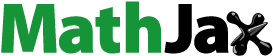
ABSTRACT
The agriculture and livestock sectors face several challenges related to achieving the current EU environmental objectives. Two of the major policy goals include reducing greenhouse gas emissions and ensuring an increased share of nitrogen, phosphorus, and potassium from renewable sources. Farmers are continuously seeking to adopt technologies and solutions to ensure sustainable food production systems. Adoption of innovation at the farm level based on a circular economy may improve resource efficiency, allow the reuse and recovery of nutrients, and reduce the negative effects of emissions on soil, water, and air. This study aims to identify the factors affecting the adoption of several circular agronomy solutions using a semi-structured questionnaire on a sample of farmers in four EU countries: Spain, Austria, the Czech Republic, and Italy. The results indicated that acceptance of proposed circular innovations is closely related to farmers’ environmental objectives, experience, university education, previous experience in innovation adoption, and environmental attitudes. Additionally, institutional support plays a significant role in adoption decisions. Factors affecting adoption decisions may assist policymakers in designing more specific and efficient measures to help farmers meet their current social needs and environmental challenges.
1. Introduction
Agri-food producers face continuous challenges related to meeting the increasing demand for affordable food while maintaining high environmental and quality standards. The current agricultural system should guarantee an efficient use of resources and increase the reuse and recovery of nutrients while addressing associated environmental issues. However, intensive production systems have led to the inefficient use of nutrients, contributing to water, air, and soil pollution. Traditional and obsolete agricultural practices in livestock and crop production lead to environmental degradation by increasing carbon dioxide (CO2) and other greenhouse gas (GHG) emissions (Rehman et al., Citation2021). This evidences that conventional agricultural systems are unsustainable and lead to substantial environmental degradation (Singh et al., Citation2022). Therefore, more effort is needed to ensure the efficient management of nutrients in agricultural systems to reduce GHG and ammonia emissions and the eutrophication of water bodies due to the excessive leakage of nutrients (Svanbäck et al., Citation2019). On average, agriculture is responsible for 77% of the total nitrogen load in the environment (CEE, Citation2020). Moreover, approximately 20% of global CO2 emissions are attributed to agricultural production (Pata, Citation2021). Rehman et al. (Citation2021) have reported that a 1% increase in agricultural production increased GHG emissions from 18 to 35%. Of the global GHG emissions (52,300 Mt of CO2 equivalents, CO2e), 3,600 Mt were generated by the 27 European Union countries, of which crop and livestock production represented 13% (478 Mt of CO2e) (Poore & Nemecek, Citation2018). The 4 countries studied in this research (Austria, the Czech Republic, Italy, and Spain) accounted for 23% of the EU agricultural emissions (Eurostat, Citation2021), of which Spain represented 10.7%, Italy 8.8%, the Czech Republic 1.9%, and Austria 1.6% ().
Figure 1. GHG emissions from the EU agricultural sector (Eurostat, Citation2021).

Within the European agri-food chain, nitrogen use is inefficient, with only one-fifth being transformed into products for human consumption, similar to what occurs with phosphorus and potassium (Circular Agronomics, Citation2018; Directive Citation91/Citation676/CEE, Citation2021). Low nutrient efficiency and poor soil management practices lead to large losses of nutrients and carbon to the environment, which reduces agricultural productivity and increases pollution, hindering the sustainability of the European agri-food chain (Sarteel et al., Citation2016). That is why one of the main challenges associated with achieving the objectives of the Common Agricultural Policy (CAP) for the European Commission is reducing the loss of nutrients in food production. and achieving environmentally sustainable nutrient levels (CEE, Citation2020). Additionally, according to the EU commitment (Albarracín-Vacca, Citation2022), another challenge is reducing GHG emissions by 55% by 2030 compared to the emission levels generated in 1990 to achieve climate neutrality by 2050 (Wolf et al., Citation2021).
The circular economy (CE) model aims to eliminate the linear model of consumption and subsequent disposal. Its objective is reusing and reinserting inputs into the economy, avoiding the production of waste and high consumption of non-renewable resources (Leiva & Paulovich, Citation2021). CE models promote the sustainable use of natural resources, thereby, reducing impacts on the ecosystem and human wellbeing (López-Páez & García-Herreros, Citation2021). Under this model, residues from agricultural biomass and food processing are retained within the food system in the form of renewable resources (inputs), thus, reducing the introduction of new inputs from outside the system (Ortiz Gutiérrez et al., Citation2022). Adopting CE solutions is crucial to achieve food security and agricultural sustainability (Nordin et al., Citation2022). Through the introduction of CE principles in agriculture, nitrogen losses can be reduced, while CO2 and other GHGs can be absorbed and retained (Johnson et al., Citation2007; Preston & Jones, Citation2006). In recent years, the European agricultural sector has implemented various sustainable technologies focused on circular agriculture, which reduce waste and GHG emissions while producing high-quality food.
The adoption of sustainable innovative technologies in agriculture aims to improve soil, crop, and livestock management (Borges et al., Citation2019). Adopting innovations involving circular practices, such as the recovery and reuse of nutrients and carbon, improves the efficiency of resources, preserves soil fertility and water quality, and avoids environmental resource degradation for future generations. Nevertheless, innovation adoption faces many constraints that differ across socioeconomic groups, and regions as well as over time (Feder et al., Citation1985).
The main objective of this study was to analyze the factors affecting farmers’ decision to adopt circular innovations and solutions developed within the Circular Agronomics H2020 project (Circular Agronomics, Citation2018). The analyzed solutions introduced circularity within livestock farming activities, which is based on the principles of resource recyclability and waste minimization. Considering that one of the measures proposed within the European Green Deal is to reduce GHGs to achieve climate neutrality by 2050 (Wolf et al., Citation2021), understanding the drivers and limitations affecting the acceptance of CE initiatives from the farmers’ perspective is highly relevant. Furthermore, it is crucial to delve deeper to understand the main factors motivating farmers to introduce circular innovations into their production systems. Knowing farmers’ expectations and needs may help policymakers establish more specific action schemes and regulations focused on increasing the adoption of innovations aimed at reducing GHG emissions and support the transition towards more sustainable practices. This study contributes to the scarce literature on the factors influencing the adoption of circular farming innovations in a comparative manner in different EU countries. It also introduces farmers’ environmental attitudes using the new ecological paradigm (NEP) scale and farmers’ preferences for agribusiness objectives using the Analytic Hierarchy Process (AHP) as two additional dimensions of the extended theory of planned behaviour (TPB). Furthermore, this study seeks to update the knowledge regarding the factors affecting farmers’ expectations and decisions towards introducing circularity through innovation at the farm level.
2. Literature review
The growing interest in circular agricultural innovation solutions and technologies and their adoption relies on their positive impact on the environment (Dorr et al., Citation2021; Fan et al., Citation2018). Understanding the factors affecting farmers’ adoption of technological innovation is key to improving agricultural sustainability and efficiency. Different studies have used both social and economic approaches to identify the main reasons farmers decide to adopt innovative and more sustainable solutions. However, the adoption process is too complex and multidimensional to be fully understood; additional and continuous efforts are needed to deeply analyze this process, which is closely related to the regions, countries, and sectors involved as well as the global economic context. Meijer et al. (Citation2015) have suggested that innovation can be a concept and that technical information can be perceived as new. Therefore, the circular agricultural concept is based on introducing changes to conventional models of production, moving towards agriculture systems in which the re-use of waste and revaluation of by-products resulting from agricultural and livestock production are necessary. These innovations are expected to optimize the efficient use of resources and reduce environmental impacts (Juárez et al., Citation2021).
Adoption may also be defined as the process by which producers decide to incorporate new techniques or technologies into their existing production systems (Martínez & Gómez, Citation2012; Taghouti et al., Citation2021). Rogers (Citation1963) defined the adoption process as the mental process an individual goes through from first hearing about an innovation until its final adoption. Innovation adoption at the farm level implies changes aimed at improving productivity and increasing efficiency in the use of resources (Cuevas et al., Citation2013). However, different factors influence farmers’ adoption decisions with regard to sustainable agricultural technologies, as can be observed in different systemic literature reviews (Serebrennikov et al., Citation2020).
Focusing on sustainable agricultural practices or technologies, several studies have implemented different qualitative and quantitative methodologies to identify the determinant factors affecting the adoption of agricultural innovations (Abegunde et al., Citation2019; Knowler & Bradshaw, Citation2007). Some studies are based on the theoretical framework of the TPB proposed by Ajzen (Citation1991), which considers the impact of additional constructs on behaviour as an extended model of the reasoned action theory (Ajzen & Fishbein, Citation1980). The conceptual model of the TPB explaining the intention to perform a behaviour considers environmental attitudes, preferences, subjective norms (social and personal), and perceived behaviour control as constructs that influence decision making (Despotović et al., Citation2019; Le, Citation2010). López and Requena (Citation2005) have indicated that there are many variables related to the characteristics of farmers and their exploitations included in the analyses of causality of adoption in the agricultural field (such as farmer age, education, dedication to agriculture, access to information channels, opinions and environmental attitudes, farm size, and other characteristics) that are relevant to the adoption decision of more sustainable practices in agriculture. Jha et al. (Citation2021) and Bonke and Musshoff (Citation2020) have shown that farmers’ environmental attitudes and behaviours strongly influence their decisions to adopt sustainable agricultural technologies. Furthermore, it has been demonstrated that the attitude of farmers towards the environment can be an extended dimension of the TPB, which, combined with other determinant factors, should be a significant construct explaining farmers’ intentions and behaviours (Ahmed et al., Citation2021). Burli et al. (Citation2021) argue that farmers’ decisions to adopt or not adopt sustainable solutions depend not only on their environmental attitudes and opinions but also on different attributes, mainly related to the innovation, farm, and farmers themselves. The initial investment, expected benefits, maintenance costs, and farm structure as well as the farmers’ socioeconomic characteristics and preferences towards alternative sustainable actions are also significant (Burli et al., Citation2021). Alomia-Hinojosa et al. (Citation2018) and Iiyama et al. (Citation2018) have indicated that the decisions to adopt innovative agricultural technologies could be influenced by farmers’ preferences and the perceived advantages of the innovations. Barbarán (Citation2014) suggests that structural (age, education level, and farm size) and attitudinal variables are determining factors affecting the adoption of sustainable solutions at the farm level. Further, Läpple and Van Rensburg (Citation2011) argue that environmental attitudes and social learning are important determinants of adoption decisions. Borges et al. (Citation2019) have indicated that the underlying motivations for innovation adoption may also involve psychological constructs in addition to economic aspects.
The activities of the agricultural sector in Europe are highly regulated, with some of these regulations potentially playing important roles in farmers’ adoption decisions. Many new technologies and innovations have already been developed in the European agricultural sector. However, not all the proposed innovative solutions are focused on circularity as a means of improving resource efficiency and the re-use and recovery of nutrients (such as manure treatment and application).
Recently, circularity has gained relevance within the European agriculture system. However, studies focusing on innovation adoption decisions that introduce circularity at the farm level are still in an initial stage. Given this background, there is a clear need to delve deeper to provide updated answers to this identified gap. At this point, it is important to determine the factors affecting European farmers’ decision-making regarding circular agricultural innovations, including their profile, psychological aspects including their environmental attitudes and preferences, and other economic aspects related to the innovations.
3. Materials and methods
Data was collected from March to August 2021 using a specifically designed face-to-face survey presented to farmers in four EU countries (), where four innovative solutions focused on circular agriculture were developed as part of the H2020 European project (Circular Agronomics, Citation2018). The target population of the study consisted of livestock farmers dedicated to porcine or bovine production located in specific case study regions; they represented the different biogeographic conditions and environmental challenges typically present in the agricultural sector. A simple random sampling procedure was carried out based on the proximity of producers to the research centres in regions where a pilot design (demo) of the innovation was available for demonstration (Institute of agrifood research and Technology, Catalonia, Spain; Lungau, Austria; University of Milano, Emilia Rogmana, Italy; and South Moravia, Czech Republic). The reliability of the sample was mainly based on the inclusion criteria of farm size and socioeconomic variables, including age and gender, to ensure heterogeneity. The innovations presented to the surveyed farmers were: ‘fertigation with microfiltered slurry/digestate’, ‘thermal/solar dryer’, ‘precision feeding’, and ‘low-input farming’. All solutions focused on reducing nutrient loss at the farm level and improving environmental sustainability.
A semi-structured questionnaire was designed with an interdisciplinary perspective. It included a wide-ranging set of questions grouped into two main sections. The first section focused on socioeconomic variables – farmer characteristics, farm structural characteristics, and farm management – and psychographic and attitudinal constructs, such as agribusiness objectives, environmental attitudes, and opinions. The second section contained a brief innovation description including technical and economic information (cost and benefit outcomes) and a short video illustrating the technology associated with the four circular solutions mentioned (). In addition, this section included questions related to the potential adoption of the proposed circular agriculture innovations. The survey was translated into the local language of each study region and applied to farmers between March and August 2021.
Table 1. Circular innovations designed and analyzed in the Circular Agronomic project.
The agribusiness objectives of farmers were analyzed using the AHP, a multicriteria technique used to identify social, economic, and environmental preferences. The NEP scale was used to assess farmers’ environmental attitudes, and the logit model was used to analyze farmers’ reactions to the potential adoption of the proposed innovations as well as the factors affecting their decision. summarizes the developed methodological approach.
3.1. Analytic hierarchy process (AHP)
The AHP method was used to identify farmers’ objectives. The AHP is a multicriteria analysis tool (Saaty, Citation2001) widely used in agricultural research (Cabello, Citation2017; Kallas & Gil, Citation2012). The AHP allows for the comparison of tangible and intangible factors by setting priorities (Arriaza & Nekhay, Citation2010). Compared to other preference methods, the advantage of the AHP as a simple multicriteria decision method is that it is based on simple pairwise comparisons, making the preferences of the compared elements easier for the interviewee to discern (Kallas et al., Citation2011). Furthermore, its calculation does not require large samples, and preference scores can be determined at the individual level. The scores obtained, unlike those in more complex elicitation methods, represent the relative importance or load of each analyzed item regarding environmental, economic, and social objectives at the farm level. The AHP method involves three stages: (1) modelling, (2) evaluation, and (3) prioritization and synthesis (Torres et al., Citation2020). In the modelling stage, three factors corresponding to each objective were defined based on a literature review. The reviewed studies focused on agricultural and livestock production as well as management alternatives used in agricultural production systems (De Roest et al., Citation2018; Payraudeau & van der Werf, Citation2005; Rafaj et al., Citation2018; Sánchez-Toledano et al., Citation2017), as shown in .
In the evaluation stage, pair-wise comparisons of all the farmer objectives within each level (a, b, and c) were performed using the verbal AHP scale of 9 point proposed by Saaty (Citation2001), (e.g. ask farmer choice between the economic objective number 1 ‘Diversify production and marketing channels’ and the economic objective number 2 ‘Increase the sales of farm products’. If both have the same importance assig the value 1, if not, the interviewed farmer has to assign the level of preference (from 1 to 9) to the selected objective).
The meanings of different values in the scale can be summarized as follows: one indicates that both criteria are equally important, while two means that the selected criterion has a slightly higher importance than the other. This importance increases up to nine, which indicates that the selected criterion has absolute importance with respect to the other.
In the prioritization and synthesis stages, all comparisons and priorities were estimated using judgments (âijk). These are the comparison values of objective i against objective j for farmer k from the Saaty matrices (Âk). The obtained values were normalized, and their relative importance was calculated for all farmers (Kallas & Gil, Citation2012).
3.2. Farmers’ environmental attitude
The NEP scale was used to analyze the farmers’ environmental attitudes. This has allowed researchers to identify pro-environmental and anthropocentric attitudes in previous studies (Moyano Díaz & Palomo Vélez, Citation2014), resulting in the incorporation of several constructs as part of general environmental awareness measurement systems (Vozmediano Sanz & San Juan Guillén, Citation2005). The NEP scale integrates different items that involve two latent dimensions: ‘ecocentric’ and ‘anthropocentric’ attitudes () (Gomera et al., Citation2013).
Table 2. Environmental attitudes based on the New Ecological Paradigm scale (Torres el al., 2020).
The NEP scale statements were evaluated on a Likert scale consisting of nine points, from ‘strongly disagree’ (number one) to ‘strongly agree’ (number nine). Principal Component Analysis (PCA) was applied using the Statistical Package for the Social Sciences (SPSS version 26.0). PCA was performed to extract two factors (eigenvalues higher than one unit) with varimax rotation to regroup the items in the two major dimensions. The suitability of the data for the application of the reduction technique was tested using the Kaiser-Meyer-Olkin (KMO) test; values greater than 0.6 indicated that the technique could be applied (Samian et al., Citation2015). Latent dimensions associated with ‘ecocentric’ and ‘anthropocentric’ attitudes were identified. Ecocentric attitudes were those related to an individual’s commitment to environmental preservation. Individuals with ecocentric attitudes display concerns regarding ecosystems; their values, behaviours, and beliefs are aligned with environmental protection. Conversely, anthropocentric attitudes relate to the belief that natural resource exploitation involves valuing the environment for its benefits to people and reflect anthropocentrism as the most important component of life (Simsar et al., Citation2021). Both dimensions describe farmers’ predominant environmental attitudes, with different signs indicating attitude clarity. A clear ecocentric attitude (+, – ), indicates that a given farmer agrees with ecocentric items and disagrees with anthropocentric items, whereas a clear anthropocentric attitude (-, +) corresponds to a farmer that disagrees with ecocentric items and agrees with anthropocentric items. When the farmer shows an undefined attitude, both values are given the same sign (+, + or –, – ) (Torres et al., Citation2020).
3.3. Farmers’ decision to adopt the circular farming solutions
The logit model was implemented to estimate the likelihood of farmers adopting the proposed circular innovations (Luna-Mena et al., Citation2016). The Logit Model is a nonlinear, binary choice model that assumes that individuals choose between two alternatives with a logistic distribution (McFadden, Citation1973). This model has allowed researchers to estimate the probability of an event occurring based on one of two values (0 = would not adopt; 1 = would adopt), using the maximum likelihood method (Cramer, Citation1999; Maddala, Citation1983).
The probability model depends on the parameters' vector
which represents the explanatory factors described above: NEP, AHP outcome, farmers and farm characteristics, attitudinal opinions, and innovation description.
where Y represents an adoption decision, (Y = 1) corresponds to an adoption decision regarding the proposed technology, and (Y = 0) corresponds to the non-adoption of the proposed technology
are explanatory variables.
The empirical specification of the logit model is:
and the logistics distribution function model is:
The probability that adoption occurs is given by:
where
is an intercept, and
are the coefficients of the independent variables representing their impact on the likelihood of the adoption of circular agronomic innovations.
Considering the natural logarithms in the previous expression, a linear expression model was obtained:
Explanatory variables were identified from a literature review and selected using Pearson's correlation analysis on SPSS (version 24.0). Highly correlated independent variables and those identified as significant in previous studies were included in the first regression model. The Wald backward and forward methods were used for the final set of selected variables, retaining only the significant variables in the model. The Hosmer–Lemeshow goodness-of-fit test was used to evaluate the global fit of the model. This statistic is used in logistic regression for models with continuous covariates and small sample sizes (Botero Soto, Citation2021). In addition, the deviation (‘–2LL’ = 2 log-likelihood) that measures how well the model fits the data was estimated. Cox and Snell's R-squared test was used to estimate the proportion of variance explained by the independent variables, and Nagelkerke's R-squared metric was used to correct the scale of the statistic to cover the full range from 0 to 1.
4. Results
A first analysis was carried out to understand the farmers’ intention to adopt (‘adopters’) or not (‘non-adopters’) in terms of their demographic and personal characteristics, farm income, and physical and geographic characteristics of their farms. The results () showed that the adopters were almost exclusively male farmers, whereas the gender composition of non-adopters was more balanced, with a statistically significant difference between the two groups. Older farmers were also better represented among adopters, highlighting the correlation between the time a farmer has been involved in farming activities and their likelihood of adoption. Furthermore, the results showed that agricultural training and professional agricultural education were highly heterogeneous across the two farmer groups. Adopters had a slightly higher proportion of farmers with university degrees, while the percentage of farmers with only practical experience was higher among non-adopters. Additionally, a higher proportion of farmers with previous adoption experience demonstrated a tendency to attend various courses, conferences, and workshops related to farming activities. However, the differences between proportions of adopters and non-adopters were not statistically significant.
Table 3. General characteristics of adopters versus non-adopters (past 10 years).
The proportion of farmers belonging to an agricultural association was almost twice as high among adopters than among non-adopters, with a statistically significant difference. Adopters were more active members of professional associations potentially seeking cooperation with other farmers to share the risks and economic burden associated with implementing certain innovations. A higher proportion of adopters were farmers whose unique economic activity was agriculture compared to non-adopters, who exhibited other off-farm income sources.
Regarding the economic variables, the results of the survey showed a higher proportion of adopters belonging to vertically integrated companies and a stronger reliance on loans than that of non-adopters. The loans of adopters were mainly related to investments in construction, machinery, and equipment, whereas those of non-adopters were associated with ensuring cash flows and financing daily operations. Adopters tended to diversify their marketing channels by selling to more clients than non-adopters. Furthermore, the proportion of large farms (> 75 ha) was higher in the adoption group than that in the non-adoption group. Finally, soil quality and fertility, measured by farmers’ subjective estimates on a 10-point Likert scale, did not play a significant role in farmers’ innovation decisions.
4.1. Farmers’ preferences and objectives at the farm level
The AHP results () showed that, at the farm level, farmers prioritized economic aspects over environmental and sociocultural objectives, reaching a cumulated relative importance of 68.33%, 28.66%, and 3.01%, respectively. The ‘maximization of farm net profit’ objective was assigned the highest relative importance, while the ‘preserving sociocultural values’ and ‘preventing rural areas depopulation’ objectives were assigned the lowest. The ‘relative importance’ of all preferences were obtained through corresponding Saaty matrices structured based on farmers’ comparisons between the elements of each conglomerate and using the row geometric mean method.
4.2. Farmers environmental attitudes
The KMO test was applied to the NEP scale items. The results showed a KMO value of 0.75, indicating the goodness of fit of the PCA methodology. The internal consistency of the NEP scale (Cronbach's alpha) was 0.74, indicating adequate consistency. Two components were extracted from the PCA: ‘ecocentric’ and ‘anthropocentric’ factors, with a total explained variability of 51.4%. The environmental attitudes of farmers were determined based on both dimensions.
The results (see ) showed that only 28% of the farmers surveyed exhibited a predominantly ecocentric attitude, with the majority of these considering that nature should be protected regardless of any potential direct benefits. An anthropocentric attitude was displayed by 20% of the farmers, agreeing with the statement that protecting nature should only be done to improve the quality of human life. Furthermore, they agreed that human beings are able to control nature and their resources. The remaining farmers (52%) were identified as having undefined or contradictory environmental attitudes, exhibiting both ecocentric and anthropocentric attitudes. In all cases, the ecocentric dimension was more important than the anthropocentric dimension for all farmers.
A graphical representation of the distribution of farmers’ environmental attitudes can be seen in . The points below the blue dotted line represent farmers with a predominantly ecocentric environmental attitude, whereas those above it corresponds to farmers with a more anthropocentric attitude.
This figure also shows whether the environmental attitude that characterizes each farmer was clearly defined, depending on the quadrant in which farmers are located. As shown in the figure, the farmers who exhibited an ecocentric attitude (dots below the diagonal line) are mostly willing to adopt the innovations presented (ratio of green dots to red dots = 6.75). While those who exhibited an anthropocentric attitude (points above the blue line), are less willing to adopt these innovations (ratio of green dots = 1.45 compared to red dots).
4.3. Factors affecting the adoption decision
The factors affecting the decision to adopt were analyzed using a binary logistic regression method. The dependent variable describing the potential adoption was dichotomous (would adopt = 1; would not adopt = 0). The independent variables used in the model corresponded to socioeconomic characteristics, and environmental attitudes and preferences about objectives. Variables included in the model were considered based on their significance in the theory of innovation adoption and empirical studies from the literature review. The model goodness of fit was R2 Nagelkerke = 0.607, explaining 60.7% of the variance. The Hosmer–Lemeshow test results (Chi square = 7.986; df = 8; p-value = 0.435), indicated the prediction capacity of the model is highly reliable.
The results in , showed that the presence of ‘off-farm income’ reduced farmers’ probability to adopt the proposed circular agriculture innovations This could be because farmers with additional sources of income are less dependent on farm activities. Similar results were identified by Genius et al. (Citation2006). They found that income from nonfarm business activities and employment in other nonfarm sectors negatively influenced the probability of innovation adoption. ‘Farm size’ positively influenced farmers’ adoption decisions. As large farms have more economic resources, they can better meet adoption costs and have a greater need to use their resources efficiently. Läpple and Van Rensburg (Citation2011) and Taghouti et al. (Citation2021) obtained similar results, indicating that farm size is a robust and positive predictor of adoption over time. The location of a farm in a ‘vulnerable area’ owing to nitrate contamination increased the likelihood of implementing new solutions and practices. This is because low fertility and unfavourable soil conditions may encourage farmers to look for technologies that help them tackle such problems and allow them to better adapt to the restrictions of vulnerable areas. This outcome aligns with the results obtained by Tadesse and Belay (Citation2004), who found that farmers’ decisions to adopt soil conservation measures were positively influenced by their perception of soil erosion. According to Tey et al. (Citation2014), farmers’ vulnerability generates a greater probability of adopting solutions that reduce a problem. The ‘trust in family and friends’ variable was found to significantly undermine the probability of adoption.
Table 4. Logistic regression analyses.
Farmers’ experience regarding the adoption of innovations in the last 10 years had a positive effect on the adoption of the proposed circular farming solutions. This may be related to the fact that producers take advantage of positive experiences from previous adoptions and are, therefore, more prone to adopt new technologies. Zhou et al. (Citation2008) also found that the experience of previous adoption of similar technologies had a positive effect, as farm administrators had become well-acquainted with the technology and benefited from its adoption. The use of diversified devices and infrastructure as solutions for ‘slurry management’ at the farm level had a positive effect on the probability of adoption when compared with farmers who relied on a single infrastructure solution (such as a tank deposit of slurry). Farmers’ tendency to investigate and implement improved solutions for slurry use increased the likelihood of adoption, similar to how the adoption experience of farmers increased the probability of new adoption. Tank volume had a negative effect on adoption decisions. This could be because larger tanks may last longer and require fewer modifications, thereby, reducing the need to adopt other innovations.
The analysis of the farmers’ opinions showed that adoption probability increases when farmers agree with the statement ‘the government should encourage innovations’ in agriculture (84% of farmers believe that the government and public institutions should encourage the implementation of new technologies in agriculture through subsidies, tax benefits, and other measures). Contrastingly, farmers with the opinion that ‘non-farm income is necessary to the financial security of the farm’ were less likely to adopt innovation. Additionally, a higher number of working-age members (19–59 years old) increased the probability of adopting the proposed innovations.
Variables related to farmers’ training and education – practical experience, vocational training, and university training – were highly significant in explaining their adoption decisions, with university training showing the strongest positive effect. Similarly, Dhraief et al. (Citation2019), Akudugu et al. (Citation2012), and Mzoughi (Citation2011) found that education had a significant effect on farmers’ adoption of modern agricultural production technologies. Regarding farmers’ environmental attitudes, a clearly defined ecocentric attitude increased the probability of adopting the proposed circular agricultural innovations. Similarly, a study on the adoption of nutrient management practices among farmers in the Irish Republic showed that ecocentric attitudes increased the adoption of a greater number of nutrient management practices. In contrast, farmers identified as having anthropocentric attitudes were found to be more likely to place greater emphasis on the economics of environmental issues and were less likely to adopt nutrient management practices (Buckley et al., Citation2015).
Furthermore, the analysis of farmers’ preferences with regard to the sustainability objectives, estimated through the AHP method, showed that farmers gave the highest relative importance to the maximization of benefits and the lowest to sociocultural objectives. This is consistent with the results reported by Sánchez-Toledano et al. (Citation2017). These sustainable objective preferences were demonstrated to be relevant when it comes to understanding farmers’ adoption decisions. Prioritization of the economic objectives ‘improve production diversification and distribution channels’ and ‘maximize net profits by reducing cost’ significantly reduced the odds of adopting the proposed innovations. In the former case, the results may be explained by the fact that implementing sustainable technologies, such as solar dryers or nano-filters, are two competing objectives that require considerable capital investment and are not focused on increasing farm productivity. According to Lozano Cabedo (Citation2013), farmers consider organic farming systems to be less profitable than traditional ones owing to the lower yield obtained without considering other issues such as receiving complementary subsidies or obtaining higher revenue from the product. He also argued that farmers consider organic farming to require an increased workload, time, and dedication, all of which raise costs. In the latter case, the goal of maximizing net profits by reducing costs also affects investment costs, leading to the adoption of fewer innovations. The lack of valuation of environmental goods and predominance of economic objectives decrease the chances of adopting sustainable technologies. Thus, the economic dimension prevails when it comes to adopting unsustainable systems (Blandi et al., Citation2018).
Finally, the model estimation showed that the prioritization of environmental goals increased the probability of adoption. These results agree with those of Sánchez-Toledano et al. (Citation2017), who found that farmers with greater interest in ecological objectives are more likely to adopt sustainable technological innovations. This finding may be justified by farmers implementing sustainable technologies to achieve their environmental goals and improve their compliance with environmental regulations.
5. Discussion
The need to implement actions that promote the reduction of emissions and allow a more efficient use of resources in agricultural farming systems has led to the generation of innovative technological solutions. However, the effectiveness of these innovations mainly depends on their acceptance by farmers, initial investment, and ease of use. Understanding the adoption process and factors affecting farmers’ preferences and decisions may facilitate the implementation of potential improvements related to both agricultural sustainability and efficiency. The adoption of agricultural innovations depends on farmers’ socioeconomic factors as well as their environmental attitudes and preferences. It further depends on the characteristics of the innovation as well as the availability of subsidies and support from public institutions for accompanying farmers during the process, among other factors highlighted in the literature. According to Serebrennikov et al. (Citation2020), the adoption of innovations may differ between regions, depending on customs, environmental conditions, and regulations. For small farmers, the adoption of innovations represents a great effort given the lack of information on new technologies and high costs of operating and implementing them, which are the main barriers to adoption. In our study, the factor that contributed the most to the adoption behaviour of circular innovations to reduce emissions and improve resource efficiency was farmers’ prioritization of environmental objectives over social or economic objectives. Furthermore, the adoption behaviour was clearly related to ‘ecocentric’ attitudes. Education and experience were also of high importance as proxies for farmers’ subjective knowledge of the level of farming activities. Akudugu et al. (Citation2012) argued that the number of years of formal schooling creates a favorable attitude towards the acceptance of new practices, which is why the complexity of technologies has a negative effect on the decision to adopt in farmers with lower education. Pathak et al. (Citation2019) indicated that the ability of a farmer to use new technologies acquired through education, training, and experience increases the likelihood of adopting agricultural technology. These empirical findings indicate that attitudes and preferences play an important role in predicting the intention to adopt, reflecting that farmers’ concerns about the environment promote the adoption of circular innovations. Our results are similar to those of previous studies that focused on the adoption of more environmentally friendly production systems, such as organic farming (Genius et al., Citation2006; Koesling et al., Citation2008; Läpple & Van Rensburg, Citation2011; López-Felices et al., Citation2023; Meijer et al., Citation2015; Mzoughi, Citation2011).
The findings of this study provide an initial roadmap for policymakers when establishing action schemes aimed at reducing GHG emissions at the farm level. It showed the importance of raising awareness among farmers about the benefits of implementing circular agriculture at both economic (improving efficiency) and environmental levels by promoting strong resource reuse policies, such as using biofertilizers. Such promotion can also be achieved through training, accompanying farmers during and after the adoption process, and disseminating good practices. Supporting farmers’ transition to more sustainable production systems using circular innovations is crucial because of the novelty and lack of experience in applying such innovations.
6. Conclusions
Studies focusing on the factors affecting circular innovation adoption on farms in the EU are lacking. This study addressed this gap in knowledge by analyzing farmers’ opinions, environmental attitudes, expectations, agribusiness objectives, and acceptance of several circular solutions and technologies aimed at closing the loop of nutrients and reducing emissions at the farm level.
The factors identified as having the greatest impact on farmers’ decisions to adopt circular agricultural innovations were environmental objectives, education level (university education), previous innovation adoption experience, and clearly defined ecocentric attitudes. Other factors, such as locations in vulnerable areas, also significantly increased the probability of adoption.
The results showed that the majority of farmers (84%) believe that government and public institutions should encourage the implementation of new technologies in agriculture through direct payments that support investments in emission reduction solutions and through tax reduction schemes. This is in accordance with the EU policy, where investments are made in emission reduction and mitigation practices through the CAP, as these are considered essential for the promotion of agricultural sustainability.
This finding allows us to suggest that a climate change mitigation and adaptation intervention scheme should be designed to endorse farmers in their decision to adopt the requisite innovations (e.g. structural one-off subsidies for investment in sustainable innovations/technologies). Additionally, with the CAP support, intervention tools for emission reduction in the livestock sector should be introduced through various measures, including yearly subsidies to cover the operating costs of emission-reducing innovations and lower-tax schemes for low GHG emissions and nutrient recovery. (e.g. through fertilizer type modifications, farm circularity implementation, and nutrient recycling).
Farmers’ characteristics, environmental attitudes, and preferences for agribusiness objectives are relevant factors that should be considered when implementing agricultural policies that better fit their needs and increase the adoption of sustainable innovations and technologies. One of the main implications of public policies aimed at improving and promoting the adoption of circular agricultural innovations is the strengthening of innovation dissemination processes. This is because access to information and knowledge promotes a greater capacity or willingness to adopt technological solutions in terms of circular agriculture, as evidenced by the present research findings. Previous experience and a high level of training increase the likelihood of adopting innovation. Factors that motivate farmers to increase the adoption of circular farming innovations, such as farmers’ objective preferences, may assist policymakers in designing more specific and efficient policy measures that can help farmers face changing social needs and current environmental challenges, including the reduction of GHG emissions and improved nutrient recovery within EU farms.
Although the sample procedure and data collection in this study were considered to be reliable, the results obtained from this study should be interpreted with caution, considering the relatively limited sample size within each case study and the hypothetical nature of the adoption decision. Exhibiting a potential acceptance of the innovations analyzed does not necessarily translate to real-life adoption. Furthermore, the moment in which data collection took place, in the recent Post-Covid period, could have contributed to creating a specific occasion and context that may have affected farmers’ perceptions and interests. Additionally, the results should be interpreted only in light of the specific circular innovations analyzed in this research, which were developed within the case studies and presented to farmers in demo sites. In this context, it is worth mentioning the need for future research to extend this study to other circular innovations, technologies, or practices and classify factors affecting adoption based on the complexity level of each circular innovation. Furthermore, there is a need to widen the sample size, not only in the regions analyzed but also in other countries in Europe, since policy interventions and regulations are adopted at the EU level, affecting all European member countries. Agri-food sectors other than livestock farming should also be assessed to carry out a heterogeneity analysis regarding the adoption of circular farming. From a methodological perspective, other adoption models and theories could also be tested, such as the Technology Acceptance Model (Mohr & Kühl, Citation2021), the unified theory of acceptance and use of technology (Ronaghi & Forouharfar, Citation2020) and the adoption model based on the extended TPB.
Authors' contributions
Conceptualization and formal analysis, writing – review and editing S.O.H, methodology, review and supervision Z.K, review and editing D.S. and F.T. review and supervision S.N.M. All authors have read and agreed to the publication of this manuscript.
Availability of data and material
The dataset generated and analyzed in the current study is available at Zenodo, a public repository [https://doi.org/10.5281/zenodo.7620395].
Code availability
Not applicable
Consent to participate
The survey in which this study is based was anonymous. Survey participation was voluntary.
Consent for publication
Participants were informed about the use, privacy, and confidentiality of the obtained data, and informed consent was obtained through an online survey. All authors accept full responsibility for all the aspects of the work described.
Ethics approval
The Ethics Committee of the Polytechnical University of Catalunya had no objections to the publication of the results of this study.
Acknowledgments
The authors thank to all farmers for their participation and to our partners for their support and guidance throughout this project.
Disclosure statement
No potential conflict of interest was reported by the author(s).
Additional information
Funding
Notes on contributors
Selene Ivette Ornelas Herrera
Selene Ornelas: Selene is a Computer Systems Engineer from the ITCH II, she completed master's studies in administration at the UACH (México) and a master's degree in Statistics and Operations Research at the Polytechnic University of Catalonia. Currently she is pursuing a Doctorate in Sustainability at the UPC in Barcelona, Spain. Where additionally, she works as a research project technician, collaborating in European projects focused on the field of rural development, where innovation and technology transfer are promoted to reduce greenhouse gas emissions and recover nutrients in the agrifood sector.
Zein Kallas
Zein Kallas: Zein holds a PhD in Agri-food Economics and is a full professor at the School of Agri-food Engineering and Biosystems of Barcelona (EEABB) of the Polytechnic University of Catalonia (UPC). For many years, Zein has been involved in several areas of consumer research, with a focus on preferences and acceptance of innovations in healthy foods. Since joining CREDA in 2006, his interest lies primarily in consumer behavior research. In particular, the decision to buy food products and the behavior toward healthier consumption, the acceptance of functional foods and sensory science. Previously, his research interests included the economic assessment of the multifunctionality of extensive agricultural systems and the impact of agricultural policy on farmers' decisions.
Dmytro Serebrennikov
Dmytro Serebrennikov: He studied at Purdue University (PhD in Agricultural Economics). His Research interests are applied economics with a focus on behavioural attitudes of food producers & consumers. Sustainable agriculture & analysis of policy instruments and interventions (taxation, export/import restrictions, knowledge transfer programs).
Fiona Thorne
Fiona Thorne: She has a B.Agr.Sc and PhD (agricultural economics) both from UCD. Currently she is research officer at Teagasc and the current projects she is working on are competitiveness of Irish Agriculture; Farm level economics and policy analysis of crop production in Ireland; A Review of the Financial Status of Farms and Future Investment Requirements; Productivity analysis of Irish dairy production; Risk analysis in Irish agriculture.
Sinéad N. McCarthy
Sinéad N. McCarthy: PhD. Public Health Nutrition (UCC/TCD 2003) and M.Sc. Food Science and Technology (UCC 1996). For many years Sinéad has been involved in many areas of nutrition and consumer research, with a focus on food and health. Since joining Teagasc in 2007, her interest lies mainly in consumer behavior research in relation to food and health. Current projects include consumer food choice and health behavior, consumer acceptance of novel food technologies, functional foods, drivers of cheese consumption and sensory science. Prior to this her earlier research interests included nutritional physiology, public health nutrition and dietary intervention design and management.
References
- Abegunde, V. O., Sibanda, M., & Obi, A. (2019). Determinants of the adoption of climate-smart agricultural practices by small-scale farming households in King Cetshwayo District Municipality, South Africa. Sustainability, 12(1), 195. https://doi.org/10.3390/su12010195
- Ahmed, N., Li, C., Khan, A., Qalati, S. A., Naz, S., & Rana, F. (2021). Purchase intention toward organic food among young consumers using theory of planned behavior: Role of environmental concerns and environmental awareness. Journal of Environmental Planning and Management, 64(5), 796–822. https://doi.org/10.1080/09640568.2020.1785404
- Ajzen, I. (1991). The theory of planned behavior. Organizational Behavior and Human Decision Processes, 50(2), 179–211. https://doi.org/10.1016/0749-5978(91)90020-T
- Ajzen, I., & Fishbein, M. (1980). Understanding attitudes and predicting social behaviour. Prentice-Hall.
- Akudugu, M. A., Guo, E., & Dadzie, S. K. (2012). Adoption of modern agricultural production technologies by farm households in Ghana: What factors influence their decisions? Journal of Biology, Agriculture and Healthcare, 2(3), 1–13.
- Albarracín-Vacca, D. A. (2022). Régimen de prestación del suministro de energía eléctrica para cumplir el Pacto Verde Europeo [Bachelor's thesis]. Universidad Internacional de La Rioja.
- Alomia-Hinojosa, V., Speelman, E. N., Thapa, A., Wei, H. E., McDonald, A. J., Tittonell, P., & Groot, J. C. (2018). Exploring farmer perceptions of agricultural innovations for maize-legume intensification in the mid-hills region of Nepal. International Journal of Agricultural Sustainability, 16(1), 74–93. https://doi.org/10.1080/14735903.2018.1423723
- Arriaza, M., & Nekhay, A. (2010). Metodología multicriterio para la integración de las preferencias de la sociedad en la gestión del territorio agrícola: aplicación al olivar de baja producción. Revista Española de Estudios Agrosociales y Pesqueros, 226(2), 39–69.
- Barbarán, L. S. A. (2014). Análisis de la adopción tecnológica de técnicas agrícolas orgánicas para productores de café. Natura@ Economía, 2(1), 71–91. https://doi.org/10.21704/ne.v2i1.1429
- Blandi, M. L., Rigotto, R. M., & Sarandón, S. J. (2018). Influencia de factores contextuales en la adopción de modelos de agricultura insustentables: La incorporación del invernáculo en agricultores platenses. Revista de la Facultad de Ciencias Agrarias. Universidad Nacional de Cuyo, 50(1), 203–2016.
- Bonke, V., & Musshoff, O. (2020). Understanding German farmers’ intention to adopt mixed cropping using the theory of planned behavior. Agronomy for Sustainable Development, 40(6), 48. https://doi.org/10.1007/s13593-020-00653-0
- Borges, J. A. R., Lansink, A. G. O., & Emvalomatis, G. (2019). Adoption of innovation in agriculture: A critical review of economic and psychological models. International Journal of Innovation and Sustainable Development, 13(1), 36–56. https://doi.org/10.1504/IJISD.2019.096705
- Botero Soto, Y. (2021). Adopción de tecnologías por parte de los agricultores del municipio de La Ceja – Oriente de Antioquia [Bachelor's thesis]. Universidad EIA.
- Buckley, C., Howley, P., & Jordan, P. (2015). The role of differing farming motivations on the adoption of nutrient management practices. International Journal of Agricultural Management, 4(1029-2017-1508), 152–162. https://doi.org/10.22004/ag.econ.262378
- Burli, P. H., Nguyen, R. T., Hartley, D. S., Griffel, L. M., Vazhnik, V., & Lin, Y. (2021). Farmer characteristics and decision-making: A model for bioenergy crop adoption. Energy, 234, 121235. https://doi.org/10.1016/j.energy.2021.121235
- Cabello, H. A. (2017). Métodos de decisión multicriterio y sus aplicaciones. The project. Retrieved March 7, 2022, from https://www.circularagronomics.eu/the-project/
- CEE. (2020). European Economic Commission, the common agricultural policy at a glance. Retrieved April 5, 2022, from https://ec.europa.eu/info/food-farming-fisheries/key-policies/common-agricultural-policy/cap-glance_en
- Circular Agronomics. (2018). Circular agronomics. The project. Retrieved July 7, 2022, from https://www.circularagronomics.eu/the-project/
- Cramer, J. S. (1999). Predictive performance of the binary logit model in unbalanced samples. Journal of the Royal Statistical Society: Series D (The Statistician), 48(1), 85–94. https://doi.org/10.1111/1467-9884.00173
- Cuevas, R. V., Baca, M. J., Cervantes, E. F., Espinosa, G. J. A., Aguilar, ÁJ, & Loaiza, M. A. (2013). Factors which determine the use of innovation technology in dual purpose cattle production units in Sinaloa, México. Revista mexicana de ciencias pecuarias, 4(1), 31–46.
- De Roest, K., Ferrari, P., & Knickel, K. (2018). Specialization and economies of scale or diversification and economies of scope? Assessing different agricultural development pathways. Journal of Rural Studies, 59, 222–231. https://doi.org/10.1016/j.jrurstud.2017.04.013
- Despotović, J., Rodić, V., & Caracciolo, F. (2019). Factors affecting farmers’ adoption of integrated pest management in Serbia: An application of the theory of planned behavior. Journal of Cleaner Production, 228, 1196–1205. https://doi.org/10.1016/j.jclepro.2019.04.149
- Dhraief, M. Z., Bedhiaf, S., Dhehibi, B., Oueslati-Zlaoui, M., Jebali, O., & Ben-Youssef, S. (2019). Factors affecting innovative technologies adoption by livestock holders in arid area of Tunisia. New Medit: Mediterranean Journal of Economics, Agriculture and Environment = Revue Méditerranéenne d'Economie Agriculture et Environment, 18(4), 3–18. https://doi.org/10.30682/nm1904a
- Directive 91/676/CEE. (2021). Protección de las aguas contra la contaminación producida por nitratos utilizados en la agricultura. Directiva 91/676/CEE del Consejo.
- Dorr, E., Koegler, M., Gabrielle, B., & Aubry, C. (2021). Life cycle assessment of a circular, urban mushroom farm. Journal of Cleaner Production, 288, 125668. https://doi.org/10.1016/j.jclepro.2020.125668
- Eurostat. (2021). Eurostat Statistics Explained. Greenhouse gas emissions by economic activity, 2021 (thousand tonnes of CO2 equivalents).
- Fan, W., Dong, X., Wei, H., Weng, B., Liang, L., Xu, Z., Wang, X., Wu, F., Chen, Z., Jin, Y., & Song, C. (2018). Is it true that the longer the extended industrial chain, the better the circular agriculture? A case study of circular agriculture industry company in Fuqing, Fujian. Journal of Cleaner Production, 189, 718–728. https://doi.org/10.1016/j.jclepro.2018.04.119
- Feder, G., Just, R. E., & Zilberman, D. (1985). Adoption of agricultural innovations in developing countries: A survey. Economic Development and Cultural Change, 33(2), 255–298. https://doi.org/10.1086/451461
- Genius, M., Pantzios, C. J., & Tzouvelekas, V. (2006). Information acquisition and adoption of organic farming practices. Journal of Agricultural and Resource Economics, 31(1), 93–113.
- Gomera, A., Villamandos, F., & Vaquero, M. (2013). Construction of indicators of environmental beliefs from the NEP scale. Acción Psicológica, 10(1), 147–160. https://doi.org/10.5944/ap.10.1.7041
- Iiyama, M., Mukuralinda, A., Ndayambaje, J. D., Musana, B. S., Ndoli, A., Mowo, J. G., Garrity, D., Ling, S., & Ruganzu, V. (2018). Addressing the paradox–the divergence between smallholders’ preference and actual adoption of agricultural innovations. International Journal of Agricultural Sustainability, 16(6), 472–485. https://doi.org/10.1080/14735903.2018.1539384
- Jha, S., Kaechele, H., & Sieber, S. (2021). Factors influencing the adoption of agroforestry by smallholder farmer households in Tanzania: Case studies from Morogoro and Dodoma. Land Use Policy, 103, 105308. https://doi.org/10.1016/j.landusepol.2021.105308
- Johnson, J. M. F., Franzluebbers, A. J., Weyers, S. L., & Reicosky, D. C. (2007). Agricultural opportunities to mitigate greenhouse gas emissions. Environmental Pollution, 150(1), 107–124. https://doi.org/10.1016/j.envpol.2007.06.030
- Juárez, J., Munuera Pérez, M. T., & Masdemont Hernández, B. (2021). Innovar para una agricultura circular. Única estrategia sostenible. XXXVIII Congreso Nacional de Riegos. https://doi.org/10.31428/10317/10146
- Kallas, Z., & Gil, J. (2012). Combining contingent valuation with the analytical hierarchy process to decompose the value of rabbit meat. Food Quality and Preference, 24(2), 251–259. https://doi.org/10.1016/j.foodqual.2011.11.006
- Kallas, Z., Lambarraa, F., & Gil, J. M. (2011). A stated preference analysis comparing the analytical hierarchy process versus choice experiments. Food Quality and Preference, 22(2), 181–192. https://doi.org/10.1016/j.foodqual.2010.09.010
- Knowler, D., & Bradshaw, B. (2007). Farmers’ adoption of conservation agriculture: A review and synthesis of recent research. Food Policy, 32(1), 25–48. https://doi.org/10.1016/j.foodpol.2006.01.003
- Koesling, M., Flaten, O., & Lien, G. (2008). Factors influencing the conversion to organic farming in Norway. International Journal of Agricultural Resources, Governance and Ecology, 7(1–2), 78–95. https://doi.org/10.1504/IJARGE.2008.016981
- Läpple, D., & Van Rensburg, T. (2011). Adoption of organic farming: Are there differences between early and late adoption? Ecological Economics, 70(7), 1406–1414. https://doi.org/10.1016/j.ecolecon.2011.03.002
- Le, C. C. (2010). The role of attitude, preference conflict, norms, and family identity in explaining intention/behavior toward fish consumption in Vietnamese families [Master's thesis]. Universitetet i Tromsø. https://hdl.handle.net/10037/2533
- Leiva, B. A., & Paulovich, C. L. (2021). Economía y Agricultura Circular como fuente de creación de valor empresarial para la industria agrícola [Doctoral dissertation]. Universidad Argentina de la Empresa.
- López, C. P., & Requena, J. C. (2005). Factors related to the adoption of organic farming in Spanish olive orchards. Spanish Journal of Agricultural Research, 3(1), 5–16. https://doi.org/10.5424/sjar/2005031-119
- López-Felices, B., Aznar-Sánchez, J. A., Velasco-Muñoz, J. F., & Mesa-Vázquez, E. (2023). Farmers’ profiles and attitudes towards the implementation of rainwater harvesting systems in intensive agriculture. International Journal of Agricultural Sustainability, 21(1), 2189402. https://doi.org/10.1080/14735903.2023.2189402
- López-Páez, V. P., & García-Herreros, M. (2021). Economía circular como tecnología social: una herramienta para el desarrollo sostenible del sector agropecuario lechero. In J. M. Palma García & J. F. C. Uribe (Eds.), Tecnologías Sociales en la Producción Pecuaria de América Latina y el Caribe (pp. 37–59). Colima.
- Lozano Cabedo, C. (2013). Social representations of the organic agriculture in Andalusia (Spain). Gazeta de Antropología, 29(2), 5. https://doi.org/10.30827/Digibug.28502
- Luna-Mena, B. M., Altamirano-Cárdenas, J. R., Santoyo-Cortés, V. H., & Rendón-Medel, R. (2016). Factores e innovaciones para la adopción de semillas mejoradas de maíz en Oaxaca. Revista Mexicana de Ciencias Agrícolas, 7(SPE15), 2995–3007.
- Maddala, G. S. (1983). Methods of estimation for models of markets with bounded price variation. International Economic Review, 24(2), 361–378. https://doi.org/10.2307/2648751
- Martínez, A. M., & Gómez, J. D. (2012). Elección de los agricultores en la adopción de tecnologías de manejo de suelos en el sistema de producción de algodón y sus cultivos de rotación en el valle cálido del Alto Magdalena. Ciencia y Tecnología Agropecuaria, 13(1), 62–70. https://doi.org/10.21930/rcta.vol13_num1_art:241
- McFadden, D. (1973). Conditional logit analysis of qualitative choice behavior. In P. Zarembka (Ed.), Frontiers in econometrics (pp. 105–142). Academic Press.
- Meijer, S. S., Catacutan, D., Ajayi, O. C., Sileshi, G. W., & Nieuwenhuis, M. (2015). The role of knowledge, attitudes and perceptions in the uptake of agricultural and agroforestry innovations among smallholder farmers in sub-Saharan Africa. International Journal of Agricultural Sustainability, 13(1), 40–54. https://doi.org/10.1080/14735903.2014.912493
- Mohr, S., & Kühl, R. (2021). Acceptance of artificial intelligence in German agriculture: An application of the technology acceptance model and the theory of planned behavior. Precision Agriculture, 22(6), 1816–1844. https://doi.org/10.1007/s11119-021-09814-x
- Moyano Díaz, E., & Palomo Vélez, G. (2014). Propiedades psicométricas de la Escala Nuevo Paradigma Ecológico (NEP-R) en población Chilena. Psico, 45(3), 415–423. https://doi.org/10.15448/1980-8623.2014.3.17276
- Mzoughi, N. (2011). Farmers adoption of integrated crop protection and organic farming: Do moral and social concerns matter? Ecological Economics, 70(8), 1536–1545. https://doi.org/10.1016/j.ecolecon.2011.03.016
- Nordin, S. M., Zolkepli, I. A., Rizal, A. R. A., Tariq, R., Mannan, S., & Ramayah, T. (2022). Paving the way to paddy food security: A multigroup analysis of agricultural education on circular economy adoption. Journal of Cleaner Production, 375, 134089. https://doi.org/10.1016/j.jclepro.2022.134089
- Ortiz Gutiérrez, B. E., González Forero, R., Fernández Lizarazo, J. C., & García Nieto, V. (2022). Agricultura circular: una estrategia sostenible para impulsar el agro colombiano. Revista de la Universidad de La Salle, 2021(87), 197–213. https://doi.org/10.19052/ruls.vol1.iss87.10
- Pata, U. K. (2021). Linking renewable energy, globalization, agriculture, CO2 emissions and ecological footprint in BRIC countries: A sustainability perspective. Renewable Energy, 173, 197–208. https://doi.org/10.1016/j.renene.2021.03.125
- Pathak, H. S., Brown, P., & Best, T. (2019). A systematic literature review of the factors affecting the precision agriculture adoption process. Precision Agriculture, 20(6), 1292–1316. https://doi.org/10.1007/s11119-019-09653-x
- Payraudeau, S., & van der Werf, H. M. (2005). Environmental impact assessment for a farming region: A review of methods. Agriculture, Ecosystems & Environment, 107(1), 1–19. https://doi.org/10.1016/j.agee.2004.12.012
- Poore, J., & Nemecek, T. (2018). Reducing food’s environmental impacts through producers and consumers. Science, 360(6392), 987–992. https://doi.org/10.1126/science.aaq0216
- Preston, B. L., & Jones, R. N. (2006). Climate change impacts on Australia and the benefits of early action to reduce global greenhouse gas emissions (p. 41). CSIRO.
- Rafaj, P., Kiesewetter, G., Gül, T., Schöpp, W., Cofala, J., Klimont, Z., Purohit, P., Heyes, C., Amann, M., Borken-Kleefeld, J., & Cozzi, L. (2018). Outlook for clean air in the context of sustainable development goals. Global Environmental Change, 53, 1–11. https://doi.org/10.1016/j.gloenvcha.2018.08.008
- Rehman, M. A., Fareed, Z., Salem, S., Kanwal, A., & Pata, U. K. (2021). Do diversified export, agriculture, and cleaner energy consumption induce atmospheric pollution in Asia? Application of method of moments quantile regression. Frontiers in Environmental Science, 9, 497. https://doi.org/10.3389/fenvs.2021.781097
- Rogers, E. M., & Ban, A. W. V. D. (1963). Research on the diffusion of agricultural innovations in the United States and the Netherlands. Sociologia Ruralis, 3(1), 38–49. https://doi.org/10.1111/j.1467-9523.1963.tb00419.x
- Ronaghi, M. H., & Forouharfar, A. (2020). A contextualized study of the usage of the Internet of things (IoTs) in smart farming in a typical middle eastern country within the context of Unified Theory of Acceptance and use of Technology model (UTAUT). Technology in Society, 63, 101415. https://doi.org/10.1016/j.techsoc.2020.101415
- Saaty, T. L. (2001). Fundamentals of the analytic hierarchy process. In D. L. Schmoldt, J. Kangas, G. A. Mendoza, & M. Pesonen (Eds.), The analytic hierarchy process in natural. Resource and environmental decision making (pp. 15–35). Springer. https://doi.org/10.1007/978-94-015-9799-9_2
- Samian, M., Mahdei, K. N., Saadi, H., & Movahedi, R. (2015). Identifying factors affecting optimal management of agricultural water. Journal of the Saudi Society of Agricultural Sciences, 14(1), 11–18. https://doi.org/10.1016/j.jssas.2014.01.001
- Sánchez-Toledano, B. I., Kallas, Z., & Gil, J. M. (2017). Importancia de los objetivos sociales, ambientales y económicos de los agricultores en la adopción de maíz mejorado en Chiapas, México. Revista de la Facultad de Ciencias Agrarias, 49(2), 269–287.
- Sarteel, M., Tostivint, C., Landowski, A., Basset, C., Muehmel, K., Lockwood, S., Ding, H., Oudet, N., Mudgal, S., Cherrier, V., Grebot, B., Naumann, S., Dooley, E., Lukat, E., Frelih Larsen, A., Wunder, S., Carter, M. S., Ambus, P., Provolo, G., … Michels, R. (2016). Resource efficiency in practice: Closing mineral cycles. European Commission, Directorate-General for the Environment. https://doi.org/10.2779/710012
- Serebrennikov, D., Thorne, F., Kallas, Z., & McCarthy, S. N. (2020). Factors influencing adoption of sustainable farming practices in Europe: A systemic review of empirical literature. Sustainability, 12(22), 9719. https://doi.org/10.3390/su12229719
- Simsar, A., Doğan, Y., & Sezer, G. (2021). The ecocentric and anthropocentric attitudes towards different environmental phenomena: A sample of Syrian refugee children. Studies in Educational Evaluation, 70, 101005. https://doi.org/10.1016/j.stueduc.2021.101005
- Singh, S. K., Patra, A., Chand, R., Jatav, H. S., Luo, Y., Rajput, V. D., & Adil, M. F. (2022). Surface seeding of wheat: A sustainable way towards climate resilience agriculture. Sustainability, 14(12), 7460. https://doi.org/10.3390/su14127460
- Svanbäck, A., McCrackin, M., Swaney, D., Linefur, H., Gustafsson, B., Howarth, R., & Humborg, C. (2019). Reducing agricultural nutrient surpluses in a large catchment – links to livestock density. Science of the Total Environment, 648, 1549–1559. https://doi.org/10.1016/j.scitotenv.2018.08.194
- Tadesse, M., & Belay, K. (2004). Factors influencing adoption of soil conservation measures in southern Ethiopia: The case of Gununo area. Journal of Agriculture and Rural Development in the Tropics and Subtropics (JARTS), 105(1), 49–62.
- Taghouti, I., Guesmi, B., Laabidi, S., & Youssef, S. B. (2021). Perspectives of adopting technological innovation in livestock-based production system. In Proceedings of the international conference of agricultural economists, international association of agricultural economics, online, 17–31 August 2021.
- Tey, Y. S., Li, E., Bruwer, J., Abdullah, A. M., Brindal, M., Radam, A., & Darham, S. (2014). The relative importance of factors influencing the adoption of sustainable agricultural practices: A factor approach for Malaysian vegetable farmers. Sustainability Science, 9(1), 17–29. https://doi.org/10.1007/s11625-013-0219-3
- Torres, M. A. O., Kallas, Z., & Herrera, S. I. O. (2020). Farmers’ environmental perceptions and preferences regarding climate change adaptation and mitigation actions; towards a sustainable agricultural system in México. Land Use Policy, 99, 105031. https://doi.org/10.1016/j.landusepol.2020.105031
- Vozmediano Sanz, L., & San Juan Guillén, C. (2005). Escala Nuevo Paradigma Ecológico: Propiedades psicométricas con una muestra española obtenida a través de Internet. Medio Ambiente y Comportamiento Humano, 6(1), 37–49.
- Wolf, S., Teitge, J., Mielke, J., Schütze, F., & Jaeger, C. (2021). The European green deal—more than climate neutrality. Intereconomics, 56(2), 99–107. https://doi.org/10.1007/s10272-021-0963-z
- Zhou, S., Herzfeld, T., Glauben, T., Zhang, Y., & Hu, B. (2008). Factors affecting Chinese farmers’ decisions to adopt a water-saving technology. Canadian Journal of Agricultural Economics/Revue canadienne d'agroeconomie, 56(1), 51–61. https://doi.org/10.1111/j.1744-7976.2007.00116.x