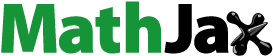
ABSTRACT
Paddy stubble burning poses a major threat to agricultural sustainability in Northern India and an innovative conservation technology ‘happy seeder technology’ is a potential solution for it. Most of the innovation adoption studies usually focus on farmers’ and farm characteristics without giving much emphasis on farmers’ perception regarding the attributes of innovation. But this study focuses on the influence of farmers’ perception regarding attributes of happy seeder technology, viz. relative advantage, compatibility, complexity, trialability and observability on its adoption speed using duration analysis. The survey was conducted in the Indian Punjab, a major contributor to farm fires in Northern India. The findings confirmed the influence of farmers’ perception regarding relative advantage, trialability, observability and complexity on the adoption speed of happy seeder technology. The relative advantage was the strongest influencer of the adoption speed amongst attributes. Based on research findings, we suggest changing farmers’ perception regarding attributes of happy seeder technology through extension activities at critical stages, development of machinery database, cost–benefit comparison, mandatory training along with subsidized machinery and low-cost informational nudges.
Introduction
Smoky skies and hazy grounds are a common occurrence in North-western India in the early winters each year due to endemic levels of air pollution (Venkatramanan et al., Citation2021). One of the primary sources of pollution during this season is the burning of paddy stubble. North-western states (Punjab, Haryana, Uttarakhand and western Uttar Pradesh) produce around 34 million tons of rice residues annually, with approximately two-thirds of this residue being burnt in fields (NAAS, Citation2017).
One ton of paddy straw can release about 4 kg PM2.5, 8 kg P10, 1514 kg CO2, 92 kg CO, 2.7 kg CH4, 0.69 kg black carbon, 1.39 kg NH3, and 0.07 kg N2O, etc. (Venkatramanan et al., Citation2021). Air quality becomes worse in the adjoining areas due to high levels of PM2.5, air pollutants, greenhouse gases and smoke (Chawala & Sandhu, Citation2020; Gupta, Citation2019; Jain et al., Citation2014; Jethva et al., Citation2019; Li et al., Citation2020; Venkatramanan et al., Citation2021). Among the pollutants, particulate matter can easily enter the lungs and cause respiratory and cardio-pulmonary problems (Gupta, Citation2019; Li et al., Citation2020), while the greenhouse gases emitted during paddy straw burning cause global warming. Chakrabarti et al. (Citation2019) estimated that the risk of acute respiratory infection, a major cause of lost disability-adjusted life year, can be three times higher in intense crop-burning areas, so eliminating crop residue burning could prevent the loss of 149,000 disability-adjusted life years, estimated to have a value of US$1.529 billion over the 5-year period. The incidents of road accidents and mobility hindrances increase due to poor visibility caused by smoke from straw burning. Literature has also revealed the loss of various soil nutrients in the fields where stubble is burnt (Kumar et al., Citation2015). Overall, paddy straw burning can be considered a threat to agricultural sustainability. The collection and management of loose paddy straw from the field is costly and laborious process (Lopes et al., Citation2020; Roy et al., Citation2018). Further, due to time-consuming procedures, collection and management can delay the sowing of wheat crops. Delays of up to 1 week from optimum sowing time can reduce wheat yield by 150 kg per acre (PAU, Citation2021). Thus paddy straw management and sowing of wheat in this short time window are challenging for farmers (Keil et al., Citation2021; Lohan et al., Citation2018; Ravindra et al., Citation2019). Despite the negative externalities of stubble burning, most farmers choose burning over other management techniques as they find it the quickest and most economical option (Chawala & Sandhu, Citation2020; Lopes et al., Citation2020).
The Indian government is trying various carrot-and-stick strategies to tackle this problem (Bhuvaneshwari et al., Citation2019; Keil et al., Citation2021) such as instituting a ban on stubble burning (Shyamsundar et al., Citation2019), subsidies on paddy straw management machinery (Keil et al., Citation2017), fine imposition on violators (Gupta, Citation2019) and cash compensation (Reddy, Citation2020). But, the persistence of air pollution due to paddy straw burning reveals that current policies further need improvements (Bhuvaneshwari et al., Citation2019). Researchers are also working on potential solutions to this problem and offered various paddy straw management techniques viz.: incorporation, mulching, composting, bioenergy (Kurinji & Kumar, Citation2021); use in mushroom production; biochar production; and residue management machinery such as baler, zero tillage, and happy seeder (Bhattacharyya et al., Citation2021; Kumar et al., Citation2015; Porichha et al., Citation2021; Ravindra et al., Citation2019). The major challenge in addressing crop residue burning is that it is not only an agricultural or waste management concern but has socioeconomic roots. So technical and capital-intensive solutions alone are less likely to succeed. Therefore, sustainable in-situ management solutions that focus on recycling nutrients from crop residue back into the same fields are more promising (Bhuvaneshwari et al., Citation2019). To manage paddy stubble, more than 1.5 lakh residue management machines have been supplied to farmers of major residue-producing states (PIB, Citation2021). Among these available rice residue management techniques, Happy Seeder Technology (HST), an in-situ management technology, has the highest economic potential, is the most time-efficient and sustainable solution (Shyamsundar et al., Citation2019) ().
With the advent of mechanization, most rice is now harvested using mechanical combine harvesters (Sidhu et al., Citation2007). However, the loose and anchored paddy stubble resulting from mechanical harvesting hinders wheat sowing with zero tillage. This prompted the development of another refined technology, resulting in the form of happy seeder (Sidhu et al., Citation2007). HST is the advancement of zero-till seeder that allows the farmers to directly sow the wheat seeds into standing paddy stubble (Saunders et al., Citation2012). It has the largest potential for reducing greenhouse gasses and air pollution among all rice residue management techniques and 10–20% more economically profitable than the burning option (Shyamsundar et al., Citation2019). Apart from economic and environmental benefits, the residues left in the field after sowing with a happy seeder act as mulch and reduce evaporation and weed growth in wheat crops (Ravindra et al., Citation2019; Saunders et al., Citation2012). The government is providing heavy subsidies to farmers (PIB, Citation2021) to facilitate the adoption of happy seeder technology, which has led to some reduction in farm fires in recent years (Kaushal, Citation2020), although residue burning remains a serious problem in the region (IANS, Citation2021; Pillai, Citation2021; PTI, Citation2021).
Researchers have explored the adoption of conservation technologies, including zero tillage and happy seeder, and identified various socio-personal, farm biophysical and economic factors as determinants of adoption (Grabowski et al., Citation2016; Keil et al., Citation2021; Knowler & Bradshaw, Citation2007; Kumar et al., Citation2010; Ntshangase et al., Citation2018). However, we argue that technology adoption is not solely the product of the constructs mentioned above, but a blend of many other constructs, of which attributes of technology and farmers’ perception regarding these attributes hold an important place. Scholars have studied the impacts of attributes on technology adoption in terms of impact of relative advantage (profitability, risk investment) and complexity on the adoption of dairy technologies (Batz et al., Citation1999); the impact of novelty, utility and adaptability of agricultural innovations (Mutsvangwa-Sammie et al., Citation2018); the impact of production (yield, drought tolerance and early maturity) and processing/consumption (storability, poundability, flour to grain ration and taste) attributes of maize varieties on its adoption (Lunduka et al., Citation2012); the impact of relative advantage, compatibility, observability, trialability and complexity on the adoption of integrated pest management in cotton (Peshin, Citation2013); the impact of input cost saving on autosteer adoption by cotton farmers (D’Antoni et al., Citation2012) and the impact of relative advantage, compatibility, and observability on the adoption of agricultural best management practices (Reimer et al., Citation2012). However, the influence of attributes on the adoption of HST has never been studied. Furthermore, adoption researchers have studied adoption by using static bivariate methods (Abdulai & Huffman, Citation2014; Bagheri et al., Citation2019; D’Antoni et al., Citation2012; Lunduka et al., Citation2012; Sidibe, Citation2005), where only the event when adoption occurred was taken as the point of reference. It is inadequate to study only the final decision (adoption or non-adoption); rather, the entire adoption process is a matter of study. This paper attempts to explore factors affecting the duration from awareness to the adoption of HST, rather than focusing solely on the adoption stage. Thus this study quantifies the effect of attributes of HST on its adoption speed using duration analysis. Duration analysis has been used by various adoption researchers (Abdulai et al., Citation2005; Alcon et al., Citation2011; Beyene & Kassie, Citation2015; Burton et al., Citation2003; D’Emden et al., Citation2006; Dadi et al., Citation2004; Murage et al., Citation2011). This study is the foremost attempt where duration analysis has been used to empirically test the conceptual framework regarding happy seeder adoption. Hence, the study addressed novel theoretical and methodological aspects related to determinants of HST adoption.
Material and methods
Study area
The study was conducted in Punjab, a north-western state of India, which extends from the latitudes 29.30° North to 32.32° North and longitudes 73.55° East to 76.50° East (). It is one of the most fertile regions on Earth, comprising alluvial soils, which is the outcome of the Indo-Gangetic river system. Agriculture is the predominant occupation of the people of Punjab, with 4.12 mha net sown area, of which 4.07 mha area is irrigated. The farming in the state is highly intensified with a cropping intensity of 189.1 (GOP, Citation2021), which indicates that on average, two crops are grown by the farmer on the same piece of land per crop year. In most parts of the state, these two crops are rice and wheat. Having only 1.53% area of the total geographical area of the country, yet it contributes about 20.9% and 37.8% of the country’s rice and wheat pool annually, respectively. Apart from contributing to agriculture production, Punjab remains in the limelight due to paddy stubble burning. Although, more than thirty thousand happy seeder were deployed in Punjab by December 2020 (Kurinji & Prakash, Citation2021). Yet by the Kharif season of 2021, about seventy thousand farm fire-burning events were recorded in Punjab (Kurinji & Prakash, Citation2021; PRSC, Citation2021).
The field surveys were conducted in 2018–2019 to collect the data. Due to non-uniform distribution of happy seeder adopters, simple random sampling was not an appropriate technique to draw a sample for empirical analysis. The sample selection was therefore done in two phases. In the first phase, a list of farmers who bought the happy seeder through the Department of Agriculture and Farmer’s Welfare Punjab was obtained. Two districts were selected from each of the five agro-climatic zones of Punjab based on the number of happy seeder adopters (Sub-mountain undulating zone: Gurdaspur, Hoshiarpur; Undulating Plain zone: Sahid Bhagat Singh Nagar, Rupnagar; Central Plain zone: Ludhiana, Patiala; Western Plain zone: Faridkot, Ferozpur; Western zone: Mansa, Bathinda). Next, a cluster of villages with the highest number of happy seeders in each district was identified to draw the farmers’ sample. From each district, 18 adopters were selected randomly, resulting in the survey of 180 adopters. However, it was observed that despite having similar exposure to the technology, several farmers had not adopted the technology, resulting in the longer duration to the adoption of happy seeder technology. Therefore 60 non-adopter farmers (6 from each cluster) were also included in the study. From the obtained 240 questionaries, 11 were rejected due to missing information. Thus a final sample of 229 farmers, comprising 177 adopters and 52 non-adopters (), was retained for the final analysis.
Table 1. Agro-climatic zone and district-wise sample of adopters and non-adopters of happy seeder technology.
Data and variables
An interview schedule was developed to collect quantitative and qualitative information regarding farmers’ perceptions of attributes of HST, and farmer and farm characteristics. The dependent variable for the study was the speed of adoption, measured in terms of duration (time in months) between the awareness and adoption time of farmers (if HST was adopted). For non-adopters, the duration between awareness and study time was considered. Based on the conceptual framework of this study and a review of previous studies, several explanatory variables were identified (). To study farmers’ perception toward the attributes of HST, the study included five major attributes outlined in , as these explained most of the variance (49–87%) in the adoption rate of innovations (Rogers, Citation2003). Relative advantage, compatibility, trialability and observability were hypothesized to be positively related to the speed of adoption of happy seeder by the farmers, whereas complexity was hypothesized to be negatively related.
Table 2. Indicators of the attributes of the happy seeder technology.
To measure the farmers’ perception of HST attributes, various indicators were developed and measured on a three-point continuum (). The study examined relative advantage of HST over conventional tillage in terms of cost of field preparation, timely sowing of wheat, reduction in weed count, time-saving in sowing, irrigation water saving, fertilizer saving, soil health improvement, reduction in required implements to manage paddy straw, air pollution control, saving of money, saving beneficial friendly insects, yield increase and increase in net profit. The compatibility of HST was studied in terms of compatibility with tractors owned by the farmer, straw management equipped with a combine harvester, seed drilling in standing stubble, soil type of the field, economic status of farmer, cultivated rice varieties, and farmer’s mindset. The complexity of HST was measured in terms of complexity in machine adjustment for appropriate seed depth, adjustment with the hydraulic system of tractor, the requirement of skilled labour, the process of purchasing and subsidy availability, the maintenance of moisture in field, sowing in standing stubbles, the control of rodents and termites, and additional equipment requirements. The trialability of HST was studied in terms of availability, approachability to agencies, land availability, high-power tractor availability and applicability in small fields. Observability was measured in terms of germination, vegetative growth, plant height and vigor, rodent infestation, reduction in fertilizer requirement, reduction in herbicide requirement, yield, environmental benefits, water savings and soil health improvement.
To provide a comprehensive understanding of farmers’ perception, the study used both quantitative and qualitative data. In addition to answering structured questions, respondents were also encouraged to share their views on the attributes of HST during interviews. Notes of the qualitative information were taken to complement the quantitative findings with narratives of the farmers representing the majority view. To protect the anonymity of the respondents, only the identification code of the farmers is provided alongside their narrative in the findings. Another category of explanatory variables included in the study was farmer and farm characteristics. Age at adoption was hypothesized to be negatively related to adoption speed (Alcon et al., Citation2011; Beyene & Kassie, Citation2015; Canales et al., Citation2017; Kallas et al., Citation2009), whereas other variables, i.e. education, farm size, extension contacts, mass media exposure, innovativeness, risk orientation and environmental consciousness were expected to be positively related with the adoption speed of HST (Alcon et al., Citation2011; Canales et al., Citation2017; D’Emden et al., Citation2006; Kallas et al., Citation2009; Lapple & Kelley, Citation2015). The latent variables innovativeness, risk orientation and environmental consciousness were measured by using scales developed by Singh (Citation1972), Supe and Singh (Citation1976) and Roy (Citation2015), respectively ().
Table 3. Operationalization of explanatory variables and their hypothesized relationship with adoption speed.
Duration analysis model specifications
Duration Analysis is the synonym of survival analysis. As the name ‘survival’ indicates, this statistical technique was developed to study the factors associated with human death in the eighteenth century (Turiano, Citation2016). In survival analysis, the time variable is referred to as survival time, because originally it was used to describe the time for which an individual survives. Another important term in survival analysis is the event, called ‘failure’, because the event of interest was usually disease, death or other negative incidents in epidemiological studies (Kleinbaum & Klein, Citation2005). This technique is used by various researchers in different fields with different names such as transition analysis in economics, failure time analysis and reliability analysis in engineering and history analysis in sociology (Allison et al., Citation2010). The prime focus of researchers in duration analysis is to study the factors associated with the time taken by subjects to transit from one state to another. Duration analysis has also been employed in agricultural technology adoption studies (Alcon et al., Citation2011; Beyene & Kassie, Citation2015; Burton et al., Citation2003; Canales et al., Citation2017; D’Emden et al., Citation2006; Dadi et al., Citation2004; Fuglie & Kascak, Citation2001; Lapple, Citation2010) to study factors affecting duration taken by farmers from first hearing of, to the adoption of, agricultural technology. In the context of adoption studies, the term event ‘failure’ can be regarded as the adoption of technology by the farmer, and survival/duration as the time taken by the farmer to reach that event, i.e. adoption.
For the present study, duration time was defined as the period from when farmers became aware of HST to the time they adopted this technology. In other words, duration was measured as the time it took for an individual farmer to move awareness to the adoption stage. The duration was used to express the speed of adoption which refers to the rate at which an individual farmer moves from the awareness to the adoption stage of HST. However some farmers had not adopted HST by the end of the study period, the exact duration of the event for these individuals is unknown. In such conditions, the appropriate statistical process to use is censoring. For non-adopters, observations were right-censored, and the time of the end of the study period was considered as the endpoint. Let T denote time duration from awareness to adoption (in months) and t is some specific value of T. So the probability of adoption of HST by the farmer at time t (when adoption has not occurred before) is defined by conditional distribution function as follows:
(1)
(1) But not all the farmers adopted HST during the study period. So, the probability of a person requiring more than some specified time (t) is defined as survival function
.
(2)
(2)
The major research question of this study, i.e. to identify the factors that influenced the duration from awareness to the adoption of HST was answered by use of hazard function . The hazard function gives the instantaneous potential per unit time to reach at adoption stage, given that farmer has not already adopted happy seeder up to time t (Kleinbaum & Klein, Citation2005).
(3)
(3)
Further to estimate the duration model, the Cox proportional hazards model (Cox, Citation1972) was used. The major reason behind the popularity of the Cox proportional hazard model is that it can give results comparable to correct parametric models when the actual distribution is unknown (Kleinbaum & Klein, Citation2005). The formula for the Cox proportional hazards model is as follows:
(4)
(4)
Here the model gives an expression for hazard at time t with a set of explanatory variables (X) for an individual. The baseline hazard function is , whereas the second term is the relative hazard. We also employed a non-parametric approach called the Kaplan–Meier estimate of the survivor function. It does not assume the distribution of survival times for the survivor function (Kaplan & Meier, Citation1958). Such summaries are useful for suggesting appropriate functional forms for further analysis (Kiefer, Citation1988). In technology adoption studies, these may also help by depicting the speed of adoption of different technologies and facilitating comparisons of individuals sampled from different populations (Gao et al., Citation2019). But in this current study, we investigated a single technology, i.e. HST, with a single population. Since the data contains censored observations, the Kaplan–Meier method was used to summarize the length of time farmers waited before adopting the technology. The event, origin, and time scale are important decisions in duration analysis. Here, the event was the adoption of HST. The origin of time was considered when a farmer became aware of HST, and the scale of time was taken as months.
For the present study, it was hypothesized that farmers’ perception regarding relative advantage, compatibility, trialability and observability has a positive relationship with the speed of adoption of HST. In contrast, their perception regarding complexity has a negative relation with the speed of adoption of this technology. In other words, the time duration between the awareness stage to the adoption stage will be less for farmers who perceive HST as relatively more advantageous, compatible, trialable, observable, and less complex or vice versa.
Results and discussion
Descriptive results for variables used in the empirical model are presented in . The average adoption time for HST by farmers was about 38 months. On average, adopters perceived HST as more advantageous, compatible, trialable and observable than non-adopters. Findings given in indicate that adopters are somewhat younger and more educated than non-adopters. The farm size of the adopters was almost double that of non-adopters but had less farming experience. The farmers who adopted HST contacted extension personnel more frequently and used more print, electronic, and social media to get agricultural information. But, in the case of behavioural constructs viz. innovativeness, risk orientation, and environment consciousness, both adopters and non-adopters had similar perceptions.
Table 4. Descriptive statistics for variables used in duration model.
The categorization of farmers into adopters and non-adopters has only been done to visualize the end product (adoption/non-adoption). The novel aspect of duration analysis studies is that it takes into consideration the whole time duration of the adoption process, rather than just the end product as in logit regression techniques. This paper is an attempt to present the journey of a farmer from the awareness of HST to its adoption as an end product, and the time taken in the process.
Duration analysis results
In the duration or survival analysis, it is common practice to have some summary of the survival times of all the individuals in the sample. Kaplan–Meier (KM) survival estimate gives a graphical representation of the estimated probability of survival at a given point of time. In the current study, the survival function represents the probability of a farmer not adopting HST at a given point of time. The survival estimates for the adoption of HST were calculated, and the distribution is illustrated in . The vertical axis of the graph represents the probability of non-adoption ranging from 0 to 1, and the horizontal axis represents the durations in months, scaled from 0 to 80.
So, at time t = 0, the probability of non-adoption is 1 meaning that none of the farmers had adopted the technology at this point of time. The decreasing value of the function across the graph indicates that for an individual farmer, the probability of not adopting HST decreases with time, indicating an increase in the adoption of the technology. At 20 months, a KM estimate of 0.86 implies that, after 20 months of being aware of HST, there is an 86% chance that farmers will take more time to adopt. It can be also looked at from another angle, that there is a 14% chance that a farmer will adopt HST within 20 months of awareness. The probability of non-adoption continues to decrease with time, leading to a reduction in non-adopters, or increase of adopters. At 40 and 60 months, the probability of continuing farming without adopting HST was 0.45 and 0.25, respectively. Data indicate that 77 farmers were unlikely to adopt happy seeder after 40 months of awareness, while only three farmers were unlikely to adopt HST after 80 months. The median time for adopting HST was about 38 months.
Factors affecting the adoption duration
The Cox proportional hazard model was employed to determine the hazards of various explanatory variables. The Cox proportional hazards model, commonly known as Cox regression, is a semi-parametric approach, which has been widely used in biomedical, economics, engineering, behavioural and social sciences (Guo, Citation2010). Before the Cox model, the researchers used other parametric models, which make strict assumptions regarding the nature of the distribution. But Cox regression is a distribution-free model. So in this model, the actual form of the survival distribution does not matter and researchers can use the same Cox regression model for all (Allison et al., Citation2010). The significance of the likelihood ratio (108.00) and Wald statistics (72.02) determined the acceptability of Cox regression model for this study ().
Table 5. Estimates of duration model for adoption speed of happy seeder technology.
It was hypothesized that attributes of innovation perceived by the farmers play a similar role to the speed of adoption as in the rate of adoption. As expected, it was found that relative advantage, trialability and observability had a significant and positive influence on the speed of adoption of HST, while complexity had a significant negative influence on the same. But contrary to the hypothesis, compatibility had no significant effect on the speed of adoption in the case of HST. Among the attributes that had significant influence, relative advantage was the highest, and complexity was the lowest influence.
The farmers, who perceived HST to be relatively advantageous compared to the conventional method of wheat sowing, had a higher likelihood of early adoption. With a one unit increase in perception regarding the relative advantage of HST, the adoption speed had probably increased by about 3.5 times. Further discussion with farmers indicated that they perceived the HST to be relatively advantageous compared to traditional wheat sown practice, in terms of saving time and money. In the conventional practice, farmers burn residues and cultivate the field to prepare the seedbed to sow wheat crops. But, HST replaces all these practices with a single practice.
Kaur et al. (Citation2021) conducted a study to assess the impact of HST in Punjab and found that sowing of the wheat crop through HST is cost-effective compared to the conventional method, since no cost is involved for the land preparation under HST (compared to an average of about 50 USDFootnote1 ha−1 involved under conventional method). In the conventional method, farmers cultivated the field multiple times with equipment for seedbed preparation after burning the paddy straw. On the other hand, HST only requires the cost of about five USD ha−1 to distribute loose straw left over after combine harvesting of paddy to avoid choking of HST. Herbicide application cost is also almost double in the conventional method as compared to HST, with a saving of about 12 USD ha−1 in HST sown wheat. HST sown wheat also prevents weed growth due to soil coverage by paddy straw as mulch. The average labour cost is also less in the HST and there is no cost of cutter cum spreader in HST, as compared to about 13 USD ha−1 in the conventional method. Overall, gross savings in various operations (land preparation, irrigation, herbicide application, labour cost and cutter cum spreader) in wheat sown with HST over the conventional method was about 47 USD ha−1. Average man-days/acre were also found to be less under HST (10.65) than the conventional method (12.41). Keil et al. (Citation2021) reported similar findings regarding the relative advantage of HST in terms of saving time and money. They also revealed that happy seeder also reduces the operational time from 430 to 168.3 min/ha leading to a reduction in cost from USD 52.65–28.50 ha−1 (Keil et al., Citation2021). Similarly, Singh et al. (Citation2008) also exhibited the financial benefit of happy seeder sown wheat over conventional tillage sown wheat, of about 33-35 USD ha-1. In addition to financial benefits at the time of sowing, various other benefits such as saving in fertilizer, herbicide, diesel, water, electricity, labour were reported by researchers (Singh et al., Citation2008). A financial analysis conducted by Sidhu et al. (Citation2007) revealed that the use of HST is economical for farmers. An average 9–15% higher grain yield of wheat was recorded with happy seeder sowing in rice residue. The paddy straw in the field acts as mulch which regulates the temperature of the soil by up to 2°C. It was also observed that wheat sown with a happy seeder had one-third less weed growth. Sidhu et al. (Citation2007) further explained that it increases the water-holding capacity of the soil and improves the organic carbon in the soil.
Observability had a significant positive influence on the speed of adoption of HST. One unit increase in the observability increased the hazards of the speed of adoption by about 2.5 times. Observability is the degree to which results of an innovation are visible (Rogers, Citation2003). Farmers likely to pay more attention to technologies with higher observable positive characteristics or vice-versa. In the case of HST, one of the non-adopter farmers recalled:
When I used happy seeder for wheat sowing in the standing stubbles, I was apprehensive about the germination of wheat seed because all I could see were the standing stubbles of rice in my field even after sowing the seed. I really wanted to plough my field, get rid of the stubbles and sow the wheat crop conventionally. (Farmer 218)
Technologies that can be tried on an installment basis in a limited space and time are adopted more often and more quickly than less-trialable innovations (Rogers & Shoemaker, Citation1971). Trialability was found to enhance the speed of adoption of HST significantly. It was found that a one-unit increase in trialability, increased hazards of early adoption by about 2.25 times. The response of farmers revealed that happy seeder and a high-power tractor were easily available for trials. In-depth discussion with the adopters revealed that agencies conducting trials of HST were easily approachable. Numerous government agencies viz. State Department of Agriculture and Farmers’ Welfare, State Agricultural Universities, Agricultural Cooperative Societies, etc. conducts demonstrations throughout the state to minimize the practice of straw burning. The government also provided 80% subsidy to the group of farmers who purchase happy seeder machines. Machines available for the trials allow farmers to have a first-hand experience of the technology, which enhance the speed of adoption. It is important to address the cognitive dissonance of the farmers. Farmers practising conventional sowing of wheat for years are stressed by contradictory beliefs. Providing them with trials not only offers a sense of credibility but also assures that they are making the right decision regarding the technology. So, increasing the number of trials helps to enhance the adoption speed of this technology.
Complexity had a significant negative influence on the speed of adoption of HST. This technology is still new for a large section of farmers. The happy seeder drill often requires on-field tuning according to soil conditions. This requires skill on the part of farmers who are using this machine. But many times, it has been observed that the machine doesn't work smoothly on the farmers’ field because of a lack of operating skills, which discourages them from using it. While conducting this research, a non-adopter farmer recalled:
I brought the happy seeder machine from the cooperative society on a custom hiring basis for sowing wheat in the standing rice stubbles on my field but the machine was not running smoothly. It was shutting down again and again. I immediately realized that this machine is not going to work on my field and I dropped the idea of using it. (Farmer 186)
Many such incidents have been narrated by farmers who lacked the skills to operate this machine. A skilled and experienced driver of this machine can make slight adjustments in the machine with respect to the tractor with which the machine is attached. One of the farmers, who operates this machine for sowing wheat on the fields of other farmers on a custom hiring basis, narrated:
Happy Seeder Machine should be operated using a tractor with 50–55 hp. A tractor with lesser hp does not allow the machine to run smoothly in the standing stubbles. Also, the machine should be operated at a double-clutch and care should be taken of the rpm (revolutions per minute) based on the type of soil, moisture content and amount of straw present in the field. To avoid the clogging of paddy straw in the tynes of the happy seeder machine, the rotor should be started initially to loosen the straw before sowing. (Farmer 32)
Conclusion and policy recommendation
Crop residue burning is a widespread practice in many countries (Lin & Begho, Citation2022) including India, where leftover of paddy crop has been burnt on a large scale in north-western states (Lin & Begho, Citation2022; Shyamsundar et al., Citation2019). Happy seeder technology, a machine that can sow in standing stubbles of crop, is considered a major solution to the residue burning problem (Keil et al., Citation2021; Shyamsundar et al., Citation2019). policymakers have questions regarding the factors influencing the adoption of this technology to develop strategies for adoption enhancement. In the adoption process, farmers evaluate the new technology by comparing with the available counterparts based on attributes such as relative advantage, compatibility, complexity, trialability, observability and move towards the adoption stage from awareness if they have a favourable perception regarding new technology. Thus current investigation has been conducted in the Indian Punjab to determine the influence of these attributes on the adoption speed of HST using duration analysis.
The findings illustrated that the favourable perception of farmers regarding relative advantage, observability and trialability of HST significantly increases the speed of adoption. In contrast, a favourable perception regarding the complexity of HST reduces the adoption speed. Thus policymakers, researchers and extension workers should emphasize changing farmers’ perception regarding HST based on these very attributes. Researchers and extension functionaries are already emphasizing the relative advantages of HST in terms of saving in cost of cultivation, time and labour (Kaur et al., Citation2021; Keil et al., Citation2021; Sidhu et al., Citation2007; Singh et al., Citation2008) that can be further visualized to farmers by encouraging to keep a financial record to reveal the cost and benefit of HST in comparison to conventional technologies ().
Figure 5. Scientists demonstrating the sowing process of wheat with Happy Seeder Technology to farmers.

To influence the farmers’ perceptions regarding observability, extension functionaries should follow the ‘seeing is believing’ principle. They should identify the critical stages, such as the sowing and germination stages, which create uncertainty in farmers’ minds regarding the growth of crops. Extension activities should be organized at these identified stages to alter farmers’ perception regarding observability. To reduce the complexity of HST, training should be made mandatory at the time of providing machines as subsidy to farmers. Youth should be trained and encouraged to provide custom hiring services of HST. These trainees can further act as opinion leaders in a particular area and enhance the adoption speed of HST.
The government is providing machines to farmers at subsidized prices (GoI, Citation2020) to make HST easily accessible to enhance trialability and adoption. Further demand and availability of machines at the village or block level should be assessed by extension functionaries. Small landholders should be provided with and encouraged to use the machinery on custom hiring basis from cooperative societies and large farmers or to purchase the happy seeder machinery only in groups to minimize the financial burden. For the ease of farmers, a database of the available machines can be made available through various ICT tools. The availability of machines in a particular area can be put in the public domain by developing and popularizing machine renting applications, such as i-machine development by the Government of Punjab, Farm Machinery app by Punjab Agricultural University, where cooperative societies and large farmers can rent out the happy seeder machines and small landholders can easily locate the machine to use on custom hiring basis. Policymakers should also acknowledge that promoting the adoption of pro-environmental technologies requires a change in attitude, which can be facilitated by creating social norms. The literature revealed that social norm interventions are effective at inducing significant changes in behavior and that descriptive norms seem to demonstrate particularly consistent effects in this regard (Farrow et al., Citation2017). So, adding a collective dimension to schemes to promote HST can improve their effectiveness by increasing initial participation and encouraging farmers to use HST on more of their land (Kuhfuss et al., Citation2016). Further low-cost informational nudges can play a major role in altering the perception of farmers and enhancing the adoption of HST (Lopes et al., Citation2023).
Thus, based on above discussed findings and policy implications, an attempt has been made towards the methodological enrichment and policy enhancement about the adoption of happy seeder technology, reduction in environmental pollution, and further improvement in agricultural sustainability.
Disclosure statement
No potential conflict of interest was reported by the author(s).
Additional information
Notes on contributors
Manmeet Kaur
Dr. Manmeet Kaur is an Associate Professor in the Department of Extension Education at Punjab Agricultural University, Ludhiana (India). Her research interests include experimenting with behavioral interventions to develop and strengthen pro-environmental behavior among farmers, as well as enhancing the adoption of conservation agricultural technologies. She has worked to develop methodologies for popularizing agricultural technology among small and marginal farmers in Punjab. Dr. Kaur has also authored and co-authored 30+ papers in reputed journals.
Dalbeer Singh
Dalbeer Singh is a Senior Research Fellow in the Department of Extension Education at Punjab Agricultural University, Ludhiana. He holds a Ph.D. in Extension Education and works in the field of sustainable agriculture. His research interests include diffusion and adoption of innovations, attitude scale construction, attitude-behavioral relationship, and behavioral change research.
Anupam Anand
Anupam Anand has completed his doctoral degree in Extension Education from Punjab Agricultural University, Ludhiana (Punjab, India). He is currently working as a MANAGE Fellow in the Centre for Agricultural Extension Innovations, Reforms & Agripreneurship at the National Institute of Agricultural Extension Management (MANAGE), Hyderabad, India. Dr. Anand has authored and co-authored ten research papers for peer-reviewed national journals in India.
Taranpreet Singh
Taranpreet Singh is a Senior Research Fellow at the Agricultural Technology Application Research Institute (ICAR-ATARI), Ludhiana. He holds an M.Sc in Extension Education from Punjab Agricultural University, Ludhiana (Punjab), and conducted research on the adoption of Happy Seeder Technology in Punjab, India for his master's degree.
Notes
1 Currency converted from Indian Rupee (INR) to United States Dollar (USD); 1 USD = 74.70 INR (31 December 2021).
References
- Abdulai, A., & Huffman, W. (2014). The adoption and impact of soil and water conservation technology: An endogenous switching regression application. Land Economics, 90(1), 26–43. https://doi.org/10.3368/le.90.1.26
- Abdulai, A., Huffman, W. E., & Curtiss, C. F. (2005). The diffusion of new agricultural technologies: The case of crossbred-cow technology in Tanzania. American Journal of Agricultural Economics, 87(3), 645–659. https://doi.org/10.1111/j.1467-8276.2005.00753.x
- Alcon, F., de Miguel, M. D., & Burton, M. (2011). Duration analysis of adoption of drip irrigation technology in southeastern Spain. Technological Forecasting and Social Change, 78(6), 991–1001. https://doi.org/10.1016/j.techfore.2011.02.001
- Allison, P. D. (2010). Survival analysis using SAS: A practical guide. SAS Publication. https://doi.org/10.1111/j.1600-0587.2013.07872.x
- Bagheri, A., Bondori, A., & Damalas, C. A. (2019). Modeling cereal farmers’ intended and actual adoption of integrated crop management (ICM) practices. Journal of Rural Studies, 70, 58–65. https://doi.org/10.1016/j.jrurstud.2019.05.009
- Batz, F.-J., Peters, K. J., & Janssen, W. (1999). The influence of technology characteristics on the rate and speed of adoption. Agricultural Economics, 21(2), 121–130. https://doi.org/10.1111/j.1574-0862.1999.tb00588.x
- Beyene, A. D., & Kassie, M. (2015). Speed of adoption of improved maize varieties in Tanzania: An application of duration analysis. Technological Forecasting and Social Change, 96, 298–307. https://doi.org/10.1016/j.techfore.2015.04.007
- Bhattacharyya, P., Bisen, J., Bhaduri, D., Priyadarsini, S., Munda, S., Chakraborti, M., Adak, T., Kumar, S., & Nimbrayan, P. (2021). Turn the wheel from waste to wealth: Economic and environmental gain of sustainable rice straw management practices over field burning in reference to India. Science of The Total Environment, 775, 145896. https://doi.org/10.1016/j.scitotenv.2021.145896
- Bhuvaneshwari, S., Hettiarachchi, H., & Meegoda, J. N. (2019). Crop residue burning in India: Policy challenges and potential solutions. International Journal of Environmental Research and Public Health, 16(5), 832. https://doi.org/10.3390/ijerph16050832
- Burton, M., Rigby, D., & Young, T. (2003). Modelling the adoption of organic horticultural technology in the UK using duration analysis. The Australian Journal of Agricultural and Resource Economics, 47(1), 29–54. https://doi.org/10.1111/1467-8489.00202
- Canales, E., Bergtold, J.S., Williams, J.R. (2017). Modeling the factors affecting farmers’ timing of adoption of in-field conservation cropping practices. Chicago. https://doi.org/10.22004/AG.ECON.258558
- Chakrabarti, S., Khan, M. T., Kishore, A., Roy, D., & Scott, S. P. (2019). Risk of acute respiratory infection from crop burning in India: Estimating disease burden and economic welfare from satellite and national health survey data for 250000 persons. International Journal of Epidemiology, 48(4), 1113–1124. https://doi.org/10.1093/ije/dyz022
- Chaudhary, A., Chhokar, R. K., Yadav, D. B., Sindhu, V. K., Ram, H., Rawal, S., Khedwal, R. S., Sharma, R. K., & Gill, S. C. (2019). In-situ paddy straw management practices for higher resource use efficiency and crop productivity in Indo-Gangetic Plains (IGP) of India. Wheat and Barley Research, 11(3), 172–198. https://doi.org/10.25174/2249-4065/2019/96323
- Chawala, P., & Sandhu, H. A. S. (2020). Stubble burn area estimation and its impact on ambient air quality of Patiala & Ludhiana district, Punjab, India. Heliyon, 6(1), e03095. https://doi.org/10.1016/j.heliyon.2019.e03095
- Cox, D. R. (1972). Regression models and life tables. Journal of the Royal Statistical Society Series B, 34(2), 187–220. https://doi.org/10.1111/j.2517-6161.1972.tb00899.x
- Dadi, L., Burton, M., & Ozanne, A. (2004). Duration analysis of technological adoption in Ethiopian agriculture. Journal of Agricultural Economics, 55(3), 613–631. https://doi.org/10.1111/j.1477-9552.2004.tb00117.x
- D’Antoni, J. M., Mishra, A. K., & Joo, H. (2012). Farmers’ perception of precision technology: The case of autosteer adoption by cotton farmers. Computers and Electronics in Agriculture, 87, 121–128. https://doi.org/10.1016/j.compag.2012.05.017
- D’Emden, F. H., Llewellyn, R. S., & Burton, M. P. (2006). Adoption of conservation tillage in Australian cropping regions: An application of duration analysis. Technological Forecasting and Social Change, 73(6), 630–647. https://doi.org/10.1016/j.techfore.2005.07.003
- Farrow, K., Grolleau, G., & Ibanez, L. (2017). Social norms and pro-environmental behavior: A review of the evidence. Ecological Economics, 140, 1–13. https://doi.org/10.1016/j.ecolecon.2017.04.017
- Fuglie, K. O., & Kascak, C. A. (2001). Adoption and diffusion of natural-resource-conserving agricultural technology. Review of Agricultural Economics, 23(2), 386–403. https://doi.org/10.1111/1467-9353.00068
- Gao, Y., Zhao, D., Yu, L., & Yang, H. (2019). Duration analysis on the adoption behavior of green control techniques. Environmental Science and Pollution Research, 26(7), 6319–6327. https://doi.org/10.1007/s11356-018-04088-9
- GoI. (2020). Central sector scheme on promotion of agricultural mechanization for in-situ management of crop residue in the states of Punjab, Haryana, Uttar Pradesh and Nct of Delhi. Mechanization and Technology Division, Department of Agriculture, Cooperation and Farmers Welfare, Ministry of Agriculture and Farmers Welfare, Government of India. https://agricoop.nic.in/sites/default/files/Guidelines-2019_%28revised%29_of_Straw_Management_Scheme_0.pdf
- GOP. (2021). Statistical Abstract of Punjab. Economic and Statistical Organization, Government of Punjab (India). https://esopb.gov.in/static/PDF/Abstract2021.pdf. https://esopb.gov.in/static/PDF/Abstract2021.pdf
- Grabowski, P. P., Kerr, J. M., Haggblade, S., & Kabwe, S. (2016). Determinants of adoption and disadoption of minimum tillage by cotton farmers in eastern Zambia. Agriculture, Ecosystems & Environment, 231, 54–67. https://doi.org/10.1016/j.agee.2016.06.027
- Guo, S. (2010). Survival analysis, pocket guides to social work research methods. Oxford University Press.
- Gupta, N. (2019). Paddy residue burning in Punjab: Understanding farmers ‘ perspectives and rural air pollution. CEEW (The Council on Energy, Environment and Water).
- Henderson, R. M., & Clark, K. B. (1990). Architectural innovation: The reconfiguration of existing product technologies and the failure of established firms. Administrative Science Quarterly, 35(1), 9–30. https://doi.org/10.2307/2393549
- IANS. (2021). Punjab farm fires till now less than last year’s, but more than 2019. Bus. Stand. News.
- Jain, N., Bhatia, A., & Pathak, H. (2014). Emission of air pollutants from crop residue burning in India. Aerosol and Air Quality Research, 14(1), 422–430. https://doi.org/10.4209/aaqr.2013.01.0031
- Jethva, H., Torres, O., Field, R. D., Lyapustin, A., Gautam, R., & Kayetha, V. (2019). Connecting crop productivity, residue fires and air quality over Northern India. Scientific Reports, 9(1), 16594. https://doi.org/10.1038/s41598-019-52799-x
- Kallas, Z., Serra, T., & Gil, J. M. (2009). Farmer’s objectives as determinant factors of organic farming adoption. 113th EAAE Seminar “A Resilient European Food Industry and Food Chain in a Challenging World.” Chania, Greece.
- Kaplan, E. L., & Meier, P. (1958). Nonparametric estimation from incomplete observations. Journal of the American Statistical Association, 53(282), 457–481. https://doi.org/10.1080/01621459.1958.10501452
- Kaur, M., Narang, M. K., Bhalla, J. S., Dhaliwal, R. K., & Sharma, S. (2021). Impact assessment of Happy Seeder Technology and its popularization among the Farmers of Punjab. Ludhiana, India.
- Kaushal, L. A. (2020). Examining the policy-practice gap – The issue of crop burning induced particulate matter pollution in northwest India. Ecosystem Health and Sustainability, 6, 18646. https://doi.org/10.1080/20964129.2020.1846460
- Keil, A., D’souza, A., & McDonald, A. (2017). Zero-tillage is a proven technology for sustainable wheat intensification in the Eastern Indo-Gangetic Plains: What determines farmer awareness and adoption? Food Security, 9(4), 723–743. https://doi.org/10.1007/s12571-017-0707-x
- Keil, A., Krishnapriya, P. P., Mitra, A., Jat, M. L., Sidhu, H. S., Krishna, V. V., & Shyamsundar, P. (2021). Changing agricultural stubble burning practices in the Indo-Gangetic plains: Is the happy seeder a profitable alternative? International Journal of Agricultural Sustainability, 19(2), 128–151. https://doi.org/10.1080/14735903.2020.1834277
- Kiefer, N. M. (1988). Economic duration data and hazard functions. J. Econ. Lit, 26(2), 646–679.
- Kleinbaum, D. G., & Klein, M. (2005). Survival analysis: A self-learning text (2nd ed). Statistics for Biology and Health. Springer Science + Business Media, Inc. https://doi.org/10.2307/2965741
- Knowler, D., & Bradshaw, B. (2007). Farmers’ adoption of conservation agriculture: A review and synthesis of recent research. Food Policy, 32(1), 25–48. https://doi.org/10.1016/j.foodpol.2006.01.003
- Kuhfuss, L., Préget, R., Thoyer, S., & Hanley, N. (2016). Nudging farmers to enrol land into agri-environmental schemes: The role of a collective bonus. European Review of Agricultural Economics, 43(4), 609–636. https://doi.org/10.1093/erae/jbv031
- Kumar, A., Prasad, K., Kushwaha, R. R., Rajput, M. M., & Sachan, B. S. (2010). Determinants influencing the acceptance of resource conservation technology : case of zero-tillage in rice-wheat farming systems in Uttar Pradesh, Bihar and Haryana states. Indian Journal of Agricultural Economics, 65(3), 448–460. https://doi.org/10.22004/ag.econ.204696
- Kumar, P., Kumar, S., & Joshi, L. (2015). Socioeconomimc and environmental implications of agricultural residue burning: A case study of Punjab, India. Springer Open.
- Kurinji, L. S., & Kumar, S. (2021). Is ex-situ crop residue management a scalable solution to stubble burning ? A punjab case study. Council on Energy, Environment and Water.
- Kurinji, L. S., & Prakash, S. (2021). Why paddy stubble continues to be burnt in Punjab ? Meeting challenges with solutions. Council on Energy, Environment and Water.
- Lapple, D. (2010). Adoption and abandonment of organic farming: An empirical investigation of the Irish drystock sector. Journal of Agricultural Economics, 61(3), 697–714. https://doi.org/10.1111/j.1477-9552.2010.00260.x
- Lapple, D., & Kelley, H. (2015). Spatial dependence in the adoption of organic drystock farming in Ireland. European Review of Agricultural Economics, 42(2), 315–337. https://doi.org/10.1093/erae/jbu024
- Li, R., Chen, W., Zhao, H., Wu, X., Zhang, M., Tong, D. Q., & Xiu, A. (2020). Inventory of atmospheric pollutant emissions from burning of crop residues in China based on satellite-retrieved farmland data. Chinese Geographical Science, 30(2), 266–278. https://doi.org/10.1007/s11769-020-1110-7
- Lin, M., & Begho, T. (2022). Crop residue burning in South Asia: A review of the scale, effect, and solutions with a focus on reducing reactive nitrogen losses. Journal of Environmental Management, 314, 115104. https://doi.org/10.1016/j.jenvman.2022.115104
- Lohan, S. K., Jat, H. S., Yadav, A. K., Sidhu, H. S., Jat, M. L., Choudhary, M., Peter, J. K., & Sharma, P. C. (2018). Burning issues of paddy residue management in north-west states of India. Renewable and Sustainable Energy Reviews, 81, 693–706. https://doi.org/10.1016/j.rser.2017.08.057
- Lopes, A. A., Tasneem, D., & Viriyavipart, A. (2023). Nudges and compensation: Evaluating experimental evidence on controlling rice straw burning. Ecological Economics, 204, 107677. https://doi.org/10.1016/j.ecolecon.2022.107677
- Lopes, A. A., Viriyavipart, A., & Tasneem, D. (2020). The role of social influence in crop residue management: Evidence from northern India. Ecological Economics, 169, 106563. https://doi.org/10.1016/j.ecolecon.2019.106563
- Lunduka, R., Fisher, M., & Snapp, S. (2012). Could farmer interest in a diversity of seed attributes explain adoption plateaus for modern maize varieties in Malawi? Food Policy, 37(5), 504–510. https://doi.org/10.1016/j.foodpol.2012.05.001
- Murage, A. W., Obare, G., Chianu, J., Amudavi, D. M., Pickett, J., & Khan, Z. R. (2011). Duration analysis of technology adoption effects of dissemination pathways: A case of “push-pull” technology for control of Striga weeds and stemborers in Western Kenya. Crop Protection, 30(5), 531–538. https://doi.org/10.1016/j.cropro.2010.11.009
- Mutsvangwa-Sammie, E. P., Manzungu, E., & Siziba, S. (2018). Key attributes of agricultural innovations in semi-arid smallholder farming systems in south-west Zimbabwe. Physics and Chemistry of the Earth, Parts A/B/C, 105, 125–135. https://doi.org/10.1016/j.pce.2018.03.001
- NAAS. (2017). Innovative viable solution to rice residue burning in rice-wheat cropping system through concurrent use of super straw management system-fitted combines and turbo happy seeder. Policy Brief No. 2. New Delhi.
- Ntshangase, N. L., Muroyiwa, B., & Sibanda, M. (2018). Farmers’ perceptions and factors influencing the adoption of no-till conservation agriculture by small-scale farmers in Zashuke, KwaZulu-Natal Province. Sustainability, 10(2), 555. https://doi.org/10.3390/su10020555
- PAU. (2021). Package of practices for crops of Punjab: Rabi. Punjab Agricultural University.
- Peshin, R. (2013). Farmers’ adoptability of integrated pest management of cotton revealed by a new methodology. Agronomy for Sustainable Development, 33(3), 563–572. https://doi.org/10.1007/s13593-012-0127-4
- PIB. (2021). Agricultural mechanization for in-situ management of crop residue [WWW Document]. Press Inf. Bur. Gov. India. Retrieved October 31, 2021, from https://pib.gov.in/Pressreleaseshare.aspx?PRID = 1707021.
- Pillai, S. (2021). Experts fear last-minute spike in farm fires this year. Hindustan Times.
- Porichha, G. K., Hu, Y., Rao, K. T. V., & Xu, C. C. (2021). Crop residue management in India: Stubble burning vs. Other utilizations including bioenergy. Energies, 14(14), 4281–4217. https://doi.org/10.3390/en14144281
- PRSC. (2021). Crop residue burning information and management system [WWW Document]. http://202.164.39.166/residue/Year_Wise.aspx
- PTI. (2021). IARI data shows spike in farm fires post monsoon in Punjab and Haryana – The Hindu. The Hindu.
- Ravindra, K., Singh, T., & Mor, S. (2019). Emissions of air pollutants from primary crop residue burning in India and their mitigation strategies for cleaner emissions. Journal of Cleaner Production, 208, 261–273. https://doi.org/10.1016/j.jclepro.2018.10.031
- Reddy, A.A. (2020). How can we solve the problem of stubble burning? [WWW Document]. Wire. Retrieved October 31, 2021, from https://science.thewire.in/environment/stubble-burning-punjab-haryana-rice-harvesting-wheat-sowing-delhi-air-pollution/.
- Reimer, A. P., Weinkauf, D. K., & Prokopy, L. S. (2012). The influence of perceptions of practice characteristics: An examination of agricultural best management practice adoption in two Indiana watersheds. Journal of Rural Studies, 28(1), 118–128. https://doi.org/10.1016/j.jrurstud.2011.09.005
- Rogers, E. M. (2003). Diffusion of innovations (5th ed). Free Press, Simon & Schuster, Inc.
- Rogers, E. M., & Shoemaker, F. F. (1971). Communication of innovations: A cross-cultural approach (2nd ed). Free Press.
- Roy, P. (2015). A comparative study on paddy straw management in Punjab and West Bengal. Punjab Agricultural University.
- Roy, P., Kaur, M., Burman, R. R., Sharma, J. P., & Roy, T. N. (2018). Determinants of paddy straw management decision of farmers in Punjab. Journal of Community Mobilization Sustainable Development, 13(2), 203–210.
- Saunders, C., Davis, L., & Pearce, D. (2012). Rice–wheat cropping systems in India and Australia, and development of the ‘happy seeder. ACIAR Impact Assessment Series Report, 77, 24–28. https://www.aciar.gov.au/sites/default/files/legacy/node/14496/ias077_rice_wheat_cropping_systems_in_india_and_12036.pdf
- Shyamsundar, P., Springer, N.P., Tallis, H., Polasky, S., Jat, M.L., Sidhu, H.S., Krishnapriya, P.P., Skiba, N., Ginn, W., Ahuja, V., Cummins, J., Datta, I., Dholakia, H.H., Dixon, J., Gerard, B., Gupta, R., Hellmann, J., Jadhav, A., Jat, H.S., … , Somanathan, R., 2019. Fields on fire: Alternatives to crop residue burning in India. Science, 365(80), 536–538. https://doi.org/10.1126/science.aaw4085
- Sidhu, H. S., Singh, M., Humphreys, E., Singh, Y., Singh, B., Dhillon, S. S., Blackwell, J., Bector, V., Singh, M., & Singh, S. (2007). The happy seeder enables direct drilling of wheat into rice stubble. Australian Journal of Experimental Agriculture, 47(7), 844–854. https://doi.org/10.1071/EA06225
- Sidibe, A. (2005). Farm-level adoption of soil and water conservation techniques in northern Burkina Faso. Agricultural Water Management, 71(3), 211–224. https://doi.org/10.1016/j.agwat.2004.09.002
- Singh, R. (1972). A behavioral contingency theory of adoption and diffusion of agricultural technology in less developed countries. University of Wisconsin.
- Singh, R. P., Dhaliwal, H. S., Humphreys, E., Singh, M., Singh, Y., & Blackwell, J. (2008). Economic assessment of the Happy Seeder for rice-wheat systems in Punjab, India. AARES 52th Annual Conference. Canberra, Australia.
- Supe, S. V., & Singh, S. N. (1976). Dynamics of rational behaviour of Indian farmers. New Heights Publisher.
- Turiano, N. A. (2016). Survival analysis. The Encyclopedia of Adulthood and Aging, 1–5. https://doi.org/10.1002/9781118528921.wbeaa148
- Venkatramanan, V., Shah, S., Rai, A. K., & Prasad, R. (2021). Nexus between crop residue burning, bioeconomy and sustainable development goals over north-western India. Frontiers in Energy Research, 8, 614212. https://doi.org/10.3389/fenrg.2020.614212