation scheme leaders invited farmers to take part in meetings based on their location within the schemes, gender and age. These farmers were expected to represent the scheme and communicate meeting outcomes to others. In Zimbabwe, farmers were invited to nominate their AIP representatives.
Table 1. Intervention participation across five southeastern African irrigation schemes in 2017.
Different approaches were exercised to facilitate the AIP meetings. In Zimbabwe, project staff provided facilitation. In Tanzania, an independent facilitator was first engaged; however, given failings of this approach, the role was consequently taken over by project staff. In Mozambique, experts from National Institute for Irrigation guided the facilitation. Facilitators were required to record and track all processes that took place under the AIP. An AIP expert from the Zimbabwe team guided the implementation in all three countries.
3.1.2. Monitoring tools details
Working in conjunction with AIPs, the ‘Wetting Front Detector’ and ‘Chameleon sensor’ devices were granted at no charge to a group of irrigators (Stirzaker et al., Citation2017). The tools were applied in four schemes (they were not applicable in Magozi as the prime crop was rice). Irrigators obtained tools based on: (1) location within the scheme; (2) perceived capability to operate tools; (3) ability to communicate the ideas, learnings and new practices from using the tools to others; and (4) reputation in the community (Bjornlund H et al., Citation2020). Subject to meeting these conditions, the tools were granted to around 20 irrigatorsFootnote3 in each of the four schemes. Consequently, each farmer was given two Wetting Front Detectors and sensor arrays and two Chameleon sensor readers were also given to each scheme for joint use (Stirzaker et al., Citation2017).
3.1.3. Survey data
The 2017 farm household survey was conducted three years after the project started. It was conducted as face-to-face interviews with irrigation household’ heads or another household member actively involved in household decision-making. Taking into consideration each study site conditions, different sampling methods were followed. Irrigator participants from Kiwere and Magozi schemes were selected using stratified sampling techniques, whilst purposive methods were applied in the Silalatshani scheme. Because of the overall small size of total irrigation households in Mkoba and 25 de Setembro schemes, efforts were made to survey all irrigators, although this was not always possible.
In total, 366 irrigation farm households participated in the 2017 survey. However, due to missing observations for some variables, only 361 responses were utilized in this study to examine the influences of AIPs (). In addition, given that monitoring tools were not offered to irrigation households within the Magozi scheme, they could not be included in the monitoring tools analysis (hence only n = 241 was available for the four schemes).
The survey gathered detailed information on a vast range of themes including farm household profiles, consumption, information access and resource use (including time and money). Changes in irrigation practices, revenues from various activities, food security, education, marketing conditions, irrigated and dryland areas, livestock holdings, and types of crops grown were also captured. The survey also gathered information with respect to AIPs and monitoring tools. Note that the 2017 farm household survey data did not allow separate econometric analysis of those directly involved in the AIP meeting. However, information on AIP initiated activities participation were gathered and hence, AIP participation in this analysis is comprised of farmers who participated in an AIP activity or event (hereinafter named ‘AIP events’). In sum, there were 270 participants, and 91 non-participants.
3.2. Dependent and independent variables
This study used objective as well as perception-based dependent variables to investigate the influences of AIPs and monitoring tools ( provides summary statistics). The household outcomes analysed included: on-farm income; food insecurity; capacity to pay for child education; and off-farm income.
Table 2. Summary statistics across five southeastern irrigation schemes in 2017.
The on-farm income variable was constructed by adding income from three different activities (irrigated crop sales, rain-fed crops sales and livestock sales) over the past year. Information was also used on the total production from different crops, the percentage of production sold for each crop, their respective market prices, rain-fed (i.e. dryland) crop income and the type and quantity of livestock owned, consumed and sold. All income figures in the survey were gathered in local currencies for Tanzania and Mozambique, whereas, in Zimbabwe, USD was the local currency at the time. We converted the on-farm income of Mozambique and Tanzania into USD using the respective country official exchange rates.
Food insecurity was computed as the number of months a given household said they experienced food shortage over the last one-year period before the survey. Furthermore, we analysed two other variables: child education and off-farm income to quantify the impacts of project interventions. In the survey, irrigators were asked to describe how their ability to fund child education and their off-farm earnings have changed since project inception in 2013. Farmers were asked to describe their answers in Likert scales where: ‘1 = much worse’; ‘2 = worse’; ‘3 = same’; ‘4 = better’ and ‘5 = much better’, which were converted into dummy variables. An irrigator's capacity to pay for child education/off-farm income, were assumed to be improved if the irrigator stated that his/her capacity/income got better or much better, and not improved if it remained the same, worse or much worse compared to four years ago.
Using insights from past literature (e.g. Abebe et al., Citation2020; Abebe et al., Citation2022; Mapila, Kirsten, & Meyer, Citation2012; Ogunniyi et al., Citation2017; Wheeler et al., Citation2017) independent variables in our model included: age, gender, family size, education, health status, cultivated land area, livestock holdings, media access, information access, affiliations to farmer groups or any community based organizations, scheme/country dummies and plot locations from the head of the irrigation canal ( provides summary statistics and definitions).
Except for scheme/country dummies, identical covariates were incorporated to investigate the roles of AIPs and monitoring tools interventions on the various dependent variables. Given that the independent variables within an investigation should not be influenced by the investigated intervention (Wooldridge, Citation2005), agricultural inputs (fertilizer, non-family labour employment, seed, water spending) were not included in the on-farm income regressions as inputs were presumed to be influenced through AIP events and monitoring tools. Furthermore, on-farm income would also influence child education and food shortage, and therefore on-farm income was not incorporated as a covariate in the capacity to pay for child education and food shortage regressions.
3.3. Descriptive statistics
The variable definition and summary statistics are illustrated in. shows that 75% and 39% of farmers participated in AIP events and received monitoring tools, respectively. displays the comparison between irrigators who participated in AIP events (and received tools) compared with those who did not. AIP participants had more household heads with good health, accessing more media and information, higher monitoring tool use and higher membership of farmer group/community-based organizations. For monitoring tools, users had a higher percentage of household heads with good health, and larger household sizes and higher membership of farmer groups/community-based organizations, compared to those who did not.
Table 3. Difference in independent variables by intervention across five southeastern African irrigation schemes in 2017.
Similarly, the differences among dependent variables, by irrigator participation, are reported in . For AIP events, a significant mean variation in on-farm income and food insecurity outcomes was revealed. For instance, on average, households involved in AIP events obtained USD441 more on-farm revenue and faced almost one month less food shortages during a year than irrigators who did not take part. In addition, irrigators using tools obtained – on average – USD621 more income than those who did not, along with an increased capacity to pay for child education.
Table 4. Differences in dependent variables by intervention across five southeastern African irrigation schemes in 2017.
As a whole, these descriptive findings signalled that interventions may have brought benefits to participating irrigators. However, one potential explanation for these results may be directly associated with the selection bias caused by the project intervention design. During the project execution, those irrigators who were offered monitoring tools and to take part in AIP events were selected in accordance with a set of pre-determined criteria. In view of this, irrigators with greater social, cultural or economic positions may have been identified to take part in these interventions, leading to potential selection bias. The next section describes the econometric techniques applied to combat selection bias connected with the observed heterogeneity between project intervention participants and non-participants and the intervention outcomes.
3.4. Econometric methodologies
An overarching aim of impact evaluation of agricultural technologies is to establish the counterfactual of the technology/skill participation. Randomized experimental methods endeavour to overcome the counterfactual matter by equally inviting every single entities to take part in an intervention, minimizing the incidence of selection bias (Imbens & Wooldridge, Citation2009). However, the use of randomized experimental method was not suitable in the present study given the fact that irrigators participating in AIP meetings and those receiving monitoring tools were purposefully selected based on a set of criteria. Moreover, some irrigators decided to take part in the AIP initiated events, while others did not, which could also lead to selection bias. In a non-randomized controlled setting, the desire to sign up into an intervention may be influenced by observed and/or unobserved factors. In this respect, any descriptive analysis comparing the outcomes of the technologies might be compromised, given it may be liable to selection bias. A range of approaches are suggested in Imbens and Wooldridge (Citation2009) to overcome this, with the choice of a desirable method broadly determined by data access and the type of the intervention. In conditions such as this study, the treatment-effect estimators using observational data methods was considered the best available option to evaluate the causal effects of AIP events and monitoring tools use.
3.4.1. Doubly robust estimators
We analysed the influence of AIP eventsFootnote4 and monitoring tools use on various irrigation farm and household outcomes by applying inverse probability weighted regression adjustment (IPWRA) (Imbens & Wooldridge, Citation2009; Wooldridge, Citation2007) and augmented inverse probability weighted (AIPW) (Bang & Robins, Citation2005) methods. These two methods were implemented by integrating the components of regression-based and propensity score-based (such as inverse probability weighting (IPW)) techniques, making them less vulnerable than regression based-methods and IPW to model misspecification issues (Bang & Robins, Citation2005; Imbens & Wooldridge, Citation2009). Hence, they are customarily named doubly robust estimators.
The use of IPWRA and AIPW relies on three main assumptions. The first is the stable unit treatment value assumption (SUTVA) (Rubin, Citation1980), which posits that the outcomes of those irrigators who did not receive monitoring tools were not influenced by monitoring tools presence or use, implying the absence of spillover effects from users to non-users on the outcome variables. The conditional independence assumption is the second binding requirement (Rosenbaum & Rubin, Citation1983), which assumes that once the observed heterogeneity between users’ and non-users’ are adjusted, potential irrigation outcomes are unrelated to the intervention. The third prerequisite is the common support assumption, where every irrigator suitable for a proposed intervention with comparable profiles has comparable odds of participating in either treatment. The second and third assumptions are named together as strong ignobility (Rosenbaum & Rubin, Citation1983).
For an intervention, it is purported that an individual irrigator
would have two potential outcomes (intervention and non-intervention) that are conceivable but mutually exclusive, as the researchers could observe only one or the other, but not both outcomes. Noting the distinct aspects,
, which are unique to every irrigator, the mutually exclusive irrigation outcome equations are given as:
(1)
(1)
(2)
(2) where
and
are the outcomes (i.e. on-farm income, child education, food insecurity and off-farm income) of the same irrigator
if assigned and not assigned in an intervention, respectively and
designates a vector of individual irrigator demographics and farm profiles that are anticipated to influence outcomes.
and
are intercepts;
and
are coefficients of
; and
and
are error terms.
The project intervention (AIP and monitoring tools) assignment model (Wollni & Zeller, Citation2007) was quantified as:
(3)
(3) where
was the irrigator’s probability of being assigned or not assigned into AIPs and monitoring tools;
was individual irrigator features that are anticipated to influence their placement in the interventions;
is a vector of coefficients; and
the error term.
Considering the above assumptions, the effects of AIPs or monitoring tools are therefore:
(4)
(4) where ATE signifies the average treatment effect;
the mean outcomes of those irrigators assigned in the interventions and
the mean outcomes if not assigned in the interventions.
Given the difficulty in obtaining outcomes for a single irrigator under both intervention and non-intervention at any one time (Holland, Citation1986); doubly robust estimators were used to overcome this issue (Imbens & Wooldridge, Citation2009). Firstl, the propensity scores of being allocated in these interventions were quantified utilizing the intervention assignment model (EquationEquation 3
(3)
(3) ). We estimated the propensity scores of participating in AIP events or monitoring tools through binary probit regression and incorporating a diverse array of independent variables in the model. Estimated results from this procedure are provided in . Then, each irrigator was weighted by the inverse of their unique propensity scores in order to generate an imaginary population such that intervention assignment is unrelated with household outcomes. Second, using the weights generated, the effects of AIPs and monitoring tool was estimated by the outcome models (Equations 1, 2 and 4). While some intervention evaluations focus on the average treatment effect on the treated (ATT) because the specific interventions are more relevant to the treated population, e.g. participants of a job training programme, the AIP and monitoring tools interventions in our study are relevant for the whole irrigation households, and therefore ATE for the whole population is of more policy use.
As a robustness check, we implemented regression-based (such as regression adjustment (RA)); propensity score matching (PSM) (Rosenbaum & Rubin, Citation1983); and inverse probability weighting (IPW) (Imbens & Wooldridge, Citation2009) techniques to evaluate the causal effects of interventions. RA was designed to gauge the influences of an intervention using an outcome model alone, whilst PSM and IPW estimated the effects of these interventions employing the intervention assignment model. In principle, these techniques are presumed to offer comparable findings and arrive at similar conclusions when the underlining models are desirably specified.
3.4.2. Spillover effects identification
It was also important to assess whether any likely spillovers exist from AIPs or tools. The assessed project was designed and applied based on the ethos that granting tools to a small number of farmers in each individual study region prompts both self and inter-farmer learnings in the respective schemes. In addition, given that AIPs were formed at the scheme level and that most of the irrigation constraints, such as infrastructure, market and transport were common to the general irrigation scheme community, any solutions devised with the support of AIP interventions may bring extensive public community benefits. For example, AIP non-participants may take advantage of better market links created with the help of AIPs to sell their production and access productive inputs, which could enable them to secure higher farm income and decrease production costs (see Bjornlund, H et al., Citation2020; Parry et al., Citation2020).
Furthermore, regarding tools, it was entirely possible that non-users adjusted their watering behaviour through learning from users, through either proximity or social links, creating positive spillovers. By design, AIP events were also expected to serve as a supplementary conduit to encourage learning as well as sharing of experiences within farming communities. As a result, monitoring tools use may not only influence the response of participants, but potentially that of non-users. Given this – the stable unit treatment value assumptions commented on previously may not be valid. Following Cerulli (Citation2017), we captured spillovers through redefining Equations (1) and (2):
(5)
(5)
(6)
(6) where
designates the spillover benefit received by irrigator
(AIP non-participant or monitoring tools non-user), which could emerge as a result of irrigator's
intervention participation.
is the sensitivity coefficient; and
is a matrix denoting the distance between intervention participants and non-participants. After some simplification, this becomes:
(7)
(7) where
and
.
As the exact geographic location of each irrigator was unknown, a matrix was composed from individual irrigator profiles to measure the extent of proximity/similarity between participators (users) and non-participators (non-users). Following Cerulli (Citation2017), this is labelled as a ‘correlation matrix’. Dryland farming experience in years and education was employed to calculate the similarity of AIP participants and non-participants. On the other hand, dryland farming experience, installed tools locationFootnote5 (with values ‘1 = on different canal’; ‘2 = on the same canal’; ‘3 = three plots away’; ‘4 = two plots away’; ‘5 = in the neighbour's plot’ and ‘6 = own tools’), and irrigation communities willingness to pay for monitoring tools were used to assess the similarity of monitoring tools users and non-users. Finally, the impacts of AIPs or monitoring tools, given likely spillovers, were predicted through the Stata command ‘ntreatreg’ as per Cerulli (Citation2017).
Collinearity checks confirmed no strong relationships among independent variables. Outlier issues were managed using the Winsorizing method: we changed the upper as well as lower-end 1% of those outlier observations with 99 and 1 percentile values respectively to alleviate any potential bias. Robust standard errors were also used to eliminate potential heteroscedasticity concerns.
4. Regression results
The econometric results are reported in and . We first estimated the drivers associated with individual irrigators’ involvement into interventions using probit regressions (). The sign of statistically significant variables was in line with expectations. In , the impact of interventions on dependent variables estimated via several estimators are reported, and reported the treatment effects after accounting for potential spillovers. and Figures A1–A3 provide various diagnostic analysis of the treatment-effect estimators. All these results together revealed that the distribution was analogous for participant and non-participant irrigators across both interventions, hence increasing confidence in the robustness of the overall results.
Table 5. Impacts of AIP and monitoring tools across five southeastern African irrigation schemes in 2017: average treatment effects (ATE).
Table 6. Spillovers from AIPs and monitoring tools across five southeastern African irrigation schemes in 2017.
4.1. Treatment effect results
showed that AIP participation statistically significantly increased irrigation on-farm income – an additional on-farm income of USD370-375 under IPWRA and AIPW (p ≤ 0.01). In addition, there was a positive impact of AIP events on irrigators’ ability to fund child education – an 11 percentage points greater ability (p ≤ 0.05 in IPWRA and p ≤ 0.1 in AIPW estimators). Participating in AIP events also had a statistically significant effect on food insecurity (p ≤ 0.01 under IPWRA and p ≤ 0.05 in AIPW) and off-farm income (p ≤ 0.05 and p ≤ 0.1 under IPWRA and AIPW estimators, respectively). To be more specific, irrigators’ taking part in AIP events experienced 1.1 fewer months of food shortage annually and had an 11-percentage-point higher probability in earning more off-farm income.
Similarly, monitoring tools use positively and statistically significantly increased irrigation on-farm income by USD419 and 430, under the AIPW and IPWRA estimators, respectively (p ≤ 0.05). Furthermore, a strong and significant positive child education influence was also observed from the uses of monitoring tools – boosted chances of irrigators’ improved ability to pay for child education by about 24 percentage points (p ≤ 0.01).
As shown in , RA, PSM and IPW findings reasonably compared to the doubly robust techniques for both interventions. Additional sensitivity analysis using traditional methods (OLS, binary Probit and Poisson models) provided comparable evidence with IPWRA and AIPW findings. Finally, it seems there is strong statistically significant and stable evidence that AIP and monitoring tools interventions improved various household outcomes for southeastern Africa irrigation communities.
4.2. The spillover estimation results
reports the influences of project interventions on several outcome variables when likely spillovers were accounted for. The findings in were built on the SUTVA assumption, reflecting that the outcomes of non-participants were assumed not to be influenced by interventions. However, this assumption was shown to be violated for the on-farm income in the AIP intervention model (), since a positive significant (p ≤ 0.05 for F-test) spillover effect was evident – although its magnitude was small (around 3%) (p ≤ 0.05). The influences of AIP intervention on other outcomes were not statistically significantly varied between the models accounting for spillovers or not, as evident by the insignificant F-test.
also reveals a significant spillover influence for on-farm income in the monitoring tools model, while no significant spillover effects was found for other outcomes. When spillovers are accounted for, the impact of monitoring tools on on-farm income increased by around 20% (p ≤ 0.01), in contrast to the impact estimated in the IPWRA model. In other words, these technologies increased not only the on-farm incomeFootnote6 of irrigators who used them but also the on-farm income of nearby non-users of monitoring tools. This result supported the initial project hypothesis that monitoring tools should encourage learning at individual, farm and societal levels and ultimately help to generate positive spillovers to non-participants.
5. Discussion
This study employed a range of econometric methods to model how participation in AIP events and using monitoring tools in five irrigation schemes across southeastern Africa fostered irrigation household outcomes. AIP event participation increased both on-farm and off-farm income, ability to fund child education and reduced food shortage. This supports other qualitative findings by Bjornlund, H et al. (Citation2020); Chilundo et al. (Citation2020); Mdemu et al. (Citation2020) and Parry et al. (Citation2020) whom explored the mechanisms by which AIPs were successful in identifying irrigation impediments and developing contextual and practical remedies to such problems. It has been suggested that AIP processes helped transform subsistence schemes to business-oriented systems. In Zimbabwe, for example, the AIPs listed all problems that negatively hampered irrigation and sought to help resolve all these issues through the participation and interaction of key stakeholders (Parry et al., Citation2020). Through these activities, irrigators were encouraged to expand their production, invested in productivity enhancing activities and thus, obtained greater output, income as well as invested in welfare improving activities.
Similarly, in Tanzania, through the AIP initiated activities and discussions, interrelated productivity and profitability barriers (such as knowledge gaps, inferior input quality and undersupply of inputs, market problems and quarrels over plot borders) were addressed. A range of AIP initiated events, such as focus group discussions, workshops, field visits and demonstration programmes were organized and held with the purposes of prompting learning, discussion, experience sharing and information flows. Training programmes on gross margin analysis and farming were prepared by the AIPs, which supported farmers to cultivate varieties with high economic returns (Mdemu et al., Citation2020). Consequently, farmers were more easily linked to input and output markets and thus, able to sell their produce with better prices, which increased income and accessed quality inputs with reasonable prices. Farmers also altered their farming practice from producing staple crops to the production of more profitable crops and engaged in scheme maintenance of their own free will. In Mozambique, the AIP events positively contributed to tackle the most pressing irrigation challenges (see Chilundo et al., Citation2020 for the detail) and as a result, better household outcomes were gained. Broadly, the AIP results presented here corresponds with the findings from Schut et al. (Citation2018) meta-analysis, which underlined that for an AIP to be functional and have meaningful benefit, it should be representative and suited to the interests and contexts of those actors operating in the sector – an approach the project followed from inception (see van Rooyen et al., Citation2017).
The second intervention, monitoring tools, has also shown a strong influence on irrigators’ on-farm income and their ability to pay for child education. The likely justification for this is that the tools enabled farmers to better utilize resources, including water, fertilizer and family labour, to maximize production and consequently provided an opportunity for cash revenue. Money was also saved on reduced inputs, as the tools allow them to use a more proficient combination of resources. The income gain could then be used for other welfare enhancing activities, such as child education. Research studies by Abdulai and Huffman (Citation2014) reported similar findings in Ghana regarding the use of conservation innovations on farm net revenue.
In addition, participation in AIP events seemed to have an estimated 3% spillover effect on non-users on-farm income – meaning that the interventions generated external benefits for the scheme as a whole. Monitoring tools also generated about 20% on-farm income spillover for non-participants. Indeed, it was a specific purpose of the project to inspire learning and experience sharing (Mdemu et al., Citation2020; Parry et al., Citation2020). The pathways on how monitoring tools use altered the ‘mindsets’ of farmers and created ‘incentives for change’ were also presented by Chilundo et al. (Citation2020) and Parry et al. (Citation2020). These studies claimed that monitoring tools reduced labour needs, input use, disputes over water use, and frequency and duration of irrigation events. Accordingly, irrigators raised their production and decreased costs.
Overall, the positive and statistically significant AIP intervention results may be explained by increased: (1) networking, market and information flows; (2) opportunities that arise from networking; (3) dispute resolution and scheme participation; and (4) additional capital, on-farm income and reduced labour spent in irrigating allows for further investment and business/social opportunity (Mdemu et al., Citation2020; Parry et al., Citation2020). This follows other research such as Ahimbisibwe et al. (Citation2020), Ogunniyi et al. (Citation2017) and Siziba et al. (Citation2013) whom outlined that AIPs led to positive consumption, income, and production effects in Uganda, Nigeria and southern Africa respectively.
It is imperative to note the limitations of our study. One particular concern is that, due to the limited number of observations, we were confined to aggregate information gathered from three countries and could not conduct individual scheme/country analyses. Consequently, a greater volume of observations from each study region, with a broader range of information, would help confirm the stability of the reported results from a country/regional perspective. Another limitation of the low number of observations is that it was not possible to identify the combined impacts of both AIPs and monitoring tools on irrigation household outcomes. In addition, exploring the impact pathways of AIPs and monitoring tools on the studied outcomes quantitatively is out of scope of our study. For example, the on-farm income impact may be due to reduced input resulting from tool use. Future research may use econometric models to test the various impact pathways and generate more policy relevant findings on how to realize more positive benefits from these interventions. As a final concern, the results are very reliant on the assumption that participation in the interventions were guided only with observed factors, meaning that there may be unobserved influences affecting involvement in the project and thus may nullify the estimated impacts. Addressing these issues could help improve future research in this field.
Conclusion
This paper examined the influence of an irrigation development project on irrigation farm household livelihood measures in five irrigation schemes across three southeastern African countries. Project applications included the development of AIPs and the distribution of monitoring tools, with treatment effect regression applied to evaluate household outcomes. Our results suggest that involvement in AIP events – or the use of monitoring tools – enhanced both on-farm income and the ability to fund child education. In addition, participating in AIP events reduced the number of months a household experienced food shortage and increased off-farm income. There was also a learning effect of AIP events on non-AIP participants and monitoring tool use on non-users.
These results emphasize the importance of assimilating both scientific knowledge and local expertise. Wider impact could be achieved through greater scale and allowing every entity – including poorer households in the agriculture jurisdiction – to be actively involved. In realizing such an outcome, a regional level of experience, ideas and institutions will be needed.
Acknowledgements
The Australian Centre for International Agricultural Research financed this research through the Transforming Irrigation in Southern Africa (TISA) projects FSC/2013/006 and LWR/2016/137. We also thank all TISA project team members for collecting the survey data and other contributions, Adam Wheeler for editing assistance and small-scale farmers who participated in the survey.
Disclosure statement
No potential conflict of interest was reported by the author(s).
Data availability statement
The data that support the findings of this study are available on request from the corresponding author.
Additional information
Funding
Notes
1 Despite the fact that numerous other terminologies are present in the literature to denote innovation platforms, we use the term agricultural innovation platforms throughout this study in order to be consistent with our project design.
2 It is relevant to note that not all entities may have all the resources and expertise but in the AIP process they are able to identify other actors along the product value chain whom they are able to connect and bring into the AIP to support or implement identified solutions to the barriers.
3 In addition to this study, another project named ‘Virtual Irrigation Academy’, was also carried out in the Kiwere site of Tanzania from 2016. Therefore, the number of surveyed irrigators with monitoring tools in Kiwere within this study was 39, rather than the intended 20. We included these observations in the analysis on the basis that some of the project team members were involved in the two projects and that both of these projects were sponsored by the same institution (ACIAR).
4 It is essential to note that due to insufficient observations, it was difficult to examine the joint influences of AIPs coupled with monitoring tools on farm household outcomes. Hence, our analysis was restricted to examine the impacts of each interventions separately, whilst incorporating the other intervention in the analysis as an independent variable.
5 Cerulli (Citation2017) advised that variables utilised to quantify the similarity of irrigation intervention participants and non-participants needs to be numeric. Given that granting monitoring tools to a group of farmers in each study schemes may lead to self as well as inter-farmer learnings, we incorporated tools location and other variables that were expected to trigger learning to produce the correlation matrix. As such, we considered semi-continuous variables along with numeric variables to calculate the matrix, and conducted extensive sensitivity testing.
6 Although these estimates offer an indication of the spillover effects of AIP events or monitoring tools, caution is advised, as our matrix may not properly reflect the extents of the similarity of intervention participant and non-participant irrigators. Our results could be under or over-estimated.
References
- Abdulai, A., & Huffman, W. (2014). The adoption and impact of soil and water conservation technology: An endogenous switching regression application. Land Economics, 90(1), 26–43. https://doi.org/10.3368/le.3390.3361.3326
- Abebe, F, Zuo, A, Wheeler, S A, Bjornlund, H, Chilundo, M, Kissoly, L., & Dube, T. (2022). The influences on farmers' planned and actual farm adaptation decisions: Evidence from small-scale irrigation schemes in South-Eastern Africa. Ecological Economics, 202, 107594. https://doi.org/10.1016/j.ecolecon.2022.107594
- Abebe, F., Zuo, A., Wheeler, S. A., Bjornlund, H., van Rooyen, A., Pittock, J., … Chilundo, M. (2020). Irrigators’ willingness to pay for the adoption of soil moisture monitoring tools in South-Eastern Africa. International Journal of Water Resources Development, 36(sup1), S246–S267. https://doi.org/10.1080/07900627.07902020.01755956
- Ahimbisibwe, B., Morton, J., Feleke, S., Alene, A., Abdoulaye, T., Wellard, K., … Manyong, V. (2020). Household welfare impacts of an agricultural innovation platform in Uganda. Food and Energy Security, 9(3), e225. https://doi.org/10.1002/fes1003.1225
- Ariga, J., Mabaya, E., Waithaka, M., & Wanzala-Mlobela, M. (2019). Can improved agricultural technologies spur a green revolution in Africa? A multicountry analysis of seed and fertilizer delivery systems. Agricultural Economics, 50(S1), 63–74. https://doi.org/10.1111/agec.12533
- Bang, H., & Robins, J. M. (2005). Doubly robust estimation in missing data and causal inference models. Biometrics, 61(4), 962–973. https://doi.org/10.1111/j.1541-0420.2005.00377.x
- Biggs, S. (2007). Building on the positive: An actor innovation systems approach to finding and promoting pro poor natural resources institutional and technical innovations. International Journal of Agricultural Resources, Governance and Ecology, 6(2), 144–164. https://doi.org/10.1504/IJARGE.2007.012701
- Bisseleua, D., Idrissou, L., Olurotimi, P., Ogunniyi, A., Mignouna, D., & Bamire, S. (2018). Multi-stakeholder process strengthens agricultural innovations and sustainable livelihoods of farmers in southern Nigeria. The Journal of Agricultural Education and Extension, 24(1), 29–49. https://doi.org/10.1080/1389224X.1382017.1392992
- Bjornlund, H., van Rooyen, A., Pittock, J., Parry, K., Moyo, M., Mdemu, M., & de Sousa, W. (2020). Institutional innovation and smart water management technologies in small-scale irrigation schemes in Southern Africa. Water International, 45(6), 621–650. https://doi.org/10.1080/02508060.02502020.01804715
- Bjornlund, H., van Rooyen, A., & Stirzaker, R. (2017). Profitability and productivity barriers and opportunities in small-scale irrigation schemes. International Journal of Water Resources Development, 33(5), 690–704. https://doi.org/10.1080/07900627.07902016.01263552
- Bjornlund, V., Bjornlund, H., & van Rooyen, A. (2020). Exploring the factors causing the poor performance of most irrigation schemes in post-independence Sub-Saharan Africa. International Journal of Water Resources Development, 36(sup1), S54–S101. https://doi.org/10.1080/07900627.07902020.01808448
- Cerulli, G. (2017). Identification and estimation of treatment effects in the presence of (correlated) neighborhood interactions: Model and stata implementation via ntreatreg. The Stata Journal, 17(4), 803–833. https://doi.org/10.1177/1536867X1801700403
- Chilundo, M., de Sousa, W., Christen, E., Faduco, J., Bjornlund, H., Cheveia, E., … Van Rooyen, A. (2020). Do agricultural innovation platforms and soil moisture and nutrient monitoring tools improve the production and livelihood of smallholder irrigators in Mozambique? International Journal of Water Resources Development, 36(sup1), S127–S147. https://doi.org/10.1080/07900627.07902020.01760799
- FAO. (2016). Water withdrawal by sector around 2010. AQUASTAT database. FAO. Retrieved from http://www.fao.org/nr/water/aquastat/tables/WorldData-Withdrawal_eng.pdf, April 10, 2019.
- García-Bolaños, M., Borgia, C., Poblador, N., Dia, M., Seyid, O. M. V., & Mateos, L. (2011). Performance assessment of small irrigation schemes along the Mauritanian banks of the Senegal river. Agricultural Water Management, 98(7), 1141–1152. https://doi.org/10.1016/j.agwat.2011.1102.1008
- Ghirotti, M. (1993). Rapid appraisal: Benefiting from the experiences and perspectives of livestock breeders. World Animal Review, 77, 26–37.
- Holland, P. W. (1986). Statistics and causal inference. Journal of the American Statistical Association, 81(396), 945–960. https://doi.org/10.1080/01621459.1986.10478354
- Hounkonnou, D., Kossou, D., Kuyper, T. W., Leeuwis, C., Nederlof, E. S., Röling, N., … van Huis, A. (2012). An innovation systems approach to institutional change: Smallholder development in West Africa. Agricultural Systems, 108, 74–83. https://doi.org/10.1016/j.agsy.2012.1001.1007
- Imbens, G. W., & Wooldridge, J. M. (2009). Recent developments in the econometrics of program evaluation. Journal of Economic Literature, 47(1), 5–86. https://doi.org/10.1257/jel.1247.1251.1255
- Jew, E. K., Whitfield, S., Dougill, A. J., Mkwambisi, D. D., & Steward, P. (2020). Farming systems and conservation agriculture: Technology, structures and agency in Malawi. Land Use Policy, 95, 104612.
- Kaaria, S., Njuki, J., Abenakyo, A., Delve, R., & Sanginga, P. (2008). Assessment of the enabling rural innovation (ERI) approach: Case studies from Malawi and Uganda. Natural Resources Forum, 32(1), 53–63. https://doi.org/10.1111/j.1477-8947.2008.00174.x
- Kamwamba-Mtethiwa, J., Weatherhead, K., & Knox, J. (2016). Assessing performance of small-scale pumped irrigation systems in Sub-Saharan Africa: Evidence from a systematic review. Irrigation and Drainage, 65(3), 308–318. https://doi.org/10.1002/ird.1950
- Kikuchi, M., Mano, Y., Njagi, T. N., Merrey, D., & Otsuka, K. (2021). Economic viability of large-scale irrigation construction in Sub-Saharan Africa: What if MWEA irrigation scheme were constructed as a brand-new scheme? The Journal of Development Studies, 57(5), 772–789. https://doi.org/10.1080/00220388.00222020.01826443
- Maass, B. L., Musale, D. K., Chiuri, W. L., Gassner, A., & Peters, M. (2012). Challenges and opportunities for smallholder livestock production in post-conflict South Kivu, eastern DR Congo. Tropical Animal Health and Production, 44(6), 1221–1232.
- Manero, A., & Wheeler, S. (2022). Perceptions of Tanzanian smallholder irrigators on impact pathways between water equity and socioeconomic inequalities. International Journal of Water Resources Development, 38(1), 80–107. https://doi.org/10.1080/07900627.07902020.01866506
- Mapila, M. A., Kirsten, J. F., & Meyer, F. (2012). The impact of agricultural innovation system interventions on rural livelihoods in Malawi. Development Southern Africa, 29(2), 303–315. https://doi.org/10.1080/0376835X.0372012.0675699
- Mdemu, M., Kissoly, L., Bjornlund, H., Kimaro, E., Christen, E., Van Rooyen, A., … Ramshaw, P. (2020). The role of soil water monitoring tools and agricultural innovation platforms in improving food security and income of farmers in smallholder irrigation schemes in Tanzania. International Journal of Water Resources Development, 36(sup1), S148–S170. https://doi.org/10.1080/07900627.07902020.01765746
- Mutiro, J., & Lautze’s, J. (2015). Irrigation in Southern Africa: Success or failure? Irrigation and Drainage, 64(2), 180–192. https://doi.org/10.1002/ird.1892
- Ogunniyi, A., Oluseyi, O. K., Adeyemi, O., Kabir, S. K., & Philips, F. (2017). Scaling up agricultural innovation for inclusive livelihood and productivity outcomes in Sub saharan Africa: The case of Nigeria. African Development Review, 29(S2), 121–134. https://doi.org/10.1111/1467-8268.12267
- Osorio-García, A. M., Paz, L., Howland, F., Ortega, L., Acosta-Alba, I., Arenas, L., … Loboguerrero, A. M. (2020). Can an innovation platform support a local process of climate-smart agriculture implementation? A case study in Cauca. Colombia. Agroecology and Sustainable Food Systems, 44(3), 378–411. https://doi.org/10.1080/21683565.21682019.21629373
- Pamuk, H., Bulte, E., Adekunle, A., & Diagne, A. (2015). Decentralised innovation systems and poverty reduction: Experimental evidence from Central Africa. European Review of Agricultural Economics, 42(1), 99–127. https://doi.org/10.1093/erae/jbu1007
- Pamuk, H., Bulte, E., & Adekunle, A. A. (2014). Do decentralized innovation systems promote agricultural technology adoption? Experimental evidence from Africa. Food Policy, 44, 227–236. https://doi.org/10.1016/j.foodpol.2013.1009.1015
- Pamuk, H., & Van Rijn, F. (2019). The impact of innovation platform diversity in agricultural network formation and technology adoption: Evidence from Sub-Saharan Africa. The Journal of Development Studies, 55(6), 1240–1252.
- Parry, K, van Rooyen, A.F, Bjornlund, H, Kissoly, L, Moyo, M., & de Sousa, W. (2020). The importance of learning processes in transitioning small-scale irrigation schemes. International Journal of Water Resources Development, 36(sup1), S199–S223. https://doi.org/10.1080/07900627.2020.1767542
- Quarmine, W., Haagsma, R., Sakyi-Dawson, O., Asante, F., Van Huis, A., & Obeng-Ofori, D. (2012). Incentives for cocoa bean production in Ghana: Does quality matter? NJAS-Wageningen Journal of Life Sciences, 60(1), 7–14. https://doi.org/10.1016/j.njas.2012.1006.1009
- Ragasa, C., & Chapoto, A. (2017). Limits to Green Revolution in rice in Africa: The case of Ghana. Land Use Policy, 66, 304–321. https://doi.org/10.1016/j.landusepol.2017.04.052
- Röling, N. (2009). Pathways for impact: Scientists’ different perspectives on agricultural innovation. International Journal of Agricultural Sustainability, 7(2), 83–94. https://doi.org/10.3763/ijas.2009.0043
- Rosenbaum, P. R., & Rubin, D. B. (1983). The central role of the propensity score in observational studies for causal effects. Biometrika, 70(1), 41–55. https://doi.org/10.1093/biomet/1070.1091.1041
- Rubin, D. B. (1980). Randomization analysis of experimental data: The fisher randomization test comment. Journal of the American Statistical Association, 75(371), 591–593.
- Sanyang, S., Taonda, S. J.-B., Kuiseu, J., Coulibaly, N. T., & Konaté, L. (2016). A paradigm shift in African agricultural research for development: The role of innovation platforms. International Journal of Agricultural Sustainability, 14(2), 187–213. https://doi.org/10.1080/14735903.14732015.11070065
- Schut, M., Cadilhon, J.-J., Misiko, M., & Dror, I. (2018). Do mature innovation platforms make a difference in agricultural research for development? A meta-analysis of case studies. Experimental Agriculture, 54(1), 96–119. https://doi.org/10.1017/S0014479716000752
- Schut, M., Kamanda, J., Gramzow, A., Dubois, T., Stoian, D., Andersson, J. A., … Kassam, S. (2019). Innovation platforms in agricultural research for development: Ex-ante appraisal of the purposes and conditions under which innovation platforms can contribute to agricultural development outcomes. Experimental Agriculture, 55(4), 575–596. https://doi.org/10.1017/S0014479718000200
- Siziba, S., Nyikahadzoi, K., Nyemeck, J. B., Diagne, A., Adewale, A., & Oluwole, F. (2013). Estimating the impact of innovation systems on maize yields: The case of Iar4d in Southern Africa. Agrekon, 52(3), 83–100. https://doi.org/10.1080/03031853.03032013.03821744
- Speelman, S., Frija, A., Perret, S., D’haese, M., Farolfi, S., & D’haese, L. (2011). Variability in smallholders’ irrigation water values: Study in north-west province, South Africa. Irrigation and Drainage, 60(1), 11–19. https://doi.org/10.1002/ird.1539
- Stirzaker, R., Mbakwe, I., & Mziray, N. R. (2017). A soil water and solute learning system for small-scale irrigators in Africa. International Journal of Water Resources Development, 33(5), 788–803. https://doi.org/10.1080/07900627.07902017.01320981
- Sumberg, J. (2005). Systems of innovation theory and the changing architecture of agricultural research in Africa. Food Policy, 30(1), 21–41. https://doi.org/10.1016/j.foodpol.2004.1011.1001
- Tahir, I. S., Mustafa, H. M., Idris, A. A., Elhashimi, A. M., Hassan, M. K., Fadul, E. M., … Assefa, S. (2020). Enhancing wheat production and food security in Sudan through scaling up improved technologies using innovation platforms. International Journal of Agricultural Sustainability, 18(4), 376–388. https://doi.org/10.1080/14735903.14732020.11787639
- The Land Matrix. (2021). Africa. Accessed 03 August 2021. Retrieved from https://landmatrix.org/observatory/africa/.
- UNDESA. (2017). World population prospects: the 2017 revision, volume I: comprehensive tables (ST/ESA/SER.A/399), New York, USA. https://esa.un.org/unpd/wpp/publications/Files/WPP2017_Volume-IComprehensive-Tables.pdf, 24 January 2019.
- van Rooyen, A., Ramshaw, P., Moyo, M., Stirzaker, R., & Bjornlund, H. (2017). Theory and application of agricultural innovation platforms for improved irrigation scheme management in Southern Africa. International Journal of Water Resources Development, 33(5), 804–823. https://doi.org/10.1080/07900627.07902017.01321530
- van Rooyen, A., & Tui, S. H.-K. (2009). Promoting goat markets and technology development in semi-arid Zimbabwe for food security and income growth. Tropical and Subtropical Agroecosystems, 11, 1–5.
- Wheeler, S., Zuo, A., Bjornlund, H., Mdemu, M. V., van Rooyen, A., & Munguambe, P. (2017). An overview of extension use in irrigated agriculture and case studies in south-Eastern Africa. International Journal of Water Resources Development, 33(5), 755–769. https://doi.org/10.1080/07900627.07902016.01225570
- Wollni, M., & Zeller, M. (2007). Do farmers benefit from participating in specialty markets and cooperatives? The case of coffee marketing in Costa Rica. Agricultural Economics, 37(2‐3), 243–248. https://doi.org/10.1111/j.1574-0862.2007.00270.x
- Wooldridge, J. (2005). Violating ignorability of treatment by controlling for too many factors. Econometric Theory, 21(5), 1026–1028.
- Wooldridge, J. (2007). Inverse probability weighted estimation for general missing data problems. Journal of Econometrics, 141(2), 1281–1301.
- World Bank. (2006). Enhancing agricultural innovation: How to go beyond the strengthening of research systems.
- World Bank. (2021). World Development Indicators. Accessed 09 September 2021. Retrieved from https://data.worldbank.org/indicator/AG.YLD.CREL.KG?view = chart.
- You, L., Ringler, C., Wood-Sichra, U., Robertson, R., Wood, S., Zhu, T., … Sun, Y. (2011). What is the irrigation potential for Africa? A combined biophysical and socioeconomic approach. Food Policy, 36(6), 770–782. https://doi.org/10.1016/j.foodpol.2011.09.001
Appendix
Table A1. Probit model results: AIP events and monitoring tools across five southeastern African irrigation schemes in 2017.
Figure A1. Overlap diagram of AIP events and monitoring tools across five southeastern African irrigation schemes in 2017.

Table A2. Over-identification tests for IPWRA, AIPW and IPW approaches across five southeastern African schemes in 2017.