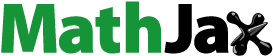
ABSTRACT
Agro-food quality certification has been gaining greater importance, because it contributes to both agricultural sustainability and food quality. However, small-scale farmers in developing countries have difficulties in obtaining certification and the economic benefits acquired from certification are unclear. This paper investigates the effects of agro-food quality certification on the farm performance which is indicated by profit, yield and price. The impacts of agricultural organizations, farmer cooperatives and agricultural companies in particular, on the adoption of certification by farmers are explored. We also investigate the effects of organizations on the farm performance of certified farmers. A dataset of 410 vegetable farmers in China is applied, and the propensity score matching (PSM) method is employed in the empirical analysis to address selection bias and reverse causality. Our study finds that, overall, the adoption of certification does not significantly influence farm performance. Cooperative membership positively influences the adoption of certification and the farm performance of certified farmers, while contract farming does not generate additional benefits for certified farmers.
1. Introduction
The intensive use of chemical fertilizers and pesticides in agriculture causes severe negative impacts on environmental sustainability, which eventually harms human health (Andersson & Isgren, Citation2021; Chatzimichael et al., Citation2022; Pretty, Citation2018). Moreover, as people’s income and awareness of environmental protection and healthy diets are growing, consumer demand for environmentally sound, safe, and high-quality foods is correspondingly increasing (Ding & Veeman, Citation2019; Narrod et al., Citation2009; Yin et al., Citation2019; Zhou et al., Citation2022). In this context, quality certification for agro-food has gained greater importance and has been promoted worldwide. It not only increases product quality (Handschuch et al., Citation2013; Jena et al., Citation2012), but also contributes to environmentally sustainable development. The latter is reflected in various environmentally friendly farming practices, e.g. the adoption of organic fertilizers (Blackman & Naranjo, Citation2012), water conservation (Haggar et al., Citation2017), integrated pest management (Meemken, Citation2021), and the improvement of eco-efficiency (Godoy-Durán et al., Citation2017), carbon stocks (Haggar et al., Citation2017), and alleviating forest degradation (Takahashi & Todo, Citation2017), etc.
Despite the importance of agro-food quality certification, the economic benefits that farmers acquire from certification are unclear. A few studies find that the adoption of certification increases farmers’ benefits because of higher prices or higher yields of certified products (e.g. Barham & Weber, Citation2012; Brako et al., Citation2021; Jouzi et al., Citation2017; Liu et al., Citation2020; Tran & Goto, Citation2019). However, poor performance of certification adoption is also reported, especially for organic certified production (e.g. Akoyi & Maertens, Citation2018; Arhin et al., Citation2022; Gather & Wollni, Citation2022; Ibanez & Blackman, Citation2016; Jena et al., Citation2017).
It is challenging for farmers to obtain certification because they have limited financial, technical, human, physical capital, and access to a high-end market (Asfaw et al., Citation2009, Citation2010; Guo and Zhang, Citation2013). The return on investment in agro-food quality certification is a long-term process and may be insignificant, which also disincentivizes farmers from adopting certifications (Sanders & Xiao, Citation2010; Snider et al., Citation2017). The situation in which farmers have difficulties or lack incentives to adopt agro-food quality certification is especially evident in developing countries, and China is no exception. Small-scale farmers are still dominating in agricultural production in China. There are 230 million famer households in China and approximately 90% of them have less than 10 mu of arable farm land.Footnote1
Both empirical observations and academic studies show that agricultural organizations, i.e. farmer cooperatives and agricultural companies, play an important role in the adoption of certification by farmers. This is reflected in two ways. First, organizations provide support for farmers to adopt agro-food quality certification (Asfaw et al., Citation2010; Handschuch et al., Citation2013; Oelofse et al., Citation2010). Second, agricultural organizations can help farmers to gain economic benefits from certification (Jena et al., Citation2012; Ssebunya et al., Citation2019). However, very limited quantitative research has been conducted to systematically investigate the role of organizations in the farm performance of certified farmers.
This study investigates the effect of quality certification on farm performance and the role of agricultural organizations in agro-food quality certification in terms of the adoption of certification and the farm performance of certified farmers. Specifically, we focus on three main types of certifications in China, i.e. the certification of pollution-free agricultural products (unique to China), green food certification (unique to China) and organic product certification. We focus on vegetable industry because vegetable production is featured by the intensive use of agrochemicals such as fertilizers and pesticides and, more importantly, certification adoption is more common in the vegetable industry (Li & Lu, Citation2020).
The following research questions were addressed. First, does vegetable quality certification affect farm performance, i.e. profit, yield, and price? Second, does farmers’ membership of farmer cooperatives or participation in contract farming increase the adoption of vegetable quality certification? Third, how do cooperative membership and contract farming influence the farm performance of certified farmers?
The remainder of this paper is organized as follows. Section 2 summarizes the literature and the contribution of the current study. Section 3 introduces the methodology of the study, including the data, empirical models, and descriptive analysis of variables. Section 4 reports the results and Section 5 is discussions for the results. We conclude in Section 6.
2. Literature review and marginal contributions
2.1. Adoption of agro-food quality certification by farmers
Many studies examine the factors that influence farmers’ adoption of quality certification. The main influencing factors are farmers’ demographic, psycho-behavioural and psychosocial characteristics (e.g. gender, age, education, year of operation, positive attitude, normative and moral obligations, risk preferences), household features (e.g. household size, labour size, farm size, access to non-farm income), production management features (e.g. supply chain coordination, land circulation) and external environment (e.g. market distance, available services, region) (Handschuch et al., Citation2013; Jena et al., Citation2012; Jena & Grote, Citation2017; Li & Guo, Citation2019; Li & Lu, Citation2020; Mohan, Citation2020; Sapbamrer & Thammachai, Citation2021; Tran & Goto, Citation2019; Van Ho et al., Citation2019).
The results reported by previous studies regarding the effects of these factors on farmers’ certification decisions are mixed. A few studies find that young farmers, farmers with high education levels or those with more experience in production are more likely to adopt certification (e.g. Handschuch et al., Citation2013; Jena et al., Citation2012; Sapbamrer & Thammachai, Citation2021). Van Ho et al. (Citation2019) and Li and Lu (Citation2020), however, find that experience in production negatively influences farmers’ adoption of certification. Jena and Grote (Citation2017) find that farmers’ education levels have a U relationship with their certification decisions. Household size, farm size and distance to the market are found to have positive effects on farmers’ certification adoption (Handschuch et al., Citation2013; Li & Lu, Citation2020; Van Ho et al., Citation2019).
The mechanism of certification adoption is also discussed by some scholars. Cui et al. (Citation2021) emphasize that social services help to address the household resource endowment constraints and extend product outlets, which therefore promote the adoption of quality certification by farmers. Wu et al. (Citation2022) find that agricultural informatization promotes the adoption of certification by family farms through releasing technical constraints and extending product outlets.
The results regarding the effect of agro-food quality certification on the economic performance are mixed. Most studies find that the adoption of quality certification has positive influences on farm output or farmers’ economic welfare (e.g. Bacon, Citation2005; Barham & Weber, Citation2012; Brako et al., Citation2021; Handschuch et al., Citation2013; Jena & Grote, Citation2017; Jouzi et al., Citation2017; Liu et al., Citation2020; Qiao et al., Citation2016; Tran & Goto, Citation2019; Wang et al., Citation2021). For example, the research by Qiao et al. (Citation2016) and Tran and Goto (Citation2019), based on tea farmers, report that quality certification positively affects product prices and sale income or profits. Barham and Weber (Citation2012) and Brako et al. (Citation2021) find increased yields and income resulted from certification adoption.
Some studies, alternatively, argue that quality certification generates no or little economic benefit for farmers (Akoyi & Maertens, Citation2018; Gather & Wollni, Citation2022; Ibanez & Blackman, Citation2016; Jena et al., Citation2012; Jena et al., Citation2017; Ruben & Fort, Citation2012; Weber, Citation2011). The production of certified products allows only limited or zero use of agrochemicals to meet environmental sustainability and product high-quality requirements. Therefore, certified products tend to have lower yields and sequentially cause profit loss (Ibanez & Blackman, Citation2016; Oya et al., Citation2018; Ruben & Fort, Citation2012).
The effects of agro-food quality certification on social and environmental outcomes have received much attention as well. These are reflected in farmer education, employment opportunities, community development, sustainable development of agriculture, and environmental sustainability (Blackman & Naranjo, Citation2012; Haggar et al., Citation2017; Jouzi et al., Citation2017; Liu et al., Citation2020; Meemken, Citation2021; Qiao et al., Citation2016; Takahashi & Todo, Citation2017).
2.2. The role of agricultural organizations in farmers’ agro-food quality certification
There is a rich body of literature about the effects of agricultural organizations, either farmer groups or contracting farming with companies, on farmers’ adoption of quality certification (Asfaw et al., Citation2010; Handschuch et al., Citation2013; Liang et al., Citation2021). Organizations facilitate the adoption of certification by farmers in multiple ways. First, agricultural organizations help farmers save the costs of certification through collective action (Asfaw et al., Citation2010; Handschuch et al., Citation2013). Second, organizations provide farmers with technical assistance and relevant training to help them meet certification requirements (Asfaw et al., Citation2010; Oelofse et al., Citation2010; Sanders & Xiao, Citation2010). Third, organizations can provide market information and a guaranteed market at good prices for farmers’ certified products (Oelofse et al., Citation2010; Sanders & Xiao, Citation2010; Wollni & Zeller, Citation2007).
The role of agricultural organizations in influencing farmers’ benefits obtained from certification is mentioned by a few scholars (Jena et al., Citation2012; Snider et al., Citation2017; Ssebunya et al., Citation2019). Jena et al. (Citation2012) find that cooperatives with good production and organizational capacities can help farmers gain the benefits from certification. Ssebunya et al. (Citation2019) report that the duration of farmer group membership, as a mediator, contributes to the effects of certification on farm incomes. However, quantitative studies on the role of organizations in the farm performance of certified farmers are hardly seen.
2.3. Contribution of the current study
This study makes three marginal contributions to the literature. First, it adds to the literature by estimating the effect of quality certification on farm performance in vegetable industry. Most current studies on the welfare effects of agro-food quality certification focus on coffee industry and small-scale farmers in the context of major coffee production countries in Latin America and Africa (e.g. Akoyi & Maertens, Citation2018; Dietz et al., Citation2020; Dragusanu et al., Citation2022; Gather & Wollni, Citation2022; Ibanez & Blackman, Citation2016; Jena et al., Citation2017; Jena & Grote, Citation2022; Ssebunya et al., Citation2019; Vellema et al., Citation2015), while the vegetable industry is paid little attention. Our empirical results show that vegetable quality certification has a negative, but not statistically significant, impact on farm performance. This may be associated with the characteristics of the farmers. Detailed discussions are provided in Section 5.
Second, this research extensively explores the roles of farmer cooperatives and agricultural companies in promoting the adoption of agro-food quality certification and gaining the benefits of certification by farmers. Although many studies report that organizations positively influence farmers’ adoption of certification (e.g. Asfaw et al., Citation2010; Handschuch et al., Citation2013; Liang et al., Citation2021), the effects of organizations on the economic benefits of certified farmers have hardly been analysed. We find that farmers’ participation in cooperatives has a positive impact on the profits and yields of certified vegetable farmers, whereas contract farming with a company does not significantly influence the farm performance of certified farmers.
Third, methodologically, this study distinguishes the effect of certification and that of agricultural organizations on the farm performance by using propensity score matching (PSM) methods. The results of most previous studies for the effects of certification on farmers’ economic benefits may be biased because they are conflated with the organization’s effects on farmers’ economic benefits (Sellare et al., Citation2020). We address the potential biased results problem by multiple estimations based on PSM.
3. Materials and methods
This section introduces the sampling strategy and data collection, establishes the empirical models, and provides a descriptive analysis of the variables.
3.1. Data
The data used in this study were collected through a survey of vegetable farmers in Hebei and Zhejiang provinces in China from June to August 2015. Stratified random sampling method was used to choose the sample. First, two provinces, Hebei and Zhejiang, were selected. Hebei is one of the largest vegetable production provinces in China. The yield of vegetables in Hebei province in 2014 was 81.26 million tons, accounting for 10.7% of the total vegetable output in China and ranked second among all the provinces.Footnote2 Comparatively, Zhejiang Province is characterized by a large population, shortage of land, limited natural resources, and an advanced economy. It mainly concentrates on the production of high-value farm products such as fruits and vegetables (Liang & Hendrikse, Citation2013).
Second, 13 main vegetable production counties in these two provinces were selected based on vegetable production intensity. Third, 20 vegetable cooperatives and 10 vegetable companies were randomly selected from each province within the 13 counties.Footnote3 Fourth, three members of a cooperative (or three farmers contracted with a company) and four individual farmers from the village where the cooperative (company) was located were randomly selected, resulting in a total of 420 farmers.
Face-to-face interviews were conducted with sampled farmers based on a structured questionnaire. Information on farm performance (e.g. the profit and yield), vegetable production, and demographic characteristics of farmers were collected. After the exclusion of 10 invalid questionnaires because of the lack of key information or inconsistent answers by the same respondent, a dataset comprising 40 cooperatives, 20 companies, and 410 farmers was established.
3.2. Model
3.2.1. Impact of agro-food quality certification on farm performance
The effect of vegetable quality certification on farm performance is estimated using the following model:
(1)
(1) where
represents farm performance of farmer
, i.e. profit, yield, and price;
represents vegetable quality certification status of farmer
, indicated by a dummy variable;
is a vector of covariates associated with farmers’ demographic features, household features, production management characteristics, and the external environment;
,
and
are parameters to be estimated;
is the error term.
Estimating the regression model directly may lead to biased results due to endogeneity problems, because farmers’ adoption of certification is a self-selection process (Asfaw et al., Citation2009). Specifically, farmers’ certification decisions may be influenced by a set of factors that simultaneously affect farm performance. It is possible that farmers with larger farm sizes have a higher tendency to adopt certification and earn higher profits in production. In this situation, the effects of certification on farm performance are confounded by the effects of observed covariates (Blackman & Naranjo, Citation2012). There is also the issue of reverse causality between farm performance and farmers’ adoption of certification, due to farmers’ limitations of financial and technical capital which are generally required by certification (Asfaw et al., Citation2009; Guo & Zhang, Citation2013). Therefore,, which represents the magnitude of the effect of certification on farm performance, would be biased if we conducted a multiple regression analysis.
To address the endogeneity problems, we estimate the model using PSM method, which is commonly used to minimize selection bias and reverse causality (e.g. Brako et al., Citation2021; Ji et al., Citation2019; Li & Lu, Citation2020; Liu et al., Citation2020; Tran & Goto, Citation2019; Verhofstadt & Maertens, Citation2014). Two groups of samples are distinguished by the matching. The treated group refers to farmers with certification, while the control group is farmers who do not adopt certification. PSM matches observations that are from treated group and control group respectively based on propensity scores which summarize the observable characteristics of the units (Rosenbaum & Rubin, Citation1983). The farm performance between the treated observations and their constructed counterfactuals using the matched control units are then compared. Specifically, it involves two steps. First, the propensity score is calculated by the following equation:
(2)
(2) where
denotes the propensity score of farmer
predicted by the probit model, i.e. the probability of farmer’s certification adoption conditional on a series of covariates;
represents a farmer’s quality certification adoption (i.e. treatment variable),
represents that the farmer adopted certification;
is a vector of observed covariates that influence a farmer’s decision to adopt certification.
Second, we calculate the average treatment effects on the treated group (ATT), that is, the average of the differences in farm performance between the treated units and their constructed counterfactuals. The equation is as follows:
(3)
(3) where
and
represent the farm performance of farmers who adopted certification and those who did not.
To obtain robust results, three methods are applied to match the treated and control groups. Nearest-neighbour matching (k = 4) requires that each unit in the treated group is matched to four units in the control group with the closest propensity scores, and the counterfactual outcome is the weighted average of the outcomes of these four units. Kernel matching constructs a counterfactual outcome using the weighted average of the outcomes of all units in the control group. Radius matching specifies a caliper, that is, the maximum distance between the propensity scores of the units in the treated and control groups. Those with absolute values of propensity score differences within the given caliper can be matched, and the counterfactual outcome is the weighted average of the outcomes of the matched control units. The caliper used in our study was set at 0.05.
3.2.2. The role of agricultural organizations in agro-food quality certification
We then examine the role of organizations, i.e. cooperatives and companies, in the adoption of certification by farmers. The following model is established:
(4)
(4) where
represents the organization participation of farmer
, i.e. individual farmer, cooperative membership or contract farming;
is a vector of covariates;
,
and
are parameters to be estimated;
is the error term.
To further investigate whether cooperative membership or participation in contract farming with companies influences the farm performance of certified farmers, we identify the sub-sample of all certified farmers. This sub-sample is divided into three groups: certified farmers with cooperative membership, certified farmers involved in contract farming, and individual certified farmers. The model for estimating the effect of participation in organizations on the farm performance of certified farmers is formulated as follows:
(5)
(5) where
is the farm performance of certified farmers;
,
and
are parameters to be estimated;
is the error term. PSM method is used again to estimate Equations Equation(4)
(4)
(4) and Equation(5)
(5)
(5) , in order to address the endogeneity problems caused by the self-selection problem and the reverse causality problem.
The relationships between quality certification, organizations, and farm performance, which are specified in the above models, are depicted in .
3.3. Variables and descriptive statistics
The definitions and descriptive statistics for each variable are provided in . Farm performance is indicated by profit, yield, and price per mu. The price here is a comprehensive indicator of product quality, market behaviours, and organization involvement. Farmers in the sample grow different vegetable varieties, are heterogeneous in marketing capabilities and other market behaviours, and are involved in alternative organizations. The mean values of the three variables were 5364.36 yuan, 5007.85 kg and 2.43 yuan/kg, respectively.
Table 1. Definitions and descriptive statistics of variables.
Agro-food quality certification is indicated by a binary variable, which refers to the adoption of pollution-free agricultural products, green food certification, and/or organic product certification.Footnote4 It takes the value of 1 if farmers adopt any one or more of the three certifications, and 0 otherwise. We do not distinguish between different types of certifications because the adoption rate of each type of certification is low. Specifically, only about 29% of the surveyed farmers adopted certification, while 71% did not. The distinction between the three types of certification would make the sample size of farmers with each type of certification too small. Among the farmers with certification, nearly 80% adopted pollution-free certification, while about 22% and 7% adopted green food certification and organic product certification, respectively.
Cooperative membership (contract farming) is a binary variable. It takes the value of 1 if a farmer is a member of a cooperative (participates in contract farming with a company), and 0 if a farmer is an individual farmer. Among the surveyed farmers, 118 farmers are cooperative members, and 42 farmers are engaged in contracting farming, accounting for 28.78% and 10.24% of all the sampled farmers respectively. The proportions of certified farmers among cooperative members and contracting farmers are 53% and 43%, respectively, while there are only about 15% of certified farmers among individual farmers.
Various potentially relevant covariates are included in the analyses, such as the farmers’ age, education, and production years. A few points are worth noting. Vegetable land area refers to the cultivated vegetable land area three years ago rather than last year, to avoid the possibility that the cultivated vegetable land area in the current year is endogenous from farmers’ choices of participation in organizations (Li & Guo, Citation2017). The mean value of the natural log of vegetable land area is approximately 1.26 mu, which reflects the small scale of farms. We also control for the category of vegetables.
4. Results
The results of empirical analyses are reported in this section.
4.1. Impact of agro-food quality certification on farm performance
Before presenting the ATT for the effects of certification on farm performance, the first stage of probit model is estimated. Three matching methods, i.e. nearest neighbour matching (k = 4), kernel matching, and radius matching, are used. The results are basically same for all the three methods; therefore, only the results for the nearest neighbour matching are reported since they are representative for all three methods. The results are displayed in . Both cooperative membership and contract farming are positively associated with farmers’ adoption of certification.
Table 2. The first stage of PSM estimation for the effects of certification on farm performance.
The quality of the matching is checked using common support and balancing tests. The result of the common support test is displayed in , and good-quality matching is confirmed. The distribution of predicted propensity scores for the treated and control groups overlaps, showing a very large common support for propensity scores between the treated and control groups. In particular, nearly all observations in the treated group are matched with those in the control group, except for one observation that is off support.
The results of the balancing test are shown in . Covariates are balanced between farmers who adopt certifications and those who do not after matching. Specifically, the biases of most covariates are sharply reduced, and their absolute values are less than 10% after matching. Meanwhile, the mean differences in almost all covariates between the treated and control groups are statistically insignificant after matching. Overall, we can conclude that the matching process is of high-quality.
Table 3. Balancing test for Model (1).
Next, the ATT for the impact of certification on farm performance is calculated, and the results are shown in . The adoption of certification has a negative, but not statistically significant, impact on farmers’ profits, vegetable yield, and prices.
Table 4. PSM results for the effects of certification on farm performance.
4.2. The role of agricultural organizations in the adoption of certification
Considering potential endogeneity problems, we use the PSM method to estimate the effects of farmers’ participation in cooperative or contract farming on their adoption of certification. The results of the first stage of PSM estimation, common support test, and balancing test for Model (2) applying nearest neighbour matching are displayed in , Figure A1, and respectively in the Appendix. The matching is of good quality.
The estimation results of ATT for the effects of cooperative membership and contract farming on farmers’ adoption of certification are reported in . The results indicate that both cooperative membership and contract farming significantly promote farmers’ adoption of certifications. Specifically, the probabilities of certification increase by approximately 34% for cooperative members and 30% for farmers who have contract farming with agricultural companies, respectively.
Table 5. PSM results for the effects of organizations on farmers’ adoption of certification.
Next, we go further to investigate whether cooperatives and companies play a role in helping farmers obtain benefits from certification and the PSM method is used to estimate the effects. To do that, we use a sub-sample of 117 certified farmers and divide them into three groups: 62 cooperative members, 18 farmers who have contract farming with companies, and 37 individual farmers. The results of the first stage of PSM estimation, common support test, and balancing test for Model (3) applying nearest neighbour matching are displayed in , Figure A2, and respectively in the Appendix. The matching is of good quality.
The estimated ATT for the effects of cooperative membership and contract farming on the farm performance of certified farmers are presented in . The results show that cooperative membership has a positive impact on the profit and yield per mu of certified farmers, yet does not exhibit a significant influence on the price. Specifically, certified farmers who are members of cooperatives obtain profits of around 3597.85–3883.69 Yuan per mu higher averagely than those of individual certified farmers. This gap for the yield is 2541.71–2685.87 kg per mu on average. However, certified farmers’ participation in contracting farming with companies does not significantly influence the profit, yield, or price.
Table 6. PSM results for the effects of organizations on farm performance of certified farmers.
4.3. Robustness of results to hidden bias
The key assumption of the PSM method is that the process of farmers’ selection into one treatment depends only on observable characteristics. Nonetheless, there may be unobservable characteristics, e.g. farmers’ management abilities, risk preferences, and awareness of environmental protection, that simultaneously affect farmers’ decisions and outcome variables. In this situation, a hidden bias might arise, which causes the association between a treatment and an outcome variable estimated based on observed factors may not be a causal effect (Aakvik, Citation2001). To check for the robustness of our estimation results, sensitivity analyses are conducted by calculating Rosenbaum bounds to test the sensitivity of the empirical results to hidden bias (Rosenbaum, Citation2002).
Two parts of the results need to be checked for the robustness. One is the results about the effects of participation in organizations on farmers’ adoption of certification. Considering that the outcome variable is certification adoption, which is a binary variable, we adopt the command developed by Becker and Caliendo (Citation2007) which applies to binary outcome variables and is suited for nearest neighbour matching without replacement. The other is the results regarding the effects of cooperative membership on farm performance of certified farmers. Given that the outcome variables, i.e. profit and yield, are continuous variables, the commonly used command developed by DiPrete and Gangl (Citation2004) is adopted, since it is mostly applicable to continuous-outcome variables and suited for the nearest neighbour matching with one neighbour.
The estimates are more robust if they are less sensitive to hidden biases. Gamma (≥1) represents the magnitude of the effect of unobservable factors on the farmers’ selection process, i.e. the magnitude of the hidden bias. The value of Γ equals 1 if these factors have no influence on farmers’ decisions; that is, there is no hidden bias. As Γ increases, the magnitude of the hidden bias increases. As Aakvik (Citation2001) indicates, if a large hidden bias does not alter our estimates of treatment effects, we can conclude that our results are less sensitive to hidden bias and are more reliable. Specifically, a larger Γ can be observed when the bounds become insignificant, that is, the estimated treatment effect is no longer significant. The range of Γ is typically set from 1 to 2 (e.g. Aakvik, Citation2001; Liu et al., Citation2020).
displays the sensitivity analysis results for the PSM estimates of the effect of organizations on the certification adoption and that of cooperative membership on the profits and yields of certified farmers. The estimated effect of cooperative membership on the adoption of certification is very robust because the estimate of ATT is still significant at 1% significance level when Γ = 2 for both bounds under the assumption of over- or under- estimation of the treatment effect. The estimated effect of contract farming on the certification adoption is less encouraging. The estimate of ATT is just not significant at 10% significance level when Γ = 1.6 for the bound under the assumption of overestimation of the treatment effect. Therefore, the effect of contract farming on the adoption of certification should be explained cautiously.
Table 7. Sensitivity analysis results for Model (2) and Model (3).
Since the effects of cooperative membership on farm performance of certified farmers are positive, only upper bounds are displayed. The estimated effect of cooperative membership on the profits of certified farmers is robust because the estimate of ATT is still significant at the 10% significance level when Γ = 1.9. Likewise, the estimated effect of cooperative membership on yields is also very robust because the estimate of ATT is still significant at the 5% significance level when Γ = 2. Overall, our results can stand to a large extent.
5. Discussion
The results regarding the effects of certification adoption on farm performance are consistent with some previous studies conducted in an international scenario (e.g. Gather & Wollni, Citation2022; Ibanez & Blackman, Citation2016; Ruben & Fort, Citation2012). These studies also report that certification for coffee does not bring farmers extra gains in production.
The main reason for the insignificant effect of certification on the yields may be that the gap in the production process and management practices between certified and non-certified farmers is small. The data show that among certified farmers, most farmers (almost 80%) adopt pollution-free certification, while only about 22% and 7% of farmers adopt green food certification and organic product certification, respectively. Since pollution-free certification has fewer limits on chemical use during production than green food or organic products do, the difference in yields between certified and non-certified farmers is small.
Theoretically, certified products are expected to obtain high selling prices because of the high quality, which is an important incentive for farmers to adopt the certification. Some studies report that certification positively affects the profits due to the price premium (e.g. Qiao et al., Citation2016; Tran & Goto, Citation2019). However, our results are inconsistent with these findings since no significant price premium is found for certified products. One reason for this may be that most farmers in China are smallholders and price-takers with weak bargaining power (Huang & Liang, Citation2018). They face challenges in obtaining premium prices for certified products. In addition, the increase in consumers’ willingness to pay for certified agro-food is still limited in China because of consumers’ insufficient knowledge and distrust of food quality standards (Scott et al., Citation2014).
Our results about the effects of cooperative membership on farm performance of certified farmers are consistent with most previous studies reporting that cooperative membership has a significantly positive impact on farmers’ profits and product yields (e.g. Abdul-Rahaman & Abdulai, Citation2018; Liang et al., Citation2021; Ma et al., Citation2022; Ma & Abdulai, Citation2016). Farmer cooperatives generally provide assistance with techniques for farmers to boost their yields (Barham et al., Citation2011). The results that contract farming does not significantly influence farm performance of certified farmers indirectly indicate that companies provide less technical training or support for farmers than cooperatives.
The effects of cooperative membership or contract farming on farm prices obtained by farmers vary in the literature. Some studies find a positive effect (e.g. Dubbert, Citation2019; Liang & Wang, Citation2020; Serra & Davidson, Citation2021; Mishra, Kumar et al., Citation2022), while others report no significant or negative impacts (e.g. Chagwiza et al., Citation2016; Mishra, Mayorga et al., Citation2022). Our results show that certified farmers with cooperative membership or involved in contract farming do not gain any extra price premium, compared with individual certified farmers. A possible reason is that most farmers in China are price takers, and the price premium, if there is any, is reaped by cooperatives or companies.
6. Conclusions
Agro-food quality certification has essential significance in agricultural sustainability and food quality. However, the adoption of certification faces challenges because small-scale farmers have difficulties in obtaining certification and the economic benefits acquired from certification are unclear. This study investigates the effects of agro-food quality certification on farm performance and further explores the role of agricultural organizations in farmers’ certification adoption and the farm performance of certified farmers. A dataset comprising 410 vegetable farmers from China is used and PSM estimation method is applied to address selection bias and reverse causality issues.
A few important findings are highlighted. First, the adoption of vegetable quality certification by vegetable farmers does not lead to significant improvements in farm performance, i.e. unit profit, unit yield, or price.
Second, agricultural organizations play an important role in farmers’ adoption of quality certifications. Cooperative membership and contract farming with companies increase farmers’ adoption of certification by approximately 34% and 30%, respectively, compared with individual farmers. However, the effect of contract farming on vegetable certification needs to be explained with caution because the estimated results are sensitive to unobservable factors and there might be overestimation of the treatment effect.
Third, cooperative membership positively influences the profit and yield per mu of certified farmers, while contract farming with agricultural companies does not have a significant impact on farm performance. Specifically, cooperative membership increases the profit of certified farmers by around 3597.85–3883.69 yuan per mu and the yield by 2541.71–2685.87 kg per mu. However, no significant effect on the price is found.
The results provide implications for farmers of all the product categories beyond vegetables and for farmers in other regions of the world as well. That is because the challenges of certification are quite similar for all the agro-food, i.e. farmers’ limitations of financial, technical, human, and/or physical capital, and the unclear economic benefits acquired from the certification. These findings can provide direction for policymakers.
First, efforts need to be made to increase the price premium of certified products by enhancing the public’s understanding of and trust in the agro-food quality certification. The importance of environmental friendly features and quality safety of products should be fully conveyed to consumers through multiple channels, e.g. by the government and the medium. Second, it is necessary for the government to provide support for the development of agricultural organizations to give full play to their role in the adoption of agro-food quality certification. For example, financial support for farmer cooperatives targeting agro-food quality certification can benefit the farmers involved and facilitate the overall development of quality certification.
There are a few limitations of our study. First, the heterogeneity of the effects across different types of certification on farm performance is not analysed due to data limits. Second, the outlets of products, e.g. the destinations of products and the types of clients, can also be important factors influencing the performance and product certification of farmers. However, they are not included in the model due to data limits. Third, the mechanisms how farmer cooperatives influence the farm performance of certified farmers can be explored in future research.
Disclosure statement
No potential conflict of interest was reported by the author(s).
Additional information
Funding
Notes
1 Data source: http://www.gov.cn/xinwen/2019-03/01/content_5369755.htm; 1 mu = 1/15 hectare.
2 Data source: China Rural Statistical Yearbook (2015), China Statistics Press.
3 The number of selected vegetable cooperatives is the double of that of vegetable companies, according to the relatively size of populations of the two types of organizations.
4 As of September 2019, the number of certificates issued by these three certifications were 68,835, 40,077 and 73,934, respectively. Data source: China Academy for Rural Development-Qiyan China Agribusiness Database (CCAD).
References
- Aakvik, A. (2001). Bounding a matching estimator: The case of a Norwegian training program. Oxford Bulletin of Economics and Statistics, 63(1), 115–143. https://doi.org/10.1111/1468-0084.00211
- Abdul-Rahaman, A., & Abdulai, A. (2018). Do farmer groups impact on farm yield and efficiency of smallholder farmers? Evidence from rice farmers in northern Ghana. Food Policy, 81, 95–105. https://doi.org/10.1016/j.foodpol.2018.10.007
- Akoyi, K. T., & Maertens, M. (2018). Walk the talk: Private sustainability standards in the Ugandan coffee sector. Journal of Development Studies, 54(10), 1792–1818. https://doi.org/10.1080/00220388.2017.1327663
- Andersson, E., & Isgren, E. (2021). Gambling in the garden: Pesticide use and risk exposure in Ugandan smallholder farming. Journal of Rural Studies, 82, 76–86. https://doi.org/10.1016/j.jrurstud.2021.01.013
- Arhin, I., Li, J., Mei, H., Amoah, M., Chen, X., Jeyaraj, A., Li, X., & Liu, A. (2022). Looking into the future of organic tea production and sustainable farming: A systematic review. International Journal of Agricultural Sustainability, 1–13. https://doi.org/10.1080/14735903.2022.2028398
- Asfaw, S., Mithöfer, D., & Waibel, H. (2009). EU food safety standards, pesticide use and farm-level productivity: The case of high-value crops in Kenya. Journal of Agricultural Economics, 60(3), 645–667. https://doi.org/10.1111/j.1477-9552.2009.00205.x
- Asfaw, S., Mithöfer, D., & Waibel, H. (2010). What impact are EU supermarket standards having on developing countries’ export of high-value horticultural products? Evidence from Kenya. Journal of International Food & Agribusiness Marketing, 22(3–4), 252–276. https://doi.org/10.1080/08974431003641398
- Bacon, C. (2005). Confronting the coffee crisis: Can fair trade, organic, and specialty coffees reduce small-scale farmer vulnerability in northern Nicaragua. World Development, 33(3), 497–511. https://doi.org/10.1016/j.worlddev.2004.10.002
- Barham, B. L., Gitter, S., Lewis, J., & Weber, J. (2011). Fair trade/organic coffee, rural livelihoods, and the ‘agrarian question’: Southern Mexican coffee families in transition. World Development, 39(1), 134–145. https://doi.org/10.1016/j.worlddev.2010.08.005
- Barham, B. L., & Weber, J. G. (2012). The economic sustainability of certified coffee: Recent evidence from Mexico and Peru. World Development, 40(6), 1269–1279. https://doi.org/10.1016/j.worlddev.2011.11.005
- Becker, S. O., & Caliendo, M. (2007). Sensitivity analysis for average treatment effects. Stata Journal, 7(1), 71–83. https://doi.org/10.1177/1536867X0700700104
- Blackman, A., & Naranjo, M. A. (2012). Does eco-certification have environmental benefits? Organic coffee in Costa Rica. Ecological Economics, 83, 58–66. https://doi.org/10.1016/j.ecolecon.2012.08.001
- Brako, D. E., Richard, A., & Alexandros, G. (2021). Do voluntary certification standards improve yields and wellbeing? Evidence from oil palm and cocoa smallholders in Ghana. International Journal of Agricultural Sustainability, 19(1), 16–39. https://doi.org/10.1080/14735903.2020.1807893
- Chagwiza, C., Muradian, R., & Ruben, R. (2016). Cooperative membership and dairy performance among smallholders in Ethiopia. Food Policy, 59, 165–173. https://doi.org/10.1016/j.foodpol.2016.01.008
- Chatzimichael, K., Genius, M., & Tzouvelekas, V. (2022). Pesticide use, health impairments and economic losses under rational farmers behavior. American Journal of Agricultural Economics, 104(2), 765–790. https://doi.org/10.1111/ajae.12244
- Cui, M., Wang, R., Guo, Y., & Zheng, F. (2021). Analysis on the influence of socialized services on farmers’ quality-safety certification behaviors. Research of Agricultural Modernization, 42(6), 1059–1070 (in Chinese). https://doi.org/10.13872/j.1000-0275.2021.0079
- Dietz, T., Chong, A. E., Grabs, J., & Kilian, B. (2020). How effective is multiple certification in improving the economic conditions of smallholder farmers? Evidence from an impact evaluation in Colombia’s coffee belt. Journal of Development Studies, 56(6), 1141–1160. https://doi.org/10.1080/00220388.2019.1632433
- Ding, Y., & Veeman, M. M. (2019). Chinese consumers’ preferences for quality signals on fresh milk: Brand versus certification. Agribusiness, 35(4), 593–609. https://doi.org/10.1002/agr.21604
- DiPrete, T. A., & Gangl, M. (2004). Assessing bias in the estimation of causal effects: Rosenbaum bounds on matching estimators and instrumental variables estimation with imperfect instruments. Sociological Methodology, 34(1), 271–310. https://doi.org/10.1111/j.0081-1750.2004.00154.x
- Dragusanu, R., Montero, E., & Nunn, N. (2022). The effects of fair trade certification: Evidence from coffee producers in Costa Rica. Journal of the European Economic Association, 20(4), 1743–1790. https://doi.org/10.1093/jeea/jvac026
- Dubbert, C. (2019). Participation in contract farming and farm performance: Insights from cashew farmers in Ghana. Agricultural Economics, 50(6), 749–763. https://doi.org/10.1111/agec.12522
- Gather, J., & Wollni, M. (2022). Setting the standard: Does rainforest alliance certification increase environmental and socio-economic outcomes for small-scale coffee producers in Rwanda? Applied Economic Perspectives and Policy, 44(4), 1807–1825. https://doi.org/10.1002/aepp.13307
- Godoy-Durán, A., Galdeano-Gómez, E. C., Pérez-Mesa, J., & Piedra-Muñoz, L. (2017). Assessing eco-efficiency and the determinants of horticultural family farming in southeast Spain. Journal of Environmental Management, 204, 594–604. https://doi.org/10.1016/j.jenvman.2017.09.037
- Guo, S., & Zhang, J. (2013). Analysis on farmers’ livelihood capital vulnerability. Economic Survey, (3), 26–30 (in Chinese). https://doi.org/10.15931/j.cnki.1006-1096.2013.03.012.
- Haggar, J., Soto, G., Casanoves, F., & Virginio, E. D. M. (2017). Environmental-economic benefits and trade-offs on sustainably certified coffee farms. Ecological Indicators, 79, 330–337. https://doi.org/10.1016/j.ecolind.2017.04.023
- Handschuch, C., Wollni, M., & Villalobos, P. (2013). Adoption of food safety and quality standards among Chilean raspberry producers – do smallholders benefit. Food Policy, 40, 64–73. https://doi.org/10.1016/j.foodpol.2013.02.002
- Huang, Z., & Liang, Q. (2018). Agricultural organizations and the role of farmer cooperatives in China since 1978: Past and future. China Agricultural Economic Review, 10(1), 48–64. https://doi.org/10.1108/CAER-10-2017-0189
- Ibanez, M., & Blackman, A. (2016). Is eco-certification a win–win for developing country agriculture? Organic coffee certification in Colombia. World Development, 82, 14–27. https://doi.org/10.1016/j.worlddev.2016.01.004
- Jena, P. R., Chichaibelu, B. B., Stellmacher, T., & Grote, U. (2012). The impact of coffee certification on small-scale producers’ livelihoods: A case study from the Jimma Zone, Ethiopia. Agricultural Economics, 43(4), 429–440. https://doi.org/10.1111/j.1574-0862.2012.00594.x
- Jena, P. R., & Grote, U. (2017). Fairtrade certification and livelihood impacts on small-scale coffee producers in a tribal community of India. Applied Economic Perspectives and Policy, 39(1), 87–110. https://doi.org/10.1093/aepp/ppw006
- Jena, P. R., & Grote, U. (2022). Do certification schemes enhance coffee yields and household income? Lessons learned across continents. Frontiers in Sustainable Food Systems, 5, Article 716904. https://doi.org/10.3389/fsufs.2021.716904
- Jena, P. R., Stellmacher, T., & Grote, U. (2017). Can coffee certification schemes increase incomes of smallholder farmers? Evidence from Jinotega, Nicaragua. Environment Development and Sustainability, 19(1), 45–66. https://doi.org/10.1007/s10668-015-9732-0
- Ji, C., Jin, S., Wang, H., & Ye, C. (2019). Estimating effects of cooperative membership on farmers’ safe production behaviors: Evidence from pig sector in China. Food Policy, 83, 231–245. https://doi.org/10.1016/j.foodpol.2019.01.007
- Jouzi, Z., Azadi, H., Taheri, F., Zarafshani, K., Gebrehiwot, K., Van Passel, S., & Lebailly, P. (2017). Organic farming and small-scale farmers: Main opportunities and challenges. Ecological Economics, 132, 144–154. https://doi.org/10.1016/j.ecolecon.2016.10.016
- Li, H., & Lu, Q. (2020). Can product quality certification improve farmers’ technological efficiency? Chinese Rural Economy, 425(5), 128–144.
- Li, L., & Guo, H. (2017). The impact of industrial organization models on farmers’ production income: The case of vegetable farmers in Hebei and Zhejiang provinces. Chinese Rural Economy, 393(9), 62–79.
- Li, L., & Guo, H. (2019). The impact of business relationships on safe production behavior by farmers: Evidence from China. Agribusiness, 35(3), 84–96. https://doi.org/10.1002/agr.21584
- Liang, Q., & Hendrikse, G. (2013). Core and common members in the genesis of farmer cooperatives in China. Managerial and Decision Economics, 34(3–5), 244–257. https://doi.org/10.1002/mde.2587
- Liang, Q., Li, L., & Bai, R. (2021). Welfare effects of vegetable producers’ inclusiveness in supply chain coordination: Direct effects and spillovers. British Food Journal, 123(4), 1305–1323. https://doi.org/10.1108/BFJ-07-2020-0652
- Liang, Q., & Wang, X. (2020). Cooperatives as competitive yardstick in the hog industry? Evidence from China. Agribusiness, 36(1), 127–145. https://doi.org/10.1002/agr.21630
- Liu, R., Gao, Z., Nayga, R. M., Shi, L., Oxley, L., & Ma, H. (2020). Can ‘green food’ certification achieve both sustainable practices and economic benefits in a transitional economy? The case of kiwifruit growers in Henan Province, China. Agribusiness, 36(4), 675–692. https://doi.org/10.1002/agr.21641
- Ma, W., & Abdulai, A. (2016). Does cooperative membership improve household welfare? Evidence from apple farmers in China. Food Policy, 58, 94–102. https://doi.org/10.1016/j.foodpol.2015.12.002
- Ma, W., Zheng, H., Zhu, Y., & Qi, J. (2022). Effects of cooperative membership on financial performance of banana farmers in China: A heterogeneous analysis. Annals of Public and Cooperative Economics, 93(1), 5–27. https://doi.org/10.1111/apce.12326
- Meemken, E. M. (2021). Large farms, large benefits? Sustainability certification among family farms and agro-industrial producers in Peru. World Development, 145, 1–12. https://doi.org/10.1016/j.worlddev.2021.105520
- Mishra, A. K., Kumar, A., Joshi, P. K., & Dsouza, A. (2022). Monopsonists, disruptive innovation and food security: The case of high-value commodity. Applied Economic Perspectives and Policy, 44(1), 460–476. https://doi.org/10.1002/aepp.13122
- Mishra, A. K., Mayorga, J., & Kumar, A. (2022). Technology and managerial gaps in contract farming: The case of specialty crop production. Journal of Agricultural and Resource Economics, 47(1), 77–96. https://doi.org/10.22004/ag.econ.307460
- Mohan, S. (2020). Risk aversion and certification: Evidence from the Nepali tea fields. World Development, 129, Article 104903. https://doi.org/10.1016/j.worlddev.2020.104903
- Narrod, C., Roy, D., Okello, J., Avendaño, B., Rich, K., & Thorat, A. (2009). Public–private partnerships and collective action in high value fruit and vegetable supply chains. Food Policy, 34(1), 8–15. https://doi.org/10.1016/j.foodpol.2008.10.005
- Oelofse, M., Høgh-Jensen, H., Abreu, L. S., Almeida, G. F., Hui, Q. Y., Sultan, T., & de Neergaard, A. (2010). Certified organic agriculture in China and Brazil: Market accessibility and outcomes following adoption. Ecological Economics, 69(9), 1785–1793. https://doi.org/10.1016/j.ecolecon.2010.04.016
- Oya, C., Schaefer, F., & Skalidou, D. (2018). The effectiveness of agricultural certification in developing countries: A systematic review. World Development, 112, 282–312. https://doi.org/10.1016/j.worlddev.2018.08.001
- Pretty, J. (2018). Intensification for redesigned and sustainable agricultural systems. Science, 362(6417), eaav0294. https://doi.org/10.1126/science.aav0294
- Qiao, Y., Halberg, N., Vaheesan, S., & Scott, S. (2016). Assessing the social and economic benefits of organic and fair trade tea production for small-scale farmers in Asia: A comparative case study of China and Sri Lanka. Renewable Agriculture and Food Systems, 31(3), 246–257. https://doi.org/10.1017/S1742170515000162
- Rosenbaum, P. R. (2002). Observational studies (2nd ed.). Springer-Verlag.
- Rosenbaum, P. R., & Rubin, D. B. (1983). The central role of the propensity score in observational studies for causal effects. Biometrika, 70(1), 41–55. https://doi.org/10.1093/biomet/70.1.41
- Ruben, R., & Fort, R. (2012). The impact of fair trade certification for coffee farmers in Peru. World Development, 40(3), 570–582. https://doi.org/10.1016/j.worlddev.2011.07.030
- Sanders, R., & Xiao, X. (2010). The sustainability of organic agriculture in developing countries: Lessons from China. The International Journal of Environment, Cultural, Economic and Social Sustainability, 6(6), 233–244. https://doi.org/10.18848/1832-2077/CGP/v06i06/54845
- Sapbamrer, R., & Thammachai, A. (2021). A systematic review of factors influencing farmers’ adoption of organic farming. Sustainability, 13(7), 3842. https://doi.org/10.3390/su13073842
- Scott, S., Si, Z., Schumilas, T., & Chen, A. (2014). Contradictions in state- and civil society-driven developments in China’s ecological agriculture sector. Food Policy, 45, 158–166. https://doi.org/10.1016/j.foodpol.2013.08.002
- Sellare, J., Meemken, E. M., Kouamé, C., & Qaim, M. (2020). Do sustainability standards benefit smallholder farmers also when accounting for cooperative effects? Evidence from Côte d’ivoire. American Journal of Agricultural Economics, 102(2), 681–695. https://doi.org/10.1111/ajae.12015
- Serra, R., & Davidson, K. A. (2021). Selling together: The benefits of cooperatives to women honey producers in Ethiopia. Journal of Agricultural Economics, 72(1), 202–223. https://doi.org/10.1111/1477-9552.12399
- Snider, A., Gutiérrez, I., Sibelet, N., & Faure, G. (2017). Small farmer cooperatives and voluntary coffee certifications: Rewarding progressive farmers of engendering widespread change in Costa Rica? Food Policy, 69, 231–242. https://doi.org/10.1016/j.foodpol.2017.04.009
- Ssebunya, B. R., Morawetz, U. B., Schader, C., Stolze, M., & Schmid, E. (2019). Group membership and certification effects on incomes of coffee farmers in Uganda. European Review of Agricultural Economics, 46(1), 109–132. https://doi.org/10.1093/erae/jby022
- Takahashi, R., & Todo, Y. (2017). Coffee certification and forest quality: Evidence from a wild coffee forest in Ethiopia. World Development, 92, 158–166. https://doi.org/10.1016/j.worlddev.2016.12.001
- Tran, D., & Goto, D. (2019). Impacts of sustainability certification on farm income: Evidence from small-scale specialty green tea farmers in Vietnam. Food Policy, 83, 70–82. https://doi.org/10.1016/j.foodpol.2018.11.006
- Van Ho, B., Nanseki, T., & Chomei, Y. (2019). Profit efficiency of tea farmers: Case study of safe and conventional farms in northern Vietnam. Environment, Development and Sustainability, 21(4), 1695–1713. https://doi.org/10.1007/s10668-017-0073-z
- Vellema, W., Casanova, A. B., Gonzalez, C., & D’ Haese, M. (2015). The effect of specialty coffee certification on household livelihood strategies and specialization. Food Policy, 57, 13–25. https://doi.org/10.1016/j.foodpol.2015.07.003
- Verhofstadt, E., & Maertens, M. (2014). Can agricultural cooperatives reduce poverty? Heterogeneous impact of cooperative membership on farmers’ welfare in Rwanda. Applied Economic Perspectives and Policy, 37(1), 86–106. https://doi.org/10.1093/aepp/ppu021
- Wang, R., Han, X., Cui, M., & Zheng, F. (2021). The income-increasing effect of adopting green technologies by farmers: From the perspective of quality economics. Research of Agricultural Modernization, 42(3), 462–473 (in Chinese). https://doi.org/10.13872/j.1000-0275.2021.0063
- Weber, J. G. (2011). How much more do growers receive for fair trade-organic coffee. Food Policy, 36(5), 678–685. https://doi.org/10.1016/j.foodpol.2011.05.007
- Wollni, M., & Zeller, M. (2007). Do farmers benefit from participating in specialty markets and cooperatives? The case of coffee marketing in Costa Rica. Agricultural Economics, 37(2–3), 243–248. https://doi.org/10.1111/j.1574-0862.2007.00270.x
- Wu, J., Han, D., & Ruan, R. (2022). Agriculture informatization and quality of agricultural products: Analysis on the behavior of quality certification of agricultural products based on family farms. Journal of Nanjing Agricultural University, 22(1), 172–184 (in Chinese). https://doi.org/10.19714/j.cnki.1671-7465.2022.0014
- Yin, S., Hu, W., Chen, Y., Han, F., Wang, Y., & Chen, M. (2019). Chinese consumer preferences for fresh produce: Interaction between food safety labels and brands. Agribusiness, 35(1), 53–68. https://doi.org/10.1002/agr.21585
- Zhou, Y., Wang, S., & Yan, F. (2022). The structure, evolution and prospect of food system in China. Issues in Agricultural Economy, 1, 100–113 (in Chinese). https://doi.org/10.13246/j.cnki.iae.2022.01.010
Appendix
Table A1. The first stage of PSM estimation for the effects of cooperative membership and contract farming on farmers’ adoption of certification.
Table A2. Balancing test for the effects of participation in specific organizations on farmers’ adoption of certification: nearest neighbour matching (k = 4).
Table A3. The first stage of PSM estimation for the effects of participation in specific organizations on farm performance indicators of certified farmers.
Table A4. Balancing test for the effects of participation in specific organizations on farm performance indicators of certified farmers: nearest neighbour matching (k = 4).