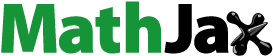
ABSTRACT
Rice production in Japan faces severe labour shortage; hence, cutting-edge technologies, such as agricultural unmanned aerial vehicle (UAV) sprayers, are being actively considered and tested for installation in agricultural settings by the government. However, the environmental impacts of agricultural UAV sprayers remain unclear. Here, we aimed to assess the environmental performance of agricultural UAV sprayers in pest control during rice production in Japan, by comparing the environmental impact during pest control by using UAV, power, and boom spraying. We performed life cycle analysis with SimaPro 8 using the ReCiPe 2016 Midpoint (H) methodology and carried out sensitivity analyses to estimate the influence of input variables on emissions as a function of operational area. UAV spraying exhibited a lower environmental impact than did boom- and power spraying – attributed to the weight and fuel consumption of boom and power spraying, which increase the environmental impact associated with material production and energy consumption. Pesticide input remained constant, while the required water volumes for pesticide dilution differed among the sprayers. These results suggest the use of lighter and energy-saving machinery to mitigate the environmental impact of pest control in rice production; UAV spraying was found to be a good alternative to conventional methods.
Introduction
Agricultural industry is faced by several challenges including harsh environmental conditions and increasing population (Juhola et al., Citation2017; Li et al., Citation2011). Sustainable agriculture is vital in the context of meeting the global food demand and working toward environmental sustainability. However, it is challenging to overhaul conventional agricultural systems, with methods including crop improvement, bio-mediated soil improvement, and the development of automated plant factories, considering artificial intelligence (AI), internet of things (IoT), robots, and drones. In recent times, such advanced technologies originally developed for indoor factory settings are now being applied to address the challenges of complex agricultural systems in open environments. Open fields pose distinct challenges characterized by unpredictable weather conditions, uneven and muddy terrain, and limited availability of reliable internet connectivity (Avgoustaki & Xydis, Citation2020; Engler & Krarti, Citation2021; O'Sullivan et al., Citation2019).
Rice production in Japan typically involves the passing on of rice-related cultural traditions through the generations. However, Japan faces severe labour shortage, with low numbers of younger generations engaged in agriculture, i.e. approximately 80% of farmers are aged over 70 (MAFF, Citation2022c). As a result, the average cultivation area per worker is expanding, with the area increasing from 2.2 hectares in 2010 to 3.1 hectares in 2020 (MAFF, Citation2018a). Technological innovation is indispensable as a solution to labour shortage and in knowledge exchange between generations of farmers. To help aging farmers and ease entry into farming, there has been an increase in labour-saving developments through the introduction of innovative agricultural machineries, such as drones and robots. Within the next decade, self-driving tractors and robots are expected to become more common in the agricultural sector, and could perform tasks that are currently time-consuming and being performed by humans. These machineries are designated to connect via the IoT that will facilitate remote control and agricultural data storage. Since 2019, the Ministry of Agriculture, Forestry and Fisheries of Japan (MAFF) has promoted the introduction and demonstration of new agricultural technologies to farming sites through their ‘Smart Agriculture Acceleration Implementation Project.’ Cutting-edge technologies are being actively considered and tested for installation in agricultural settings. For example, field demonstration is underway for contributions to rice production from self-driving tractors, self-driving transplanting machineries, automated watering management systems, remote sensing, and pest-control using agricultural drones (i.e. agricultural unmanned aerial vehicle (UAV) sprayers) (MAFF, Citation2018b).
UAV sprayer in agricultural settings is one of the most popular machineries recently introduced in rice production in Japan (MAFF, Citation2019a). Despite being faced by major regulatory hurdles (i.e. a limited number of operators per unit, and restrictions on the flight level, the payload, and the types of pesticides used), UAVs are being used to complement power and boom sprayers, which have been conventionally used in rice production. Studies have evaluated the validity of UAVs in pest-control and estimated that UAVs decrease both the cost and labour compared to conventional sprayers (Seo & Umeda, Citation2021; Umeda et al., Citation2022). However, the environmental impacts of agricultural UAV sprayers remain unclear. In addition to assessing the effectiveness of these technologies in agricultural settings, it is crucial to prioritize environmentally friendly management practices to achieve sustainable agriculture. Agriculture, being a major sector, should address and mitigate its environmental impacts (Giuliana et al., Citation2022; McCartney & Lefsrud, Citation2018). The newly introduced technologies must be coupled with the design of a sustainable agricultural system, with this coupling being more effective if combined during the early stages of technology introduction (Santiteerakul et al., Citation2020).
Compared to conventional equipment, UAV sprayers have the advantage of being lightweight and requiring shorter operational time to cover the same area (Sun, Citation2020), suggesting a higher potential for environmental friendliness. On the other hand, it is important to note that they may still possess elements that contribute to environmental burdens, such as the use of lightweight and durable new materials or factors like batteries and chargers. Thus, verifying these claims is crucial. To estimate the environmental impact, life cycle assessment (LCA) is widely used, which is a systematic methodology used to evaluate the environmental impacts of a product, process, or service throughout its entire life cycle. It considers the environmental burdens associated with raw material extraction, manufacturing, use, and disposal. LCA provides a comprehensive analysis, considering various factors such as energy consumption, resource depletion, greenhouse gas emissions, water usage, and waste generation (Hauschild, Citation2005; SAIC, Citation2006; US EPA, Citation1993). In the context of agriculture, LCA can be utilized to assess the environmental impacts of farming practices, including crop production, livestock farming, and agricultural technologies. It helps identify areas of high environmental impact, allowing for the optimization of agricultural processes and the development of sustainable practices (Alhashim et al., Citation2021). LCA can quantify the contributions of different inputs, such as fertilizers, pesticides, machinery, and energy sources, to the overall environmental footprint of agricultural systems. By conducting LCA studies in agriculture, decision-makers can make informed choices to minimize environmental burdens and promote sustainable practices. This includes selecting more environmentally friendly inputs, optimizing resource efficiency, reducing emissions, and adopting innovative technologies. LCA also facilitates the comparison of different agricultural systems, enabling stakeholders to identify the most environmentally preferable options. Overall, LCA plays a crucial role in promoting sustainable agriculture by providing a scientific and holistic assessment of the environmental impacts associated with farming practices, guiding decision-making towards more sustainable and environmentally friendly approaches.
Thus, here, we conducted a comparative analysis of pest-control sprayers used in rice production at the farm level in Japan, specifically focusing on tractor-mounted boom sprayers (referred to as boom sprayers), power sprayers, and unmanned aerial vehicles (UAVs). Our aim was to assess the environmental impact of utilizing UAVs for crop spraying. For our study, we chose two model farms that implement the preventative control method, which involves the regular application of pesticides (twice per year) as a preemptive measure against pest and disease outbreaks. We conducted a Life Cycle Assessment (LCA) and compared three different agricultural spraying machinery options. To assess the impact of farm size, we designed 11 scenarios, ranging from 5 to 100 hectares, for sensitivity analysis.
Materials and methods
Goal and scope definition
We selected two model farms (): One is Farm H, located in the Kabato District, Hokkaido, where rice is produced via large-scale farming. The local climate is characterized by an average annual temperature of 10.0°C, and rainfall occurs mainly in summer, with an annual average rainfall of approximately 1010.5 mm. The other selected study site is Farm K, located in Kameoka City, Kyoto Prefecture, where rice is typically produced in rice fields in mountainous areas. The local climate here is characterized by an average annual temperature of 16.2°C, and rainfall occurs mainly in summer, with an annual average rainfall of approximately 1522.9 mm. Information regarding the pest control operations in each model farm was obtained through interviews (). Both farms introduced UAV methods for pest control in 2020. Farm H replaced an area of boom spraying-treated land (22.41 ha) with UAV treatments (23.13 ha), while Farm K replaced an area of power spraying-treated land (19 ha) with UAV treatments (30 ha). The labour hours associated with pest control decreased from 36 to 18 h and 60 to 42 h at Farms H and K, respectively, representing a consistent decrease by approximately 50% due to UAV introduction at both farms.
Table 1. Description of model farms.
As functional unit (FU), the cultivation of 1 ha of land and 1 year due to the period of rice cultivation. The functional unit for Life Cycle Assessment (LCA) is defined as the quantifiable measure that represents the function or benefit provided by the product or system being assessed. It serves as a reference unit for comparing different alternatives or scenarios within the life cycle analysis. In Japan, rice is cultivated once a year from spring to autumn. describes the system boundary, which delineates the system under evaluation, including relevant processes and activities. In life cycle assessment (LCA), the life cycle encompasses various stages including raw material extraction, fabrication/manufacturing, production, transportation/distribution, use, and end of life (disposal or recycling) (US EPA, Citation1993). In rice production, farming corresponds to the fabrication/manufacturing stage, which includes raising seedlings, tillage and fertilization, planting, pest control, harvesting, and drying (). After harvesting, rice is transported to the market sector. Inputs to farming (such as machinery, chemicals, seeds, water, electricity, and fuel) are supplied throughout the life cycle from material extraction to fabrication manufacturing. In this study, our focus was on the pest-control phase of the farming process and the material input phase of the manufacturing process. Through farmer interviews, we identified the pest control inputs as agricultural sprayers, electricity, fuel, pesticides, and water.
Life-cycle inventory (LCI)
The inventory data were collected through semi-structured interviews conducted in August 2021. Two participants from Farm K and one participant from Farm H were involved in the interviews. The interviews aimed to gather information on pest-control operations, including details on sprayers, pest-control periods, and duration, as well as farm management aspects such as yield and farm area.
The inventory data for pesticide control consist of two main components: the sprayers and the pesticides/water. For the sprayers, information regarding their product lifespan, weight, and accessories was collected from relevant studies and technical notes provided by manufacturers. This information was necessary to calculate the input per unit area over a one-year period. As for the pesticides and water, data were obtained through interviews using a questionnaire; information on pesticide control, specifically for the years 2018 for boom spraying, 2019 for power spraying, and 2020 for UAV treatment. The details of the calculation methods for these components are described below.
Machinery items were entered as a percentage of service time by dividing the machinery weight by the product lifespan (Hokazono & Hayashi, Citation2012); 7 years for three sprayers (Matsumoto, Citation2020). Therefore, 5.71 kg/ha of boom sprayer and 1.47 kg/ha of power sprayer were applied. Inputs to the body and accessories of UAVs were adopted from the literature (Koiwanit, Citation2018). The UAV body is composed of 0.3 kg/ha of carbon fibre reinforced plastic (CFRP) and 0.02 kg/ha of aluminium. UAV accessories include a lithium-ion battery and a charger; 0.30, 0.06 kg/ha, respectively () .
Table 2. Product lifespan and weight of boom sprayer, power sprayers, and UAVs.
Energy (L/ha) for boom and power sprayers was calculated using the theoretical operation timeboom, power (h) and fuel consumption (L/h, kg) (EquationEquation 1(1)
(1) ). The energy values were then converted to MJ for diesel (boom sprayer) and kg for gasoline (power sprayer). Theoretical operation timeboom, power (h) was estimated by area (ha), spraying width (m), and the operational speed (km/h) (Equation (1)-1, S.1).
(1)
(1)
(1-1)
(1-1)
The boom sprayer used 24.4 L of diesel per 22.4 ha, while the power sprayer consumed 25.3 L of gasoline per 19.0 ha. To calculate the electricity consumed by the UAV, the number of battery replacements and the battery capacity (measured in watt-hours, Wh) were applied (Equation 2).
(2)
(2)
The number of battery replacements was estimated using the theoretical flight timeUAV (min) and average flight time (min/number of flights) (Equation 2-1). The theoretical flight timeUAV (min) indicates the flight time for the full target area to be sprayed, and was calculated using the area, flight speed, and spray width (Equation 2-2). Average flight time (min/number of flights) was estimated using maximum and minimum flight time (Equation 2-3). The maximum flight time is the flight time of a UAV without a payload, and the minimum flight time is the flight time of a full payload. We determined that a UAV used 0.28 Wh/ha at Farm H and 0.25 Wh/ha at Farm K.
(2-1)
(2-1)
Theoretical flight timeUAV (min) = Area (m2) / Flight speed (m/min) / Spraying width (m) (2-2)
(2-2)
(2-2)
(2-3)
(2-3)
The amounts of pesticide and water consumed were calculated by dividing the value provided in the farmer interviews by the control area. Water was only used to dilute pest-control chemicals used in boom and power sprayers. The amount of pesticide applied per area was consistent for both farms because only the dilution concentration at the time of application differed between the sprayers. Fungicide was applied in Farm H, with an application rate of 0.40 kg/ha for the boom sprayer and 0.46 kg/ha in UAV. Fungicide and insecticide application rates at Farm K were 0.57 kg/ha for the power sprayer, and 0.57 kg/ha for the UAV ().
Table 3. Life cycle inventory for Farm H and Farm K.
Data were then modelled on Ecoinvent 3.8 in Simapro 8 (Wernet et al., Citation2016). The Ecoinvent 3.8 database contains LCI data for production and transportation of agricultural machineries, fuel, electricity, chemicals, and tap water. Average process data from the international databases were used in this study and adapted to be as consistent as possible with the objective and scope of the study.
Life-cycle impact assessment (LCIA)
The ReCiPe 2016 Midpoint (H) has been used to analyze the environmental performance of pest control in rice production. We chose this methodology because it allows for a comprehensive assessment of the environmental impacts of rice production by considering 18 different impact categories. The use of the Recipe 2016 Midpoint method is also prevalent in LCA studies of rice production, making it a widely used and recognized approach. This methodology provides detailed and specific results that contribute to a thorough understanding of the environmental implications associated with rice production (Escobar et al., Citation2022; Rezaei et al., Citation2021; Xu et al., Citation2022).
Sensitivity analysis
Sensitivity analysis is performed to assess the effects of various input parameters (Groen et al., Citation2017). It has been used to evaluate how the operational area influences the impact categories at the midpoint level. When introducing new machines, the number of machines is determined by considering operational efficiency and area to achieve management goals (Hokkaido Prefecture, Citation2014). Our study specifically examined the influence of the operation area on the selection of agricultural sprayers. To investigate this, we developed 11 scenarios with varying areas, ranging from 5 to 100 hectares. Global Warming Potential (GW) for a functional unit of 1 ha in each scenario was estimated. Based on the questionnaire results, the use of pesticides and water was assumed to vary in proportion to the operational area. The number of sprayers according to the operation area were determined based on Governmental manuals (Hokkaido Prefecture, Citation2019). UAV was assumed a unit up to 100 ha (MAFF, Citation2019b) () .
Table 4. Machinery units of pest-control area (ha).
Results and discussion
Recipe 2016 midpoint (E)
LCIA results highlighted the inputs with the most significant impact of pest-control phases in rice production. The results of the assessment are shown in . In addition, we presented the results as a relative impact (), which represents the emissions as a proportion of the total emissions. Expression of the results as percentages renders LCIA results more usable, especially when using calculation methods such as ReCiPe 2016 Midpoint considers 18 impact categories with different units of measurement. By presenting the results as a relative impact, we are able to analyze the different categories on the same scale and effectively highlight specific characteristics or patterns within the data.
Figure 3. LCIA for the pest-control phase of rice production in Japan, considering use of (A) a boom sprayer at Farm H; (B) a UAV at Farm H; (C) a power sprayer at Farm K; and (D) a UAV at Farm K.

Table 5. LCIA results of pest-control operation using boom spraying, power spraying, and UAV treatment.
Following replacement of boom spraying by UAV treatment at Farm H, the overall environmental impact was reduced, specifically in 14 out of the 18 categories. Considering the Global Warming Potential (GW) impact category as an example, UAVs produced13.0 kg CO2 eq, which is approximately one-third of the boom spraying impact (39.5 kg CO2 eq). The impact of UAVs on fossil resource scarcity (FRS) was 43% of the boom spraying impact. However, Terrestrial Ecotoxicity (TE), Freshwater Ecotoxicity (FEC), Marine Ecotoxicity (MED), and Human Non-Carcinogenic Toxicity (HNCT) increased for UAV and accounted for 1.2–1.7 times more environmental impact than that of boom spraying. At Farm K, replacement of power spraying by UAV treatment resulted in increases in seven categories: Terrestrial Acidification Potential (TA), Freshwater Eutrophication Potential (FE), TE, FEC, ME, HNCT, and Land Use Potential (LU) increased. The other 11 categories exhibited a reduction for UAV compared to power spraying. For example, the Global Warming Potential reduced from 17.8 kg CO2 eq for power spraying to 14.2 kg CO2 eq for UAV. FRS decreased from 7.6 kg oil eq to 6.0 kg oil eq for UAV. However, the environmental impact due to UAV replacement was lower at Farm K than at Farm H ( ).
Table 6. Parameters of input of energy .
Comparisons could be made between all three different agricultural sprayers because UAV application is common between the two model farms. Results suggested that boom spraying produced the highest environmental impact for a unit area (1 ha) per year, followed by power spraying, with UAVs exhibiting the lowest environmental impact. This can be explained by the higher weight and energy consumption of the boom sprayer, which is approximately 4 and 14 times more than that of the power sprayer and UAV, respectively. The weight of the power sprayer is approximately seven times greater than that of the UAV. The fuel consumption of the boom sprayer is 39.9 MJ of diesel, compared with 0.99 kg of gasoline for the power sprayer and 0.25 kWh of electricity for the UAV. Although the units are different, the consumption is noticeably larger for boom spraying than for other methods. Hence for boom spraying, a greater volume of inputs was consumed in the production phase, thus resulting in a greater environmental impact.
The type of construction material can also affect the environmental impact. The UAV body is made of carbon fibre reinforced plastic (CFRPs) and aluminium (Al), which are promising composite materials aimed to revolutionize the aerial industry. These materials provide higher strength, lower weight, and less maintenance than that of traditional materials, and are beneficial in reducing fuel consumption (Witik et al., Citation2013; Yang et al., Citation2012). However, it is worth noting that the production process of CFRP is currently not optimized to minimize environmental impact, primarily due to its high energy footprint (Stergiou et al., Citation2022). Therefore, while the environmental impact of the UAV materials was slightly lower than that of the power sprayer, it is important to consider that the UAV utilizes a highly functional material, which requires numerous processing steps and results in significant consumption of raw materials and energy. Additionally, despite the UAV's lighter weight compared to the power sprayer, the overall environmental impact should be assessed holistically, taking into account all stages of the life cycle and the specific context of each technology.
The relative impacts of different inputs are illustrated in . For the boom spraying in Farm H, machinery had the largest environmental impact. Machinery had a significant impact on 15 out of the 18 categories, 9 of which accounted for more than 70% of the total environmental impact. Machinery was followed by pesticides, with the second largest impact, which accounted for 4–56% of the total impact in each category. Energy had the third greatest impact on average, accounting for 3–30% of the total impact in each category. For the UAV treatment at Farm H, machinery accounted for 20–93% of the total impact in each category, followed by pesticides, which accounted for 7–80%. The contribution of energy to the total environmental impact was <1% throughout the categories, which can be explained by the low electricity consumption due to the short UAV flight time and high operational efficiency (5 ha/h) compared to the boom sprayer (2.3 ha/h). For the UAV, the effect of water was reduced by 52% to 5% in the Water Consumption Potential (WC) category, because more water was used to dilute pesticides than that used in boom sprayers, i.e. higher concentration of pesticides was used in UAV treatments.
In Farm K, pesticides had the largest environmental impact overall, showing 2–83% for power spraying and 2–87% for UAV, followed by machinery accounting for 5–64% for power sprayer and 13–83% for UAV, respectively. For power spraying, pesticides exerted a significant impact on 11 out of the 18 categories, accounting for >50% of the total impact in each category. In particular, pesticides were significant in Stratospheric Ozone Depletion Potential (SOD) and Marine Eutrophication Potential (ME), which showed 2.81 × 10−5 kg CFC11 eq (83% of the total) and 1.36 × 10−3 kg N eq (77% of the total), respectively. This trend was similar to that in Farm H. Pesticides are known to be one of the major factors contributing to the environmental impact of paddy rice production, especially in the categories of ME, FE, MED, HNCT, and SDO (Giuliana et al., Citation2022; Rezaei et al., Citation2021). Less environmentally harmful alternatives are under development (Sande et al., Citation2011).
The general trend was similar between the two farms but the contribution of machinery and pesticides to the total environmental impact differed. Machinery was the greatest contributor to the environmental impact at Farm H, while agrochemicals were most significant at Farm K. This is likely due to the type of pesticides and their different applications according to regional weather and disease outbreak (MAFF, Citation2020), i.e. fungicide was used at Farm H, compared to both fungicide and insecticide at Farm K. Despite similar agrochemical input at both farms, the impact of the fungicide/insecticide combination (Farm K) was higher than fungicide (Farm H) across all impact categories. For example, comparison of the UAV treatments between both farms for the GW category revealed that fungicide use resulted in a 5.29 kg CO2 eq impact, compared to 8.96 kg CO2 eq for fungicide/insecticide. For the FRS category, fungicide use resulted in 2.46 kg oil eq, compared to 4.57 kg oil eq for fungicide /insecticide. In recent years, most agrochemicals for paddy rice production in Japan have become UAV-compliant (MAFF, Citation2022a); thus, there has been little increase in environmental impact following pest-control machinery replacement.
Sensitivity analysis
To evaluate the environmental performance of various agricultural sprayers, we conducted a sensitivity analysis to examine the influence of specific input parameters on the results. Specifically, the analysis considered the change in machinery inputs for the three different agricultural sprayers (). Results show Global Warming Potential (GW) for a functional unit of 1 ha in each scenario. Boom spraying showed the largest impact on GW for different operational areas. For example, at 5 ha, the impact of boom spraying (142.4 kg CO2 eq) was more than three times that of power spraying and UAV treatment. This difference gradually decreased with increasing area, with only moderate differences observed between the methods at an area of 60 ha. For an area of 100 ha, where 2 units of boom sprayer was applied, the impact of boom spraying increased again, measuring 23.2 kg CO2 eq. This suggests that boom spraying is recommendable for farms smaller than 50 ha to mitigate the environmental burden.
Power spraying and boom spraying showed similar trends in their GW impact, showing a sharp decrease from 5 ha to 20 ha. The GW impact of power spraying reduced from 39.1 kg CO2 eq to 17.5 kg CO2 eq, and the GW impact of UAV treatment decreased from 39.0 kg CO2 eq to 14.6 kg CO2 eq. Both power spraying and UAV treatment showed similar contributions at 5 ha, but from 10 ha, while UAV showed the greatest decrease of the three agricultural sprayers. The difference from power sprayer also increased gradually from 0.1 kg CO2 eq for an area of 5 ha, to 1.9 kg CO2 eq for 10 ha, and was then stable at 7.6–9.1 kg CO2 eq for areas above 50 ha. Both curves flattened from 20 ha, although power sprayer was up slightly in 30 ha, due to which, the number increased to 2. Power sprayer is usually used on the back, which restricts the operational speed and spray width; thus, more power spraying is needed as the area is increased, compared with boom spraying and UAV spraying. A UAV sprayer is capable of covering up to 100 ha due to its high operation speed and spray width. The environmental impact of the UAV there decreased with increasing operational area, reaching 8.1 kg CO2 eq for an area of 100 ha; this is the lowest environmental impact of the three sprayers. Therefore, our results show that the UAV sprayer is the most environmentally friendly for areas of up to 100 ha.
Machinery contributed the most to the total environmental impact for boom spraying. For example, it accounted for 93% of the total impact for an operational area of 5 ha, and 69% for 30 ha, and 57% of the impact of boom spraying at 100 ha. With increasing area, the amount of machinery input decreased; thus, the environmental impact of boom spraying per ha decreased sharply due to the machinery weight. For UAV treatment, machinery also contributed the most to the environmental impact across all operational areas. For power spraying, machinery had a significant contribution to total impact at areas of 5 and 10 ha, but at areas of 20 ha and greater, pesticides contributed the most to the total impact, with pesticide contributions for UAV treatment being 9.0 kg CO2 eq, compared to contributions from machinery of 7.2 kg CO2 eq.
The sensitivity analysis shows that the choice of agricultural sprayer in a given area could significantly reduce environmental impacts, indicating that lighter and time-saving sprayers can reduce the environmental impact. Our results suggested that UAV treatment can be used to mitigate the environmental burden in up to operational areas of 100 ha in the pest control phase of paddy rice production in Japan. Although UAV treatment reduces the time required for treatment and the environmental burden, there are still barriers to its use due to the strict regulations in Japan. Operators require a license and for safety, one operator must observe whilst another operates the UAV (Seo & Umeda, Citation2021). These kinds of cutting-edge technologies also require infrastructure such as provision of efficient energy supplies, trained operators, and high-speed wi-fi. For example, commonly, a farm must be redesigned to allow effective and safe movement of robots or automated equipment. High speed field wi-fi facilitates the transfer of precise cultivation data to a server. To realize future innovation in the agricultural sector, it is necessary to evaluate performance of possible interventions from the beginning, and to build new systems that facilitate technology introduction (Smith et al., Citation2021; Vasconez et al., Citation2019).
Limitations and future recommendations
This study compared various agricultural sprayers used for pest control in rice production. However, it is important to acknowledge certain limitations in our research. The target farms were selected to be representative of typical Japanese paddy rice farms. In order to apply our results and conclusions to other areas, many factors need to be considered, including the climate, operation area, and UAV regulations. The operational area range of 20–30 ha covered in this study is large compared to typical operational areas used in China and India, the largest global rice producers, where most farms are <10 ha (DES, Citation2020; MAFF, Citation2022b). Effective introduction and validation of UAVs in smallholder farmers is needed. UAV regulation is reasonably strict in Japan, with at least two operators and a license required for field spraying. This could influence the number of UAVs used for pest control application.
When considering the limitations of LCA analysis, it is important to acknowledge the approach taken in this study to calculate the electricity consumption of UAVs. The battery replacement frequency was estimated by calculating the average flight speeds based on the maximum and minimum values, assuming a relationship between battery replacement frequency and flight speed, while also considering the payload. It is generally recognized that higher flight speeds tend to result in increased battery consumption. However, it is crucial to note that this estimation method may lack certainty due to the absence of precise records. There is also the possibility that factors other than flight speed could influence the frequency of battery replacement. For example, wind conditions can affect the flight path, leading to drift that may alter the direction of spraying. Although this study assumed that pest control using UAVs was conducted under consistent conditions with wind speeds below 5 m/s, following the safety guidelines outlined in the UAV Flight Manual by the Civil Aviation Bureau of the Ministry of Land, Infrastructure, Transport and Tourism in Japan (MLIT, Citation2021), where the impact of wind could be disregarded, it is essential to conduct further research to assess the influence of drift. Additionally, it is important to acknowledge that the system boundary of this study was limited to the raw materials and the pest-control operation. To provide a more comprehensive understanding of the environmental impact and enable a precise comparison between UAVs and conventional sprayers, it would be necessary to extend the analysis to include the end-life phase of the products.
The agricultural sector is currently undergoing a transformation in various inputs, such as chemicals, machinery, and energy (Santiteerakul et al., Citation2020). As part of this transformation, the study conducted a comparison of different agricultural sprayers used for pest control in rice production. It demonstrated the environmental friendliness of UAVs in specific operational areas. However, the adoption of new technology in agriculture is influenced by multiple factors. One significant factor is the reliance on individual farmers in the agricultural sector. Due to region-specific geology, soil conditions, and weather, there are limited standardized approaches to crop cultivation. Farmers have their own cultivation methods, which may result in resistance to adopting new technologies. Additionally, evaluating the impact of new technology is time-consuming, given the typical one-year cultivation cycle (Aboal et al., Citation2019; Smith et al., Citation2021; Vasconez et al., Citation2019). To provide more practical information for farmers and policymakers, future research should not only focus on the environmental impacts of rice farming but also consider the social impacts. This can be achieved by increasing the number of studies employing Social Life Cycle Assessment (S-LCA), which is currently lacking in rice farming research (Giuliana et al., Citation2022). By assessing the social factors influencing the sustainable development of rice production, S-LCA can serve as a valuable tool for local policymakers to enhance the well-being and safety of farmers.
Conclusion
The aim of this study was to assess the environmental impact of three different agricultural sprayers used for pest control in paddy rice farming in Japan. Two farms located in Kyoto Prefecture and Hokkaido were selected, and a life cycle assessment (LCA) was conducted. As a result, in terms of comparing the environmental impact per unit area, UAVs exhibited a significant reduction compared to boom sprayers due to their use of materials requiring advanced processing and the inclusion of accessories such as batteries and chargers. However, the reduction in environmental impact compared to power sprayers was found to be minimal. Furthermore, sensitivity analysis conducted based on field area indicated that UAVs demonstrated significant environmental advantages for operational areas ranging from 5 to 100 hectares. While there was only a slight reduction observed between 50 and 100 hectares, it was evident that UAVs achieved comparable or reduced environmental impact compared to conventional machinery. Based on these findings, we can conclude that the adoption of lighter and low-energy machinery holds promise as a pathway to reduce environmental impact and promote sustainable agriculture. This study is significant as it provides an environmental impact assessment based on empirical data when substituting conventional boom sprayers and power sprayers with UAVs in pest control operations.
Acknowledgements
The authors would like to thank to the farmers for their participation in the interviews.
Disclosure statement
No potential conflict of interest was reported by the author(s).
References
- Aboal, D., Mondelli, M., & Vairo, M. (2019). Innovation and productivity in agricultural firms: Evidence from a country-wide farm-level innovation survey. Economics of Innovation and New Technology, 28(6), 616–634. https://doi.org/10.1080/10438599.2018.1546558
- Alhashim, R., Deepa, R., & Anandhi, A. (2021). Environmental impact assessment of agricultural production using LCA: A review. Climate, 9(11), 164. https://doi.org/10.3390/cli9110164
- Avgoustaki, D. D., & Xydis, G. (2020). Plant factories in the water-food-energy nexus era: A systematic bibliographical review. Food Security, 12(2), 253–268. https://doi.org/10.1007/s12571-019-01003-z
- Directorate of Economics and Statistics. (2020). Pocket book of agricultural statistics 2020. Retrieved November 22, 2022, from https://desagri.gov.in/wp-content/uploads/2021/06/Pocket-2020-Final-web-file.pdf
- Engler, N., & Krarti, M. (2021). Review of energy efficiency in controlled environment agriculture. Renewable and Sustainable Energy Reviews, 141, Article 110786. https://doi.org/10.1016/j.rser.2021.110786
- Escobar, N., Bautista, I., Peña, N., Fenollosa, M. L., Osca, J. M., & Sanjuán, N. (2022). Life cycle thinking for the environmental and financial assessment of rice management systems in the Senegal River valley. Journal of Environmental Management, 310, Article 114722. https://doi.org/10.1016/j.jenvman.2022.114722
- Giuliana, V., Lucia, M., Marco, R., & Simone, V. (2022). Environmental life cycle assessment of rice production in northern Italy: A case study from Vercelli. The International Journal of Life Cycle Assessment, 1–18. https://doi.org/10.1007/s11367-022-02109-x
- Groen, E. A., Bokkers, E. A., Heijungs, R., & de Boer, I. J. (2017). Methods for global sensitivity analysis in life cycle assessment. The International Journal of Life Cycle Assessment, 22(7), 1125–1137. https://doi.org/10.1007/s11367-016-1217-3
- Hauschild, M. Z. (2005). Assessing environmental impacts in a life-cycle perspective. Environmental Science & Technology, 39(4), 81a–88a. https://doi.org/10.1021/es053190s
- Hokazono, S., & Hayashi, K. (2012). Variability in environmental impacts during conversion from conventional to organic farming: A comparison among three rice production systems in Japan. Journal of Cleaner Production, 28, 101–112. https://doi.org/10.1016/j.jclepro.2011.12.005
- Hokkaido Prefecture. (2014). Agricultural machinery introduction plan formulation guide (in Japanese). Retrieved December 12, 2022, from http://www.pref.hokkaido.lg.jp/ns/gjf/kankyo/tokuteikouseinou_keikaku.pdf
- Hokkaido Prefecture. (2019). Hokkaido agricultural production technology system (5th ed.). Public Interest Incorporated Foundation Hokkaido Agricultural Extension Association (in Japanese).
- Juhola, S., Klein, N., Käyhkö, J., & Neset, T. S. S. (2017). Climate change transformations in Nordic agriculture? Journal of Rural Studies, 51, 28–36. https://doi.org/10.1016/j.jrurstud.2017.01.013
- Koiwanit, J. (2018). Analysis of environmental impacts of drone delivery on an online shopping system. Advances in Climate Change Research, 9(3), 201–207. https://doi.org/10.1016/j.accre.2018.09.001
- Li, Y., Conway, D., Xiong, W., Gao, Q., Wu, Y., Wan, Y., Li, Y., & Zhang, S. (2011). Effects of climate variability and change on Chinese agriculture: A review. Climate Research, 50(1), 83–102. https://doi.org/10.3354/cr01038
- MAFF. (2018a). Development of smart agriculture (in Japanese). Retrieved December 10, 2022, from https://www.affrc.maff.go.jp/docs/smart_agri_pro/attach/pdf/smart_agri_pro-11.pdf
- MAFF. (2018b). Smart agriculture demonstration project (in Japanese). Retrieved December 10, 2022, from https://www.affrc.maff.go.jp/docs/smart_agri_pro/attach/pdf/smart_agri_pro-20.pdf
- MAFF. (2019a). Toward wider use of agricultural drones (in Japanese). Retrieved November 22, 2022, from https://www.maff.go.jp/j/kanbo/smart/attach/pdf/drone-165.pdf
- MAFF. (2019b). The implementation status of aerial spraying of pesticides (in Japanese). Retrieved December 10, 2022, from https://www.maff.go.jp/j/syouan/syokubo/gaicyu/g_kouku_zigyo/attach/pdf/index-49.pdf
- MAFF. (2020). Prevention, control and spread of diseases and pests (in Japanese). Retrieved December 12, 2022, from https://www.maff.go.jp/j/syouan/syokubo/keneki/attach/pdf/arikata-19.pdf
- MAFF. (2022a). Pesticide registration information system (in Japanese). Retrieved December 12, 2022, from https://pesticide.maff.go.jp/
- MAFF. (2022b). Food subcommittee of the council on food, agriculture and rural policies. Retrieved November 22, 2022, from https://www.maff.go.jp/j/council/seisaku/syokuryo/210226/attach/pdf/index-36.pdf
- MAFF. (2022c). FY2021 summary of the annual report on food, agriculture and rural areas in Japan. Retrieved November 22, 2022, from https://www.maff.go.jp/j/wpaper/w_maff/r3/zenbun.html
- Matsumoto, H. (2020). Effects of cooperative work using an agricultural robot in a paddy field farming. The Farm Management Society of Kanto Tokai Hokuriku District, 110, 51–58 (in Japanese). https://fmrp.rad.naro.go.jp/KTHJFM/dl_files/110/KTHJFM_110_059-064.pdf.
- McCartney, L., & Lefsrud, M. (2018). Protected agriculture in extreme environments: A review of controlled environment agriculture in tropical, arid, polar, and urban locations. Applied Engineering in Agriculture, 34(2), 455–473. https://doi.org/10.13031/aea.12590
- MLIT. (2021). UAV flight manual (in Japanese). Retrieved July 13, 2021, from https://www.mlit.go.jp/common/001218180.pdf
- O'Sullivan, C. A., Bonnett, G. D., McIntyre, C. L., Hochman, Z., & Wasson, A. P. (2019). Strategies to improve the productivity, product diversity and profitability of urban agriculture. Agricultural Systems, 174, 133–144. https://doi.org/10.1016/j.agsy.2019.05.007
- Rezaei, M., Soheilifard, F., & Keshvari, A. (2021). Impact of agrochemical emission models on the environmental assessment of paddy rice production using life cycle assessment approach. Energy Sources, Part A: Recovery, Utilization, and Environmental Effects, 1–16. https://doi.org/10.1080/15567036.2020.1864066
- Sande, D., Mullen, J., Wetzstein, M., & Houston, J. (2011). Environmental impacts from pesticide use: A case study of soil fumigation in Florida tomato production. International Journal of Environmental Research and Public Health, 8(12), 4649–4661. https://doi.org/10.3390/ijerph8124649
- Santiteerakul, S., Sopadang, A., Yaibuathet Tippayawong, K., & Tamvimol, K. (2020). The role of smart technology in sustainable agriculture: A case study of wangree plant factory. Sustainability, 12(11), 4640. https://doi.org/10.3390/su12114640
- Scientific Applications International Corporation. (2006). Life-cycle assessment: Principles and practice. National Risk Management Research Laboratory (US), Office of Research and Development, Environmental Protection Agency, United States. http://people.cs.uchicago.edu/~ftchong/290N-W10/EPAonLCA2006.pdf
- Seo, Y., & Umeda, S. (2021). Evaluating farm management performance by the choice of pest-control sprayers in rice farming in Japan. Sustainability, 13(5), 2618. https://doi.org/10.3390/su13052618
- Smith, H. E., Sallu, S. M., Whitfield, S., Gaworek-Michalczenia, M. F., Recha, J. W., Sayula, G. J., & Mziray, S. (2021). Innovation systems and affordances in climate smart agriculture. Journal of Rural Studies, 87, 199–212. https://doi.org/10.1016/j.jrurstud.2021.09.001
- Stergiou, V., Konstantopoulos, G., & Charitidis, C. A. (2022). Carbon fiber reinforced plastics in space: Life cycle assessment towards improved sustainability of space vehicles. Journal of Composites Science, 6(5), 144. https://doi.org/10.3390/jcs6050144]
- Sun, W. (2020). Efficient pest control technology using multicopters in mountainous regions (NARO Technical Report 5; pp. 30–33). https://www.naro.go.jp/publicity_report/publication/files/naro_technical_report_no5.pdf
- Umeda, S., Yoshikawa, N., & Seo, Y. (2022). Cost and workload assessment of agricultural drone sprayer: A case study of rice production in Japan. Sustainability, 14(17), 10850. https://doi.org/10.3390/su141710850
- United State Environmental Protection Agency. (1993). Office of research and development, life cycle assessment: Inventory guidelines and principles (EPA/600/R-92/245). https://cfpub.epa.gov/si/si_public_record_Report.cfm?Lab=NRMRL&dirEntryID=124777
- Vasconez, J. P., Kantor, G. A., & Cheein, F. A. A. (2019). Human–robot interaction in agriculture: A survey and current challenges. Biosystems Engineering, 179, 35–48. https://doi.org/10.1016/j.biosystemseng.2018.12.005
- Wernet, Gregor, Bauer, Christian, Steubing, Bernhard, Reinhard, Jürgen, Moreno-Ruiz, Emilia, & Weidema, Bo. (2016). The ecoinvent database version 3 (part I): Overview and methodology. The International Journal of Life Cycle Assessment, 21(9), 1218–1230. http://dx.doi.org/10.1007/s11367-016-1087-8
- Witik, R. A., Teuscher, R., Michaud, V., Ludwig, C., & Månson, J. A. E. (2013). Carbon fibre reinforced composite waste: An environmental assessment of recycling, energy recovery and landfilling. Composites Part A: Applied Science and Manufacturing, 49, 89–99. https://doi.org/10.1016/j.compositesa.2013.02.009
- Xu, Q., Dai, L., Gao, P., & Dou, Z. (2022). The environmental, nutritional, and economic benefits of rice-aquaculture animal coculture in China. Energy, 249, Article 123723. https://doi.org/10.1016/j.energy.2022.123723
- Yang, Y., Boom, R., Irion, B., van Heerden, D. J., Kuiper, P., & de Wit, H. (2012). Recycling of composite materials. Chemical Engineering and Processing: Process Intensification, 51, 53–68. https://doi.org/10.1016/j.cep.2011.09.007