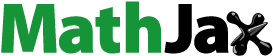
ABSTRACT
A large number of farms in developing countries are smallholder farms. In China, this trend is expected to persist for the foreseeable future. However, smallholder farmers in China face several challenges, including excessive fertilizer use for higher yields, low levels of education, aging and limited credit information. Moreover, they are struggling with a digital divide, which hampers their agricultural productivity and limits their economic and social integration. Agricultural services based on information and communication technology (ICT) can bridge the digital gap, but are hindered by complexity and cost. Aiming at serving the numerous smallholders and building a sustainable digital ecosystem, this paper presents a distributed agriculture service system that provides decision support to farmers by considering both social and physical information. The system is lightweight, low cost and intelligent, managing personnel, plots, crops and equipment using technologies such as wireless sensor networks, cloud computing and distributed systems architecture. Services include crop planning, production guidance, equipment control, etc. User terminals include computers, mobile applications and Applets. A case study on fruit production in a typical solar greenhouse illustrates the system's functionality. The distributed agricultural services system can well support smallholder farmers and promote sustainable agriculture.
1. Introduction
A large number of farms are located in developing countries (Pretty, Citation2018), and out of the 570 million farms worldwide, 84% are smallholder farms covering less than 2 hectares (Lowder et al., Citation2016). Smallholders face a crucial challenge of information imbalance between supply and demand. Conversely, modern agriculture is expected to be highly competitive, knowledge-intensive and market-driven (Mondal & Basu, Citation2009). Information asymmetry (between supply and demand) and lack of knowledge have led to soil pollution, unmarketable products and economic losses (Abokyi et al., Citation2020; Kang et al., Citation2018). Bridging small-scale farmers with the advancements in modern agriculture is expected to be a breakthrough, where a key action is to develop an agricultural service system that integrates information and intelligent technologies (Trendov et al., Citation2018). Nevertheless, most smallholder farmers have limited access to science-based agricultural advice, which poses a significant hurdle in obtaining up-to-date information for farmers (Naika et al., Citation2021).
In China, as of 2019, more than 98% of farms are still smallholder farms, with approximately 210 million households cultivating less than 0.67 hectares of land. It is expected that smallholder farms will continue to dominate in the long term, according to estimates derived from the average annual growth rate of rural land transferred area over the past decade (China Government Report in 2018). Being the primary source of food production in China, smallholder farmers often rely on excessive fertilizer usage to achieve high yields. However, this type of production negatively affected the country's food production and environmental sustainability (Zhang et al., Citation2020). In addition, smallholder farmers in China are generally aged and low educated, making it difficult for them to adaptquickly to market changes or knowledge updates. Until 2017, only 8.3% of farmers have high school education, and 33.6% are over the age of 55 (Third National Agricultural Census Data Report, Citation2017). Besides, farmers generally lack credit information, which makes it difficult for banks to assess their credit risk. The inadequate use of scientific and technological innovations has led to a decline in the quality of agricultural personnel, making it difficult to meet the current high-level needs of national agricultural development (Yu et al., Citation2020).
One-stop-shop online agriculture service system is expected to address the issues mentioned above, covering the whole production chain (Kieti et al., Citation2021). Services include those for pre-cultivation, cultivation and post-cultivation stages, such as environmental monitoring, crop planning and remote technical support (Naika et al., Citation2021). The Food and Agriculture Organization of the United Nations (FAO) has launched the 1000 Digital Village Initiative to promote digital innovations (www.fao.org) aiming to convert at least 1000 rural localities worldwide into digital village hubs, offering a wide range of ICT-based services. The concept of a digital village promotes digital innovations in rural communities, enabling economic livelihoods, individual well-being, and social cohesion. It is worthy to note that there is no singlular pathway or approach for a rural community to become 'Digital', as there are many ways to apply and leverage digital innovations and services. Worldwide, the widespread adoption of basic mobile phone technology provides an avenue to enhance in-person agricultural extension efforts that were previously costly and faced accountability challenges (Fabregas et al., Citation2019). Mobile phones can benefit farmers in low- and middle-income countries by improving access to agricultural advice on productivity and market price information in rapidly changing economic conditions. However, market failures still likely prevent efficient scaling of valuable digital advice applications (Fabregas et al., Citation2019). In China, disseminating knowledge about high-yield, high-efficiency practices to millions of smallholder farmers is crucial for achieving sustainable intensification and the goal of increased production with reduced environmental impacts (Jiao et al., Citation2019). Additionally, to address the challenge of unbalanced information between supply and demand, new agricultural businesses, such as contract farming, have emerged, which are featured by arranging the production plan according to the predetermined order. Valuable insights can be drawn from other applications of the shared economy, such as housing leasing (Koster et al., Citation2021) and transportation (Lau & Li, Citation2021), where digital platforms play a vital role in providing various services.
Currently, the adoption of Internet of Things (IoT) facilities in agriculture is not widespread. A fragmented digital agricultural ecosystem has been linked to the slow scale-up of digital platforms and other digital technology solutions for agriculture (Naika et al., Citation2021). The technological transformation from the laboratory to practical implementation in the field remains a challenge. Two key factors, namely platform-wide efficiency and loyalty-centredness, have been identified, which are related to user experience, including reducing search costs and ensuring quality and reliability for platform users. Some argue that, for small agricultural businesses, technology adoption is not just a matter of efficiency but also maintaining a social identity within the supply chain (Tsai et al., Citation2021). In addition, the agricultural system has social and economic properties (Moreno-Miranda & Dries, Citation2022). Based on an investigation of digital agriculture concerning farm production systems, value chains and food systems by social science researchers, it is concluded that so far digital agriculture socio-cyber-physical-ecological system conceptualization is still weakly developed (Klerkx et al., Citation2019). Considering the effects of economic and social factors, an agricultural cyber-physical-social system (CPSS) framework was proposed (Kang et al., Citation2018). As a result, agricultural systems must be designed and implemented in line with the national and even regional social and economic environment. How to reach the grassroots farmers in a cost-effective way is still a challenge.
The contribution of this paper is (1) to design a digital agriculture service system that fits the features and needs of smallholder farmers, with a social dimension; and (2) to design and demonstrate a sustainable environmental control system for low-cost greenhouses, which are typically lightweight and affordable. Compared to open-field grain crops, horticultural products are more sensitive to price variation and less storable, making them particularly reliant on digital agriculture service. In China, the dominating greenhouses are plastic greenhouse and solar greenhouse, accounting for 65.4% and 30.4% respectively. Such greenhouses are generally small and cost-effective compared to modern glass greenhouse, making them accessible to smallholder farmers. However, the greenhouse building is often non-standardized, appealing for personalized control policy and the communication of IoT facilities are not always stable in remote area. Therefore, a distributed system architecture is proposed where the local system can run independently with or without a network connection. Intelligent control helps to achieve desired environmental conditions for standardized horticultural product. A case study on a management and control system of solar greenhouses in Shandong province is provided to show how the proposed design and implementation can be applied and adapted to suit the needs of smallholder farmers.
This paper is organized as follows. Section 2 provides a literature review of the related studies. Section 3 presents the framework for a distributed agricultural services system, including its architecture, key functions and targeted users. Section 4 illustrates a case study of the subsystem designed for monitoring and controlling a solar greenhouse. Section 5 discusses the features and potential of the proposed system for the future. Finally, Section 6 summarizes the key findings and conclusions of the paper.
2. Literature review
With the advancement of information and communication technology (ICT), two important concepts, Internet-of-Things (IoT) and cloud computing, have emerged (Evans, Citation2011), which are expected to be used in agriculture (Fantana et al., Citation2013; Kaloxylos et al., Citation2012). The main goals of IoT include enhancing efficiency, accuracy, economic gains, and improving the overall quality of life (Höller et al., Citation2014). These technologies and innovations, when applied in agriculture, are called smart agriculture, smart farming, or agriculture 4.0 (Cui et al., Citation2018). Agriculture 4.0 aims at providing information and solutions to enhance crop productivity without increasing crop area, optimize irrigation processes by reducing water and energy consumption, and enable more efficient pest control. These are achieved by integrating technologies for environmental measurements, prediction and automation tools (Rettore de Araujo Zanella et al., Citation2020). Over the past decades, decision support systems have been developed to provide farmers with specialized knowledge for managing their farms. For example, Rossi et al. (Citation2014) designed a decision support system to help grapevine farmers make the right decision at the proper time for pesticide treatment. This system can monitor basic parameters in the vineyard about air, soil, plants, pests and diseases, and analyse the data to provide alerts and decision support information. Similarly, Popović et al. (Citation2017) developed a private IoT platform for research in the fields of smart agriculture and ecological monitoring. This platform has already been used for the development of intelligent spraying and irrigation, assessment of the marine environment and monitoring of fish/mussel farm.
Recent advancementsin artificial intelligence (AI) and machine learning have led to the emergence of intelligent agricultural systems. In the irrigation field, monitoring, control and decision-making solutions have been developed to save water and improve production. Navarro-Hellín et al. (Citation2016) proposed an automatic Smart Irrigation Decision Support System (SIDSS) to manage agricultural irrigation, which can estimate the weekly irrigation needs of a plantation. Rajalakshmi and Devi Mahalakshmi (Citation2016) developed a system for monitoring fields using sensors (soil moisture, temperature, humidity, light) and automating the irrigation system. Goap et al. (Citation2018) presented an open-source technology-based smart system to predict the irrigation requirements of a field using the sensing of ground parameters like soil moisture, soil temperature and environmental conditions, along with weather forecast data from the Internet. In farm management and environmental control, Lee et al. (Citation2017) designed and implemented a smart farm environmental management system that can collect and monitor information on crop growth, and control horticulture facilities. This system enables remote monitoring and control of smart farm environmental information and utilizes cloud-based big data analysis to optimize the environment for crop growing. Musat et al. (Citation2018) presented an intelligent platform for farmers to effectively manage their greenhouses and interact with other farmers. Tran et al. (Citation2017) proposed a system architecture and relevant solutions for monitoring the farm environment, performing remote automation and user control by integrating different modules related to sensing systems, communication and data analytics into a whole system. In disease monitoring and prediction, Trilles et al. (Citation2020) applied a low-cost sensory platform called SEnviro to monitor mildew disease in vineyards. Oliver et al. (Citation2018) developed a comprehensive IoT environment to monitor and predict certain vineyard diseases to help farmers improve the product quality and reduce losses.
Web-service platforms are emerging in the agricultural domain, offering various functionalities and benefits to users. Raducu et al. (Citation2015) presented a multilayer architecture for a real-time alert service integrated into a specific intelligent environment, namely, 'Smart farms'. Colezea et al. (Citation2018) developed an integrated web-based services platform that improves the quality of products grown on farms and supports business development in agricultural-related fields. This platform allows users to effectively locate and manage their farms by providing access to different types of statistics and forecasts. It also acts as a social network where users can interact with each other by sending private messages or posting on the forum or the blog.
Smart agriculture inherits ideas from precision agriculture and brings agricultural production and management from mechanization and informalization to intelligentization with automatization (Huang, Citation2019). Smart Agriculture is very helpful to farmers in optimizing their resources. However, to date, its adoption rate is still below expectations (Higgins et al., Citation2017; McConnell, Citation2019; Pathak et al., Citation2019). This can be attributed, in part, to the initial investment required to implement the technologies and procedures, which represents a long-term investment. Moreover, the complexity of the novel technologies involved necessitates a certain level of learning and skill acquisition for their effective adoption and management (Pathak et al., Citation2019), which varies among individuals and can thus affect successful implementation. The market offers a wide range of technological solutions with diverse functions that can be combined (Cisternas et al., Citation2020). However, farmers need a minimum level of knowledge to properly choose the right combination of available technologies (Higgins et al., Citation2017).
While precision farming practices have been widely adopted in developed countries, developing countries, especially small-scale farms, are now starting to adopt the technology (Pallottino et al., Citation2021). However, the adoption of IoT-based smart agriculture programs in different countries is hindered by factors such as implementation costs, limited availability of technologies, and a lack of knowledge. Additionally, many small-scale farmers in developing countries may not see the immediate benefits of implementing such technologies, further hampering adoption. Accordingly, developing suitable precision farming systems for smallholder farmers remains a challenge for researchers and developers (Sinha & Dhanalakshmi, Citation2022).
3. System design
The overriding consideration of system design is to bring convenience to farmers and let them focus on the agricultural operation itself, and minizing the requirement for extensive knowledge or abilities in information processing, management and product sales. Focusing on producers, the system is designed to maximize the values for stakeholders involved in the production chain. It is expected that such timely information exchange among related stakeholders will reduce resource waste and minimize environmental impacts. This new mode is driven by both supply and demand sides: farmers are increasingly concerned with sustainable production practices, while consumers expect healthy and safe foods and are willing to pay somewhat higher prices for them.
3.1. System frame
In a scenario with smallholder farmers and dedicated social service providers, including those involved in the sale of fertilizers, seeds, seedlings, machinery, and final agricultural products, an interconnected agricultural network is formed. This network can be supported by one or multiple entities. The foundation of this network is the smart agriculture framework of an agricultural CPSS, which consists of information perception, decision support and decision execution (application) (Kang et al., Citation2018). The smart agriculture framework relies on the integration of both physical and social information. Physical information includes the environment of crop growth, crop yield and the states of greenhouse facilities, and social information includes the product price (reflecting the balance of product supply and demand), growers' abilities, etc. Decision execution is achieved by automatic control of the environment with mobile applications. Decision support is the kernel of this system, as shown in .
Intelligent decision support aims to provide services covering the entire production chain, including pre-cultivation, cultivation and post-cultivation stages. While the suggestions are given in advance, it is essential to adapt decisions based on the actual situation, considering the variations in environmental conditions and management practices. Given the complexity of agricultural production systems, a parallel method that is composed of Artificial system, Computational experiment and Parallel execution (ACP) has been proposed for intelligent decision support, including description, prediction and prescription (Kang & Wang, Citation2017).
3.2. System functions
The main functions of the intelligent decision support system include monitoring and predicting product prices, crop planning, management of stakeholders (growers, technicians, experts, customers and managers), environment monitoring and control, planting records, etc. Without special claims, the listed functions are for the director users (farm managers). The following explains the details of the main functions.
3.2.1. Monitoring and forecast of the agricultural product price
In the age of big data, agricultural commodity prices are usually available on websites, especially for large-scale markets. This social information provides valuable insights into the daily fluctuations of fruit and vegetable prices. However, without filtering and analysis, it is very easy for smallholder farmers to get lost in the ocean of information. Fortunately, the web-crawl technique offers a convenient solution for gathering price information. Price prediction models employ various approaches, include regression methods, time series methods and machine learning methods (Su & Wang, Citation2021).
In our work, the neural network algorithm has been applied to forecast the monthly, weekly and daily price of agricultural products (Weng et al., Citation2019). The input layer is the historical price of agricultural products, assume that there are nodes, noted as
; the output layer is the forecasting price of agricultural products, assume that there are
nodes, noted as
; the output of the hidden layer includes
nodes, noted as
; the weight matrix and the bias matrix between the input and output layers are
and
, the activation function is
; the weight matrix and the bias matrix between the hidden and output layers are
and
, the activation function is
. The matrix of the actual future price of the agricultural product is
; the sample size is
. The BP neural network can be computed as follows:
The input layer to hidden layer:
(1)
(1) The output of the hidden layer is calculated by hidden activation function:
(2)
(2)
The hidden layer to output layer:
(3)
(3) The output of the network is calculated by output activation function:
(4)
(4)
Thus, the output of the model can be computed according to the (2) and (4):
(5)
(5) Using a neural network algorithm, based on the monitored price, the price for the following days, weeks and months can be predicted (). The accuracy of the prediction for the next day or week can reach 85%. Pricing with different time scales can help farmers make crop planning in pre-cultivation and harvesting time, respectively.
Figure 2. Actual and forecasted cucumber prices in Beijing Xinfadi market from 21 May 2018 to 23 July 2018 (Weng et al., Citation2019). The circles represent the actual prices. The line represents the forecasted prices using the BP network method.

3.2.2. Crop planning
Crop planning is to provide solutions to determine when, what species and how many areas to plant. Taking the greenhouse as an example, the planting time and area must be carefully scheduled to ensure a continuous market supply or maximize profits. Crop planning is very challenging as weather and economic conditions are varying. Study in this respect appeared since 1893 in the field of operations research (Vandenburg, Citation1893). Crop planning is often modelled as a non-linear programming problem (Dury et al., Citation2012). Objectives include yield or income, with ecological and/or economic constraints. Our recent work (Fan et al., Citation2022) is to simulate the decision-making process and income of individual farmers using an agent-based model, based on weekly and monthly price information in heuristic searching of the target; the plan is dynamically updated in response to price variations. If each farmer makes his own plan, the supply and demand for agricultural products are often unbalanced and ultimately lead to a loss of profits. Such a system, known as the 'Merton' system (Wang, Citation2012), analogous to traffic flow, where emergent properties may appear in the behaviour of the system. Similarly, as the traffic light and induction can change the system behaviour, the computed plan can be recommended to users to achieve a balance between supply and demand in the agricultural market.
3.2.3. Environment monitoring and control
Crop management is dependent on the developmental stages of the crop, which are determined by environmental conditions. For example, during the flowering stage when the fruit is highly sensitive to temperature and light (Mata & Botto, Citation2011), the set-points of the environment differ from the vegetative stage. The experienced service providers are generally know the required temperatures for the different stages. Therefore, it is necessary to integrate the management and control into a production management system to provide all-in-one services to farmers, particularly in controlled environment agriculture (CEA). Through the use of IoT devices, users can effectively monitor the environment inside the greenhouse, including the temperature and humidity of the air and soil, light intensity, CO2 concentration, soil pH value, EC level, etc. Such information is useful for evaluating not only the management level of the farmers but also the quality and quantity of the yield.
Since different factors can influence each other, highly sophisticated environmental models have been developed for modern greenhouses (Katzin et al., Citation2020). In plastic greenhouses, however, the standardization level is generally low, making the application of theoretical model challenging. In such cases, data-driven models can be applied to estimate indoor greenhouse temperature based on open-field data and indoor monitoring (Hua et al., Citation2017). For instance, in scenarios involving CO2 injection, optimizing ventilation becomes crucial as it not only impacts temperature regulation but also influences CO2 emissions (Weng et al., Citation2020).
3.2.4. Service on farming operations
Agricultural operations include a range of procedures involved in production management, including irrigation, fertilization, fruit thinning, etc., at different stages of development. These procedures are the detailed implementation of the crop planning. While some operations, like irrigation, can be automated, many are still done manually, such as leaf pruning. Agricultural technical consultation plays a crucial role in providing guidance to farmers regarding when and how to perform these operations. Traditionally, technicians would visit the farm to provide their services. However, with the advent of digital systems, the range of service can be expanded by providing online suggestions and receiving feedback. The exchange of information during these operations can be multi-modal (text, image, audio or video). This information can be used in a system to trace the lifetime of the crop.
Machine learning techniques enable the automatic extraction of some phenotypic information, such as flowering and fruiting, from photos. This capability allows for the continuous monitoring of crop development, enabling recommendations for agricultural operations to be updated according to the actual situation. While currently, it is still common to have fully human intelligence Q&A for crop disease identification or nutrition diagnosis, the potential of AI foundation models in information understanding and interpretation has been demonstrated (Kang et al., Citation2023). As AI advances and knowledge accumulates, it is anticipated that disease identification or nutrition diagnosis, which are still in their early stages, could be more machine-driven in the future.
3.3. Dedicated users
According to the role of stakeholders in the physical-social agricultural system, two types of users can be defined, namely direct users and indirect users, as shown in . A distributed network, instead of a centralized structure, is expected.
3.3.1. Direct users
The direct users of the system are farmers, who benefit from a range of services covering the entire production chain, including pre-cultivation, production and post-production. In the pre-cultivation phase, the main service is crop planning, which offers recommendations on optimal planting areas and timing according to certain goals, such as ensuring a steady supply of yield per week (Kang et al., Citation2018) or maximizing the profits of farmers (Fan et al., Citation2022). During the production phase, the system provides valuable insights on the timing and quantity of agricultural material inputs (e.g. fertilizers, pesticides), as well as guidance on essential agricultural operations (e.g. irrigation, disease preventive treatments). In the post-production phase, the system plays a vital role by providing market information (e.g. prices and demands of agricultural products) to farmers. Additionally, it serves as an information bridge by providing customers with insights into the processes of crop growth. Through their active participation in the production process and data recording, farmers contribute to both the physical world by producing the agricultural products and the digital world by generating agricultural data.
3.3.2. Indirect users
The indirect users of the system are various stakeholders, including suppliers of fertilizers, seeds, seedlings, machineries, wholesalers of agricultural products, third-party financial organizations, individual experts, government and personal customers. Those users together form an interconnected online business network. Field data collected through the system can provide additional value to these stakeholders (). For example, agricultural input suppliers can provide precise technical suggestions and sales information based on the farmer's planting data, allowing them to provide tailored recommendations and services while gaining insights into user preferences. Another valuable application is the financial service provided by third-party (e.g. agricultural insurance). Credit assessment is often obtained through costly on-site visits by village managers or neighbours for identifying credible farmers. However, a digital system can provide information on the assets of agricultural areas. Nevertheless, defining a service license within the platform to form an online virtual community of agricultural stakeholders remains an ongoing challenge that requires further attention and development.
Table 1. Gained information and benefits for different stakeholders.
3.4. System implementation
Given the highly heterogeneous environment of agricultural services, the technical design of the system must be scalable and robust.
3.4.1. Fog computing
Smallholder farmers often lack access to professional servers and technical expertise. To address this, a lightweight, cost-effective, and intelligence system architecture has been designed for the user side (). This architecture combines both cloud and local control systems. The cloud system handles computationally intensive tasks, such as model training, and provides key parameters to local systems. On the other hand, the local system is dedicated to the specific environmental conditions and tasks (e.g. the ventilation control of a solar greenhouse). The local systems form a so-called 'fog architecture' with many distributed characteristics. The advantage of such an architecture is that the local system can run independently, with or without a network connection. This is particularly beneficial for smallholder farmers who may have remotely distributed farms with limited communication infrastructure. By reducing the need for continuous communication and computation with a remote server, the system alleviates the pressure on user-side operations. It is important for smallholders whose farm may be remotely distributed with poor communication. However, the initial configuration of the local control system is often carried out by experienced technicians or farmers. By recording and analyzing the monitored environment and performance data, knowledge can be learned and transferred to other local systems. External and internal climatic conditions, as well as the ventilation opening operation, will be applied to build the prediction model of future greenhouse temperature (Hua et al., Citation2017). The actual temperature at different stages of crop development provides key knowledge of cultivation. Through cloud-based training, the local system can be regularly updated using adaptive learning techniques based on management experiences and environmental factors. This iterative process enables the system to make better decisions that align with the real-time situation. This concept follows the idea of 'local simple and remote complex', which has been applied in parallel system (Chen, Citation2003).
3.4.2. Cloud and local system
The cloud system aims to serve many agricultural planting organizations rather than adapt it to a specific enterprise. Meanwhile, different types of devices are considered, including IoT devices, PC terminals, mobile App and WeChat Applets. The system architecture is designed with flexibility, separating the front end from the back end within the web service platform. The back end provides data for the mobile application (App) and browser access via the application programming interfaces (APIs), without being concerned with the user interface (UI). It was designed using micro-services Architecture (msA): individual modules are implemented in Java language and JAX-RS, and deployed on Tomcat servers; modules communicate with each other in JSON format; all modules are managed by a registry. Cloud-based systems are deployed on such platforms robustly, and provide professional services.
The IoT device terminal utilizes the Java language and the Netty network library, while also leveraging the InfluxDB time series database for efficient data management. Netty is a network library based on the event loop mode, which is well-suited for the scenes of IoT. In addition, the concurrency efficiency of Netty is much higher than that of traditional thread-based models, making it capable of meeting the concurrent needs of up to 100,000 devices. InfluxDB is a mainstream time series database with specialized optimizations for time series data, ensuring efficient storage, retrieval and statistics of time series data.
3.4.3. Local system
The local system is responsible for collecting information and executing decisions. Three types of terminals can be chosen: web browsers, Apps and applets. JavaScript is chosen for both App and web terminals for quick development and easy communication between system developers. Moreover, the React library is used for web and application development, and allows the reuse of parts of Web, IOS and Android codes.
Different user terminals have been considered. Web terminal is a traditional way to suit for handling large amounts of information in office environment due to its characteristics of a big screen and convenient input options (e.g. field descriptions, agricultural inputs and crop planning) as shown in . Users can operate by accessing the web page and logging into their accounts without installing any local software. In rural areas, mobile phones have become very common, and the mobile networks can cover the entire country. Mobile Apps are more convenient than web-based applications for ordinary farmers. Farm managers can record farm operations without time and location limitations, verify the environmental conditions of greenhouses, and share information on social media using the application terminal.
Compared to the mobile application, WeChat Applet is more convenient in China without the need for users to download or install any additional software. The advantage of Applet is that it is associated to the WeChat account without performing the login procedure repeatedly. Given the widespread usage of WeChat in China (with over 1.3 billion users by 2023), one can easily access the system with the Applet terminal. Although the Applet has limited complexity, functions can be split into multiple Applets, and each Applet is responsible for specific functions (e.g. for operation records and crop planning).
3.4.4. Environmental monitor and control system
The design principle of the environmental monitoring system includes low cost, convenient installation, considering the low cost of the dominant plastic greenhouse. The management of solar greenhouse is mainly concerned with temperature, humidity and light. In the local system, the monitoring device consists of temperature and humidity sensors, as well as light sensors. Two modes for data transmission have been developed. The first mode is to use GPRS to upload the data with the advantage of the widespread coverage of 4G/5G networks in rural areas. This approach requires only a single device without installing cables within the greenhouse, making it well-suited for smallholder farms. The other way is to build a local network for centralized uploading in a larger planting area. Each device contains solar panels, ensuring long-lasting operation even in the absence of direct light. Since the temperature sensor could drift under sunlight, two solutions are provided: data-based calibration and the use of Venetian blinds as a protective cover. The printed circuit board (PCB) is designed following a modular concept, allowing for easy integration of multiple sensors, including temperature, humidity and light intensity, for efficient and flexible data collection.
The control of greenhouse facilities, such as the manipulation of the plastic membrane for ventilation purposes, is mainly achieved by controlling the motor state, as shown in . This allows for adjustments according to the shape of the greenhouse and internal and external environmental conditions. The operating system is Linux, and the device program is developed uisng C++/QT. QT is adopted to meet the primary cross-platform design goal. With such benefits, the program can run on various platforms, including PCs, mobile phones, embedded devices, etc. Compared to a Java/Android solution, the C++/QT program needs much fewer hardware resources and can achieve system functions at a lower cost in the local system. The QT library provides a range of functions, including graphical user interface (GUI), network communication, internationalization, etc., which can satisfy various needs, including graphical display, touch screen control, network communication and Chinese interface. Since solar greenhouse lack standardization in their structures, the implementation of control goals must be customized according to the specific greenhouse conditions and outdoor climate. Once the control strategy is established and stabilized, the local control system can be carried out according to the predetermined strategy until the next training cycle.
3.4.5. System interfaces
Due to the linkage between software and hardware components, as well as the integration of different local application systems, the system defines several types of interfaces (), as follows.
The hardware device interface plays a crucial role in facilitating communication between the local control system and the cloud management system. Its mainfunction is to enable the transmission of sensor data from the local control system to the cloud, as well as to receive control and feedback commands from the cloud management system. Additionally, the hardware device interface incorporates functionalities that automatically assess the network conditions of the local devices. It determines when to wait for remote instructions, when to run autonomously, and record and report the status of the devices. It is an important component of fog computing.
The 'Token' interface is responsible for the communication between the web browser, App, Applet and the cloud management system. It is mainly used for the interaction between the users and the server, including data querying, viewing statistical charts, uploading farm operation records, viewing equipment status, and issuing device operation instructions. The token interface must also process user login, access authorization, etc.
Due to the design of micro-services on the back end, modules with different functions are all implemented as network services and accessed via internal interfaces. These interfaces form an internal network, invisible to the external network, and all external accesses are transferred through the Token interface. Currently, internal interfaces primarily include licensing, planting, equipment, expert Q&A and marketing.
4. Case study
Starting from 2014, with the creation of a novel organization, Qingdao Academy of Intelligent Industries (QAII), jointly by CASIA and Qingdao government (Shandong province), the authors started the design and implementation of Distributed Complex Adaptive Systems in Agriculture (DiCASA). With the research background in GreenLab plant modelling (de Reffye et al., Citation2021), one of our original motivations was to build and demonstrate an application scenario for plant model. GreenLab has been calibrated on several horticultural crops, including tomato (Kang et al., Citation2011), Chrysanthemum (Kang et al., Citation2012) and cucumber (Wang et al., Citation2020). Shandong province has the largest vegetable production area (∼200,000 hectares), around 40% of the total vegetable cultivation in China, served as an ideal setting for this research. The products include fruits and vegetables such as strawberry, melon, etc. To facilitate the project, our team cooperated with a largest service company that had initially operated as a fertilizer seller but had expanded its business to include the sale of high-quality fruits, while also providing agricultural expertise and information technique services to individual farmers. We encountered various challenges distinct from research area. These challenges included ensuring stable communication, training users in using the system effectively, addressing cost sensitivity, and evaluating the added value brought about by ICT solutions. To address these challenges, a case study focusing on fruit production in solar greenhouses was conducted. The main objective at the initial stage was to build a digital system for remote management and environmental control.
4.1. User terminal
Initially, a sophisticated web terminal was developed, as shown in . The web page allowed users to log in with their accounts and manage the planting information of area, activities, date of planting and fruit setting, etc. However, although logically complete and correct, the web terminal faced challenges as it requires farmers to use a computer for data entry, which was not a familiar practice for them.
The second trial is a mobile application and an applet. Especially, the WeChat applet offers the convenience of instant access without the need for downloading or installing additional software. Since 'seeing is believing', users are mainly required to take photos instead of writing down the text or numbers, as shown in (a). Photos are important data as they can show the status of the plant and phenotypic stage. A sequence of photos containing the corresponding location, time and environmental data can be produced for environmental monitoring and operation records, including the application of various inputs (fertilizers, pesticides, if applicable). Cultivation operations can be displayed with a growth chart, as shown in (b). This chart outlines the key developmental stages of melon growth along with corresponding dates. Users have the option to selectively share information, including photos, environmental data, input useage, and field-specific information, on social media platforms such as WeChat. The complete transparent tracing process is available to potential customers, ensuring trust and accountability.
4.2. Environment control
The key knowledge of the service organization is the optimal day and night greenhouse temperature depending on the stage of plant development. Temperature is mainly regulated by the size of the ventilation. However, manually adjusting the plastic membrane for ventilation with mechanical equipment can be time-consuming for farmers. To address this challenge, a control system was implemented to automate the process based on real-time monitored data. By setting a desired temperature setpoint, the mechanical installations can be actuated, and the opening size of the ventilation can be adjusted dynamically according to the monitored data. A simple but practical threshold-based control method was adopted since the temperature dropped rapidly with the opening of the ventilation. shows a result of controlling the opening of the solar greenhouse vent automatically to reach the desired air temperature. This allows farmers to save time and expand their capacity. Note that even when the upper setpoint of temperature is 28°C, the actual temperature inside the greenhouse may exceed this limit due to the limitations imposed by the greenhouse structure, even with the ventilation fully open.
4.3. Management of stakeholders
In this context, the main users of the system are farmers and technicians, each having their own access to the applet. Farmers are responsible for the actual operation of farming, such as irrigation, pesticide spraying, fruit thinning, etc. At the same time, they are also responsible for patrol inspection to record the growth of crops by taking photos. Each farmer has corresponding technicians, who can access the system and monitor the planting situation of the farmers under their supervision. Meanwhile, technicians can make agricultural arrangements in advance and provide operation suggestions to farmers. This makes it possible to improve the standardization of agricultural products and to naturally construct the traceability information of agricultural products.
4.4. Crop planting
Crop planning is visually represented using a Gantt chart to show planting time and expected harvest time (). Planting records are presented in two dimensions. In the temporal dimension, users can check the planting and harvest history of each plot/greenhouse. In the spatial dimension, users can check the planting progresses of all plots/greenhouses at a given date.
5. Discussion
5.1. Redesigned sustainable agriculture
The development of a redesigned sustainable agriculture has attracted a lot of attention (Cui et al., Citation2018). The redesigned agriculture is knowledge-intensive and includes not only the applications of biological agriculture and nanotechnology, but also the comprehensive applications of big data and ICT technologies (Haggar et al., Citation2021). In practice, innovation platforms have emerged worldwide, focusing on the co-production of technologies to advance the sustainable management of agriculture and land (Pretty et al., Citation2020). In China, the science and technology backyard platforms (STBs) (Zhang et al., Citation2016) were established to enhance the sharing of knowledge and skills between scientists and farmers. STBs bring agricultural scientists to live in villages and use field demonstrations, agricultural schools and yield contests to involve farmers in locally and externally developed innovations. This successful practice demonstrates the importance and effectiveness of knowledge transfer between individual farmers, and the ICT platform can enhance the dissemination capacity of knowledge initiated by individual scientists. AI techniques can mimic the experienced growers to improve product yield and quality, which has been proven in many other areas (Silver et al., Citation2016, Citation2017). However, the process of redesigning agriculture is not only a technical challenge but also a social and institutional one. It necessitates the creation and effective utilization of human capital, encompassing not only knowledge adaptation and innovation but also social capital to promote landscape-scale change (Pretty et al., Citation2020). The term 'social capital' is used to describe the importance of social connections, trust and reciprocity, and collective action through institutions in driving transformative agricultural practices.
In line with this idea, recently, more startups in the field of smart agriculture have emerged. These startups aim to coordinate the actions among small-scale farmers with one-stop services that encompass both the planting and sale of cash crops like tomatoes, blueberries and strawberries. These services are often supported by Software as a Service (SaaS) platform. The promotion of ICT facilities is driven by marketing service that sell the product online by professionals in popular video platform. The whole service package is composed of not only ICT facilities (rather cheap) but also on-site consultation, allowing growers to focus on their planting activities. Such multi-functional team, which owns know-how in electronic commerce, information technology and agricultural knowledge, has proven to be vital for the successful adoption and promotion of agricultural technologies. The availability of these services has made it easier for individuals interested in ecological agriculture to get involved, while also providing new opportunities for young people.
The digital social agriculture service system provides a basis for prescribing activities in the real world. The term 'prescription' has been commonly used in agriculture for fertilizer application, based on the soil measurement and nutrition needs assessment (Bacenetti et al., Citation2020). Broadly speaking, prescription involves decision-making throuthout the entire production chain, including crop planning and environmental control. The digital mapping of the real system leads to a parallel agricultural system, where prescriptions are generated based on predictions of the future, which in turn are based on the description of system activities. Once moved from physical space to digital space, algorithms can be applied to solve computational problems. Computation in the digital world can support decision-making for physically dispersed smallholder farmers, seamlessly linking demand and supply, and promoting the sustainable development of agriculture. A more organized agricultural network is expected through a combination of online and on-site services.
5.2. Sustainable environment control of low-cost greenhouse
In this paper, the sustainability of the environment control system of low-cost greenhouse lies in: (1) within a context of integrated production and market service, the environment control of low-cost greenhouses becomes demand-driven to produce more standardized products; (2) sensors are equipped in such a way to meet the most common daily need of the greenhouse, striking a balance between cost and performance. It has been proven that such a system with a solar energy source can operate for several years in a greenhouse without maintenance, given the high-humidity high-temperature environment of the solar greenhouse; this feature is important for aged people as the learning cost is low; (3) the IoT-based agricultural environmental control system is closely related to the production chain system. The former deals with information acquisition and task implementation within a specific local environment (Jayaraman et al., Citation2016; Kamilaris et al., Citation2017), while the latter mainly focuses on information management and decision support for production processes (e.g. crop planning) (Kaloxylos et al., Citation2014; Pavón-Pulido et al., Citation2017). However, as mentioned, successful environmental control depends on establishing control goals, which are related to the species and their growth stages. This needs knowledge of crop ecophysiology and experience in production management, which is often lacking among IoT experienced technicians. Conversely, the satisfactory achievement of production goals depends on environmental control and the implementation of various installations. An analogue is a manufacturing execution system (MES) in industry. The integration of multidisciplinary experience and knowledge is a key feature of such integration. Deep integration of ICT technology and agricultural knowledge has the potential to drive transformative advancements in agricultural technology (Ding et al., Citation2022).
5.3. Distributed and social digital system
Designing and implementing a CPSS for traditional farms poses several challenges. First, the production relationship needs to be well understood, as well as the needs and level of end-users. It is not the most advanced but the most suitable technology that works, indicating the importance of the social dimension (Steinke et al., Citation2021). As previously mentioned, the authors have experienced the development of a sophisticated and logical system which was unacceptable by users. For smallholder farmers, the system must be easy-to-use, cheap and most of all, help them make a profit. In China, the widely popular platform WeChat serves as a valuable tool. With 78% of people in rural areas using WeChat for payment purposes in 2020, it has become a common platform for remote monitoring and tracing. Through WeChat-based Applet, users can access the digital system with just one click. The cost of an IoT-based monitoring system, such as the one mentioned here, can be as low as 200 RMB for a greenhouse. A digital system is a tool rather than replace the on-site management team composed of service technicians. The system can expand service capacities by using AI techniques: once a cultivation operation model is built, the system can provide farmers with suggested activities, based on the monitored environmental and crop data. Second, with the increase in associated farmers, the computational complexity must be addressed. For example, the service company in the case study plans to extend to 20,000 smallholder farmers in the future. Centralized computing can be unaffordable, both technically (e.g. considering crop planning) and socially (e.g. considering the diversity of local socio-economic conditions). It is expected that distributed AI can provide support by enabling decentralized computing (Wang et al., Citation2021). Data security is another critical concern. Blockchain technology can give potential solutions by ensuring data integrity and privacy (Yuan et al., Citation2017). Methods such as consensus algorithms (Yuan et al., Citation2016) and security encryption (Zhu et al., Citation2021) can support the right use of data, based on joint planning among stakeholders. The cyber-physical-social system naturally leads to the concept of an agricultural metaverse (Wang et al., Citation2022), which is featured by real-virtual interaction of social network.
Based on the concept of micro-service, the designed system makes full use of the interface to tailor the function. In addition, the separating design between the front-end and the back-end makes it easy to add intelligent computation into the system. For example, using the monitored environmental data, crop phenology can be predicted and will provide guidance for agricultural operations in advance (Hua et al., Citation2017). Additionally, crop models for yield forecasting (Fan et al., Citation2015) can be integrated into the system to support planting schedules. However, the level of intelligence is related to the abundance of data, or users. Accompanying the construction of an online farm, one requirement is to establish online planting standards that encompass comprehensive data recording reflecting the whole production processes and verifying organic or nuisance-free cultivation. Currently, practical data recording standards are lacking in existing planting standards.
5.4. From Newton to Merton system
As previsously mentioned, small-scale business entities in China are expected to remain for a period of time. At present, farmers usually rely on the market situations from the previous year and the planting practices in neighbouring areas to make crop planning. However, this approach contributes to price fluctuations and the loss of farmers' profits. The system with such behaviours can be called the 'Merton' system (Wang, Citation2012, Citation2015), where human choice can affect the status of the overall system. In contrast, a digital service system can guide users' behaviours based on comprehensive big data analysis, thus affect the market.
The complexity of agricultural prescription also lies in the periodicity of agricultural production (e.g. the growth of vegetables needs one to several months), which depends on the local environment and cultivation techniques. Predicting crop phenology and yield is a key technology in agricultural production management systems. Compared with the crop model built into the experimental environment, the data and knowledge-driven model has potential with great adaptability to the actual planting environment, as well as integrating the knowledge of crop growth and development (Fan et al., Citation2015, Citation2018). The availability and quantity of agricultural-related physical and social data far exceeds the past age, and knowledge automation provides a solution for automatically acquiring experience from data (Fan et al., Citation2015). When objects in the system evolve from the environment to the plant-environment until the plant-environment-market, the system evolves from the Newton system to the Merton system.
6. Conclusion
This paper presents the design and implementation of a distributed agricultural services system aimed at meeting the needs of massive smallholder farmers. The system is designed with a focus on lightweight, low cost and intelligence. The direct users of the system are the farmers, and the indirect users are stakeholders involved in agricultural supply chains such as service providers. Building an online agricultural network with such a system can support smallholders with functions such as crop planning, operational recommendation, environmental control and finally better profit and sustainability.
Disclosure statement
No potential conflict of interest was reported by the author(s).
Additional information
Funding
Notes on contributors
Jing Hua
Jing Hua is currently an Assistant Professor of Computer Science with the Institute of Automaton, Chinese Academy of Sciences. His research interest covers computer science and agriculture, including virtual plant modeling, smart agriculture, programming languages, distributed computer systems, and computer graphics.
Haoyu Wang
Haoyu Wang is currently an Assistant Professor of Computer Science with the Institute of Automaton, Chinese Academy of Sciences, Beijing. His research interest covers computer science and agriculture, including virtual plant modeling, smart agriculture, programming languages, and information system.
Mengzhen Kang
Mengzhen Kang is currently an Associate Professor with the Institute of Automation, Chinese Academy of Sciences, and also with the School of Artificial Intelligence, University of Chinese Academy of Sciences, Beijing. Her current research interests include parallel agriculture and computational plants.
Xiujuan Wang
Xiujuan Wang is currently an Associate Professor with the Institute of Automaton, Chinese Academy of Sciences. Her current research interests include plant growth modeling and parallel agriculture.
Shaoxin Guo
Shaoxin Guo is currently an engineer with the Institute of Automaton, Chinese Academy of Sciences. His current research interests include smart agriculture, programming languages, and information system.
Fangle Chang
Fangle Chang is currently an Assistant Professor with Ningbo Innovation Center, Zhejiang University, Ningbo, China. Her research interest covers target detection and multi–source data fusion, complex system management and control.
Fei-Yue Wang
Fei–Yue Wang is currently a Professor with the Institute of Automation, Chinese Academy of Sciences, Beijing, China, also with the School of Artificial Intelligence, University of Chinese Academy of Sciences, Beijing. His current research focuses on methods and applications for parallel systems, social computing, and knowledge automation.
References
- Abokyi, E., Strijker, D., Asiedu, K. F., & Daams, M. N. (2020). The impact of output price support on smallholder farmers’ income: Evidence from maize farmers in Ghana. Heliyon, 6(9), Article e05013. https://doi.org/10.1016/j.heliyon.2020.e05013
- Bacenetti, J., Paleari, L., Tartarini, S., Vesely, F. M., Foi, M., Movedi, E., Ravasi, R. A., Bellopede, V., Durello, S., Ceravolo, C., Amicizia, F., & Confalonieri, R. (2020). May smart technologies reduce the environmental impact of nitrogen fertilization? A case study for paddy rice. Science of the Total Environment, 715, Article 136956. https://doi.org/10.1016/j.scitotenv.2020.136956
- Chen, L. (2003). A new neuro-fuzzy system and its implementation of “local simple remote complex” control principle. Master, Institute of Automation, Chinese Academy of Sciences. http://www.wanfangdata.com.cn/details/detail.do?_type = degree&id = Y545323.
- Cisternas, I., Velásquez, I., Caro, A., & Rodríguez, A. (2020). Systematic literature review of implementations of precision agriculture. Computers and Electronics in Agriculture, 176, Article 105626. https://doi.org/10.1016/j.compag.2020.105626
- Colezea, M., Musat, G., Pop, F., Negru, C., Dumitrascu, A., & Mocanu, M. (2018). CLUeFARM: Integrated web-service platform for smart farms. Computers and Electronics in Agriculture, 154, 134–154. https://doi.org/10.1016/j.compag.2018.08.015
- Cui, Z., Zhang, H., Chen, X., Zhang, C., Ma, W., Huang, C., Zhang, W., Mi, G., Miao, Y., & Li, X. (2018). Pursuing sustainable productivity with millions of smallholder farmers. Nature, 555(7696), 363–366. https://doi.org/10.1038/nature25785
- de Reffye, P., Hu, B., Kang, M., Letort, V., & Jaeger, M. (2021). Two decades of research with the GreenLab model in agronomy. Annals of Botany, 127(3), 281–295. https://doi.org/10.1093/aob/mcaa172
- Ding, J., Jia, X., Zhang, W., & Klerkx, L. (2022). The effects of combined digital and human advisory services on reducing nitrogen fertilizer use: Lessons from China’s national research programs on low carbon agriculture. International Journal of Agricultural Sustainability, 20(6), 1136–1149. https://doi.org/10.1080/14735903.2022.2057643
- Dury, J., Schaller, N., Garcia, F., Reynaud, A., & Bergez, J. E. (2012). Models to support cropping plan and crop rotation decisions. A review. Agronomy for Sustainable Development, 32(2), 567–580. https://doi.org/10.1007/s13593-011-0037-x
- Evans, D. (2011). The internet of things: How the next evolution of the internet is changing everything. Cisco Internet Business Solutions Group (IBSG), 1, 1–11. http://www.cisco.com/web/about/ac79/docs/innov/IoT_IBSG_0411FINAL.pdf
- Fabregas, R., Kremer, M., & Schilbach, F. (2019). Realizing the potential of digital development: The case of agricultural advice. Science, 366(6471), Article eaay3038. https://doi.org/10.1126/science.aay3038
- Fan, M., Kang, M., Wang, X., Hua, J., He, C., & Wang, F.-Y. (2022). Parallel crop planning based on price forecast. International Journal of Intelligent Systems, 37(8), 4772–4793. https://doi.org/10.1002/int.22739
- Fan, X.-R., Kang, M., Heuvelink, E., de Reffye, P., & Hu, B.-G. (2015). A knowledge-and-data-driven modeling approach for simulating plant growth: A case study on tomato growth. Ecological Modelling, 312, 363–373. https://doi.org/10.1016/j.ecolmodel.2015.06.006
- Fan, X.-R., Wang, X., Kang, M., Hua, J., Guo, S., de Reffye, P., & Hu, B.-G. (2018). A knowledge-and-data-driven modeling approach for simulating plant growth and the dynamics of CO2/O2 concentrations in a closed system of plants and humans by integrating mechanistic and empirical models. Computers and Electronics in Agriculture, 148, 280–290. https://doi.org/10.1016/j.compag.2018.03.006
- Fantana, N. L., Riedel, T., Schlick, J., Ferber, S., & Svensson, S. (2013). Internet of things: Converging technologies for smart environments and integrated ecosystems. River Publishers Series in Communications.
- Goap, A., Sharma, D., Shukla, A. K., & Rama Krishna, C. (2018). An IoT based smart irrigation management system using machine learning and open source technologies. Computers and Electronics in Agriculture, 155, 41–49. https://doi.org/10.1016/j.compag.2018.09.040
- Haggar, J., Nelson, V., Lamboll, R., & Rodenburg, J. (2021). Understanding and informing decisions on sustainable agricultural intensification in Sub-Saharan Africa. International Journal of Agricultural Sustainability, 19(5-6), 349–358. https://doi.org/10.1080/14735903.2020.1818483
- Higgins, V., Bryant, M., Howell, A., & Battersby, J. (2017). Ordering adoption: Materiality, knowledge and farmer engagement with precision agriculture technologies. Journal of Rural Studies, 55, 193–202. https://doi.org/10.1016/j.jrurstud.2017.08.011
- Höller, J., Tsiatsis, V., Mulligan, C., Karnouskos, S., & Boyle, D. (2014). From machine-to-machine to the internet of things: Introduction to a new age of intelligence. Academic Press. https://doi.org/10.1016/C2012-0-03263-2
- Hua, J., Wang, X., Kang, M., Wang, H., & Fan, X.-R. (2017). Prediction of crop phenology – a component of parallel agriculture management. Chinese Automation Congress & Intelligent Manufacturing International Conference (CAC2017 & CIMIC2017), Jinan, Shandong.
- Huang, Y. (2019). Perspectives and experiences on the development and innovation of agricultural aviation and precision agriculture from the Mississippi Delta and recommendations for China. Smart Agriculture, 1(4), 12–30. https://doi.org/10.12133/j.smartag.2019.1.4.201909-SA003
- Jayaraman, P., Yavari, A., Georgakopoulos, D., Morshed, A., & Zaslavsky, A. (2016). Internet of things platform for smart farming: Experiences and lessons learnt. Sensors, 16(11), Article 1884. https://doi.org/10.3390/s16111884
- Jiao, X.-Q., Zhang, H.-Y., Ma, W.-Q., Wang, C., Li, X.-L., & Zhang, F.-S. (2019). Science and technology backyard: A novel approach to empower smallholder farmers for sustainable intensification of agriculture in China. Journal of Integrative Agriculture, 18(8), 1657–1666. https://doi.org/10.1016/S2095-3119(19)62592-X
- Kaloxylos, A., Eigenmann, R., Teye, F., Politopoulou, Z., Wolfert, S., Shrank, C., Dillinger, M., Lampropoulou, I., Antoniou, E., Pesonen, L., Nicole, H., Thomas, F., Alonistioti, N., & Kormentzas, G. (2012). Farm management systems and the future internet era. Computers and Electronics in Agriculture, 89, 130–144. https://doi.org/10.1016/j.compag.2012.09.002
- Kaloxylos, A., Groumas, A., Sarris, V., Katsikas, L., Magdalinos, P., Antoniou, E., Politopoulou, Z., Wolfert, S., Brewster, C., Eigenmann, R., & Maestre Terol, C. (2014). A cloud-based farm management system: Architecture and implementation. Computers and Electronics in Agriculture, 100, 168–179. https://doi.org/10.1016/j.compag.2013.11.014
- Kamilaris, A., Kartakoullis, A., & Prenafeta-Boldú, F. X. (2017). A review on the practice of big data analysis in agriculture. Computers and Electronics in Agriculture, 143, 23–37. https://doi.org/10.1016/j.compag.2017.09.037
- Kang, M., Fan, X.-R., Hua, J., Wang, H., Wang, X., & Wang, F.-Y. (2018). Managing traditional solar greenhouse with CPSS: A just-for-fit philosophy. IEEE Transactions on Cybernetics, 48(12), 3371–3380. https://doi.org/10.1109/TCYB.2018.2858264
- Kang, M., Heuvelink, E., Carvalho, S. M. P., & de Reffye, P. (2012). A virtual plant that responds to the environment like a real one: The case for chrysanthemum. New Phytologist, 195(2), 384–395. https://doi.org/10.1111/j.1469-8137.2012.04177.x
- Kang, M., & Wang, F.-Y. (2017). From parallel plants to smart plants: Intelligent control and management for plant growth. IEEE/CAA Journal of Automatica Sinica, 4(2), 161–166. https://doi.org/10.1109/JAS.2017.7510487
- Kang, M., Wang, X., Wang, H., Hua, J., de Reffye, P., & Wang, F.-Y. (2023). The development of AgriVerse: Past, present, and future. IEEE Transactions on Systems, Man, and Cybernetics: Systems, 1–10. https://doi.org/10.1109/TSMC.2023.3271396
- Kang, M., Yang, L., Zhang, B.-G., & de Reffye, P. (2011). Correlation between dynamic tomato fruit-set and source–sink ratio: A common relationship for different plant densities and seasons? Annals of Botany, 107(5), 805–815. https://doi.org/10.1093/aob/mcq244
- Katzin, D., van Mourik, S., Kempkes, F., & van Henten, E. J. (2020). Greenlight – An open source model for greenhouses with supplemental lighting: Evaluation of heat requirements under LED and HPS lamps. Biosystems Engineering, 194, 61–81. https://doi.org/10.1016/j.biosystemseng.2020.03.010
- Kieti, J., Waema, T. M., Ndemo, E. B., Omwansa, T. K., & Baumüller, H. (2021). Sources of value creation in aggregator platforms for digital services in agriculture - insights from likely users in Kenya. Digital Business, 1(2), Article 100007. https://doi.org/10.1016/j.digbus.2021.100007
- Klerkx, L., Jakku, E., & Labarthe, P. (2019). A review of social science on digital agriculture, smart farming and agriculture 4.0: New contributions and a future research agenda. NJAS: Wageningen Journal of Life Sciences, 90-91(1), Article 100315. https://doi.org/10.1016/j.njas.2019.100315
- Koster, H. R. A., van Ommeren, J., & Volkhausen, N. (2021). Short-term rentals and the housing market: Quasi-experimental evidence from Airbnb in Los Angeles. Journal of Urban Economics, 124, Article 103356. https://doi.org/10.1016/j.jue.2021.103356
- Lau, H. C., & Li, B. (2021). Solving the winner determination problem for online B2B transportation matching platforms. Transportation Research Part E: Logistics and Transportation Review, 150, Article 102324. https://doi.org/10.1016/j.tre.2021.102324
- Lee, M., Kim, H., & Yoe, H. (2017). Intelligent environment management system for controlled horticulture. 2017 4th NAFOSTED Conference on Information and Computer Science.
- Lowder, S. K., Skoet, J., & Raney, T. (2016). The number, size, and distribution of farms, smallholder farms, and family farms worldwide. World Development, 87, 16–29. https://doi.org/10.1016/j.worlddev.2015.10.041
- Mata, D. A., & Botto, J. F. (2011). Photoperiod, light, and temperature requirements to control plant architecture and flowering time in Salvia exserta. The Journal of Horticultural Science and Biotechnology, 86(4), 408–414. https://doi.org/10.1080/14620316.2011.11512782
- McConnell, M. D. (2019). Bridging the gap between conservation delivery and economics with precision agriculture. Wildlife Society Bulletin, 43(3), 391–397. https://doi.org/10.1002/wsb.995
- Mondal, P., & Basu, M. (2009). Adoption of precision agriculture technologies in India and in some developing countries: Scope, present status and strategies. Progress in Natural Science, 19(6), 659–666. https://doi.org/10.1016/j.pnsc.2008.07.020
- Moreno-Miranda, C., & Dries, L. (2022). Assessing the sustainability of agricultural production - a cross-sectoral comparison of the blackberry, tomato and tree tomato sectors in Ecuador. International Journal of Agricultural Sustainability, 20(7), 1373–1396. https://doi.org/10.1080/14735903.2022.2082764
- Musat, G.-A., Colezea, M., Pop, F., Negru, C., Mocanu, M., Esposito, C., & Castiglione, A. (2018). Advanced services for efficient management of smart farms. Journal of Parallel and Distributed Computing, 116, 3–17. https://doi.org/10.1016/j.jpdc.2017.10.017
- Naika, M. B. N., Kudari, M., Devi, M. S., Sadhu, D. S., & Sunagar, S. (2021). Chapter 8 - Digital extension service: Quick way to deliver agricultural information to the farmers. In C. M. Galanakis (Ed.), Food technology disruptions (pp. 285–323). Academic Press. https://doi.org/10.1016/B978-0-12-821470-1.00006-9
- Navarro-Hellín, H., Martínez-del-Rincon, J., Domingo-Miguel, R., Soto-Valles, F., & Torres-Sánchez, R. (2016). A decision support system for managing irrigation in agriculture. Computers and Electronics in Agriculture, 124, 121–131. https://doi.org/10.1016/j.compag.2016.04.003
- Oliver, S. T., González-Pérez, A., & Guijarro, J. H. (2018). An IoT proposal for monitoring vineyards called SEnviro for agriculture. Proceedings of the 8th International Conference on the Internet of Things, Santa Barbara, California, USA. https://doi.org/10.1145/3277593.3277625
- Pallottino, F., Figorilli, S., Cecchini, C., & Costa, C. (2021). Light drones for basic In-field phenotyping and precision farming applications: RGB tools based on image analysis. In P. Tripodi (Ed.), Crop breeding. Methods in molecular biology (Vol. 2264, pp. 269–278). Humana, New York, NY. https://doi.org/10.1007/978-1-0716-1201-9_18
- Pathak, H. S., Brown, P., & Best, T. (2019). A systematic literature review of the factors affecting the precision agriculture adoption process. Precision Agriculture, 20(6), 1292–1316. https://doi.org/10.1007/s11119-019-09653-x
- Pavón-Pulido, N., López-Riquelme, J. A., Torres, R., Morais, R., & Pastor, J. A. (2017). New trends in precision agriculture: A novel cloud-based system for enabling data storage and agricultural task planning and automation. Precision Agriculture, 18(6), 1038–1068. https://doi.org/10.1007/s11119-017-9532-7
- Popović, T., Latinović, N., Pešić, A., Zečević, Ž, Krstajić, B., & Djukanović, S. (2017). Architecting an IoT-enabled platform for precision agriculture and ecological monitoring: A case study. Computers and Electronics in Agriculture, 140, 255–265. https://doi.org/10.1016/j.compag.2017.06.008
- Pretty, J. (2018). Intensification for redesigned and sustainable agricultural systems. Science, 362(6417), Article eaav0294. https://doi.org/10.1126/science.aav0294
- Pretty, J., Attwood, S., Bawden, R., van den Berg, H., Bharucha, Z. P., Dixon, J., Flora, C. B., Gallagher, K., Genskow, K., Hartley, S. E., Ketelaar, J. W., Kiara, J. K., Kumar, V., Lu, Y., MacMillan, T., Maréchal, A., Morales-Abubakar, A. L., Noble, A., Prasad, P. V. V., … Yang, P. (2020). Assessment of the growth in social groups for sustainable agriculture and land management. Global Sustainability, 3, Article e23. https://doi.org/10.1017/sus.2020.19
- Raducu, I., Bojan, V., Pop, F., Mocanu, M., & Cristea, V. (2015). Real-time alert service for cyber-infrastructure environments. 2015 10th International Conference on P2P, Parallel, Grid, Cloud and Internet Computing (3PGCIC).
- Rajalakshmi, P., & Devi Mahalakshmi, S. (2016). IOT based crop-field monitoring and irrigation automation. 2016 10th International Conference on Intelligent Systems and Control (ISCO).
- Rettore de Araujo Zanella, A., da Silva, E., & Pessoa Albini, L. C. (2020). Security challenges to smart agriculture: Current state, key issues, and future directions. Array, 8, Article 100048. https://doi.org/10.1016/j.array.2020.100048
- Rossi, V., Salinari, F., Poni, S., Caffi, T., & Bettati, T. (2014). Addressing the implementation problem in agricultural decision support systems: The example of vite.net®. Computers and Electronics in Agriculture, 100, 88–99. https://doi.org/10.1016/j.compag.2013.10.011
- Silver, D., Huang, A., Maddison, C. J., Guez, A., Sifre, L., van den Driessche, G., Schrittwieser, J., Antonoglou, I., Panneershelvam, V., Lanctot, M., Dieleman, S., Grewe, D., Nham, J., Kalchbrenner, N., Sutskever, I., Lillicrap, T., Leach, M., Kavukcuoglu, K., Graepel, T., & Hassabis, D. (2016). Mastering the game of Go with deep neural networks and tree search. Nature, 529(7587), 484–489. https://doi.org/10.1038/nature16961
- Silver, D., Schrittwieser, J., Simonyan, K., Antonoglou, I., Huang, A., Guez, A., Hubert, T., Baker, L., Lai, M., Bolton, A., Chen, Y., Lillicrap, T., Hui, F., Sifre, L., van den Driessche, G., Graepel, T., & Hassabis, D. (2017). Mastering the game of Go without human knowledge. Nature, 550(7676), 354–359. https://doi.org/10.1038/nature24270
- Sinha, B., & Dhanalakshmi, R. (2022). Recent advancements and challenges of internet of things in smart agriculture: A survey. Future Generation Computer Systems, 126, 169–184. https://doi.org/10.1016/j.future.2021.08.006
- Steinke, J., van Etten, J., Müller, A., Ortiz-Crespo, B., van de Gevel, J., Silvestri, S., & Priebe, J. (2021). Tapping the full potential of the digital revolution for agricultural extension: An emerging innovation agenda. International Journal of Agricultural Sustainability, 19(5-6), 549–565. https://doi.org/10.1080/14735903.2020.1738754
- Su, Y., & Wang, X. (2021). Innovation of agricultural economic management in the process of constructing smart agriculture by big data. Sustainable Computing: Informatics and Systems, 31, Article 100579. https://doi.org/10.1016/j.suscom.2021.100579
- The Third National Agricultural Census Data Report. 2017. http://www.stats.gov.cn/english/pdf/202010/P020201012325158189120.pdf.
- Tran, M. Q., Phan, T., Takahashi, A., Thanh, T., Duy, S., Thanh, M., & Hong, C. (2017). A cost-effective smart farming system with knowledge base. https://doi.org/10.1145/3155133.3155151
- Trendov, N., Varas, S., & Zeng, M. (2018). Digital technologies in agriculture and rural areas status report. https://www.researchgate.net/publication/344041500.
- Trilles, S., Torres-Sospedra, J., Belmonte, Ó, Zarazaga-Soria, F. J., González-Pérez, A., & Huerta, J. (2020). Development of an open sensorized platform in a smart agriculture context: A vineyard support system for monitoring mildew disease. Sustainable Computing: Informatics and Systems, 28, Article 100309. https://doi.org/10.1016/j.suscom.2019.01.011
- Tsai, M.-C., Wang, J.-F., & Chen, Y.-T. (2021). Effect of social identity on supply chain technology adoption of small businesses. Asia Pacific Management Review, 26(3), 129–136. https://doi.org/10.1016/j.apmrv.2020.12.001
- Vandenburg, M. W. (1893). Nature's rotation of crops. Science, ns-22(549), 77–78. https://doi.org/10.1126/science.ns-22.549.77.c
- Wang, F.-Y. (2012). A big-data perspective on AI: Newton, Merton, and analytics intelligence. IEEE Intelligent Systems, 27(5), 2–4. https://doi.org/10.1109/MIS.2012.91
- Wang, F.-Y. (2015). CC 5.0:Intelligent command and control systems in the parallel AGE. Journal of Command & Control, 1(1), 107–120. http://www.jc2.org.cn/CN/Y2015/V1/I1/107
- Wang, G., Yin, J., Hossain, M. S., & Muhammad, G. (2021). Incentive mechanism for collaborative distributed learning in artificial intelligence of things. Future Generation Computer Systems, 125, 376–384. https://doi.org/10.1016/j.future.2021.06.015
- Wang, X., Kang, M., Fan, X.-R., Yang, L., Zhang, B.-G., Huang, S.-W., de Reffye, P., & Wang, F.-Y. (2020). What are the differences in yield formation among two cucumber (Cucumis sativus L.) cultivars and their F1 hybrid? Journal of Integrative Agriculture, 19(7), 1789–1801. https://doi.org/10.1016/S2095-3119(20)63218-X
- Wang, X., Kang, M., Sun, H., de Reffye, P., & Wang, F.-Y. (2022). DeCASA in AgriVerse: Parallel Agriculture for Smart Villages in Metaverses. IEEE/CAA Jounal of Automatica Sinica, 9(12), 2055–2062. https://doi.org/10.1109/JAS.2022.106103
- Weng, Y., Wang, X., Hua, J., Wang, H., & Kang, M. (2020). Greenhouse Environment Control based on Computational Experiments. Proceedings of the 2020 4th High Performance Computing and Cluster Technologies Conference & 2020 3rd International Conference on Big Data and Artificial Intelligence, Qingdao, China. https://doi.org/10.1145/3409501.3409518
- Weng, Y., Wang, X., Hua, J., Wang, H., Kang, M., & Wang, F.-Y. (2019). Forecasting horticultural products price using ARIMA model and neural network based on a large-scale data set collected by web crawler. IEEE Transactions on Computational Social Systems, 6(3), 547–553. https://doi.org/10.1109/TCSS.2019.2914499
- Yu, L., Qin, H., & Xiang, P.-A. (2020). Incentive mechanism of different agricultural models to agricultural technology information management system. Sustainable Computing: Informatics and Systems, 28, Article 100423. https://doi.org/10.1016/j.suscom.2020.100423
- Yuan, Y., Ni, X., Zeng, S., & Wang, F.-Y. (2016). Blockchain consensus algorithms: The state of the art and future trends. Acta Automatica Sinica, 44(11), 2011–2022. https://doi.org/10.16383/j.aas.2018.c180268
- Yuan, Y., Zhou, T., Zhou, A., Duan, Y., & Wang, F.-Y. (2017). Blockchain technology: From data intelligence to knowledge automation. IEEE/CAA Journal of Automatica Sinica, 43(9), 1485–1490. https://doi.org/10.16383/j.aas.c180710
- Zhang, Q., Chu, Y., Xue, Y., Ying, H., Chen, X., Zhao, Y., Ma, W., Ma, L., Zhang, J., Yin, Y., & Cui, Z. (2020). Outlook of China's agriculture transforming from smallholder operation to sustainable production. Global Food Security, 26, Article 100444. https://doi.org/10.1016/j.gfs.2020.100444
- Zhang, W., Cao, G., Li, X., Zhang, H., Wang, C., Liu, Q., Chen, X., Cui, Z., Shen, J., Jiang, R., Mi, G., Miao, Y., Zhang, F., & Dou, Z. (2016). Closing yield gaps in China by empowering smallholder farmers. Nature, 537(7622), 671–674. https://doi.org/10.1038/nature19368
- Zhu, J., Wang, F.-Y., Wang, G., Tian, Y.-L., Yuan, Y., Wang, X., Qi, H.-W., & Jia, X.-F. (2021). Federated control: A distributed control approach towards information security and rights protection. Acta Automatica Sinica, 47(8), 1912–1920. https://doi.org/10.16383/j.aas.c210182