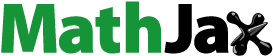
ABSTRACT
Low-carbon agricultural practices have received increasing attention, and the rapid development of agricultural product quality certification in China has provided a potential way to implement low-carbon agriculture. This study employs an endogenous switching regession to examine the effects of quality certification on low-carbon production behaviors using the survey data of 1,042 rice farmers in Hubei Province, China. The empirical results show that, first, the quality certification participation has a positive and statistically significant impact on farmers’ low-carbon production behaviors. In particular, the treatment effect of quality certification participation is to increase farmers’ low-carbon production level to appropriately 71.85%. Second, a disaggregated analysis by quality certification type reveals no significant difference between public geographical indication certification and private green food and organic product certification in promoting farmers’ low-carbon production behaviors. Nevertheless, the effect of multiple certifications on low-carbon production behaviors is significantly stronger than that of single certification. Third, disaggregated analysis indicates that new agricultural business entities are more likely to implement low-carbon production after participating in quality certification than traditional farmers.
1. Introduction
Climate change exacerbated by carbon emissions has become a global environmental problem that restricts the development of human society and economy (IPCC, Citation2021; Hong et al., Citation2021). Agricultural production has emitted large amounts of greenhouse gases such as carbon dioxide (Tian & Yin, Citation2022), and agricultural low-carbon production behavior can effectively reduce agricultural carbon emissions (Rakotovao et al., Citation2017; Haggar et al., Citation2017). Low-carbon production behaviors represent technologies that aim to reduce agricultural carbon emissions and protect the environment (Rakotovao et al., Citation2017), including increasing green inputs before production, innovating agricultural green production methods during production, and promoting waste resource utilization after production (Jiang et al., Citation2018; Li et al., Citation2021). Practice and theory prove that low-carbon production behavior can reduce CO2 emissions not only by reducing damage to soil structure, but also by processing waste into fertilizer, feed, and energy (Zhao et al., Citation2022). To promote low carbon production in agriculture, the Chinese government has formulated a series of policies to promote effective and ecologically efficient rice varieties, implement farmland conservation tillage, reduce fertilizer production to increase efficiency, and encourage the comprehensive utilization of crop straw.Footnote1 However, as implementers and stakeholders of low-carbon production, small farmers have not responded positively to these policies (Li et al., Citation2021). For example, He et al. (Citation2021) showed that the average adoption rate of low carbon production technologies, including water-saving irrigation, straw crushing and returning, deep fertilization, and mechanized deep-tillage and no-tillage sowing is less than 20%. Therefore, it is crucial to effectively motivate farmers to implement low-carbon production behavior.
The rapid development of quality certification has provided farmers a potential way to implement low-carbon production behavior. At present, the Chinese government emphasizes the establishment of a product quality certification system represented by green food certification, organic product certification, and agro-product geographical indications.Footnote2 As of 2018, the number of products certified in China has reached 121,743. Implementation of certified production serves two purposes. The first is to promote green production by strictly controlling agricultural production factor inputs at source. The second is to enhance the market competitiveness of high-quality agricultural products and promote farmers’ income growth (Haggar et al., Citation2017; Sellare et al., Citation2020). Hence, under the requirement of quality certification to limit production factor inputs and improve the quality of agricultural products, farmers inevitably adopt low-carbon production behaviors. Nevertheless, this view is not universally supported. Some studies found that some farmers would increase the use of chemical inputs with the gimmick of certified production to seek higher returns due to the imperfect supervision mechanism in the agricultural production process in China (Defries et al., Citation2017; Cheng & Zheng, Citation2018). Rice has a long cultivation history in China and is one of the main food crops for the country’s residents. However, rice produces vast amounts of greenhouse gases and represents a significant part of world’s greenhouse gas emissions (Zhao et al., Citation2022). It is imperative for the rice industry to development low-carbon rice production. Meanwhile, the amount of rice products under quality certification in China totaled 7,220 in 2021, accounting for 17.5% of the total certified agricultural products. To date, it is still not clear whether quality certification leads to improved low carbon production behaviors in rice production among farmers, particularly when taking both positive and negative effects into account.
The above differences might arise because the existing studies have generally ignored the type differences of quality certification and the heterogeneity of farmers. Actually, farmers can participate in any one or a combination of green food certification, organic product certification, and agro-product geographical indications (Vanderhaegen et al., Citation2018). Quality certification heterogeneity determines the input intensity, premium level, and quality standard of the means of production (Li et al., Citation2021), which could affect farmers’ decision-making on low-carbon agricultural production. For example, Li and Lu (Citation2020) revealed that organic product certification promotes farmers’ low-carbon production more significantly than the effect of green food certification and agro-product geographical indications. Meanwhile, farmers participating in the same type of quality certification might face different endowment conditions, and the effect of quality certification on low-carbon production varies due to the farmer type heterogeneity. For example, Yang and Chen (Citation2016) showed that new agricultural business entities, compared with traditional farmers, might ignore ecological and environmental issues for pursuing economic interests, thus inhibiting their low-carbon production. Thus, investigation of the relationship between quality certification and farmers’ low-carbon production behaviors must consider quality certification types and farmers’ heterogeneity.
The critical review above reveals the need to address three crucial questions regarding the applications of low-carbon production behaviors: (1) Does quality certification motivate farmers to apply low-carbon production in the entire rice production process? (2) Is there a difference in the impact of various types and combinations of quality certification on farmers’ low-carbon production behaviors? (3) Is there any difference in the impact of quality certification on the low-carbon production behaviors of different types of farmers? To answer these questions, this study uses the endogenous switching regression (ESR) model to empirically estimate the average treatment effect of quality certification on farmers’ low-carbon production behaviors in the context of rice production in China. This study further explores the difference in the impact from the perspective of quality certification types and farmers’ heterogeneity.
We make two significant contributions to the literature on farmers’ low-carbon production behaviors. First, the existing discussions on quality certification and agricultural low-carbon production behavior are mainly qualitative or apply quality certification as the control variable, and no consistent conclusion has been drawn. To the best of our knowledge, this is the first attempt to place product quality certification and farmers’ low-carbon production behavior into the same analytical framework and empirically reveal the relationship between them. Unlike previous studies that only estimate the homogenous quality certification and farmer types, our heterogeneous effect estimations could provide more valuable information that policy makers can use to design appropriate policies aimed at guiding different farmers to participate in different quality certification. Second, some studies have explored the relationship between quality certification and farmers’ low-carbon production behaviors without considering when farmers select themselves (self-selection) as quality certification adopters or non-adopters. The existing research has also ignored the endogenous problem of mutual causation between quality certification and low-carbon production behaviors. We employ an endogenous switching regression (ESR) model and multiple test methods to address the selection bias and endogenous issues, thereby making the results robust and effective.
2. Theoretical framework
Compared to traditional production behaviors, low-carbon production behaviors exhibit high adoption costs and outstanding overall benefits. Firstly, low-carbon production behaviors are more diverse and complicated to operate, and farmers need to spend energy and money to apply them (Liang et al., Citation2021; Anugwa et al., Citation2022), even facing the risk of yield reduction from improper application (Marteya & Kuwornu, Citation2021). Secondly, a bundle of low-carbon production behaviors can maximize the quality of products and premium income while protecting the agroecological environment through carbon sequestration and emission reduction, such as soil and water conservation technology, integrated pest management practices, chemical fertilizer alternative technologies, and straw return (Brako et al., Citation2021; He et al., Citation2021; Zhao et al., Citation2022; Kumar et al., Citation2023). This suggests that farmers might consider the costs and benefits of adoption when making decisions, thus affecting their low-carbon production. However, it is difficult for farmers to minimize the cost or maximize the benefits of low-carbon production behavior with limited resource endowment (Defries et al., Citation2017).
We argue that agricultural quality certification can optimize the costs and benefits of implementing low-carbon production behaviors through organizational support, price premium, and quality regulation (Sellare et al., Citation2020; Brako et al., Citation2021; Vinholis et al., Citation2021), thus driving farmers to implement low-carbon agricultural production. The mechanism of quality certification on farmers’ low-carbon production comprises three paths (see ). The first path highlights the importance of organizational support. Farmers who participate in quality certification have more access to technical training and production materials provided by the certification organization (Zhang & Huang, Citation2022). Among them, technical training can increase farmers’ knowledge about the comprehensive benefits of low-carbon production behaviors. Material supply (e.g. free access to high-quality seeds and uniform purchase of organic fertilizers) can reduce the information-seeking and material costs of implementing low-carbon production (Brako et al., Citation2021), thus promoting low-carbon agricultural production. The second path underscores the role of the price premiums. Quality certification, with the function of information collection and authoritative disclosure, enhances consumers’ trust and willingness to pay a premium for low-carbon agricultural products. Compared to ordinary products, certified products have a price advantage (Haggar et al., Citation2017) and significant price premiums due to better quality (Kumar et al., Citation2023). Therefore, farmers participating in quality certification have sufficient economic incentives to adopt low carbon production behavior (Ssebunya et al., Citation2019). Moreover, to maintain the long-term reputation of certified products and maintain sales channels, farmers are willing to increase long-term investments in low-carbon production practices (e.g. conservation tillage) (Makate et al., Citation2019). The third path stresses the advantages of quality supervision. When farmers’ product can't meet the requirements of certification organization, they face difficulty obtaining a premium price (Li & Lu, Citation2020). To guarantee product quality and prevent the opportunistic behavior of farmers selling an inferior product, the quality certification organization might use process supervision and product supervision to monitor farmers’ production. Furthermore, the process supervision directly controls farmers’ inputs of pesticides and fertilizers at the source. The product supervision (e.g. quality grading, quality testing and product traceability systems) also pushes farmers toward green and low-carbon production (Vinholis et al., Citation2021).
3. Data and descriptive statistics
3.1 Data
The data for this study came from a questionnaire survey conducted between July to August 2022 among rice farmers in Hubei Province. Located in central China, Hubei Province is one of the major rice production regions in China. In 2021, the total rice planting area and rice yield in this area accounted for 7.60% and 8.85% of China’s total rice planting area and rice yield, respectively. In addition, the total agricultural carbon emissions of Hubei Province ranked second in China in 2019 (Tian & Yin, Citation2022), which mainly originated from agriculture rice planting (45%). For these reasons, we selected the main rice producing areas in Hubei Province as the survey area for this study. We used a multistage random sampling technique to select sample households. Firstly, we limited our survey sample to five cities in Hubei Province: Xiangyang, Jingmen, Qianjiang, Xiantao, and Jingzhou. All five cities have achieved certain levels of rice quality certification development. Hubei Province focuses on the development of agricultural product quality certification, having built 28 provincial demonstrative bases. Xiangyang and Jingmen are cities with more demonstration bases in the single-season rice regions, and Qianjiang, Xiantao, and Jingzhou are cities with more certification bases in single-and-double-crop rice regions (see ). Secondly, one to two counties were randomly selected in each city. Qianjiang is contiguous to Xiantao, and the two counties share similar agricultural production and socio-economic conditions. Hence, only one county is selected in these two cities. Two sample counties are selected in other cities: Nanzhang and Xiangzhou counties in Xiangyang city, Jingshan and Zhongxiang counties in Jingmen city, and Jianli and Shishou counties in Jingzhou city. Thirdly, based on the relevant data from the statistical yearbooks of each county, three towns were randomly selected from the three levels of high, middle, and low economic development levels. Fourthly, two to four villages in each county were randomly selected for data collection. Finally, approximately ten to twenty households in each village were randomly selected.
According to the above sampling method, we completed a survey of 59 villages, resulting in a total sample of 1,047 households. A total of 1,042 valid samples were obtained in this study, after eliminating the questionnaires with missing key information and outliers. Four criteria were used to overcome measurement and understanding biases in the survey. First, we adjusted the questionnaire content and optimized the sample area selection based on the pre-test survey feedback. A baseline survey that consists of low-carbon agricultural production behavior and quality certification participation behavior was conducted among 958 farmers in these five cities in 2021. The survey showed significant differences in farmers’ understandings of low-carbon production and in the development of quality certification in different regions. Therefore, we modified the questionnaire items. The final questionnaire content mainly included the basic information of farmers, agricultural production and management, participation in quality certification, low carbon technology adoption behaviors, and basic conditions of the village. Meanwhile, based on the implementation of quality certification in the survey area, we randomly selected certified and non-certified farmers among them to conduct a questionnaire. Second, before the formal interview, several professional trainings were conducted with the postgraduates conducting face-to-face interviews with sample households. Third, the interviewees were introduced to agricultural emission reduction policies in China and informed that this survey is only for academic research before the interview. We also prepared a 20-yuan misuse allowance to reduce non-reflection bias. Fourth, we promptly displayed relevant pictures and videos related to low-carbon agricultural production behavior to the interviewees in the survey to enable them to understand the questionnaire content and improve the accuracy of their answers.
In terms of the basic characteristics of sample farmers (see Appendix ), the respondents are mainly men (84.64%), aged 44 years old to 73 years old (87.04%), and 83.68% of them received a junior high school education or below. This is reflected in the male-dominated household decision-making, a high degree of aging, and low education. These characteristics are basically consistent with the current reality in rural China. Family characteristics indicated that the family size is generally in the range of 3 to 6 people (67.37%). The household labor force is mainly 3 or 4 people (61.32%), with an average of 3.28. Of the sample households, 55.07% have an annual income of less than 100,000 yuan. In addition, the sample data calculations of per capita income of household indicate 27,000 yuan. Dates from Hubei Province Rural Statistical Yearbook show that, the average labor force per household in 2021 is 2.12, and per capita income of household is 24,500 yuan. The sample data is basically in accordance with the official data, indicating that the samples are representative. In addition, 45.59% of households transferred to land. The household farmland area of the interviewees is relatively large, with 66.47% of households more than 10 mu. These figures deviate from the per capita arable land area of 7.81 mu in Hubei Province. However, since the study area belongs to the high-quality rice sector in Hubei Province and the transfer of land is relatively common, the larger per capita arable land area is also consistent with reality.
3.2 Measurement of key variables
This study adopts a counterfactual hypothesis to analyze the treatment effect of quality certification on farmers’ low-carbon production behavior, so product quality certification is set as a dummy variable. Based on the study by Li and Lu (Citation2020), if the rice cultivated and sold by sample farmers has obtained at least one quality certification in green food certification, organic product certification, and agro-product geographical indications certification, the value is 1 (as the treatment group). If the sample farmer is not certified, a value of 0 is assigned as the control group.
Regarding the measurement of farmers' low-carbon production behaviors, studies have established different measurement categories and quantitative methods according to the research topics (Liang et al., Citation2021; Li et al., Citation2021; Anugwa et al., Citation2022). The low-carbon production behavior of farmers in this study refers to the production behavior of 'low energy consumption, low pollution, and low emission' . Under the premise of basically not affecting rice yield and production efficiency, rice farmers can reduce the production and emission of methane and nitrous oxide in the paddy field through the use of green inputs before production, innovation of green technology methods during production, and resource utilization of agricultural waste after production. With reference to the study by Jiang et al. (Citation2018), we select two low-carbon rice production methods each before, during, and after the rice production process, which constitute a subset of farmers' low-carbon production behavior. The six low-carbon production methods include the use of water-saving and drought-resistant rice varieties; conservation tillage such as less tillage or no-tillage of paddy fields; the use of soil formula fertilizer, organic fertilizer, and green manure; the use of pest control technology by biological and physical method (e.g. biopesticide, natural enemies, insect prevention net, insect trap plate); rice straw crushing and returning to the field; and agricultural film recycling. In order to quantify farmers’ low-carbon production behaviors as a whole, this study uses the comprehensive value of the sum of the number of farmers’ low carbon production behaviors to measure.
This study comprehensively considers the influence of internal characteristics and external factors and draws on previous studies (Tran & Goto, Citation2019; Ssebunya et al., Citation2019; Li & Lu, Citation2020). Fourteen variables in the four categories of individual characteristics, household characteristics, management characteristics, regional control variables, and one instrumental variable were selected as control variables. The individual characteristics include age, education, risk preference, and environmental awareness. Household characteristics include household size, agricultural income, and distance to market. Management characteristics include farm size, soil fertility, productive assets, and technical training. We use regional control variables to control regional-fixed effects such as climatic conditions and social culture.
The instrumental variable is the demonstration village, that is, whether the village belongs to the 'One village, one product' demonstration village in China or Hubei Province. We chose this variable as an instrumental variable because, as a prerequisite for farmers to participate in quality certification, the local organization must have obtained the qualification of quality certification. Agricultural products in the 'One village, one product' demonstration village have a greater probability of obtaining quality certification. Therefore, the demonstration village largely determines farmers’ participation decision-making in the quality certification. Moreover, the factors at the village level are less affected by the individual characteristics and family characteristics of farmers. In addition, the construction of the 'One village, one product' demonstration village has no direct impact on individual farmers’ low-carbon production behavior. Therefore, whether the village is a demonstration village can well satisfy the correlation and exogenesis characteristics of the instrumental variables. The definition of each variable and the descriptive statistical results are shown in .
Table 1. Definition and summary statistics of selected variables.
3.3 Model setting
We assume that the outcome variable (low-carbon production behavior) is a linear function of quality certification () and other explanatory variables (
) as follows:
(1)
(1) where
is the low-carbon production behavior farmer
.
is a variable indicating farmers’ quality certification participation decision.
is a vector of exogenous variables that we vary to investigate the reliability of our estimates in various ways, as explained further below.
is the random disturbance term.
、
are the parameters to be estimated.
In EquationEquation (1(1)
(1) ), the impact of quality certification on low-carbon production behaviors is captured by the parameter
. If the variable representing the quality certification (
) is exogenously determined, we employ an ordinary least square (OLS) regression approach to estimate EquationEquation (1
(1)
(1) ). However, farmers’ quality certification participation decision is not a random assignment but the optimal choice made by farmers under various constraints. This implies that farmers’ quality certification participation decisions exhibit characteristics of self-selection. Some unobservable factors also affect quality certification participation decisions and low-carbon production application behaviors, such as farmers’ personal capabilities. If the problem is ignored, the result is biased. In order to address the self-selection issue caused by observable and unobservable factors, this study refers to Maddala (Citation1983). We use the endogenous switching regression (ESR) model to empirically analyze the impact of quality certification on farmers’ low-carbon production behaviors. This approach has four significant advantages: (1) it can solve the self-selection issue of quality certification participation decision and endogenous problem, considering the effect of observable factors and unobservable factors simultaneously; (2) it helps identify factors that affect low-carbon production behaviors for quality certification participants and non-participants; (3) it employs the full information maximum likelihood method to deal with the problem of effective information omission; and (4) It realizes a counterfactual analysis.
The model is divided into two stages. In the first stage, the determinants of quality certification participation behavior, which are captured by EquationEquation (2(2)
(2) ), are investigated by estimating a probit model. Specifically, the expected utilities of adopters of quality certification (
) and nonadopters of quality certification (
) are compared. Farmers choose to participate in quality certification only if the utility from adopting is strictly greater than the utility from not adopting it, or mathematically,
. Since these two utilities are unobservable, observation of the choice made by the i-th household is impossible. However, these utilities can be expressed as a function of observable elements as:
(2)
(2) In EquationEquation (2
(2)
(2) ),
represents a binary selection variable indicating whether farmers participate in quality certification.
is the relevant factor that affects the quality certification participation behavior.
is the random disturbance item.
、
are the parameters to be estimated, and
is the instrumental variable to ensure the identifiability of the ESR model. The instrumental variable (IV) in this study represents whether the village where the farmer lives is a 'one village, one product' demonstration village. The reason for choosing this IV is that demonstration villages only affect farmers’ quality certification participation decisions but do not directly affect their low-carbon production behavior.
In the second stage, the determinants of the outcome variables (low-carbon production behavior) are regressed separately for quality certification adopters and non-adopters, as shown in Equations (Equation3(3)
(3) ) and (Equation4
(4)
(4) ).
(3)
(3)
(4)
(4) In Equations (Equation3
(3)
(3) ) and (Equation4
(4)
(4) ),
and
are outcome variables representing low-carbon production behavior for quality certification participants and non-participants, respectively.
and
refer to a vector of exogenous variables that affect farmers’ low-carbon production application behavior.
、
are the coefficients associated with a set of explanatory variables;
and
are error terms.
To estimate the treatment effects of quality certification participation on farmers’ low-carbon production behaviors, some further calculations are required. Within the ESR framework, the treatment effects can be calculated by comparing the expected values of outcome variables for quality certification participants with the expected values of the outcome variables for those who did not participate. Specifically, in the observable scenario, the expected values of the outcome variables for quality certification participants can be expressed as follows:
(5)
(5) In the counterfactual scenario, the expected values of the outcome variables for quality certification participants, had they not adopted, can be specified as:
(6)
(6) Thus, the average treatment effects on the treated (ATT), which is the treatment effect of quality certification participation on farmer’ low-carbon production behavior, can be derived by calculating the difference of outcomes between Equations (Equation5
(5)
(5) ) and (Equation6
(6)
(6) ):
(7)
(7)
3.4 Descriptive statistics
According to Appendix , 76.76% of farmers believe that low carbon production is needed in agricultural production, while 79.46% of farmers believe that adopting low-carbon production behavior can protect the environment. Figure A1 in the appendix shows the distribution of farmers with different levels of adoption of low-carbon production behaviors. The results showed that the proportion of 'not adopted', ‘adopted 1 item', ‘adopted 2 items', ‘adopted 3 items', ‘adopted 4 items', ‘adopted 5 items’ and 'adopted 6 items’ in the low-carbon production behaviors of sample farmers were 1.06%, 17.27%, 30.61%, 29.27%, 16.89%, 4.32%, and 0.58%, respectively. Almost all farmers in our sample adopt at least one of low-carbon production behaviors and the implementation of the policy of low-carbon production by the Chinese government has achieved certain results. Among the six categories of low carbon production behavior (see Appendix Figure A2), farmers mainly implemented crushing and returning rice straw to the field (92.99%), preventing plant disease and insect pests using biological and physical methods (41.46%) and with soil formula fertilizer, organic fertilizer, and green fertilizer (40.60%). In addition, the average farmer adopts 43.15%Footnote3 of the mitigation measures included in the survey. This indicates that the adoption degree of low-carbon production behavior of sample farmers should be further improved.
As shown in , 65.26% of rice farmers in the survey sample did not participate in quality certification, and only 34.74% of farmers participated in quality certification. Moreover, presents that 24.76%, 23.22%, and 7.97% of the total sample farmers participated in geographical indication certification, green food certification, and organic product certification, respectively. This means that rice quality certification is being carried out step by step in China's agricultural production practice. However, the overall level is still low, based on the low participation in quality certification. In addition, the proportion of geographical indication certification and green food certification is higher than that of organic product certification.
Table 2. Analysis of different types of quality certification and farmers’ low-carbon production behavior.
also reports the mean differences in the characteristics between quality certification participants and non-participants. Compared to non-participants, those who engage in quality certification tended to have higher levels of education, greater awareness of risk preference and low-carbon agricultural production, more family agricultural income, larger farm size, better soil fertility, more productive assets, and more technical training. The table also reveals that the average adoption of low-carbon production behaviors for quality certification participants is higher than those for non-participants. In addition, the statistical results in columns 5 and 6 of show that the number of low-carbon production behaviors adopted by farmers participating in geographical indication certification, green food certification, and organic product certification is 2.938, 3.003, and 3.530, respectively, which is significantly higher than those farmers without corresponding certification. This is a preliminary indication that quality certification can motivate farmers to adopt low-carbon production behaviors. However, simple descriptive statistics could not be interpreted as the treatment effects of quality certification, as they did not account for confounding factors. It is also necessary to fully consider the problems of self-selection and endogeneity of samples. Thus, a more scientific ESR model will be applied in the following paper for empirical testing.
4. Empirical results
4.1 Simultaneous estimation results of quality certification on farmers’ low-carbon production behavior
reports the results of the simultaneous estimation of farmers’ quality certification participation decision-making and farmers’ low-carbon production behavior implementation decision-making. The Wald test rejects the null hypothesis at the 1% level that the choice equation and the outcome equation are independent of each other. and
are the correlation coefficients of the error terms of the decision-making models pertaining to both quality certification participation and the low-carbon production behavior of farmers who participate and do not participate, respectively. The estimates of both correlation coefficients are statistically significant, indicating that there is indeed a sample selection bias in the decision of farmers to participate in quality certification, which should be corrected. These findings confirm the appropriateness of using the ESR model in this study.
Table 3. Joint estimation results of farmers’ quality certification participation decision-making model and farmers’ low-carbon production behavior model.
4.1.1 Estimation results of farmers’ quality certification participation decision model
The results of the selection equation in show that age has a significant positive impact on farmers’ participation in quality certification decision-making. Older farmers have limited energy in rice production management and product marketing. They are more willing to participate in quality certification to obtain technical guidance services and premium acquisition services. Risk is an important factor affecting farmers’ agricultural production decisions (Paudel et al., Citation2000). The probability of farmers with risk preference who participate in agricultural product quality certification is higher than those who don’t, which is in line with the results by Zhang and Huang (Citation2022). Environmental awareness has a significant positive impact on farmers’ participation in quality certification. Farmers with stronger environmental awareness are more willing to send quality signals through participation in quality certification to achieve an income increase (Vanderhaegen et al., Citation2018).
In addition, agricultural income significantly positively affects farmers’ participation in quality certification. Farmers with higher agricultural income are more dependent on agriculture, and they seek to achieve high quality and good prices through participation in quality certification. This is consistent with the research conclusion of Tran and Goto (Citation2019). Furthermore, farm size and soil fertility significantly positively affect farmers’ quality certification participation. Generally speaking, the size and quality of cultivated land reflect the natural capital owned by a family. The larger the farm size and the better the soil fertility, the more chances farmers have to obtain the channels for participation in quality certification and reach the participation threshold. The above results are basically consistent with the conclusions of Haggar et al. (Citation2017) and Ssebunya et al. (Citation2019). Technical training significantly positively affects farmers’ participation in quality certification decision-making. As pointed out by Li and Lu (Citation2020), trainers introduce farmers to high-quality agricultural development knowledge, which can enhance farmers’ awareness and ability, thus increasing their participation in quality certification.
In terms of instrumental variables, the results show that demonstration villages have a significant positive impact on farmers’ participation in quality certification. This means that farmers belonging to the 'one village, one product' demonstration villages are more likely to participate in the quality certification. We tested the validity of instrumental variables. Durbin-Wu-Hausman’s endogeneity test result is 20.655, thus rejecting the hypothesis that quality certification is an exogenous variable. This means quality certification is an endogenous variable. The value of Cragg-Donald Wald F statistic is 44.515, thereby rejecting the hypothesis of weak instrumental variables. In addition, the Pearson correlation coefficient shows that instrumental variables are significantly related to quality certification participation decision. Moreover, the results of the selection equation show that the instrumental variables have a significant impact on quality certification, which indicates that the instrumental variables satisfy the correlation condition. Therefore, it is appropriate to choose the 'one village, one product' demonstration village as the instrumental variable in this study.
4.1.2 Estimation results of farmers’ low-carbon production behavior model
Education has a significant negative effect on the low-carbon production behavior for quality certification participants. This result contradicts the findings of Anugwa et al. (Citation2022). In contrast, education has a spillover effect. Farmers with higher education have more off-farm employment opportunities and higher incomes, thus increasing the opportunity cost of farmers engaged in agricultural production (Scherr & Hazell, Citation1994). Therefore, farmers participating in quality certification will not choose to invest more time and energy in low-carbon agricultural production, especially low-carbon technologies with labor-intensive characteristics (Liang et al., Citation2021). The positive and significant coefficient of the risk preference variable suggests that an increase in the risk preference level tends to increase low-carbon production behavior among quality certification participants and non-participants. Rice production itself has high natural risks, and the application of low carbon technology may also pose a risk of cost increase and yield reduction. Therefore, both our study and that of Meraner and Finger (Citation2019) assert that farmers with risk preference have a higher probability of applying low-carbon agricultural production. Environmental awareness has a significant positive effect on low carbon production behavior for quality certification participants. The higher the environmental protection awareness of farmers participating in quality certification, the more willing they will be to actively adopt low-carbon rice production and then adopt them as an internal response (Yang et al., Citation2022). Jiang et al. (Citation2018) and Kreft et al. (Citation2021) also drew similar conclusions.
Distance to market has a significant positive effect on the low-carbon production behavior for quality certification participants. This is because farmers usually choose to purchase materials/inputs from the nearest market of the village, which is more likely to reduce fuel-based agricultural inputs to minimize transaction costs (Makate et al., Citation2019). In addition, the closer the farmers are to the market, the more technical services they can get from the agricultural technology extension service center, which is necessary for technology adoption, given that low-carbon agricultural production is a knowledge-based innovation (Li et al., Citation2021).
Farm size is a significant and positive predictor of applying low-carbon production behaviors for both participants and non-participants. This could be because farmers with large farms find it difficult to achieve 'intensive cultivation' of rice under the condition of limited family labor force and even need to spend more money to hire a labor force (Marteya & Kuwornu, Citation2021). Thus, when other conditions remain unchanged, farmers tend to increase the inputs of pesticides, fertilizers, and other factors to make up for the lack of labor force, resulting in low enthusiasm for low-carbon production. Soil fertility also has a significant positive effect on the low-carbon production behavior for quality certification participants. However, productive assets have a significant positive impact on the low-carbon production behavior for quality certification non-participants. The quantity and quality of production factors such as land, agricultural machinery, and tools are important factors affecting farmers’ decision-making on agricultural production behavior (Li et al., Citation2021). Farmers participating in quality certification are more concerned about land productivity, and the better the land quality is, the more willing they are to adopt low-carbon production behaviors (Marteya & Kuwornu, Citation2021).
4.2 Treatment effect of quality certification on farmers’ low-carbon production behavior
reports the average treatment effect of participation in quality certification on farmers’ low-carbon production behavior. The results show that quality certification has a positive effect on farmers’ low-carbon production behavior, which is statistically significant. Among them, the ATT estimates show that if farmers who previously participated in quality certification do not continue, their low-carbon production level decreases by 71.85%. Moreover, the ATU estimates show that, if farmers who do not participate in quality certification can participate in quality certification, their agricultural low-carbon production level will increase from 2.392–4.116 by about 72.07%. In general, quality certification plays an important role in promoting the adoption of low-carbon production behaviors among farmers.
Table 4. Average treatment effect of farmers’ participation in quality certification on low-carbon production behavior.
4.3 Robustness test
To check the robustness of the estimation, we further use different methods to estimate the impact of quality certification on low-carbon production behaviors. Firstly, the propensity score matching method (PSM) and the treatment effects model (TEM) were used to explore the effects of quality certification on farmers’ low-carbon production behavior. The PSM method is one of the quasi-natural experimental methods widely used by scholars to solve the problem of sample selective bias. In this study, the standardization bias of independent variables except soil fertility decreased significantly after matching with various means. This indicates that the PSM method can effectively reduce the standardization bias, basically achieving the effect of the randomized experiment. in the Appendix reports the results of propensity score matching on the impact of quality certification on farmers’ low-carbon production behavior. The ATT values of nearest neighbor matching, caliper matching, and kernel matching are respectively 0.797, 0.836, and 0.856. In other words, quality certification participation can significantly improve the probability of farmers adopting low-carbon production behavior, and the increase range is 79.7%–85.6%. In addition, like the ESR model, TEM addresses the selection bias generated by both observable and unobservable factors (Ma et al., Citation2020). Another advantage of the treatment effects model is that it estimates the direct impact of quality certification on farmers’ low-carbon production behavior. The results in the last column of also indicate that quality certification has a significant positive impact on farmers’ low-carbon production behavior.
Secondly, the robustness test is carried out by replacing the explained variables. The virtual variable, which is converted from the comprehensive value of farmers’ adoption of low-carbon production behavior and the mean value of all samples, is used to measure low-carbon production behavior. A quantity of low-carbon production adopted by farmers that is greater than the sample mean (2.589) indicates that farmers have adopted low-carbon production behavior, and the value is 1. Otherwise, it is 0. Since the dependent variable is a binary dummy variable, we use the ESP model for estimation. The results in columns 2 and 3 of in the Appendix show that quality certification can improve the probability of farmers adopting low-carbon production behaviors. This also shows that the estimation results in this study are robust.
Finally, it is difficult for elderly farmers to participate in quality certification and the adoption of low-carbon technologies due to their physical and cognitive decline. In this paper, survey participants aged 75 and above were excluded according to the World Health Organization’s age classification standard for the elderly. Columns 4 and 5 of report the average treatment effect after the sample size adjustment. The results still suggest that quality certification can significantly increase the probability of farmers adopting low-carbon production behaviors. It is further verified that the estimation results in this paper are robust.
5. Discussion
5.1 Heterogeneity of different types of quality certification
Different types of quality certification differ in the input intensity of agricultural production and quality standard requirements, thus bringing different impacts on farmers’ low-carbon production behaviors. From the perspective of property right attribute, geographical indication certification is a public product certification with regional characteristics whose ownership is shared by the production subject in the region. Green food certification and organic product certification are independently applied by industrial organizations, including farming cooperatives and companies whose ownership belongs to the corresponding entities. Previous studies found that geographical indication certification is a 'quasi-public good' due to its non-exclusive or non-competitive characteristics (Li et al., Citation2021). Furthermore, unclear property rights may induce the 'free-rider' phenomenon of farmers’ low-carbon production behavior (Cheng & Zheng, Citation2018). Meanwhile, the descriptive results in show that the number of low carbon production adopted by farmers participating in geographical indication certification is slightly less than that of farmers participating in green food certification and organic product certification. Those findings imply the potential heterogeneous effects of different quality certifications on low-carbon production. Therefore, this study further divides rice quality certification into geographical indication certification and green food and organic product certification and explores their heterogeneous effects on low-carbon production behavior.
reports the results regarding the heterogeneous impacts of different quality certifications on farmers’ low-carbon production behavior. The geographical indication certification and green food and organic product certification have a positive and statistically significant impact on farmers’ low-carbon production behavior. The ATT estimates show that, if farmers who have participated in geographical indication certification and green food and organic product certification do not continue, their low-carbon production level decreases by 81.20% and 73.61%, respectively. The ATU estimates show that farmers without geographical indication certification and green food and organic product certification increase their adoption level of low-carbon production behavior by 68.68% and 81.54%, respectively, if they have the corresponding type of quality certification. Our results reflect no significant difference between geographical indication certification and green food and organic product certification in promoting farmers’ low-carbon production behavior, which is unlike the results of previous studies (e.g. Li & Lu, Citation2020; Zhang & Huang, Citation2022). On the one hand, rice geographical indication certification in Hubei Province is in its infancy. In order to launch the 'geographical name card', the local government has invested large technical support and quality supervision in the entire product quality chain. On the other hand, geographical indication certification has a typical reputation accumulation mechanism, and its product quality reputation has a long-term impact on farmers’ product sales. The reputation effect can stimulate producers’ sense of moral responsibility and reverse constrained farmers’ productive behaviors (Zhang & Huang, Citation2022). In addition, rice farmers are also willing to use green inputs, adopt green technologies, and recycle waste to achieve the long-term benefits from geographical indication certification (Vanderhaegen et al., Citation2018).
Table 5. Effects of different types of quality certifications on farmers’ low-carbon production behavior.
From the perspective of the number of quality certifications, farmers can apply multiple different certification schemes of geographical indication certification, green food certification, and organic product certification (Vanderhaegen et al., Citation2018). So, are multiple certifications more effective than a single certification in promoting low carbon production behavior? The heterogeneity effects based on quality certification number are presented in . The results show that the positive effects of single certification and multiple certifications are significant. ATT estimate results show that, if farmers participating in a single certification and multiple certifications do not participate in the corresponding quality certification, their adoption level of low-carbon production behavior will decrease by 63.08% and 82.00%, respectively. ATU estimates suggest that, if farmers without single and multiple certifications can participate in the corresponding quality certification, their adoption level of low-carbon production behavior will increase by 18.47% and 78.86%, respectively. Generally, the effect of multiple certifications on farmers’ low-carbon production behavior is stronger than that of a single certification, confirming that the impacts of different number of quality certification are heterogeneous.
5.2 Heterogeneity of traditional farmers and new agricultural business entities
With the acceleration of new industrialization, informatization, and urbanization, agricultural business subjects have gradually evolved into traditional farmers and new agricultural business entities. New agricultural business entities, based on family tenant management, are a production and operation organization form engaged in the specialization, intensification, and socialization of modern agriculture. It mainly includes four types: large and specialized family businesses, family farms, farmer cooperatives, and agribusinesses (Yang et al., Citation2021). Different types of farmers have great differences in low-carbon agricultural production (Azadi et al., Citation2015), and existing research has not reached a unified conclusion. The first strand of literature reveals that new agricultural business entities can effectively integrate agricultural resources and are more likely to participate in quality certification (Tran & Goto, Citation2019), especially in the organic product certification (Arunrat et al., Citation2022). Since the use of synthetic substances such as pesticides, chemical fertilizers, and hormones is prohibited in the production of organic products, agricultural business entities use high-quality information channels and strong financial support to select water-saving and drought-resistant rice varieties and adopt rice-fish co-culture and green manure. These measures increase soil carbon sequestration and reduce greenhouse gas emissions, thus achieving low-carbon production (Yang et al., Citation2021; Arunrat & Sereenonchai, Citation2022). The second smaller strand of literature shows that new agricultural business entities would never give up their economic benefits to protect the agricultural ecological environment, thus only adopting less low-carbon production behaviors (Yang & Chen, Citation2016). Therefore, this study further considers the heterogeneous effects of quality certification participation among farmers of different types. Referring to the existing literature (Yang et al., Citation2021), this study defines big-specialized households, family farms, agricultural professional cooperatives, and agricultural enterprises as new agricultural business entities, and vice versa for traditional farmers. As shown in , quality certification has a significant impact on low-carbon production behaviors for both new agricultural business entities and traditional farmers. According to the ATT, the effect value of low-carbon production behavior adopted by new agricultural business entities participating in quality certification is 2.231, much higher than that of traditional farmers (1.745). This means that the impact of quality certification on farmers’ low-carbon production behavior is heterogeneous due to the differences in household types. Moreover, the level of low-carbon production adopted by new agricultural business entities after quality certification is higher than that of traditional farmers.
Table 6. Average treatment effects of quality certification on low-carbon production behavior by selected types of agricultural business entity.
6. Conclusions and policy implications
This study focused on the relationship between quality certification and the low-carbon production behavior of rice farmers. The statistical results show that the average farmer adopts 43.15% of the low-carbon measures included in the survey, indicating that farmers’ low-carbon production level needs improvement. The results of the ESR model showed that quality certification can encourage farmers to adopt low-carbon production behaviors. In particular, the treatment effect of quality certification participation is to increase farmers’ low-carbon production level to appropriately 71.85%. In addition, after distinguishing quality certification types based on property rights attributes, there is no significant difference between geographical indication certification and green food and organic product certification in promoting farmers’ low-carbon production behavior. However, from the perspective of the number of quality certifications, the effect of multiple certifications on farmers’ low-carbon production behavior is significantly stronger than that of single certification. Furthermore, the impact of quality certification on farmers’ low-carbon production behavior is heterogeneous due to the differences in household types. The level of low-carbon production adopted by new agricultural business entities after quality certification is higher than that of traditional farmers. Additionally, we showed that farmers’ age, risk preference, environmental awareness, agricultural income, farm size, soil fertility, and technical training are the main factors that determine farmers’ participation decision regarding quality certification.
Implementation of quality certification and low-carbon production in the agricultural field also exist in other regions in China. Thus, our findings have important implications for policymakers in China. Firstly, we need to make good use of quality certification to help low-carbon agricultural production. The government should use information services and technical training to enhance farmers’ awareness of the benefits associated with quality certification and participation in quality certification, thus increasing farmers’ participation in low-carbon production. Secondly, different types of quality certification have heterogeneous impacts on agricultural low-carbon production behavior, which indicate that various types of quality certification should be developed collaboratively. In practice, each region should develop geographical indication certificates, green food certificates, and organic product certificates according to local conditions. Moreover, given the strong association between multiple certification and farmer’ low-carbon production behavior, there is a need to encourage farmers to participate in multiple certifications. Third, considering the heterogeneous effects of quality certification participation on low-carbon production behavior among farmers at different types, the government should engage in efforts to motivate farmers to participate in quality certification and encourage new agricultural business entities to play a leading role.
Standardized policies and measures for quality certification and low-carbon production behavior can reduce policy implementation costs, which helps promote these practices throughout China. However, it should still be noted that China is a vast and diverse country, and each region still needs to develop differentiated specific policies based on regional characteristics. In addition, our analysis is based on the cross-sectional data collected from 1,042 rice farmers in China. Due to data limitations, we are unable to capture the dynamic effects of quality certification on low-carbon production behavior. However, we believe this is a promising area to investigate in the future when the required panel data are available.
Acknowledgements
We are grateful to the members of the survey team who worked hard to collect the data.
Disclosure statement
No potential conflict of interest was reported by the author(s).
Data availability statement
The data that support the findings of this study are available from the corresponding author, Sanxia Du, upon reasonable request. E-mail: [email protected].
Additional information
Funding
Notes on contributors
Sanxia Du
Sanxia Du is a PhD student majored in agricultural economic management at the college of Economics & Management, Huazhong Agricultural University. Her research interests include low carbon agriculture, and pesticide reduction.
Xiaofeng Luo
Xiaofeng Luo is professor at the College of Economics & Management, Huazhong Agricultural University. His research interests include agricultural resources and environmental economy, agricultural policy analysis, and rural development. He has published more than 100 scientific articles, including Food policy, Pest management science, Environmental Science and Pollution Research.
Lin Tang
Lin Tang is lecturer at the School of Law and Business, Wuhan Institute of Technology. His research interests include agricultural resources and environmental economy, and rural environmental governance. He has published more than 16 scientific articles, including Food policy, Journal of Chinese Political Science, Environment, Development and Sustainability.
Aqian Yan
Aqian Yan is a PhD student majored in agricultural economic management at the college of Economics & Management, Huazhong Agricultural University. Her research interests include pesticide reduction and chemical fertilizer reduction.
Notes
1 Refer to the notice of the Implementation Plan for Carbon Reduction and Fixation in Agricultural and Rural Areas issued by the Ministry of Agriculture and Rural Affairs and the National Development and Reform Commission of China in June 2022 (http://www.moa.gov.cn/govpublic/KJJYS/202206/t20220630_6403715.htm).
2 Small farmers can obtain product quality certification for their agricultural products in two forms: (1) apply for certification directly, and (2) indirectly through the 'company+farmer' or 'cooperatives+farmer' to obtain.
3 The mean value of adoption of low-carbon production behaviors among farmers was 2.589, accounting for 43.15% of the overall six production behaviors.
References
- Anugwa, I. Q., Onwubuya, E. A., Chah, J. M., Abonyi, C. C., & Nduka, E. K. (2022). Farmers’ preferences and willingness to pay for climate-smart agricultural technologies on rice production in Nigeria. Climate Policy, 22(1), 112–131. https://doi.org/10.1080/14693062.2021.1953435
- Arunrat, N., & Sereenonchai, S. (2022). Assessing ecosystem services of rice–fish co-culture and rice monoculture in Thailand. Agronomy, 12(5), 1241. https://doi.org/10.3390/agronomy12051241
- Arunrat, N., Sereenonchai, S., Chaowiwat, W., Wang, C., & Hatano, R. (2022). Carbon, nitrogen and water footprints of organic rice and conventional rice production over 4 years of cultivation: A case study in the lower north of Thailand. Agronomy, 12(2), 380. https://doi.org/10.3390/agronomy12020380
- Azadi, H., Samiee, A., Mahmoudi, H., Jouzi, Z., Khachak, P. R., Maeyer, P. D., & Witlox, F. (2015). Genetically modified crops and small-scale farmers: main opportunities and challenges. Critical Reviews in Biotechnology, 36, 434–446.
- Brako, E. B., Richard, A., & Alexandros. (2021). Do voluntary certification standards improve yields and wellbeing? Evidence from oil palm and cocoa smallholders in Ghana. International Journal of Agricultural Sustainability, 19(1), 16–39. https://doi.org/10.1080/14735903.2020.1807893
- Cheng, J. X., & Zheng, S. F. (2018). Analysis of influence of government regulation on peasant households’ behavior based on regional brand agricultural product quality and safety. Journal of Northwest A&F University (Social Science Edition), 18, 115–122.
- Defries, R. S., Fanzo, J., Mondal, P., Remans, R., & Wood, S. A. (2017). Is voluntary certification of tropical agricultural commodities achieving sustainability goals for small-scale producers? a review of the evidence. Environmental Research Letters, 12(3), 0033001. https://doi.org/10.1088/1748-9326/aa625e
- Haggar, J., Soto, G., Casanoves, F., & Virginio, E. M. (2017). Environmental-economic benefits and trade-offs on sustainably certified coffee farms. Ecological Indicators, 79, 330–333. https://doi.org/10.1016/j.ecolind.2017.04.023
- He, K., Wang, H., & Zhang, J. B. (2022). Agricuiltral transformation path with respect to the target of carbon peak and carbon neutrality: from the market to the “market”. Journal of Huazhong Agricultural University (Social Science Edition), 1, 1–9. In Chinese.
- He, P. P., Zhang, J. B., & Li, W. J. (2021). The role of agricultural green production technologies in improving low-carbon efficiency in China: necessary but not effective. Journal of Environmental Management, 293, 112837.
- Hong, C. P., Burney, J. A., Pongratz, J., Nabel, J. E. M. S., Mueller, N. D., Jackson, R. B., & Davis, S. J. (2021). Global and regional drivers of land-use emissions in 1961-2017. Nature, 589(7843), 554–561. https://doi.org/10.1038/s41586-020-03138-y
- IPCC. (2021). Climate change 2021: The physical science basis, the working group I contribution to the sixth assessment report. Cambridge University Press.
- Jiang, L. L., Zhang, L., Zhang, J. B., & Wang, H. (2018). The influence mechanism of rice farmers’ low-carbon production behaviors: An analysis based on in-depth interviews with 102 rice farmers in Hubei Province. China Rural Survey, 4, 86–101. In Chinese.
- Kreft, C., Huber, R., Wuepper, D., & Finger, R. (2021). The role of non-cognitive skills in farmers’ adoption of climate change mitigation measures. Ecological Economics, 189, 107169. https://doi.org/10.1016/j.ecolecon.2021.107169
- Kumar, R., Kumar, S., Yashavanth, B. S., Venu, N., Meena, P. C., Dhandapani, A., & Kumar, A. (2023). Natural farming practices for chemical-free agriculture: implications for crop yield and profitability. Agriculture, 13(3), 647. https://doi.org/10.3390/agriculture13030647
- Li, D., Zhou, H., & Zhou, L. (2021a). Brand premium and the quality and safety of agricultural products: examples from rice planting in Jiangsu province. Journal of Finance and Economics, 47, 34–48. In Chinese.
- Li, H., & Lu, Q. (2020). Can product quality certification improve farmers’ technological efficiency? Chinese Rural Economy, 5, 128–144. In Chinese.
- Li, W. J., Ruiz-Menjivar, J., Zhang, L., & Zhang, J. B. (2021b). Climate change perceptions and the adoption of low-carbon agricultural technologies: Evidence from rice production systems in the Yangtze River Basin. Science of the Total Environment, 759, 143554. https://doi.org/10.1016/j.scitotenv.2020.143554
- Liang, Z. H., Zhang, L., Li, W. J., Zhang, J. B., & Frewer, L. J. (2021). Adoption of combinations of adaptive and mitigatory climate-smart agricultural practices and its impacts on rice yield and income: Empirical evidence from Hubei, China. Climate Risk Management, 32, 100314. https://doi.org/10.1016/j.crm.2021.100314
- Ma, W. L., Nie, P., Zhang, P., & Renwick, A. (2020). Impact of Internet use on economic well-being of rural households: Evidence from China. Review of Development Economics, 24(2), 503–523. https://doi.org/10.1111/rode.12645
- Maddala, G. S. (1983). Limited dependent and qualitative variables in econometrics. Cambridge University Press.
- Makate, C., Makate, M., Mango, N., & Siziba, S. (2019). Increasing resilience of smallholder farmers to climate change through multiple adoption of proven climate-smart agriculture innovations. Lessons from Southern Africa. Journal of Environmental Management, 231, 858–868.
- Marteya, E., & Kuwornu, J. K. M. (2021). Perceptions of climate variability and soil fertility management choices among smallholder farmers in Northern Ghana. Ecological Economics, 180, 106870. https://doi.org/10.1016/j.ecolecon.2020.106870
- Meraner, M., & Finger, R. (2019). Risk perceptions, preferences and management strategies: evidence from a case study using German livestock farmers. Journal of Risk Research, 1–26.
- Paudel, K. P., Lohr, L., & Martin, N. R. (2000). Effect of risk perspective on fertilizer choice by sharecroppers. Agricultural Systems, 66(2), 115–128. https://doi.org/10.1016/S0308-521X(00)00039-1
- Rakotovao, N. H., Razafimbelo, T. M., Rakotosamimanana, S., Randrianasolo, Z., Randriamalala, J. R., & Albrecht, A. (2017). Carbon footprint of smallholder farms in Central Madagascar: The integration of agroecological practices. Journal of Cleaner Production, 140, 1165–1175. https://doi.org/10.1016/j.jclepro.2016.10.045
- Scherr, S. J., & Hazell, P. B. R. (1994). Sustainable agricultural development strategies in fragile lands (No. 1). International Food Policy Research Institute (IFPRI).
- Sellare, J., Meemken, E. M., Kouamé, C., & Qaim, M. (2020). Do sustainability standards benefit smallholder farmers also when accounting for cooperative effects? Evidence from Côte d'Ivoire. American Journal of Agricultural Economics, 102(2), 681–695. https://doi.org/10.1002/ajae.12015
- Ssebunya, B. R., Morawetz, U. B., Schader, C., Stolze, M., & Schmid, E. (2019). Group membership and certification effects on incomes of coffee farmers in Uganda. European Review of Agricultural Economics, 46(1), 109–132. https://doi.org/10.1093/erae/jby022
- Tian, Y., & Yin, M. H. (2022). Re-evaluation of China’s agricultural carbon emissions: basic status, dynamic evolution and spatial spillover effects. Chinese Rural Economy, 3, 104–127. In Chinese.
- Tran, D., & Goto, D. (2019). Impacts of sustainability certification on farm income: evidence from small-scale specialty green tea farmers in Vietnam. Food Policy, 83, 70–82. https://doi.org/10.1016/j.foodpol.2018.11.006
- Vanderhaegen, K., Akoyi, K. T., Dekoninck, W., Jocqué, R., Muys, B., Verbist, B., & Maertens, M. (2018). Do private coffee standards ‘walk the talk’ in improving socio-economic and environmental sustainability? Global Environmental Change, 51, 1–9. https://doi.org/10.1016/j.gloenvcha.2018.04.014
- Vinholis, M., Saes, M., Carrer, M. J., & Filho, H. M. (2021). The effect of meso-institutions on adoption of sustainable agricultural technology: a case study of the Brazilian low carbon agriculture plan. Journal of Cleaner Production, 280, 124334. https://doi.org/10.1016/j.jclepro.2020.124334
- Yang, G., & Chen, Y. (2016). Design of the incentive mechanism that new agricultural operators participate in low-carbon agricultural development. China Population, Resources and Environment, 26, 94–99. In Chinese.
- Yang, X., Zhou, X. H., & Deng, X. H. (2022). Modeling farmers’ adoption of low-carbon agricultural technology in Jianghan Plain, China: An examination of the theory of planned behavior. Technological Forecasting and Social Change, 180, 121726. https://doi.org/10.1016/j.techfore.2022.121726
- Yang, X. J., Qi, Z. H., Yang, C. Y., & Liu, Z. (2021). Can the new type of agricultural management promote the promotion of ecological agriculture technologies: Taking rice and shrimp co-cultivation technology as an example. Resources and Environment in the Yangtze, 30(10), 2545–2556. In Chinese.
- Zhang, Y., & Huang, Y. Z. (2022). Research on the influence of geographical indication brand participation on the quality and safety of agricultural products. Journal of Huazhong Agricultural University (Social Sciences Edition), 5, 123–135. In Chinese.
- Zhao, M. J., Shi, R., & Yao, L. Y. (2022). Analysis on the goals and paths of carbon neutral agriculture in China. Journal of Agrotechnical Economics, 9, 24–34. In Chinese.
Appendices
Figure A1. Proportion distribution of farmers with different comprehensive values of low carbon production behaviors.

Table A1. Basic characteristics of the sample farmers.
Table A2. Farmers’ perception of low-carbon production behavior.
Table A3. Robustness test with PSM and TEM method.
Table A4. Robustness test of variable and sample replacement.