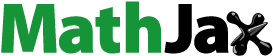
ABSTRACT
The aim of this paper is to investigate agricultural sustainability as a collective issue involving multiple rather than individual farms. Through the utilization of energy consumption as a proxy, we propose a novel methodology that evaluates the impact of farm consolidations on agricultural sustainability while accounting for resource preferences. Our approach incorporates the ordered weighted averaging (OWA) operator within an inverse data envelopment analysis (IDEA) model to identify post-merger farms that meet preset efficiency targets. We employ a DEA cross-efficiency (DEA-CE) procedure to select merger plans that maximize agricultural sustainability for each preference scenario. By analysing a case study of 43 tomato greenhouse farms in Biskra, northern Algeria, our findings demonstrate that mergers can significantly enhance agricultural sustainability, surpassing the potential of individual farms by a factor of over 15. Additionally, the adoption of the most sustainable merger plan can lead to energy savings of more than 69%. Irrespective of the preference scenario, substantial energy savings in machinery, fertilizers, diesel, and electricity ranging from 22.92% to 73.73% were observed. These results emphasize the strategic role of merger processes in promoting agricultural sustainability and optimizing resource utilization.
1. Introduction
The world population is projected to hit the cap of 8.5 billion in 2030 and grow further to 9.7 billion by 2050 (United Nations, Citation2022), which amplifies drastically the challenge of meeting the global demand for food (Abu Hatab et al., Citation2019). With the agricultural sector bearing such a burden, more resources are required for the operations performed on-farm and throughout the agricultural food supply chain, such as water, land, fuel, fertilizers, pesticides, machinery, etc. (Gorjian et al., Citation2021). The extensive use of these resources leads naturally to an increase of the environmental impact of agriculture, a major issue that has drawn an intense debate on sustainable agriculture (Lampridi et al., Citation2019). Though a consensus could hardly be reached regarding a common definition of the concept (Binder et al., Citation2010; de Olde et al., Citation2017), there is still an agreement among scholars that agricultural sustainability needs to assess environmental, economic, and social aspects associated with agricultural practices (Pham & Smith, Citation2014). Per se, a sustainable agriculture is expected to be economically viable, ecologically sound, socially just and humane (Zahm et al., Citation2019). A huge number of publications appeared on sustainable agriculture. Recently, Piñeiro et al. (Citation2020) reviewed nearly 18,000 publications only on incentive programmes for sustainable agricultural practices. On another side, Chopin et al. (Citation2021) presented a review of avenues for improving farming sustainability assessment in terms of tools, framing and indicators. The reader is referred to Lampridi et al. (Citation2019) for a systematic review of the literature relating to agricultural sustainability.
At the farms’ level, production of goods and/or services, management of resources, and influence on rural dynamics are generally regarded as, respectively, the economic, the environmental, and the social pillars of a sustainable agriculture (Latruffe et al., Citation2016). Nevertheless, it appears that most of the studies focus more on the environmental rather than on the economic and the social indicators of agricultural sustainability (Dakpo et al., Citation2016). This trend is notably due to the excessive consumption of natural resources, especially energy, which exacerbates the risk of greenhouse gas (GHG) emissions and hinders environmental sustainability (Liu et al., Citation2016). Thus, ensuring efficient agricultural energy consumption is an important step towards balancing the need for energy with responsible and sustainable energy management practices (Rasheed et al., Citation2022).
The evaluation of energy efficiency is a typical multi-criteria decision making (MCDM) problem, which often involves many agricultural production factors (Gésan-Guiziou et al., Citation2020). The stochastic frontier analysis (SFA) and data envelopment analysis (DEA) are the most commonly used techniques for agricultural efficiency assessment. The DEA approach (Charnes et al., Citation1978) is a non-parametric approach that is based on linear programming and can evaluate the efficiency of homogenous groups of decision making units (DMUs) defined with multiple inputs and multiple outputs (Oukil et al., Citation2016). As opposed to the SFA approach (Aigner et al., Citation1977), DEA does not require prior assumptions on the functional form of the production technology nor the probability distribution of the random error.
Recent applications of SFA to the evaluation of agricultural energy efficiency include the studies of Ali et al. (Citation2019), Khan et al. (Citation2021), Bibi et al. (Citation2021) and Khan et al. (Citation2023). The environmental efficiency of agricultural systems has also been investigated through SFA in Vu et al. (Citation2019), Khan and Ullah (Citation2020), Le et al. (Citation2019) and Tu et al. (Citation2019) but the investigations were carried out from the perspective of undesirable outputs rather than energy inputs.
Studies that apply DEA for the evaluation of agricultural energy efficiency are relatively more abundant. A recent survey reports that DEA applications to agricultural sustainability account for not less than 63% of the extant literature (Zhou et al., Citation2018), with a remarkable prevalence of the environmental aspects (Dakpo et al., Citation2016), where energy is the most critical indicator (Hercher-Pasteur et al., Citation2020). The majority of DEA-based studies that deal with agricultural energy efficiency are carried out for open field agriculture where different crops are cultivated, including sugarcane (Kaab et al., Citation2019), arecanut (Paramesh et al., Citation2018), cotton (Singh et al., Citation2022), rice (Najafabadi et al., Citation2022; Nayak et al., Citation2023), almond (Baran et al., Citation2020), chickpeas (Elhami et al., Citation2016), paddy (Mohammadi et al., Citation2015; Nabavi-Pelesaraei et al., Citation2017), wheat (Esfahani & Rafati, Citation2022; Ilahi et al., Citation2019; Pishgar-Komleh et al., Citation2020; Singh et al., Citation2019; Singh et al., Citation2021), peanuts (Hosseinzadeh-Bandbafha et al., Citation2018), grape (Mohseni et al., Citation2018), saffron (Saeidi et al., Citation2022), barley (Payandeh et al., Citation2021), rice-wheat-green gram (Bhunia et al., Citation2021), and maize (Mwambo et al., Citation2021). There are fewer studies in the greenhouse (GH) production, conducted mostly in arid regions, for tomato (Nourani & Bencheikh, Citation2020; Raheli et al., Citation2017), eggplant (Nourani & Bencheikh, Citation2021), button mushroom (Ebrahimi & Salehi, Citation2015), cucumber (Mardani Najafabadi & Taki, Citation2020; Soheilifard et al., Citation2021) and mix GH crops (Elhag & Boteva, Citation2019; Liang et al., Citation2019).
The reader is refereed to Kyrgiakos et al. (Citation2023) for a recent review of DEA applications to sustainability assessment in the agricultural sector.
Regardless of the DEA techniques that are adopted, all the aforementioned studies deal with the farms as independent production units, not sharing any resources with each other. More specifically, the farms’ relative efficiency is evaluated to capture performance gaps and identify the input factors that may necessitate improvement for each inefficient farm (Sow et al., Citation2016). The improvement potential is estimated through the slack values of these inputs. Though the slack schemes can lead to efficiency improvement of individual farms, recent studies have established that more savings are possible under Mergers & Acquisitions (M&A) schemes, where a group of farms agree to merge resources for the sake of enhancing collective production capabilities (Oukil, Citation2023b).
Farm mergers are business deals that involve the consolidation of two or more individual farms seeking various strategic, economic, or operational goals. The scale of these mergers can vary from small family farms to large commercial farming operations. A census of the Food and Agriculture Organization (FAO) across 30 countries reveals that there are approximately 500 million family farms, representing globally not less than 88% of all farms (FAO, Citation2014). Though most of these farms are small in size – operating under 2 hectares – and produce 80% of the world’s food (Graeub et al., Citation2016), they definitely face constraints related to scale, fragmentation and resource access (Agarwal et al., Citation2021). Therefore, the potential of farm mergers as an alternative model to small family farms needs to be investigated in terms of the economies of scale, which can result in more savings, an increased production efficiency and an enhanced approach to sustainability and environmental practices.
Farm mergers have generally been promoted within the framework of group farming (e.g. Agarwal, Citation2018; Agarwal et al., Citation2021), land consolidation (e.g. Xu et al., Citation2022; Zeng et al., Citation2018), and farmers’ cooperatives (e.g. Candemir et al., Citation2021; Grashuis & Su, Citation2019; Sarkar et al., Citation2022). Whether these studies are conducted in India (e.g. Agarwal, Citation2020; Sugden et al., Citation2021), Ireland (e.g. Cush & Macken-Walsh, Citation2016), France (Agarwal & Dorin, Citation2019), or the United Kingdom (Ingram & Kirwan, Citation2011), it is notable that the common purpose remains the evaluation of the impact of mergers decisions on productivity besides identifying the factors which influence potential changes (improvement/deterioration). In a study carried out in Kerala, India, Agarwal (Citation2018) concluded, through a regression model, that group farming outperforms individual farms in terms of annual output per hectare and net returns, with institutional design, state support, group heterogeneity, commercial crops as the major determinants of positive performance. Using Jiangsu province, China, as a case study, Zeng et al. (Citation2018) applied SFA to assess the effect of land consolidation on the agricultural technical efficiency. Sarkar et al. (Citation2022) adopted the theory of planned behaviour to evaluate the roles of the kiwi-fruit farmer's cooperative in Meixian, China, for fostering environmentally friendly production technologies. Hýblová (Citation2014) analysed agriculture mergers in the Czech Republic from a financial performance perspective and proved that, though the size of the merging entities in terms of balance sheet total decreased, the corresponding performance increased. Considering cow-calf farms, Holmström et al. (Citation2018) highlighted the positive impact of pastures’ expansion on Swedish suckler-based beef production due to lower operating costs brought on by economies of scale. Ho et al. (Citation2022) employed DEA to assess the influence of sustainable land management practices on the efficiency for orange cultivation in northwest of Vietnam and concluded that efficiency is strongly correlated with production scale. Llones et al. (Citation2022) applied SFA to show that shared irrigation management among farmers can improve significantly on-farm outcome for rice farms in Northern Thailand. Başer and Bozoğlu (Citation2023) developed a multi-stage approach to prove that a higher farm size has a positive effect on the sustainability of beef cattle farming in Samsun Province, Turkey. Ren et al. (Citation2019) reported similar conclusions. Lozano and Adenso-Díaz (Citation2021) proposed a DEA model to determine the most gainful merger within a set of dairy farms through estimating the total technical efficiency.
Apart from Lozano and Adenso-Díaz (Citation2021), no known study has addressed explicitly farm mergers through DEA. Furthermore, a recent systematic review of research on sustainability in M&A (González-Torres et al., Citation2020) reveals that DEA has not been used to investigate sustainability of merger processes neither in agriculture nor any other business sector. As such, to the best of the authors’ knowledge, the present study introduces for the first time the concept of DEA to the field of sustainability in M&A, regardless of the business field.
On another hand, it is noteworthy that the aim of Lozano & Adenso-Díaz’s study is restricted to estimating post-merger efficiency gains by employing the entire input levels of the merging farms. Yet, before sealing the merger deal, the farmers might be interested to know more about the optimal levels of inputs that each merging farm must contribute for the sake of achieving an efficiency target set a priori for the planned merger. In other words, the efficiency score of the planned merger becomes a known parameter of an optimization problem for which the decision variables are the input contributions of the merging farms. Such a statement reflects the essence of an inverse optimization problem and, within a DEA setting, Inverse DEA (IDEA) appears to be the perfect modelling tool (Pendharkar, Citation2002). Instead of pursuing the estimation of the efficiency for known inputs and outputs of a DMU, IDEA, contrary to the traditional DEA, searches for the optimal input and/or output levels that qualify a DMU to reach a preset efficiency target.
There are relatively fewer studies related to the application of IDEA to M&A. A recent review of the IDEA literature reveals a clear domination of the banking sector (Emrouznejad & Amin, Citation2023). Other sectors include energy (Lin et al., Citation2020), higher education (Amin & Oukil, Citation2019a), hospitality (Oukil et al., Citation2024) and agriculture (Oukil, Citation2023b; Oukil, Nourani, et al., Citation2022; Oukil et al., Citation2023), In the agricultural sector, Oukil (Citation2023b) is the first study that applied IDEA to investigate prospective gains from the merger of farms. This study’s findings reported proportions of potential resource gains ranging between 21% and 72%, on average, with the uppermost gains expected for water and electricity. Also, Oukil, Nourani, et al. (Citation2022) used IDEA to evaluate the impact of farm mergers on energy consumption. The results indicated that the average proportions of potential energy gains range between 20.68% and 78.33% per post-merger GH farm. In spite of succeeding to underline the ability of mergers to enhance resource gains in the agricultural sector, the common drawback of these two studies resides in the implicit assumption that the farm’s inputs (resources) are equally important; a feature that is clearly reflected through the adoption of an IDEA model (Gattoufi et al., Citation2014) whose objective coefficients are all equal. In real-life situations, there are resources that can be regarded as relatively more important than others. The importance scale depends primarily on the decision making settings, which may involve local or global policies, corporate strategies, market trends, or other technical factors that are specific to the farming process. For instance, water is commonly the most important resource for agricultural production but its importance is more emphasized in arid regions because of its scarcity (Al-Mezeini et al., Citation2020). Electricity, as a requirement for water pumping and distribution, is a key resource for irrigated agriculture, regardless of the agricultural context (Langarita et al., Citation2017). On the other hand, organic fertilizers are rather essential for a sandy soil, which requires frequent amendments with organic matter to improve its ability to hold onto nutrients (Yu et al., Citation2012). The role of human labour is strategic to the agricultural sector, but its importance is likely to be greater for territories with low involvement of the local populace, such as Sub-Sahara Africa (Ibidunni et al., Citation2020). Hence, treating all the resources in the same way is not always appropriate and may lead to biased decisions. Thus, another new aspect that the present study aims to investigate is the impact of resource prioritization on the agricultural sustainability of farm mergers. In light of the reviewed literature, it is the first time that such a topic is studied for either individual or group farming. Considering the preferences of different stakeholders towards the agricultural resources, we develop a new approach that exploits the properties of the Ordered Weighted Averaging (OWA) operator (Yager, Citation1988) to produce an importance weight for each resource. As a result, we propose an extended IDEA model that enables the decision maker’s (DM) preference to be duly integrated into the merger’s process.
As environmental sustainability is a collective issue that does require collective actions, the next stage of this study is also concerned with planning the best matches between pairs of farms, in view of maximizing the sustainability of the whole agricultural production sector. In other words, it is hypothetically assumed that the pairs of farms to be merged are not set a priori and the objective is to select collectively the best partners for each merger. To this end, we propose, for the first time, a DEA cross-efficiency (DEA-CE) methodology to determine the most sustainable merger plan for different DM’s preference schemes.
Consequently, the present paper contributes to the body of knowledge relating to sustainability over four fronts.
It is the first DEA-based study that investigates sector-wide sustainability from M&A perspective.
The IDEA approach is introduced for the first time as a potent tool for evaluating mergers’ sustainability.
A new IDEA model that incorporates the DM’s preference is proposed as a way to prioritize the farming resources over the merger decision process.
A new DEA-CE methodology is developed to determine the most sustainable pairs of farms and, hence, yield the most sustainable merger plan..
The proposed methodological framework is implemented on a case study of 43 tomato GH farms from Biskra, Algeria.
The next sections of the paper are set out as follows. In Section 2, the traditional approach of sustainability, based on standard DEA models, is described, followed by the new IDEA model developed for enhancing sustainability through mergers under DM’s preferences. Section 3 presents the case study. In Section 4, the results are thoroughly discussed for selected DM’s optimism levels. Section 5 is dedicated to a new DEA-CE methodology for building the most sustainable merger plan with various DM’s optimism levels. In Section 6, we close the study with concluding remarks, policy implications, some of the study’s limitations and potential venues for future research.
2. Methodology
2.1. Traditional sustainability evaluation
Let’s consider a sample of K farms, each farm is defined with energy inputs
and energy outputs
where
and
Assume that we are interested in evaluating the sustainability of
The sustainability of
depends predominantly on its performance in using the available energy inputs
to produce energy outputs
Within the production possibility set comprising the K farms,
s performance can be estimated through its relative efficiency score
computed via the following input-oriented BCC model (Banker et al., Citation1984).
represents the optimal efficiency score of farm
Thus,
is efficient if
otherwise, it is inefficient. In the case
is inefficient, it may need to reduce its energy inputs with an amount
(Oukil et al., Citation2021; Soltani et al., Citation2021) where
(1)
(1) Here,
is the energy saving that is required for input
If
and
for all
is strongly efficient. Contrariwise,
is weakly efficient if
for one energy input
or more (Oukil & Al-Zidi, Citation2018).
In the coming sections, we show that, even if is strongly efficient, its merger with another farm may lead to substantial energy savings. As such, we propose a methodology for enhancing sustainability through farm mergers. Although the proposed mathematical model deals with pairwise mergers, its extension to more than two farms remains possible. To enable discarding the impact on the results of possible fluctuations due to market disruptions or other exogenous factors, we also assume that the energy input consumption within the farms is deterministic over the merger planning horizon.
2.2. Enhancing sustainability through mergers
Let’s consider the merger of two farms and
into a larger farm
Regardless of the efficiency status of
and
the targeted efficiency of the post-merger farm
is set a priori to
Assuming that
retains the total energy outputs of
and
i.e.
the DM is concerned with finding the minimum levels of energy inputs
and
which are needed to operate
and achieve the target
If we also need to integrate the DM’s preference into the merger’s evaluation, the IDEA model of (Amin & Ibn Boamah, Citation2020) can be extended as follows:
The inverse property of E-IDEA stems from the fact that, contrary to traditional DEA optimization models, the targeted efficiency
is a known parameter of the problem whereas the energy inputs
and
are decision variables whose optimal values must be determined. Here,
is the weight of input
which is integrated into the original model to account for the relative importance of the energy inputs. In real-life problems, the DM may set a preference scale on the inputs, depending on the application context. A specific energy input
may be perceived more important based, e.g. on its market price, its scarcity, its indispensableness to the production process or other considerations pertaining to the decisional context.
The post-merger farm is evaluated relatively to the set of peers R, which may include either
or
or none. In a survival, the acquiring farm, i.e.
or
will carry on operating alone with the previous name. In a consolidation,
and
will unite into a new farm, like
Enhancing the farms’ sustainability being the main aim of our study, all the mergers will be consolidations, i.e. none of the merging farms will belong to R.
Definition 1:
A merger
is sustainable if
and
or
for one or more input
Definition 2:
A merger
is a major consolidation if and only if
and
for
and
As such, a major consolidation is not sustainable in the sense that it does not lead to energy gains.
Definition 3:
Given a sustainable post-merger
the potential energy gains of
and
for energy input
are
and
respectively.
Definition 4:
The cumulative energy gains of post-merger
for energy input
is
for a proportion
of the initial energy inputs.
It is important to note that, although model E-IDEA is developed for pairwise mergers, it can be easily extended to the merger of more than two farms.
3. Case study
The new approach is illustrated through a case study of 43 GH farms producing tomato in Biskra, north east of Algeria ().
3.1. Study area
Biskra serves as a transitional zone between the northern and the southern parts of the country. With predominantly flat terrain, apart from the surrounding Ziban mountains, the outskirts of Biskra feature small water sources, particularly within the oases and canyons surrounding El Kantara. Located approximately 430 km south-east of the capital city of Algiers, Biskra resides at an average elevation of 88 m above the sea level.
Characterized by a subtropical hot desert climate, Biskra experiences arid and exceedingly hot summers, coupled with very cold winters. During summer, temperatures average around 43.5°C, often peaking above 48°C, with a relative humidity of 12%. Winters bring an average minimum temperature of 4°C, a relative humidity of 89%, and an annual rainfall of 138 mm, occurring over fewer than 31 days each year.
Biskra, along with other regions in southern Algeria, has recently witnessed significant development in irrigated crops, primarily relying on groundwater extraction. While renowned as the leading producer of date palm fruits in Algeria, Biskra has arisen as a prominent hub for greenhouse (GH) production as well. Since its introduction to the region in 1984, GH technology has experienced rapid expansion, with the cultivated area growing from 1370 ha in 1999 to 4050 ha in 2013. Despite occupying no more than 4% of the region’s entire irrigated land, GH vegetable production in Biskra accounts for over half the country’s GH crop market. Among the various crops, tomato GH production holds significant importance, covering nearly 34% of the region’s area allocated to GH production.
3.2. Data collection
Primary data were collected in the course of the cropping season of 2017–2018 by conducting face-to-face interviews with 43 farmers. These farmers were nominated in a random manner from six municipalities known for their significant cultivation of tomatoes in greenhouses. The municipalities include M’ziraa, Ainnaga, Sidi-Okba, Elaghrous, Doucen, and Lioua (), where lycopersicon esculentum mill is the only type of tomatoes that is cultivated. As such, each GH farm is defined with six inputs, namely human labour machinery
fertilizers
pesticides
diesel fuel
and electricity
in addition to yield
as an output. presents the descriptive statistics of the original data sample, prior to its energy conversion.
Table 1. Descriptive statistics for the inputs and output.
Human labour refers to the total number of hours spent on field by the workers. The majority of workers are hired from neighbouring municipalities and include both genders, males and females. The workers who ensure the maintenance of the crop are generally employed on permanent job contracts, whereas the harvest and other casual agricultural tasks are allocated on a seasonal contact basis. These two categories are aggregated in the computation of the human labour time.
Farm machinery represents the time that is required for handling mechanized agricultural activities. In Biskra’s GH farming, mechanization is limited to tillage, commonly carried out with 45 horse power tractors for drawing iron ploughs. The remaining agricultural tasks are done manually.
Fertilizers comprise the amounts of nitrogen, phosphorus, and potassium as well as manure applied to the soil. In the study’s specific context, the application of the fertilizers is performed randomly without prior analysis of the soil or the crop requirements. Accordingly, the survey reveals an over-usage of fertilizers. Pesticides are used by most farmers to manage weeds, pests, and illnesses. Here, they are applied every year for preventive purposes rather than treatment, which may also explain the excessive usage amounts per hectare. Here, it is worth noting that nitrogen, phosphorus, potassium and manure are aggregated under the variable Fertilizers whereas fungicides and insecticides are combined into Pesticides
This inputs’ aggregation is primarily meant to reduce the number of variables involved in the DEA model and, hence, increase its discriminatory power.
In our study, the GH farms’ irrigation system relies fully on groundwater. As the major sources of agricultural energy, Electricity and Diesel fuel are extensively utilized to operate power generators, which supply electricity to water pumps for abstraction besides pressurization of the water into the irrigation system. Diesel fuel is typically used in areas without access to a power grid or as emergency backup during grid failures. Thus, electricity and diesel fuel are treated as proxies for water consumption. Water consumption has not been included separately among the inputs because all the farmers have reported the same regulated quota for the consumption amount. Having the same input value for all the GH farms will not have any impact on the DEA results.
summarizes the input and output values per hectare of land along the energy equivalents for the whole sample.
Table 2. Data summary with energy equivalents.
The energy equivalents are calculated using the corresponding coefficients shown in of the Appendices. presents the proportions of energy inputs associated to the tomato GH production sample. The energy proportions per input are displayed in for the whole study sample.
The total energy consumption per GH farm is 81.388 GJ.ha−1, with fertilizers and electricity displaying proportions of 41.21% and 30.14%, respectively, which are the highest. Pesticides follow with a share of 11.20%, while machinery appears as the least energy consuming resource.
4. Results and discussion
4.1. GH farming sustainability evaluation
For each farm
BCC model is solved to compute its optimal efficiency
its intensity vector
and the associated slack values
Though the average efficiency of 0.9653 may suggest that the GH tomato production is highly efficient, 14 of the 43 farms, or 32.56%, are inefficient
and display overconsumption of energy for all the inputs, as shown in .
Table 3. Results for the inefficient GH farms.
These results are comparable with those reported in previous studies, such as, e.g. Rahbari et al. (Citation2013) and Oukil, Nourani, et al. (Citation2022) where the mean efficiency scores are, respectively, 0.9955 and 0.9784 for tomato GH production. However, there appears to be more variability for the proportions of inefficient GH farms, which are 29.41% (Oukil, Nourani, et al., Citation2022) and 13.33% (Rahbari et al., Citation2013). Such inconsistency can be partially attributed to the differences between the size of the samples of GH farms in comparison with the corresponding number of inputs and outputs in these studies.
To become efficient, each inefficient farm must reduce its energy consumption by
with its energy output unaffected. As a result, the energy savings associated to the GH tomato production are presented in the following .
Table 4. Energy savings patterns per resource.
The slack analysis reveals a potential of 155.7 GJ ha−1 energy savings, where fertilizers have the highest proportion of 41.11%, more than electricity, whose share is 34.60%. Thus, the energy saving index is 4.45% for 3499.17 GJ ha−1 energy usage. In other words, the GH tomato production can be declared sustainable if the inefficient farms can save, individually, 4.45% of the total energy consumption. Although the latter index is more than double the value of 2.08% reported in Oukil, Nourani, et al. (Citation2022), it is much lower than the 24.46% of Khoshnevisan et al. (Citation2013).
Whatever the savings achievable at the GH farm individual level, the next section will show that group actions involving more than one GH farm may generate better results.
4.2. Computation of the importance weights
In order to derive a comprehensive judgment regarding the importance of the energy inputs, 15 agricultural experts, including researchers, academicians and professionals, have been presented with a list of 6 inputs and they have been requested to rank them from 1 to 6, where 1 and 6 represent, respectively, the ranks of the inputs that are the most and the least important for the production process The responses are displayed in .
Table 5. Ranking matrix of the production inputs.
For instance, we can see that Expert #8 judges that Human labour is the most important production input whereas Pesticides are the least important. Irrigation water being the next important input may suggest that Expert #8’s ranking is motivated by the scarcity of the resources rather than any other consideration. Such an approach is emphasized in the ranks assigned by Expert #13 who places Human labour, Irrigation water and Pesticides at the same importance level. Overall, it seems that a similar ranking strategy is adopted by most of the area experts, even if the ranking patterns could be different.
The preference voting matrix that is derived from the ranking matrix
is presented in .
Table 6. Preference voting matrix of the production inputs.
The columns of represent the rank positions
of the production inputs while each value
provides the number of times the experts voted for input
to be ranked
th. For instance, 10 out of 15 experts voted for Human labour to be 1st, which may qualify this input to be the most important under an optimistic stance but the least important under a pessimistic stance, though no such conclusion is possible before aggregating the votes over each row. In order to measure the impact of the inputs’ importance weights on the GH farms’ mergers, we consider three aggregation scenarios, depending on the attitude of the DM: optimistic (α = 0.73), neutral (α = 0.5) and pessimistic (α = 0.27). Hence, we solve the minimax disparity model (WP) presented in Appendix 3 for each optimism level α and I = 6, to produce the OWA weight vectors
shown in .
Table 7. OWA weight vectors .
The aggregate votes corresponding to each of these scenarios are exhibited in .
Table 8. Aggregate votes .
For instance, when α = 0.27, is computed as follows:
As expected,
, which is the weight of Human labour, is the smallest
with a pessimistic DM (α = 0.27) and the highest
with an optimistic DM (α = 0.73).
4.3. Mergers of GH farms
With a sample of G = 43 GH farms, 903 pairwise mergers
are possible. The solution of model (E-IDEA) for each potential merger
with
efficiency target, produces the optimal input blends for each preference scenario. Out of 903 potential mergers, only 742 are found potentially sustainable, i.e. 82.17%. This proportion is the same for all preference scenarios and the corresponding pairs of merging farms are also the same. These results suggest that the importance weights of the energy inputs have no effect on the sustainability status of the mergers. It is also worth noting that 345 sustainable post-merger GH farms, i.e. 46.5%, are hybrid pairs that comprise one efficient along with one inefficient GH farms, while only 75 sustainable mergers, i.e. 10.1%, include solely inefficient GH farms. Nevertheless, the most notable result is indeed the presence of 312 sustainable mergers, i.e. 43.4%, which are pairs of only efficient GH farms. Therefore, regardless of its efficiency status from an individual assessment perspective, a GH farm has potential for energy savings under a merger.
Due to the large number of sustainable mergers and, in the interest of space, shows only 10 sustainable mergers under the optimistic stance. In the meantime, the results are discussed for all sustainable mergers under the three preference scenarios.
Table 9. Optimal energy usages (MJ ha−1) of 10 post-merger GH farms with.
Consider, for instance, the consolidation of and
into
With an efficiency target
the optimal energy levels of labour, machinery, fertilizers, pesticides, diesel and electricity required for the post-merger
are
and
The fact that
and
suggests that the collective contributions to the merger
in terms of labour, diesel and electricity, respectively, are at the level of the energy inputs of
or less.
The energy inputs of and
at the pre-merger stage are
and
Thus, the potential energy gains for fertilizers, due to the merger
include a portion of the pre-merger energy usage of
that is,
in addition to the entire energy input of
which is
Hence, the cumulative energy gains derived for fertilizers are
which accounts for not less than 43.77% of the collective energy inputs allotted individually to the GH farms
and
Likewise, the gains for labour, diesel, electricity, machinery and pesticides are, respectively, 56.19%, 16.76%, 85.34%, 43.10%, and 45.30%. It is also important to note that
and
are both strongly efficient, i.e.
and
and no energy savings are needed from neither GH farms while operating individually. Yet, the merger of these farms into
leads to energy savings at the proportion levels given earlier. presents the results for 10 post-merger GH farms
Table 10. Cumulative energy gains (MJ.ha−1) of 10 post-merger GH farms with related proportions.
The full picture about the extent of the energy gains is illustrated through the average proportions shown in , which include the whole set of sustainable post-merger GH farms ,
The average proportions of energy gains are almost the same for machinery, fertilizers, diesel and electricity, nearing 60.92%, 70.32%, 22.92% and 73.73%, respectively, regardless of the preference stance. Under the optimistic and the neutral scenarios, labour and pesticides have still close proportions, with averages of 66.89% and 53.77%, respectively, slightly different from the pessimistic stance, which is lower for labour (47.92%) and higher for pesticides (71.04%). Such a gap can be partly explained by looking closer at the patterns of energy gains. Indeed, almost 97% sustainable post-merger GH farms of the neutral case show results that are similar to those of the optimistic case. This fraction falls to 57% when the latter results are compared to those of the pessimistic stance. In the meantime, the proportions of energy gains can reach, for some sustainable post-merger GH farms, picks as high as 81.28%, 88.62%, 95.27%, 98.09%, 85.45% and 96.55%, respectively, for labour, machinery, fertilizers, pesticides, diesel and electricity. In other words, there are post-merger GH farms that can be operated with only 18.72%, 11.38%, 4.73%, 1.91%, 14.55% and 3.45% of their current usages of respective energy inputs. The latter proportions are consistent with the findings of Oukil, Nourani, et al. (Citation2022) where the minimum operating requirements for the post-merger GH farms were 19.22%, 7.76%, 2.73%, 2.71%, 10.66%, and 3.28% for labour, machinery, fertilizers, pesticides, diesel and electricity, respectively. Here, the slight gaps between the results can be attributed to the impact of the importance weights assigned to the inputs in the E-IDEA model as well as the size of the GH farms’ sample.
To perceive more tangibly the impact of a merger on sustainability, let’s consider, for instance, the consolidation of and
into
Under a standard DEA framework,
and
are found strongly efficient, that is, none of them needs to reduce its current energy consumption. After merging, the derived post-merger GH farm
could preserve the efficiency level
by using only fractions of the collective energy inputs of the original GH farms, as small as 39.75% for labour, 61.09% for machinery, 17.29% for fertilizers, 29.59% for pesticides, 38.74% for diesel and 47.96% for electricity.
These proportions, together with the averages, are enough evidence to support the potential of mergers in enhancing sustainability, notwithstanding the optimistic case. To better corroborate such a statement, it is important to evaluate the impact of a broader scale merger on the sustainability of the whole tomato GH farming sector. To this end, we develop a procedure for building the most sustainable merger plan for each DM’s preference stances, as detailed in the next section.
5. Most sustainable merger plan
To develop the most sustainable merger plan, we develop an approach that enables selecting the best post-merger farms based on the potential energy gains associated to the inputs of the alternative pairs
The energy gains satisfy the dictum ‘more is better’ and qualify to be viewed as an output of the merger process (Oukil & El-Bouri, Citation2021). Hence, considering each pair
as a DMU defined with the outputs
DEA-CE (Sexton et al., Citation1986) is adopted to rank the pairs
prior to selecting the most sustainable merger plan.
5.1. Computing the matrix of CE scores
Here, we introduce the following Sustainable Merger Model (SMM):
where
is the multipliers associated to the
th output and
is the measure of returns to scale. As a self-efficiency model, SMM can only categorize the post-merger farms into efficient
and inefficient
and, hence, cannot fully rank the whole set of farms. For this reason, we resort to DEA-CE.
Under a CE methodological framework, each post-merger farm is enabled to evaluate its peers with its optimal multipliers
(Oral et al., Citation2014; Oukil & Govindaluri, Citation2020). However, as SMM is likely to have alternative optima, different scores may result for the same CE evaluation and, hence, the uniqueness of the ranking is not guaranteed. One way to alleviate such a drawback is the adoption of a secondary goal for the CE evaluation (Doyle & Green, Citation1994). Though many secondary goal models are proposed in the DEA literature, we extend the Most Resonated Appreciative (MRA) model (Oral et al., Citation2015; Oukil & Amin, Citation2015) as follows.
MRA is the only model that generates a customized vector of multipliers for each CE evaluation, which enhances discrimination at an early stage of the ranking process (Amin & Oukil, Citation2019b; Oukil & Govindaluri, Citation2017). Here,
is the CE score of post-merger farm
as assigned by the assessing farm
using the multipliers
that preserve
’s self-efficiency score at the level
(Oukil, Soltani, et al., Citation2022; Oukil & Amin, Citation2023). Once each post-merger farm
completes the evaluation of its 741 peers
a separate matrix of CE scores
is obtained for each optimistic stance, with the self-efficiency scores forming the diagonal of
Matrix
cannot be presented here due to its size (742 × 742). Next,
is used to compute the ultimate efficiency scores, which are required for ranking the 742 sustainable farms, as explained in the next section.
5.2. Ranking the sustainable post-merger farms
Using the matrix of CE scores as a ground, we present the successive steps for computing the ultimate efficiency score
corresponding to each post-merger farm
Let denote the column of CE scores assigned to
in
The computation of
entails aggregating the elements of
(Oukil, Citation2018, Citation2019, Citation2020, Citation2022, Citation2023a). In order to take the preference scenario into account, we employ again OWA aggregation, as explained below.
Step 1: Sort the CE scores
The CE scores of each column are sorted in ascending order to enable attaching more importance to smaller scores assigned to post-merger farm
by its peers
The smaller
the more important is farm
perceived by farm
Let
be the vector of sorted CE scores for column, where
Step 2: Generate the vector of OWA weights
Model (WP) is used to generate the aggregation weights ,
Three different optimism levels are considered for each preference scenario, namely
and
depending on whether matrix
corresponds to the optimistic, neutral and pessimistic stance.
The solution of WP is a vector of 742 weights
with decreasing values for
and increasing values for
For all the weights
are equal.
Step 3: Compute the ultimate efficiency score
With the OWA weights on hand, the ultimate efficiency score can be computed for post-merger farm
through aggregating the CE scores of vector
by using formula (3) as
Once steps 1 to 3 are completed for each post-merger farm
these farms are ranked from the most to the least sustainable. presents an excerpt of the 10 leading farms under each optimistic stance.
Table 11. Ranking patterns of the 10 leading post-merger GH farms.
To evaluate the effect of the DM’s preference on the variability of the ultimate efficiency scores, we run a two-tail Student test at 5% significance level with paired samples. Each sample consists of 742 -values of same post-merger farms under different optimistic stances. Thus, three tests are run separately, with the outcomes exhibited in .
Table 12. Tests of the effect of preference on the variability of the ultimate efficiency scores.
Based on the p-values, there is not enough evidence to reject the hypothesis that the difference between the means of the ultimate efficiency scores’ populations is significant. Hence, at 5% significance level, we can assert that the DM’s preference has an impact on the variability of the ultimate efficiency scores.
Would the same conclusion stand with the ranking patterns?
The smaller the ultimate efficiency score the better the ranking of the efficient post-merger farm
Hence, as shown in ,
is the leading post-merger farm for the pessimistic as well as the neutral samples whereas the optimistic sample is led by
Again, we may need to investigate whether the DM’s preference affects the variability of the ranking patterns. Here, we perform a two-tailed Wilcoxon signed-ranks test for paired samples at 5% significance level to test the following null hypothesis:
H0: The ranking patterns produced under both stances are identical.
The tests’ results are summarized in , where T = min(T+, T–) with T+ and T– representing, respectively, the rank sums associated to positive and negative differences.
Table 13. Tests of the effect of preference on the variability of ranking patterns.
At 5% significance level, we can conclude that the ranking patterns produced under the neutral and the pessimistic stances are not identical. The same conclusion stands for the neutral and the optimistic stances. However, p-value > 0.05 suggests that the ranking patterns of the optimistic and the pessimistic stances are identical. Although the latter conclusion applies only to the case study, it is apparently reliable enough to refute the hypothesis that the DM’s preference may affect the mergers’ ranking.
5.3. Building the most sustainable merger plan
To build the most sustainable merger plan, the best mergers are selected according to the rank orders while discarding subsequent pairs that overlap with previously selected pairs. As such, the pairs of farms, presented in , are selected as the potential most sustainable mergers for each preference stance.
Table 14. Most sustainable merger plans.
Overall, the most sustainable merger plans developed for the three preference stances are different although exhibiting similarities for some of the selected post-merger farms.
In the pessimistic plan, has not been selected in spite of ranking 2nd because
has
in common with
The same applies to the neutral plan where six pairs are discarded, namely
and
which share either
or
with the leading post-merger farm
. Thus, the exclusion of better ranked post-merger farms is essentially based on the occurrence of an overlap between these pairs and the ones that are previously selected. Such exclusion criterion applies also to
and
which occupy the next five positions of the optimistic ranking pattern but share, at least, one GH farm with
Therefore, the next pair to be selected is
because it does not overlap with
Under the pessimistic stance, all the GH farms are paired except
and
Two additional exceptions, i.e.
and
are found for both the neutral and the optimistic cases. Practically, pairwise mergers are still possible among the excluded GH farms but remain not sustainable. Remarkably, almost 50% of the selected pairs consist solely of GH farms that are strongly sustainable, no matters the preference case. Such a result brings more support to the potential of mergers in enhancing sustainability, even if the merging entities are individually efficient.
5.4. Managerial implications
At this stage of the analysis, it is important to evaluate the impact of the prospective merger plans on environmental sustainability. summarizes the total energy savings for each merger plan.
Table 15. Energy savings for sustainable merger plans.
The results reveal that the most sustainable merger plan is more likely under the pessimistic stance, where the largest energy savings are 2440.26 GJ ha−1. The neutral merger plan is probably the least sustainable with almost 15 GJ ha−1 less savings. Thus, with the current energy usage estimated to 3499.17 GJ ha−1, the energy savings indices are 69.74%, 69.33% and 69.45% for the pessimistic, neutral and optimistic sustainable merger plans, respectively. Hence, there is potential for more than 15 times improvement of the GH production sustainability. Specifically, displays the proportions of savings for each preference scenario.
Regardless of the sustainable merger plan, the energy savings on each input are close to each other and follow almost a similar pattern. Fertilizers and electricity are prevailing, with proportions that exceed 40.5% and 33.8%, respectively. The lowest proportions are found for machinery, whose shares are less than 1.9%. Relatively higher proportions are expected for labour and diesel at levels of more than 5.8% and 4.7%, respectively. The proportions of energy savings on pesticides fall between 12.1% and 12.7%. Interestingly, these sustainability patterns comply with the current distribution of energy usage (refer to ), which suggests that the proposed merger plans can be an important step towards an optimal energy consumption and, hence, more sustainable agricultural production.
In practical terms, chemical fertilizers energy savings can be achieved by adopting more precise fertilization practices. Conducting seasonal soil analyses can assist in determining the most adequate quantities on a weather basis. However, replacing chemical fertilizers with organic or microbial alternatives is certainly the preferable choice. The Integrated Pest Management (IPM) method can be adopted as a long-term pest management approach.
Diesel fuel is typically used to power electric generators in areas without access to a power grid or as emergency backup during grid failures. To minimize reliance on diesel and electricity, the adoption of renewable energy sources is recommended. Given the region's high solar irradiation all over the year, solar panels can possibly be installed to provide a cleaner and more sustainable energy alternative. Alternatively, the energy needed for irrigation can be reduced by using low-flow resistance foot valves for pumps besides rigid PVC pipes for the suction and delivery operations. More energy savings are also possible through an appropriate substitution of undersized pipes in addition to employing less elbows and friction-causing fittings.
Implementing conservation tillage practices significantly decreases the need for machinery throughout various agricultural tasks. These practices also improve soil structure through the retention of vegetative matter, facilitating carbon storage in the soil.
However, it is crucial not to overlook the human aspect of the entire process. GH farmers should receive training on implementing new practices and be educated about the importance of energy mitigation as a global imperative for sustainable farming.
6. Concluding remarks
The present study investigated the impact of mergers among farms on environmental sustainability from different preference perspectives, with a focus on energy consumption. Based on experts’ judgments, a preference vector, produced through an OWA-based approach, is incorporated into an IDEA model to estimate the energy input requirements for a preset sustainability target under three preference stances: pessimistic, neutral and optimistic.
Using a case study of 43 GH farms for an efficiency target the proposed IDEA model is solved for pairwise consolidations under each preference scenario. The results revealed that 742 post-merger farms are potentially sustainable, where 43.4% are pairs involving only sustainable GH farms, i.e. GH farms that have been declared individually efficient and, hence, do not require energy input savings.
Thus, we can establish that neither the sustainability status of the merging DMUs nor the preference of the DM have an influence on the sustainability status of the post-merger DMUs.
In terms of energy inputs, the results of the case study show average proportions of gains that are almost the same with the three preference scenarios for machinery, fertilizers, diesel and electricity, reaching 60.92%, 70.32%, 22.92% and 73.73%, respectively. A slight deviation is noted, under the pessimistic stance, for energy gains on labour and pesticides, which still remain relatively high. In all cases, the energy gains per post-merger farm can reach proportions as high as 80.78%, 88.62%, 95.27%, 97.29%, 85.45% and 96.55%, respectively, for labour, machinery, fertilizers, pesticides, diesel and electricity. These proportions, together with the corresponding averages, substantiate the potential of mergers to enhance sustainability, regardless of the preference stance.
The next stage of the present study is concerned with maximizing the collective sustainability effort through determining the most sustainable merger plans under different preference stances. A DEA-CE model is developed to select the sets of post-mergers with the best matching pairs of GH farms under each preference stance.
The most sustainable merger plans that are built under the three preference stances include different post-merger farms even if similarities can be found. Almost half of the selected pairs entail individual GH farms that are strongly efficient. This result brings more support to the potential of mergers in enhancing sustainability, no matters the efficiency status of the merging farms.
The majority of individual GH farms are paired, except a few. The excluded ones can still form mergers, which are de facto not sustainable.
The total energy savings of the sustainable merger plans exceed 69% for all preference scenarios, representing more than 15 times relating outcomes under the individual GH farm sustainability framework. The distribution of these energy savings among inputs follows almost the same pattern. Fertilizers and electricity prevail with proportions of over 40.5% and 33.8%, respectively, followed by 12.1% to 12.7% for pesticides. Relatively lower proportions are observed for labour and diesel, with more than 5.8% and 4.7%, respectively, whereas machinery records the lowest share of less than 1.9%. Interestingly, the sustainability patterns conform to the energy usage distribution. For that reason, the proposed merger plans can be an important platform for an optimal energy consumption and, hence, more sustainable agricultural production.
6.1. Policy implications
This study's findings underscore the imperative for the development of progressive policies to reinforce sustainability within the GH farming sector. In practical terms, such a step is particularly vital to ensure a meaningful engagement of diverse stakeholders throughout the GH agricultural production supply chain.
One key policy recommendation revolves around incentivizing GH farmers to adopt energy-efficient practices within the context of potential mergers. These incentives might encompass state-level direct and indirect subsidies, tax exemptions besides relaxation of import duties. The GH farmers could be eligible for these incentives as they embrace energy-saving initiatives, contributing to reduced GHG emissions and optimal resource consumption. These initiatives may involve (1) the procurement of biodegradable plastic films and versatile construction materials for infrastructure development; (2) the transition from chemical to environmentally friendly organic or microbial fertilizers; (3) the adoption of integrated pest management (IPM) strategies for sustainable pest control; (4) the installation of solar panels to harness cleaner, renewable energy sources as an alternative to fossil fuels; and (5) the incorporation of cutting-edge technologies and techniques for fertilization and irrigation, including the implementation of smart irrigation systems (Zaier et al., Citation2015).
Recognizing the central role of the human factor in merger dynamics entails an additional set of policies, which should be primarily dedicated to raising awareness among GH farmers regarding the benefits of mergers. These policies may consist of the organization of comprehensive awareness campaigns aimed at promoting mergers and highlighting their potential to optimize energy usage and mitigate environmental impacts. Furthermore, state-sponsored training sessions could be developed to provide GH farmers with the knowledge and skills required for collaborative management in post-merger GH farms. Additionally, an incentivizing framework could be established, featuring an annual award to commend and incentivize the best-performing post-merger GH farms.
Though, it is paramount to underscore that prior to the adoption of merger-related policies, extensive investigations are necessary to gauge GH farmers’ readiness to participate in merger initiatives and identify market-driven incentives that are likely to spur their willingness to join such plans. Conducting these investigations will provide crucial insights for crafting policies that effectively address the unique needs and challenges of GH farmers while advancing sustainability within the sector.
6.2. Future research directions
While this study has made substantial progress in highlighting the benefits of GH farm mergers, it has also brought to light several key research areas that warrant further investigation. These future research directions would build upon the foundation laid by this study and expand our understanding of the complex dynamics surrounding GH farm mergers.
Consider the geographical locations of the farms
The geographical distribution of GH farms is a crucial aspect that has been inadvertently overlooked in the present study but holds significant relevance for the prospects of mergers. Current assumptions suggest that mergers are feasible irrespective of the farms’ physical locations, leading to the representation of pairwise consolidations as a complete graph with edges for G farms (Oukil, Citation2008). However, this approach raises practical challenges, particularly for large values of G, due to the computational cost associated with solving N IDEA models. Furthermore, proximity issues among merging GH farms may render the field implementation of optimal energy-saving solutions impracticable. Future research should consider addressing these limitations by adopting a more realistic approach that accounts for the sparse graph structure depicted through the GH farms’ network. In this context, only adjacent farms would be considered as merger candidates, potentially leading to geographically advantageous and more feasible merger plans.
Conduct a profitability analysis of the mergers
The implementation of energy-efficient measures within post-merger GH farms incurs additional costs, necessitating a deeper exploration of pertaining economic aspects. Such analysis can be critical in supporting merger decisions and providing more accurate estimates of each partner's share of the incremental expenses. To achieve this objective, researchers can envisage an energy-cost efficiency analysis that incorporates cost-weighted energy inputs. This approach will produce a robust framework for quantifying the dollar value of the merger per unit of energy input, offering stakeholders a more comprehensive financial perspective (Amin & Ibn Boamah, Citation2020).
Assess the long-term impact of the mergers
The present study has been carried out over a single cropping season, which may restrict the temporal scope of its findings. Understanding the long-term implications of GH farm mergers is essential for assessing their sustainability and adaptability. Future studies should investigate the impact of mergers over extended time horizons, encompassing multiple periods to account for the dynamic nature of the system (Moghaddas et al., Citation2022). This multi-period analysis will provide valuable insights into the evolving dynamics of merged GH farms, aiding in the development of strategies for long-term success.
Extend the sample size and the methodology
It is known that the discriminatory power of the standard DEA approach is attenuated when the number of inputs and outputs is too large, compared to the number of DMUs (GH farms in our case). As such, the number of inputs considered for the present study has been reduced through aggregation for the sake of increasing discrimination. Future studies may provide better insights if the inputs are used without aggregation but with larger samples of GH farms. On the methodology side, the IDEA model can be extended to include GHG emissions as undesirable inputs and/or outputs. Another research direction may consider the integration of the mergers’ gain estimation and the building of the most sustainable merger plan into a unified framework.
Expand the application scope
Although this study’s practical context was focused on agricultural GH production, its methodological framework holds potential for applications in other agricultural sectors where energy consumption is a critical concern, such as livestock production systems (Sefeedpari et al., Citation2020) and dairy farms (Nacer et al., Citation2016). Prospective research could also explore the implementation of the proposed approach across non-agricultural sectors to better identify the energy efficiency challenges of mergers.
IJAS_Reply_to_reviewers.doc
Download MS Word (83 KB)Disclosure statement
No potential conflict of interest was reported by the author(s).
References
- Abu Hatab, A., Cavinato, M. E. R., Lindemer, A., & Lagerkvist, C. J. (2019). Urban sprawl, food security and agricultural systems in developing countries: A systematic review of the literature. Cities, 94, 129–142. https://doi.org/10.1016/j.cities.2019.06.001
- Agarwal, B. (2018). Can group farms outperform individual family farms? Empirical insights from India. World Development, 108, 57–73. https://doi.org/10.1016/j.worlddev.2018.03.010
- Agarwal, B. (2020). A tale of two experiments: Institutional innovations in women’s group farming in India. Canadian Journal of Development Studies/Revue canadienne d'études du développement, 41(2), 169–192. https://doi.org/10.1080/02255189.2020.1779673
- Agarwal, B., Dobay, K. M., & Sabates-Wheeler, R. (2021). Revisiting group farming in a post-socialist economy: The case of Romania. Journal of Rural Studies, 81, 148–158. https://doi.org/10.1016/j.jrurstud.2020.10.006
- Agarwal, B., & Dorin, B. (2019). Group farming in France: Why do some regions have more cooperative ventures than others? Environment and Planning A: Economy and Space, 51(3), 781–804. https://doi.org/10.1177/0308518X18802311
- Aigner, D., Lovell, C. K., & Schmidt, P. (1977). Formulation and estimation of stochastic frontier production function models. Journal of Econometrics, 6(1), 21–37. https://doi.org/10.1016/0304-4076(77)90052-5
- Ali, Q., Yaseen, M. R., & Khan, M. T. I. (2019). Energy budgeting and greenhouse gas emission in cucumber under tunnel farming in Punjab, Pakistan. Scientia Horticulturae, 250, 168–173. https://doi.org/10.1016/j.scienta.2019.02.045
- Al-Mezeini, N. K., Oukil, A., & Al-Ismaili, A. M. (2020). Investigating the efficiency of greenhouse production in Oman: A two-stage approach based on data envelopment analysis and double bootstrapping. Journal of Cleaner Production, 247, Article 119160. https://doi.org/10.1016/j.jclepro.2019.119160
- Amin, G. R., & Ibn Boamah, M. (2020). A new inverse DEA cost efficiency model for estimating potential merger gains: A case of Canadian banks. Annals of Operations Research, 295(1), 21–36. https://doi.org/10.1007/s10479-020-03667-9
- Amin, G. R., & Oukil, A. (2019a). Flexible target setting in mergers using inverse data envelopment analysis. International Journal of Operational Research, 35(3), 301–317. https://doi.org/10.1504/ijor.2019.101196
- Amin, G. R., & Oukil, A. (2019b). Gangless cross-evaluation in DEA: An application to stock selection. RAIRO – Operations Research, 53(2), 645–655. https://doi.org/10.1051/ro/2018060
- Banker, R. D., Charnes, A., & Cooper, W. W. (1984). Some models for estimating technical and scale inefficiencies in data envelopment analysis. Management Science, 30(9), 1078–1092. https://doi.org/10.1287/mnsc.30.9.1078
- Baran, M. F., Eren, O., Gökdoğan, O., & Oğuz, H. I. (2020). Determination of energy efficiency and greenhouse gas (GHG) emissions in organic almond production in Turkey. Erwerbs-Obstbau, 62(3), 341. https://doi.org/10.1007/s10341-020-00507-9
- Başer, U., & Bozoğlu, M. (2023). The impact of farm size on sustainability of beef cattle farms: A case study of the Samsun province, Turkey. International Journal of Agricultural Sustainability, 21(1), Article 2253647. https://doi.org/10.1080/14735903.2023.2253647
- Bhunia, S., Karmakar, S., Bhattacharjee, S., Roy, K., Kanthal, S., Pramanick, M., & Mandal, B. (2021). Optimization of energy consumption using data envelopment analysis (DEA) in rice-wheat-green gram cropping system under conservation tillage practices. Energy, 236, Article 121499. https://doi.org/10.1016/j.energy.2021.121499
- Bibi, Z., Khan, D., & Haq, I. U. (2021). Technical and environmental efficiency of agriculture sector in South Asia: A stochastic frontier analysis approach. Environment, Development and Sustainability, 23(6), 9260–9279. https://doi.org/10.1007/s10668-020-01023-2
- Binder, C. R., Feola, G., & Steinberger, J. K. (2010). Considering the normative, systemic and procedural dimensions in indicator-based sustainability assessments in agriculture. Environmental Impact Assessment Review, 30(2), 71–81. https://doi.org/10.1016/j.eiar.2009.06.002
- Bojacá, C. R., Casilimas, H. A., Gil, R., & Schrevens, E. (2012). Extending the input–output energy balance methodology in agriculture through cluster analysis. Energy, 47(1), 465–470. https://doi.org/10.1016/j.energy.2012.09.051
- Candemir, A., Duvaleix, S., & Latruffe, L. (2021). Agricultural cooperatives and farm sustainability – a literature review. Journal of Economic Surveys, 35(4), 1118–1144. https://doi.org/10.1111/joes.12417
- Charnes, A., Cooper, W. W., & Rhodes, E. (1978). Measuring the efficiency of decision making units. European Journal of Operational Research, 2(6), 429–444. https://doi.org/10.1016/0377-2217(78)90138-8
- Chopin, P., Mubaya, C. P., Descheemaeker, K., Öborn, I., & Bergkvist, G. (2021). Avenues for improving farming sustainability assessment with upgraded tools, sustainability framing and indicators. A review. Agronomy for Sustainable Development, 41(2), 1–20. https://doi.org/10.1007/s13593-021-00674-3
- Cush, P., & Macken-Walsh, A. (2016). Farming ‘through the ages’: Joint farming ventures in Ireland. Rural Society, 25(2), 104–116. https://doi.org/10.1080/10371656.2016.1225833
- Dakpo, K. H., Jeanneaux, P., & Latruffe, L. (2016). Modelling pollution-generating technologies in performance benchmarking: Recent developments, limits and future prospects in the nonparametric framework. European Journal of Operational Research, 250(2), 347–359. https://doi.org/10.1016/j.ejor.2015.07.024
- de Olde, E. M., Moller, H., Marchand, F., McDowell, R. W., MacLeod C, J., Sautier, M., Halloy, S., Barber, A., Benge, J., Bockstaller, C., Bokkers, E. A. M., de Boer, I. J. M., Legun, K. A., Le Quellec, I., Merfield, C., Oudshoorn, F. W., Reid, J., Schader, C., Szymanski, E., & Manhire, J. (2017). When experts disagree: The need to rethink indicator selection for assessing sustainability of agriculture. Environment, Development and Sustainability, 19(4), 1327–1342. https://doi.org/10.1007/s10668-016-9803-x
- Doyle, J., & Green, R. (1994). Efficiency and cross-efficiency in DEA: Derivations, meanings and uses. Journal of the Operational Research Society, 45(5), 567–578. https://doi.org/10.1057/jors.1994.84
- Ebrahimi, R., & Salehi, M. (2015). Investigation of CO2 emission reduction and improving energy use efficiency of button mushroom production using data envelopment analysis. Journal of Cleaner Production, 103, 112–119. https://doi.org/10.1016/j.jclepro.2014.02.032
- Elhag, M., & Boteva, S. (2019). Conceptual assessment of energy input-output analysis and data envelopment analysis of greenhouse crops in Crete Island, Greece. Environmental Science and Pollution Research, 26(35), 35377–35386. https://doi.org/10.1007/s11356-019-05544-w
- Elhami, B., Akram, A., & Khanali, M. (2016). Optimization of energy consumption and environmental impacts of chickpea production using data envelopment analysis (DEA) and multi objective genetic algorithm (MOGA) approaches. Information Processing in Agriculture, 3(3), 190–205. https://doi.org/10.1016/j.inpa.2016.07.002
- Emrouznejad, A., & Amin, G. R. (2023). Advances in inverse data envelopment analysis: Empowering performance assessment. IMA Journal of Management Mathematics, 34(3), 415–419. https://doi.org/10.1093/imaman/dpad004
- Esfahani, S. M. J., & Rafati, M. (2022). The share of farm-scale on optimizing energy consumption and greenhouse gas emissions in irrigated wheat farms in eastern Iran. Sustainable Energy Technologies and Assessments, 53, Article 102465. https://doi.org/10.1016/j.seta.2022.102465
- FAO. (2014). The state of food and agriculture report, innovation in family farming. United Nations Food and Agricultural Organization (FAO). ISBN 978-92-5-108536-3.
- Gattoufi, S., Amin, G. R., & Emrouznejad, A. (2014). A new inverse DEA method for merging banks. IMA Journal of Management Mathematics, 25(1), 73–87. https://doi.org/10.1093/imaman/dps027
- Gésan-Guiziou, G., Alaphilippe, A., Aubin, J., Bockstaller, C., Boutrou, R., Buche, P., & van der Werf, H. M. (2020). Diversity and potentiality of multi-criteria decision analysis methods for agri-food research. Agronomy for Sustainable Development, 40(6), 1–11. https://doi.org/10.1007/s13593-020-00650-3
- González-Torres, T., Rodríguez-Sánchez, J.-L., Pelechano-Barahona, E., & García-Muiña, F. E. (2020). A systematic review of research on sustainability in mergers and acquisitions. Sustainability, 12(2), 513. https://doi.org/10.3390/su12020513
- Gorjian, S., Ebadi, H., Trommsdorff, M., Sharon, H., Demant, M., & Schindele, S. (2021). The advent of modern solar-powered electric agricultural machinery: A solution for sustainable farm operations. Journal of Cleaner Production, 292, Article 126030. https://doi.org/10.1016/j.jclepro.2021.126030
- Graeub, B. E., Chappell, M. J., Wittman, H., Ledermann, S., Kerr, R. B., & Gemmill-Herren, B. (2016). The state of family farms in the world. World Development, 87, 1–15. https://doi.org/10.1016/j.worlddev.2015.05.012
- Grashuis, J., & Su, Y. (2019). A review of the empirical literature on farmer cooperatives: Performance, ownership and governance, finance, and member attitude. Annals of Public and Cooperative Economics, 90(1), 77–102. https://doi.org/10.1111/apce.12205
- Hamedani, S. R., Shabani, Z., & Rafiee, S. (2011). Energy inputs and crop yield relationship in potato production in Hamadan province of Iran. Energy, 36(5), 2367–2371. https://doi.org/10.1016/j.energy.2011.01.013
- Hercher-Pasteur, J., Loiseau, E., Sinfort, C., & Hélias, A. (2020). Energetic assessment of the agricultural production system. A review. Agronomy for Sustainable Development, 40(4), 1–23. https://doi.org/10.1007/s13593-020-00627-2
- Ho, B. V., Kingsbury, A., & Ho, N. S. (2022). Production efficiency and effect of sustainable land management practices on the yield of oranges in northwest Vietnam. International Journal of Agricultural Sustainability, 20(7), 1237–1248. https://doi.org/10.1080/14735903.2022.2088003
- Holmström, K., Hessle, A., Andersson, H., & Kumm, K. I. (2018). Merging small scattered pastures into large pasture-forest mosaics can improve profitability in Swedish suckler-based beef production. Land, 7(2), 58. https://doi.org/10.3390/land7020058
- Hosseinzadeh-Bandbafha, H., Nabavi-Pelesaraei, A., Khanali, M., Ghahderijani, M., & Chau, K. W. (2018). Application of data envelopment analysis approach for optimization of energy use and reduction of greenhouse gas emission in peanut production of Iran. Journal of Cleaner Production, 172, 1327–1335. https://doi.org/10.1016/j.jclepro.2017.10.282
- Hýblová, E. (2014). Analysis of mergers in Czech agriculture companies. Agricultural Economics, 60(10), 441–448. https://doi.org/10.17221/15/2014-agricecon
- Ibidunni, A. S., Ufua, D. E., Okorie, U. E., & Kehinde, B. E. (2020). Labour productivity in agricultural sector of sub-Sahara Africa (2010–2017) a data envelopment and panel regression approach. African Journal of Economic and Management Studies, 11(2), 207–232. https://doi.org/10.1108/ajems-02-2019-0083
- Ilahi, S., Wu, Y., Raza, M. A. A., Wei, W., Imran, M., & Bayasgalankhuu, L. (2019). Optimization approach for improving energy efficiency and evaluation of greenhouse gas emission of wheat crop using data envelopment analysis. Sustainability, 11(12), 3409. https://doi.org/10.3390/su11123409
- Ingram, J., & Kirwan, J. (2011). Matching new entrants and retiring farmers through farm joint ventures: Insights from the fresh start initiative in Cornwall, UK. Land Use Policy, 28(4), 917–927. https://doi.org/10.1016/j.landusepol.2011.04.001
- Kaab, A., Sharifi, M., Mobli, H., Nabavi-Pelesaraei, A., & Chau, K. W. (2019). Use of optimization techniques for energy use efficiency and environmental life cycle assessment modification in sugarcane production. Energy, 181, 1298–1320. https://doi.org/10.1016/j.energy.2019.06.002
- Khan, D., Nouman, M., Popp, J., Khan, M. A., Ur Rehman, F., & Oláh, J. (2021). Link between technically derived energy efficiency and ecological footprint: Empirical evidence from the ASEAN region. Energies, 14(13), 3923. https://doi.org/10.3390/en14133923
- Khan, D., Nouman, M., & Ullah, A. (2023). Assessing the impact of technological innovation on technically derived energy efficiency: A multivariate co-integration analysis of the agricultural sector in South Asia. Environment, Development and Sustainability, 25(4), 3723–3745. https://doi.org/10.1007/s10668-022-02194-w
- Khan, D., & Ullah, A. (2020). Comparative analysis of the technical and environmental efficiency of the agricultural sector: The case of Southeast Asia countries. Custos E Agronegocio Line, 16, 2–28.
- Khoshnevisan, B., Rafiee, S., Omid, M., & Mousazadeh, H. (2013). Reduction of CO2 emission by improving energy use efficiency of greenhouse cucumber production using DEA approach. Energy, 55, 676–682. https://doi.org/10.1016/j.energy.2013.04.021
- Khoshroo, A., Mulwa, R., Emrouznejad, A., & Arabi, B. (2013). A non-parametric data envelopment analysis approach for improving energy efficiency of grape production. Energy, 63, 189–194. https://doi.org/10.1016/j.energy.2013.09.021
- Kyrgiakos, L. S., Kleftodimos, G., Vlontzos, G., & Pardalos, P. M. (2023). A systematic literature review of data envelopment analysis implementation in agriculture under the prism of sustainability. Operational Research, 23(1), 7. https://doi.org/10.1007/s12351-023-00741-5
- Lampridi, M. G., Sørensen, C. G., & Bochtis, D. (2019). Agricultural sustainability: A review of concepts and methods. Sustainability, 11(18), 5120. https://doi.org/10.3390/su11185120
- Langarita, R., Chóliz, J. S., Sarasa, C., Duarte, R., & Jiménez, S. (2017). Electricity costs in irrigated agriculture: A case study for an irrigation scheme in Spain. Renewable and Sustainable Energy Reviews, 68, 1008–1019. https://doi.org/10.1016/j.rser.2016.05.075
- Latruffe, L., Diazabakana, A., Bockstaller, C., Desjeux, Y., Finn, J., Kelly, E., Ryan, M., & Uthes, S. (2016). Measurement of sustainability in agriculture: A review of indicators. Studies in Agricultural Economics, 118(3), 123–130. https://doi.org/10.7896/j.1624
- Le, T. L., Lee, P. P., Peng, K. C., & Chung, R. H. (2019). Evaluation of total factor productivity and environmental efficiency of agriculture in nine East Asian countries. Agricultural Economics, 65(6), 249–258. https://doi.org/10.17221/50/2018-AGRICECON
- Liang, Y., Jing, X., Wang, Y., Shi, Y., & Ruan, J. (2019). Evaluating production process efficiency of provincial greenhouse vegetables in China using data envelopment analysis: A green and sustainable perspective. Processes, 7(11), 780. https://doi.org/10.3390/pr7110780
- Lin, Y., Wang, Y. M., & Shi, H. L. (2020). Mergers and acquisitions matching for performance improvement: A DEA-based approach. Economic Research – Ekonomska istraživanja, 33(1), 3545–3561. https://doi.org/10.1080/1331677X.2020.1775673
- Liu, C., Cutforth, H., Chai, Q., & Gan, Y. (2016). Farming tactics to reduce the carbon footprint of crop cultivation in semiarid areas. A review. Agronomy for Sustainable Development, 36(1), 1–16. https://doi.org/10.1007/s13593-016-0404-8
- Llones, C. A., Mankeb, P., Wongtragoon, U., & Suwanmaneepong, S. (2022). Production efficiency and the role of collective actions among irrigated rice farms in Northern Thailand. International Journal of Agricultural Sustainability, 20(6), 1047–1057. https://doi.org/10.1080/14735903.2022.2047464
- Lozano, S., & Adenso-Díaz, B. (2021). A DEA approach for merging dairy farms. Agricultural Economics – Zemedelska Ekonomika, 67(6), 209–219. https://doi.org/10.17221/418/2020-agricecon
- Mardani Najafabadi, M., & Taki, M. (2020). Robust data envelopment analysis with Monte Carlo simulation model for optimization the energy consumption in agriculture. Energy Sources, Part A: Recovery, Utilization, and Environmental Effects. https://doi.org/10.1080/15567036.2020.1777221
- Moghaddas, Z., Oukil, A., & Vaez-Ghasemi, M. (2022). Global multi-period performance evaluation: A new model and a new productivity index. RAIRO Operations Research, 56(3), 1503–1521. https://doi.org/10.1051/ro/2022065
- Mohammadi, A., & Omid, M. (2010). Economical analysis and relation between energy inputs and yield of greenhouse cucumber production in Iran. Applied Energy, 87(1), 191–196. https://doi.org/10.1016/j.apenergy.2009.07.021
- Mohammadi, A., Rafiee, S., Jafari, A., Keyhani, A., Dalgaard, T., Knudsen, M. T., & Hermansen, J. E. (2015). Joint life cycle assessment and data envelopment analysis for the benchmarking of environmental impacts in rice paddy production. Journal of Cleaner Production, 106, 521–532. https://doi.org/10.1016/j.jclepro.2014.05.008
- Mohseni, P., Borghei, A. M., & Khanali, M. (2018). Coupled life cycle assessment and data envelopment analysis for mitigation of environmental impacts and enhancement of energy efficiency in grape production. Journal of Cleaner Production, 197, 937–947. https://doi.org/10.1016/j.jclepro.2018.06.243
- Mwambo, F. M., Fürst, C., Martius, C., Jimenez-Martinez, M., Nyarko, B. K., & Borgemeister, C. (2021). Combined application of the EM-DEA and EX-ACT approaches for integrated assessment of resource use efficiency, sustainability and carbon footprint of smallholder maize production practices in sub-Saharan Africa. Journal of Cleaner Production, 302, Article 126132. https://doi.org/10.1016/j.jclepro.2021.126132
- Nabavi-Pelesaraei, A., Rafiee, S., Mohtasebi, S. S., Hosseinzadeh-Bandbafha, H., & Chau, K. W. (2017). Energy consumption enhancement and environmental life cycle assessment in paddy production using optimization techniques. Journal of Cleaner Production, 162, 571–586. https://doi.org/10.1016/j.jclepro.2017.06.071
- Nacer, T., Hamidat, A., & Nadjemi, O. (2016). A comprehensive method to assess the feasibility of renewable energy on Algerian dairy farms. Journal of Cleaner Production, 112, 3631–3642. https://doi.org/10.1016/j.jclepro.2015.06.101
- Najafabadi, M. M., Sabouni, M., Azadi, H., & Taki, M. (2022). Rice production energy efficiency evaluation in north of Iran; application of robust data envelopment analysis. Cleaner Engineering and Technology, 6, Article 100356. https://doi.org/10.1016/j.clet.2021.100356
- Nayak, H. S., Parihar, C. M., Aravindakshan, S., Silva, J. V., Krupnik, T. J., McDonald, A. J., & Sapkota, T. B. (2023). Pathways and determinants of sustainable energy use for rice farms in India. Energy, 272, Article 126986. https://doi.org/10.1016/j.energy.2023.126986
- Nourani, A., & Bencheikh, A. (2020). Energy requirement optimization of greenhouse vegetable production using data envelopment analysis (DEA) method in Algeria. Acta Technologica Agriculturae, 23(2), 60–66. https://doi.org/10.2478/ata-2020-0010
- Nourani, A., & Bencheikh, A. (2021). Applying of DEA approach to optimize the energy required and GHG emission for greenhouse vegetable production in Algeria. Agricultural Engineering International: CIGR Journal, 23(3), 156–164.
- Oral, M., Amin, G. R., & Oukil, A. (2015). Cross-efficiency in DEA: A maximum resonated appreciative model. Measurement, 63, 159–167. https://doi.org/10.1016/j.measurement.2014.12.006
- Oral, M., Oukil, A., Malouin, J.-L., & Kettani, O. (2014). The appreciative democratic voice of DEA: A case of faculty academic performance evaluation. Socio-Economic Planning Sciences, 48(1), 20–28. https://doi.org/10.1016/j.seps.2013.08.003
- Oukil, A. (2008). Exploiting sparsity in vehicle routing algorithms [Doctoral diss., Lancaster University], https://eprints.lancs.ac.uk/id/eprint/45150.
- Oukil, A. (2018). Ranking via composite weighting schemes under a DEA cross-evaluation framework. Computers & Industrial Engineering, 117, 217–224. https://doi.org/10.1016/j.cie.2018.01.022
- Oukil, A. (2019). Embedding OWA under preference ranking for DEA cross-efficiency aggregation: Issues and procedures. International Journal of Intelligent Systems, 34(5), 947–965. https://doi.org/10.1002/int.22082
- Oukil, A. (2020). Exploiting value system multiplicity and preference voting for robust ranking. Omega, 94, Article 102048. https://doi.org/10.1016/j.omega.2019.03.006
- Oukil, A. (2022). Selecting material handling equipment through a market weight scheme based DEA cross-efficiency approach. International Journal of Management Science and Engineering Management, 1–14. https://doi.org/10.1080/17509653.2022.2116122
- Oukil, A. (2023a). A two-level induced OWA procedure for ranking DMUs under a DEA cross-efficiency framework. In Intelligent and Transformative Production in Pandemic Times: Proceedings of the 26th International Conference on Production Research (pp. 495–521). Springer International Publishing. https://doi.org/10.1007/978-3-031-18641-7_47
- Oukil, A. (2023b). Investigating prospective gains from mergers in the agricultural sector through inverse DEA. IMA Journal of Management Mathematics, 34(3), 465–490. https://doi.org/10.1093/imaman/dpac004
- Oukil, A., & Al-Zidi, A. (2018). Benchmarking the hotel industry in Oman through a three-stage DEA-based procedure. Journal of Arts and Social Sciences [JASS], 9(2), 5–23. https://doi.org/10.24200/jass.vol9iss2pp5-23
- Oukil, A., & Amin, G. R. (2015). Maximum appreciative cross-efficiency in DEA: A new ranking method. Computers & Industrial Engineering, 81, 14–21. https://doi.org/10.1016/j.cie.2014.12.020
- Oukil, A., & Amin, G. R. (2023). An induced OWA aggregation operator with dual preference setting for DEA cross-efficiency ranking. Soft Computing, 27(24), 18419–18440. https://doi.org/10.1007/s00500-023-09235-0
- Oukil, A., Channouf, N., & Al-Zaidi, A. (2016). Performance evaluation of the hotel industry in an emerging tourism destination: The case of Oman. Journal of Hospitality and Tourism Management, 29, 60–68. https://doi.org/10.1016/j.jhtm.2016.05.003
- Oukil, A., & El-Bouri, A. (2021). Ranking dispatching rules in multi-objective dynamic flow shop scheduling: A multi-faceted perspective. International Journal of Production Research, 59(2), 388–411. https://doi.org/10.1080/00207543.2019.1696487
- Oukil, A., & Govindaluri, S. M. (2017). A systematic approach for ranking football players within an integrated DEA-OWA framework. Managerial and Decision Economics, 38(8), 1125–1136. https://doi.org/10.1002/mde.2851
- Oukil, A., & Govindaluri, S. M. (2020). A hybrid multi-attribute decision-making procedure for ranking project proposals: A historical data perspective. Managerial and Decision Economics, 41(3), 461–472. https://doi.org/10.1002/mde.3113
- Oukil, A., Kennedy, R. E., Al-Hajri, A., & Soltani, A. A. (2024). Unveiling the potential of hotel mergers: A hybrid DEA approach for optimizing sector-wide performance in the hospitality industry. International Journal of Hospitality Management, 116, Article 103620. https://doi.org/10.1016/j.ijhm.2023.103620
- Oukil, A., Nourani, A., Soltani, A. A., & Benchikh, A. (2022). Using inverse data envelopment analysis to investigate potential impact of mergers on energy use optimization – application in agricultural production. Journal of Cleaner Production, 381(1), Article 135199. https://doi.org/10.1016/j.jclepro.2022.135199
- Oukil, A., Soltani, A. A., Al-Mezeini, N., Al-Ismaili, A., & Nourani, A. (2023). Mergers as an alternative for energy use optimization: Evidence from the cucumber greenhouse production using the inverse DEA approach. Environment, Development and Sustainability, 1–26. https://doi.org/10.1007/s10668-023-04144-6
- Oukil, A., Soltani, A. A., Boutaghane, H., Abdalla, O., Bermad, A., Hasbaia, M., & Boulassel, M. R. (2021). A Surrogate Water Quality Index to assess groundwater using a unified DEA-OWA framework. Environmental Science and Pollution Research, 28(40), 56658–56685. https://doi.org/10.1007/s11356-021-13758-0
- Oukil, A., Soltani, A. A., Zeroual, S., Boutaghane, H., Abdalla, O., Bermad, A., Hasbaia, M., & Boulassel, M. R. (2022). A DEA cross-efficiency inclusive methodology for assessing water quality: A Composite Water Quality Index. Journal of Hydrology, 612, Part A, Article 128123. https://doi.org/10.1016/j.jhydrol.2022.128123
- Ozkan, B., Ceylan, R. F., & Kizilay, H. (2011). Comparison of energy inputs in glasshouse double crop (fall and summer crops) tomato production. Renewable Energy, 36(5), 1639–1644. https://doi.org/10.1016/j.renene.2010.11.022
- Paramesh, V., Arunachalam, V., Nikkhah, A., Das, B., & Ghnimi, S. (2018). Optimization of energy consumption and environmental impacts of arecanut production through coupled data envelopment analysis and life cycle assessment. Journal of Cleaner Production, 203, 674–684. https://doi.org/10.1016/j.jclepro.2018.08.263
- Payandeh, Z., Jahanbakhshi, A., Mesri-Gundoshmian, T., & Clark, S. (2021). Improving energy efficiency of barley production using joint data envelopment analysis (DEA) and life cycle assessment (LCA): Evaluation of greenhouse gas emissions and optimization approach. Sustainability, 13(11), 6082. https://doi.org/10.3390/su13116082
- Pendharkar, P. C. (2002). A potential use of data envelopment analysis for the inverse classification problem. Omega, 30(3), 243–248. https://doi.org/10.1016/S0305-0483(02)00030-0
- Pham, L. V., & Smith, C. (2014). Drivers of agricultural sustainability in developing countries: A review. Environment Systems and Decisions, 34(2), 326–341. https://doi.org/10.1007/s10669-014-9494-5
- Piñeiro, V., Arias, J., Dürr, J., Elverdin, P., Ibáñez, A. M., Kinengyere, A., Opazo, C. M., Owoo, N., Page, J. R., Prager, S. D., & Torero, M. (2020). A scoping review on incentives for adoption of sustainable agricultural practices and their outcomes. Nature Sustainability, 3(10), 809–820. https://doi.org/10.1038/s41893-020-00617-y
- Pishgar-Komleh, S. H., Zylowski, T., Rozakis, S., & Kozyra, J. (2020). Efficiency under different methods for incorporating undesirable outputs in an LCA+ DEA framework: A case study of winter wheat production in Poland. Journal of Environmental Management, 260, Article 110138. https://doi.org/10.1016/j.jenvman.2020.110138
- Rahbari, H., Mahmoudi, A., & Ajabshirchi, Y. (2013). Improving energy use efficiency of greenhouse tomato production using data envelopment analysis (DEA) technique. International Journal of Agriculture, 3(3), 559.
- Raheli, H., Rezaei, R. M., Jadidi, M. R., & Mobtaker, H. G. (2017). A two-stage DEA model to evaluate sustainability and energy efficiency of tomato production. Information Processing in Agriculture, 4(4), 342–350. https://doi.org/10.1016/j.inpa.2017.02.004
- Rasheed, N., Khan, D., & Magda, R. (2022). The influence of institutional quality on environmental efficiency of energy consumption in BRICS countries. Frontiers in Energy Research, 10, Article 943771. https://doi.org/10.3389/fenrg.2022.943771
- Ren, C., Liu, S., Van Grinsven, H., Reis, S., Jin, S., Liu, H., & Gu, B. (2019). The impact of farm size on agricultural sustainability. Journal of Cleaner Production, 220, 357–367. https://doi.org/10.1016/j.jclepro.2019.02.151
- Saeidi, E., Dehkordi, A. L., & Nabavi-Pelesaraei, A. (2022). Potential for optimization of energy consumption and costs in saffron production in central Iran through data envelopment analysis and multi-objective genetic algorithm. Environmental Progress & Sustainable Energy, 41(5), e13857. https://doi.org/10.1002/ep.13857
- Saeidi, R. G., Oukil, A., Amin, G. R., & Raissi, S. (2015). Prioritization of textile fabric defects using ordered weighted averaging operator. The International Journal of Advanced Manufacturing Technology, 76(5), 745–752. https://doi.org/10.1007/s00170-014-6227-3
- Sarkar, A., Wang, H., Rahman, A., Qian, L., & Memon, W. H. (2022). Evaluating the roles of the farmer's cooperative for fostering environmentally friendly production technologies – a case of kiwi-fruit farmers in Meixian, China. Journal of Environmental Management, 301, Article 113858. https://doi.org/10.1016/j.jenvman.2021.113858
- Sefeedpari, P., Shokoohi, Z., & Pishgar-Komleh, S. H. (2020). Dynamic energy efficiency assessment of dairy farming system in Iran: Application of window data envelopment analysis. Journal of Cleaner Production, 275, Article 124178. https://doi.org/10.1016/j.jclepro.2020.124178
- Sexton, T. R., Silkman, R. H., & Hogan, A. J. (1986). Data envelopment analysis: Critique and extensions. New Directions for Program Evaluation, 1986(32), 73–105. https://doi.org/10.1002/ev.1441
- Singh, G., Singh, P., & Sodhi, G. P. S. (2019). Applying DEA optimization approach for energy auditing in wheat cultivation under rice-wheat and cotton-wheat cropping systems in north-western India. Energy, 181, 18–28. https://doi.org/10.1016/j.energy.2019.05.147
- Singh, G., Singh, P., Sodhi, G. P. S., & Tiwari, D. (2021). Energy auditing and data envelopment analysis (DEA) based optimization for increased energy use efficiency in wheat cultivation (Triticum aestium L.) in north-western India. Sustainable Energy Technologies and Assessments, 47, Article 101453. https://doi.org/10.1016/j.seta.2021.101453
- Singh, J. (2002). On farm energy use pattern in different cropping systems in Haryana, India [Master of Science, International Institute of Management, University of Flensburg].
- Singh, P., Singh, G., & Sodhi, G. P. S. (2022). Data envelopment analysis based optimization for improving net ecosystem carbon and energy budget in cotton (Gossypium hirsutum L.) cultivation: Methods and a case study of north-western India. Environment, Development and Sustainability, 24(2), 2079–2119. https://doi.org/10.1007/s10668-021-01521-x
- Soheilifard, F., Taki, M., & van Zelm, R. (2021). Impact of energy flow optimization on the mitigation of environmental consequences and costs in greenhouse cucumber production. Environmental Science and Pollution Research, 28(7), 8421–8433. https://doi.org/10.1007/s11356-020-11219-8
- Soltani, A. A., Oukil, A., Boutaghane, H., Bermad, A., & Boulassel, M. R. (2021). A new methodology for assessing water quality, based on data envelopment analysis: Application to Algerian dams. Ecological Indicators, 121, Article 106952. https://doi.org/10.1016/j.ecolind.2020.106952
- Sow, O., Oukil, A., Ndiaye, B. M., & Marcos, A. (2016). Efficiency analysis of public transportation subunits using DEA and bootstrap approaches–Dakar Dem Dikk case study. Journal of Mathematics Research, 8(6), 114–127. https://doi.org/10.5539/jmr.v8n6p114
- Sugden, F., Agarwal, B., Leder, S., Saikia, P., Raut, M., Kumar, A., & Ray, D. (2021). Experiments in farmers’ collectives in Eastern India and Nepal: Process, benefits, and challenges. Journal of Agrarian Change, 21(1), 90–121. https://doi.org/10.1111/joac.12369
- Tu, V. H., Can, N. D., Takahashi, Y., Kopp, S. W., & Yabe, M. (2019). Technical and environmental efficiency of eco-friendly rice production in the upstream region of the Vietnamese Mekong delta. Environment, Development and Sustainability, 21(5), 2401–2424. https://doi.org/10.1007/s10668-018-0140-0
- United Nations. (2022). Peace, dignity and equality on a healthy planet. Retrieved October 6, 2023, from https://www.un.org/en/global-issues/population
- Vu, T. H., Peng, K. C., & Chung, R. H. (2019). Evaluation of environmental efficiency of edible canna production in Vietnam. Agriculture, 9(11), 242. https://doi.org/10.3390/agriculture9110242
- Wang, Y.-M., & Parkan, C. (2005). A minimax disparity approach for obtaining OWA operator weights. Information Sciences, 175(1), 20–29. https://doi.org/10.1016/j.ins.2004.09.003
- Xu, X., Chen, Q., & Zhu, Z. (2022). Evolutionary overview of land consolidation based on bibliometric analysis in web of science from 2000 to 2020. International Journal of Environmental Research and Public Health, 19(6), 3218. https://doi.org/10.3390/ijerph19063218
- Yager, R. R. (1988). On ordered weighted averaging aggregation operators in multicriteria decision making. IEEE Transactions on Systems, Man, and Cybernetics, 18(1), 183–190. https://doi.org/10.1109/21.87068
- Yager, R. R. (1995). An approach to ordinal decision making. International Journal of Approximate Reasoning, 12(3), 237–261. https://doi.org/10.1016/0888-613X(94)00035-2
- Yu, H., Ding, W., Luo, J., Geng, R., & Cai, Z. (2012). Long-term application of organic manure and mineral fertilizers on aggregation and aggregate-associated carbon in a sandy loam soil. Soil and Tillage Research, 124, 170–177. https://doi.org/10.1016/j.still.2012.06.011
- Zahm, F., Ugaglia, A. A., Barbier, J. M., Boureau, H., Del’homme, B., Gafsi, M., Gasselin, P., Girard, S., Guichard, L., Loyce, C., Manneville, V., Menet, A., & Redlingshöfer, B. (2019). Évaluer la durabilité des exploitations agricoles. La méthode IDEA v4, un cadre conceptuel combinant dimensions et propriétés de la durabilité. Cahiers Agricultures, 28(5), 1–11. https://doi.org/10.1051/cagri/2019004
- Zaier, R., Zekri, S., Jayasuriya, H., Teirab, A., Hamza, N., & Al-Busaidi, H. (2015). Design and implementation of smart irrigation system for groundwater use at farm scale. In 7th International Conference on Modelling, Identification and Control (ICMIC) (pp. 1–6). IEEE.
- Zeng, S., Zhu, F., Chen, F., Yu, M., Zhang, S., & Yang, Y. (2018). Assessing the impacts of land consolidation on agricultural technical efficiency of producers: A survey from Jiangsu province, China. Sustainability, 10(7), 2490. https://doi.org/10.3390/su10072490
- Zhou, H., Yang, Y., Chen, Y., & Zhu, J. (2018). Data envelopment analysis application in sustainability: The origins, development and future directions. European Journal of Operational Research, 264(1), 1–16. https://doi.org/10.1016/j.ejor.2017.06.023
Appendices
Appendix 1. List of abbreviations
Appendix 2
Table A1. Energy equivalent coefficients in tomato GH production.
Appendix 3. OWA aggregation operator
An OWA aggregation operator of dimension I is a mapping associated with a weigh vector
and defined as
where
is the value of the
th largest factor of the vector of arguments
and
its associated OWA weight (Saeidi et al., Citation2015). In our case, we use the following minimax disparity model (Wang & Parkan, Citation2005) to generate the OWA weight vector
(A1)
(A1) The objective of model (WP) consists of minimizing the deviation
between successive aggregation weights
and
,
Such a deviation is duly formulated through constraints (A1). The DM’s optimism level is reflected by the parameter
also known as orness value (Yager, Citation1995).
In each vector of votes relating to energy input
the importance of each vote
is implicitly induced by the associated rank
i.e. the smaller the value of
the more important is the vote
Therefore, the vector of weights
can be used directly to aggregate the votes of
into a single vote
without an a priori ordering, where
(A2)
(A2) The aggregate vote
reflects the relative importance of input
from the perspective of a DM whose optimism level is
High values of
imply that a large number of experts voted for energy input
to be among the leading inputs.