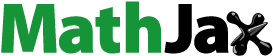
ABSTRACT
Technologies are essential for reducing environmental impact and increasing agricultural productivity. Previous adoption studies indicate several socioeconomic, technological, and institutional variables influencing the adoption of agricultural technologies. With less than a decade to the United Nation's sustainable development agenda for 2030, there are yet reports on farmers' resistance to sustainable agricultural technologies. Using a survey and multistage sampling procedure in selecting the respondents of 405 smallholder Chinese farmers, the study models farmers' adoption intensity of sustainable agricultural technologies and their impact on productivity. A 5-point Likert scale was used to analyse farmers' SAT perception levels. The PSM was used to eliminate a major proportion of bias when estimating a more precise treatment effect or impact of SAT adoption on productivity. The findings showed that farmers have mixed perceptions (positive and negative) about SAT adoption, which is reflected in their adoption decisions. The study found that family members as extension officers positively impacted farmers' adoption behaviour. On the contrary, regular visits by agricultural extension services had no impact on farmers' adoption behaviour, but positive on productivity. The results clearly showed elements of constraints in farmers' decision-making. The ATT results showed a significant and positive impact of SATs' adoption on productivity.
1. Introduction
A key challenge for the agriculture sector is to feed an increasing global population while reducing the environmental impact and preserving natural resources for future generations (Bannor et al., Citation2020). By 2050, the world’s growing population is projected to increase food demand by 60%. The United Nations’ Sustainable Development Goal (SDG-2), therefore, aims to achieve ‘zero hunger’ (Abideen et al., Citation2021; Vabi Vamuloh et al., Citation2019). Consequently, food production expansion objectives and practices threaten water scarcity, environmental degradation and negative social impacts on humans and natural ecosystems (Arhin et al., Citation2022). By incorporating SDG-2, the term ‘sustainable agriculture’ refers to a comprehensive and integrated approach to food production and land management to achieve food security, enhance nutrition, ecosystem services and sustainable livelihoods and mitigate the harmful effects of agriculture on the environment (Anang et al., Citation2020; Atieno et al., Citation2020). The shift towards sustainable agriculture is important for countries like China, where agriculture is pivotal to the economy and faces special difficulties because of fast industrialization and population increase (Vabi Vamuloh et al., Citation2019). Sustainable agricultural technologies (SATs) are more environment-friendly and allow the production of goods and services without destroying human and natural systems (Li et al., Citation2020). SATs in modern agricultural development contribute to higher yields using fewer inputs and less damage to agricultural production (Sitthisuntikul et al., Citation2018). SATs are also key to the social, technological and economic development of countries (Lampach et al., Citation2017).
During the last two decades, the agricultural sector has witnessed an upsurge of interest in sustainable technologies (Anang et al., Citation2020). For example, smartphone applications have been used for remote monitoring and controlling farming equipment. Soil and crop smart sensors are used to read crop health and essential nitrogen levels in water (Pello et al., Citation2021; Vabi Vamuloh et al., Citation2019). Sustainable agricultural practices such as climate-smart agriculture, green production and conservation tillage are the anticipated trend of solutions for current sustainability issues (Chuang & Wang, Citation2020). The adoption of sustainable technologies and practices often requires a paradigm shift in policies and practices. Nevertheless, the agriculture sector often experiences low uptake and an array of adoption barriers from farmers (Biggs et al., Citation2018). Farmers’ adoption barriers include lack of finance, land constraints, lack of commitment, knowledge from farmers, etc. (Bannor et al., Citation2020). The literature on agricultural technology adoption has also been characterized by many driving factors such as farmers’ intentions, socio-economics, psychological, institutional and individual farmers’ characteristics (Asiedu-Darko, Citation2014; Lampach et al., Citation2017; Qiao et al., Citation2016).
In the tea sector, studies such as (Arhin et al., Citation2023; Atieno et al., Citation2020; Liu et al., Citation2019) have questioned the production methods used by Chinese tea farmers, the environmental effects of their farming practices and the overall sustainability of tea farming. For example, (Asiedu-Ayeh et al., Citation2022) indicated that excessive chemical usage, pesticides, weedicides, etc. in the Chinese tea industry cause detrimental production challenges and sustainability issues (Chavas & Nauges, Citation2020; Mariyono, Citation2019). Nevertheless, most studies on agricultural technologies in tea farming are limited to the adoption intensity (Baumgart-Getz et al., Citation2012; Bui & Nguyen, Citation2021; Mahama et al., Citation2020; Tey & Brindal, Citation2012), farmers’ adoption behaviour from the context of a specific technology adoption (Adesina & Baidu-Forson, Citation1995; Arellanes & Lee, Citation2003; Azman et al., Citation2013; Koner & Laha, Citation2021; Loos & Wehrden, Citation2018; van Hulst et al., Citation2020), farmer characteristics which influence the farmers’ demands or willingness to adopt sustainable technologies (Chang, Citation2013;Gołaś et al., Citation2020;Li et al., Citation2020; Viatte, Citation2001; Williamson, Citation2002) and perception of farmers (Bu et al., Citation2020; Mottaleb, Citation2018). Other studies looked at the social, economic and environmental aspects of producing tea (Sarkar et al., Citation2020; Wang et al., Citation2022). Nevertheless, a significant research gap still exists in fully comprehending how Chinese tea farmers view and incorporate SATs into their practices, amidst the rising awareness of environmental concerns in the tea industry. There is a lack of study that comprehensively explores the farmers’ perspectives on SATs and their consequences for tea production, particularly for China. The following questions or gaps exist in the literature: What is the perception of Chinese tea farmers towards SATs? What effects do the perceptions have on SATs’ adoption in tea production? Also, many agricultural technologies have the potential to help address the current challenges in agriculture (Anang, Citation2018). Yet, some technologies can create new and even more challenging problems, particularly when the technologies are not governed in a sustainable way (Mottaleb, Citation2018). Hence, SATs must be carefully integrated with the perceptions, attitudes and goals of the farmers. Some examples of SATs measured in the study include the application of biofertilizers, organic fertilizers, advanced sensors, drones, GPS mapping, data analytics (to maximize crop yields, lower inputs like water and organic fertilizer and decrease waste), integrated pest management (IPM) system and drip irrigation.
The study applies SATs in the tea sector instead of other agricultural sectors to investigate novel strategies of SATs that farmers incorporate into tea farming aiming at maintaining both productivity and ecological balance. Also, the Chinese tea industry faces complex problems that call for deliberate intervention as the global market observes dynamic transitions in agricultural practices towards sustainability (Calicioglu et al., Citation2019). Thus, the objectives of the study are three folds: first to examine farmers’ perception of SATs, second to model the adoption behaviour and evaluate the factors that have a significant influence on smallholder farmers’ decisions. The final objective is to assess the impact of SATs’ adoption on productivity. The study contributes to SATs adoption literature and a guide to stakeholders’ choices and practical initiatives aimed at improving China’s tea industry sustainability. Sustainable agricultural study in China is unique as China is the largest emerging agricultural country in the world and among the top tea-producing countries. Accordingly, the findings of the study will serve as a model for studies of similar tea countries. The study findings will be useful in the development of sustainable agricultural policies for tea-growing countries. For example, it will help in developing sustainable technology control interventions that are adaptive to different local conditions and farming systems across tea-growing countries. The rest of the study is organized as follows. Section two looks at the literature review, and section three considers the materials and methods. Section four looks at the results. Section five deals with discussion, conclusions and recommendations.
2. Literature review on SATs
There are currently many sustainable agricultural practices or technologies carried out in the tea industry. For example, sustainable soil management techniques are essential for preserving soil health and increasing tea yield (Munasinghe et al., Citation2017). IPM techniques are also popular as eco-friendly substitutes for chemical pest control techniques in tea farms (Dunn et al., Citation2023). For example, pheromone traps and biological control agents are commonly used (de Mello Brandão Vinholis et al., Citation2021) to manage common tea pests. IPM helps protect the environment and provides safer tea products by using fewer pesticides. This improves tea flavours, and taste and enhances human health. The literature on SATs in the Chinese tea industry highlights various efforts to promote environmental sustainability and productivity. For example, sustainable soil management, covering crops, intercropping and IPM techniques are being employed to maintain soil health, reduce erosion and limit the use of synthetic fertilizers and pesticides (Gerasimova et al., Citation2021). Organic farming practices, such as composting and biological pest control, are also gaining traction for their positive impact on soil health and reducing chemical inputs. However, climate change poses challenges, prompting the development of climate-resilient tea cultivars (Liu et al., Citation2019). Although the advantages of SATs are acknowledged (Silva et al., Citation2023), widespread adoption is still difficult due to economic factors, particularly in areas with high rates of poverty (Hu et al., Citation2022). Also, SATs are being adopted due to many factors, including environmental concerns, norms, perceived costs and benefits and knowledge. The adoption by farmers is impeded by various factors such as age, land type, financial incentives, technological support and training. Thus, China is yet known to use a lot of chemicals, especially fertilizers, which may lead to ecological imbalances. There is room for improvement in the efficiency of tea production, due to the ageing population of tea-producing farmers which has negative effects. While there might exist an inverted U-shaped relationship between farmers’ age and technical efficiency, the ageing of tea-producing farmers exerts a negative impact on technical efficiency in tea production. Asiedu-Ayeh et al. (Citation2022) study result shows that the five most important factors promoting the adoption of agricultural green production technologies include knowledge (0.828), perceived cost and benefit (0.819), descriptive norm (0.810), moral and environmental concern (0.809) and injunctive norm (0.807). The study of Arhin et al. (Citation2023) established the significance and suitability of different approaches in farmers’ adoption behaviour in the tea industry and emphasized that the specificity of farmers’ sustainable agriculture technologies adoption behaviour is essentially not compromised by any codified variables but the interrelationship among the determinants, which includes socio-demographic, agro-ecological and environmental, technological, institutional and social networks. According to (López-Felices et al., Citation2023) sustainable agriculture is a vital aspect of modern farming practices and its adoption is critical to ensure the longevity of agricultural productivity and protect the environment. Assessing risk perception of pests and diseases in tea farmers in Sichuan province, China, Hu et al. (Citation2022) found that risk perception has a negative impact on behavioural intention. Performance expectancy, effort expectancy and social influence can positively affect behavioural intention and facilitating conditions can also positively influence usage behaviour (Dong & Liang, Citation2023). In measuring tea farmers’ perceptions of sustainable agriculture in Turkey, Ul Haq et al. (Citation2022) study showed that education level, age, participation in farming events, watching television and using the internet affected farmers’ perception of sustainable farming in the region. To understand farmers’ decision to convert to organic tea cultivation in Vietnam, Bui and Nguyen (Citation2021) survey reveals that farm size, participation in training programmes, access to credit, access to extension services, technology support and market access had a positive impact on the adoption of organic farming methods. Furthermore, the novice organic tea farmers living in undeveloped regions, one with high poverty levels and economic motives played an important role in the decision to convert to organic practices (López-Felices et al., Citation2023). Although the tea farmers had a good awareness of the health and environmental benefits brought by organic farming practices, this had so far not led to conversion on a large scale. Liu et al., Citation2019 shows that the average technical efficiency in tea production in Meitan County, China is 0.581, implying great room for improving technical efficiency in tea production.
3. Materials and methods
3.1. The study area and sampling method
The study was carried out in China. China has a prominent landscape of rivers and lakes, mountainous areas and tropics and boasts of large-scale tea production (Lu & Xie, Citation2018). Different socio-economic and agro-ecological areas were selected as the sample area. The five distinctive selected tea-producing provinces are Zhejiang province (the oldest and first province to have exported organic tea), Anhui and Fujian Provinces, all from Eastern China (Wang et al., Citation2016), the Hubei provinces from Southern Chinaand the Yunnan province from Southwestern China. These provinces are significant to the production of tea and special tea such as organic tea. In this study, tea productivity is measured as the quantity of output a farmer can generate over a specific time frame. The provinces have certified organic areas (Li et al., Citation2020) and land used for cultivating crops such as tea, rice and vegetables. See Appendix for the 2020 annual tea production of the provinces in metric tons. SATs and sustainable agriculture are modern concepts that have an element of complexity to understand and practices. Farmers in the five selected provinces are considered relatively well-educated, specialized in high-value products and receptive to new ideas (Zheng et al., Citation2019). Hence, sustainable issues such as the adoption of SATs are justifiable to be conducted in these provinces. Environmental issues are currently a big problem for tea production, particularly pesticide and fertilizer use leading to a significant loss of agricultural biodiversity, soil deterioration and soil contamination. Many areas of the country, including the five selected provinces, are affected by these issues. Switching to more sustainable production practices will benefit the country (Gaitán-Cremaschi et al., Citation2020). In the face of the natural environmental conditions favourable for growing tea and the support for sustainable tea production from the government, the study seeks to improve SATs’ integration in tea production. shows the geographical location of the selected provinces.
Using the survey approach to collect data in the five provinces, the target population was smallholder organic tea farmers who have adopted at least one of the measured SATs used in the study or smallholder farmers who have not adopted any of the SATs. The organic tea sector was purposively chosen due to its significant role in sustainable agriculture and the unique features of its cultivation, production and marketing requirements. The data collection lasted for 6 months (i.e. from May 2021 to October 2021). The sampling procedure is as follows: A multi-stage sampling procedure was adopted to select the respondents. First, a multi-stage stratified random sampling procedure was adopted. In the first stage, the five provinces were purposively selected based on their unique and significant contributions to the total tea production in China. In the second stage, three counties with major tea production were randomly selected from each of the provinces. This brought a total of 15 counties. In the third stage, two or three communities within the counties were selected based on their contribution to tea production. Finally, there were 27 households in total, randomly selected from a list of farmers. The sample size of 405 was computed as shown below.
First, 5 provinces selected = 5 provinces.
Second, 3 counties selected from each province = 3 × 5 = 15 counties.
Third, 27 respondents’(household) from 2 or 3 communities under the 15 counties = 15× 27 = 405 respondents.
3.2. Econometric models
The three models used include the ranking technique, probit model and propensity score matching (PSM). The study adopted the ranking technique to analyse farmers’ SATs’ perception levels. As perception affects the adoption of technology, the study adopted the probit model to determine the factors that drive farmers’ adoption. After adoption, the study measured the adoption effect on farmers’ productivity. The PSM was used to eliminate the major proportion of bias when estimating a more precise treatment effect or impact of SATs’ adoption on farmers’ productivity. The ranking technique is a method used in research to measure individuals’ perceptions or preferences by having them rank a set of items or options according to a specified criterion. It allows researchers to understand the relative importance or order of preference among different items based on respondents’ perspectives. This technique is particularly useful when dealing with subjective or qualitative data, such as perceptions, attitudes or preferences as used in the study. Using a 5-point Likert scale as the ranking technique, this study examined the variables affecting farmers’ perceptions. The ranking for the farmers’ perception variable was as follows:
Strongly disagree = 1.
Disagree = 2.
Neutral = 3.
Agree = 4.
Strongly agree = 5.
The ranking variables were interpreted in this study as the degree of satisfaction utility a respondent experiences. The more satisfied a farmer, the more probable it is that they will rate SATs high.
The probit model was used to determine the factors that drive farmers’ SATs’ adoption. There are two approaches to finding these factors (risk sharing and transaction cost approach). In risk sharing, there are risk preferences, farm characteristics or producer characteristics. We adopted the risk-sharing approach because the risk-sharing approach involves considering the risks associated with adopting SATs as new technologies. The model assesses the factors influencing farmers’ perceptions of these risks since new technology adoption frequently implies uncertainty. The model also measures uncertainty and how it affects farmer perceptions, which offers a methodical approach to examining the complexity of elements affecting perceptions. A risk-sharing coefficient or parameter was introduced i.e Tj∗ = α⋅σ(Zj+μj), where α represents the risk-sharing coefficient that quantifies the extent to which risk is shared and σ(Zj+μj) denotes the standard deviation of the sum of the instrument Zj and the error term μj. The adoption of SATs is the dependent variable and social-economic factors including age, educational level, farm size, income, a family member being an extension officer and visits to extension services are the independent variables. Using the random utility framework to model the SATs’ adoption decision and following (Ali & Abdulai, Citation2010).
Let’s suppose T* denotes the difference between the utility from SATs’ adoption (UA) and non-adoption (UNA). A utility maximizing farmer j would adopt SATs if the utility derived from adoption is greater than the utility from non-adoption such that T* = UA − UNA > 0. Given that utility is unobservable, it can be expressed as a function of an observable element in the latent variable, as expressed in Equation (2):
(1)
(1) Given that,
is a binary indicator variable that equals 1 if a farmer adopts SATs and zero if otherwise,
is a vector of parameters to be estimated,
is a vector of explanatory variables associated with adoption and μ is the random error term.
Following this assumption, it is proposed that the probability of a farmer adopting SATs is expected to be influenced by household size, farm size and participation in cooperatives. Also, farmer characteristics, such as education level, are expected to increase the probability of SATs’ adoption. Gender is expected to influence adoption and access to extension services is expected to positively influence adoption. Income level among smallholder farmers is expected to influence SATs’ adoption (Anang et al., Citation2020; Mottaleb, Citation2018). Participating in cooperatives is included to ascertain how social network influences smallholder farmers’ adoption decisions. Social networks, for instance, serve as platforms for the communication of knowledge, experiences and information among tea farmers. Farmers who are part of vibrant networks may have access to insightful information regarding SATs, which may positively affect perspectives.
The PSM is the probability of a subject receiving a treatment T conditional on the set of confounders (X). The study adopted the PSM matching to eliminate the major proportion of bias when estimating a more precise treatment effect or impact of SATs’ adoption on farmers’ productivity. Also, PSM is used as it offers more advantages such as controlling for confounding by indication in observational studies over the traditional regression methods. The choice of the methods used in the study is subject to the research objectives and the characteristics of the dataset. In this study, PSM is sufficient to address the research questions without the need for more complex techniques like the Endogeneity Selection Rule (ESR). Matching algorithms are statistical techniques applied in research to establish comparable groups from a collection of subjects, predominantly when randomization is not possible. In this study, the three matching algorithms (Nearest neighbour matching, Kernel matching and Calliper matching) were employed to address potential biases and confounding factors when comparing farmers of adopters and non-adopters in the organic tea industry.
The matching algorithms were used to produce matched groups that are comparable in terms of important factors that could otherwise skew the study's findings. For example, a variety of factors affect adoption outcomes in the context of SATs’ adoption among farmers in the organic tea sector (income, education, farm size, family member as extension officer, etc.) Using the different matching algorithms complemented the weakness of each algorithm in ensuring that the groups being compared are comparable in these areas and help minimize the effect of confounding factors on the study's conclusion. The study adopted the content validity technique (CVI) to measure the validity of the specified domain of variables that are relevant to the concept measured. The experts in the field were consulted. A sample of the questionnaires was sent to the experts (professors) to check the relevance of the questions to the study objectives and measured variables. These experts rated the questionnaire in order of ‘Very good, good, not good, bad’. The validity of the instrument was then measured using the Content validity index (CVI). Overall, the CVI was 0.648, which is conclusive that the questionnaires were relevant to the study objectives. According to the literature (Kothari, Citation2004), a CVI alpha (x) of 0.6 or more is an indication that the instrument has acceptable validity. To check for data reliability, the study adopted Cronbach’s alpha coefficient to measure the degree to which the research instrument yielded consistent results after trials. The internal consistency was 0.78 using the Cronbach alpha coefficient for most of the variables. Generally, a Cronbach value of 0.75 or more is considered acceptable or indicates consistency and reliable measure for the variables used. shows how study variables were measured in the following order.
Table 1. The measurement of study variables.
4. Results
4.1. The socio-economic characteristics of tea farmers
shows the socio-economic characteristics of tea farmers. Farmers were grouped into adopters and non-adopters. Adopters are farmers who adopted at least one of the SATs measured in the study. The non-adopted farmers did not use any of the SATs measured in the study on farms. The overall results show that both adopters and non-adopters were educated. Nevertheless, comparatively, 100% of every population adopter’s farmer had a higher level of education compared with non-adopters. Finally, a relative high margin is seen at the masters’ level between adopters and non-adopters with (4%, n = 11) and (9.5%, n = 11), respectively. Off-farm employment shows (32%, n = 92) and (68%, n = 198) of adopters said ‘Yes’ and ‘NO’ compared to (46%, n = 53) and (54%, n = 62) of non-adopters who said ‘Yes’ and ‘No’, respectively. This implies that most adopted farmers have no off-farm employment. Adopters had slightly higher household numbers than non-adopters. The average years of farming for adopters are 15 years compared to 13 years for non-adopters. Again, off-farm income shows a significant difference between adopters and non-adopters with 11,827.59 and 32, 779.13 RMB, respectively. Moreover, the farm sizes of adopters and non-adopters show significant differences with 4.2 and 4.5 acres, respectively. Again (88%, n = 254) of adopters belong to farmer cooperatives compared with (80%, n = 92) of non-adopters. Finally, the average annual income of adopters is slightly higher than non-adopters with 36,551.03 and 36,346.09 RMB, respectively.
Table 2. Socio-economic characteristics of tea farmers.
4.2. The perception of SATs adopters’ farmers
shows the ranking of smallholder farmers’ SATs’ adoption perception. The results indicate that most farmers ranked ‘SATs’ adoption leads to improved yields or output’ as top in agreement, followed by ‘SATs’ adoption can improve soil cover and fix nitrogen’. The least ranked variables were ‘SATs’ adoption can lead to improved soil nutrients’ and ‘SATs’ adoption prevents bush burning’. Interestingly, two variables ‘SATs’ adoption leads to water conservation’ and ‘SATs’ adoption improves food security that was ranked the same as the third position on the list. This implies that farmers have mixed perceptions of SATs and this might have impacted farmers’ sustainable practices.
4.3. The determinants of SATs adoption
shows farmers’ SATs’ adoption determinants. The Pseudo R2 of (0.0475) shows a significant level and normally distributed data. The individual results show that age has a positive and significant impact on adoption decisions. The impact of education on SATs adoption was not fixed but different impacts at various levels. Given that, farmers who received upper secondary, undergraduate and master’s levels of education were positively impacted by the adoption decision. While farmers, who had lower secondary and college diplomas, were reluctant in their adoption decisions. Again, farm size had a negative impact on adoption with a coefficient of −0.064. However, the income levels of farmers positively impacted adoption even though it was not significant with a coefficient of 0.004. Interestingly, family members, who are extension officers, positively influenced farmers’ adoption decisions. On the contrary, frequent visits by extension services had no significant impact on farmers’ adoption decisions.
Table 3. Determinants of sustainable agricultural Technology Adoption.
4.4. The impact of the variables on productivity
To demonstrate how efficiently the land is being used to produce tea, the study estimated the factors that impacted productivity (Note: Productivity is measured by dividing the entire amount of tea produced (in kilograms) by the total area of land used for tea growing (in acres)). Although the main goal is to measure productivity, there may be trade-offs that need to be considered. Thus, measuring the impact of other variables was essential. Understanding trade-offs is aided by measuring other variables. Measuring the effects of variables on productivity, in short, offers a more comprehensive and nuanced view that is crucial for sustainable development, well-informed decision-making and the effective adoption of new procedures or technologies. Using the probit model estimates, shows the results of the variables that impacted farmers’ productivity. The prob > chi2 shows a significant level of 0.022. Interestingly, farmer cooperatives had the highest impact on productivity with a coefficient of 0.358, followed by a log of income with a coefficient of 0.077. Extension services, years of farming experience and credit access impacted positively productivity with coefficients of 0.025, 0.02 and 0.012, respectively. Gender, age and farm size , negatively impacted productivity with coefficients of −0.12, −0.009 and −0.042, respectively. This implies that aside from farmer characteristics, external services, such as credit access, cooperative and extension services are important in enhancing farmers’ productivity.
Table 4. Impact of variables on productivity (probit model estimates).
4.5. The average treatment effect using (ATT) estimate
To assess the impact of SAT adoption on smallholder tea farmers’ productivity, the study recorded the productivity before and after SAT adoption. Note: (productivity was calculated based on the amount of tea leaves gathered in each area per unit of the previous year compared to the subsequent year’s productivity after adoption). shows the average treatment effect using (ATT) estimate. The study adopted three matching techniques: nearest neighbour matching, kernel matching and calliper matching to check the robustness of the results. The three matching algorithms were employed to address potential biases and confounding factors when comparing formers of adopters and non-adopters in the organic tea industry. The matching algorithms were used to produce matched groups that are comparable in terms of important factors that could otherwise skew the study's findings. Generally, the three different matching algorithms show consistency in the results. On average, SATs-adopted farmers were 16.6% more productive than farmers who did not adopt. The results also show that the overall T-stat for ‘Nearest neighbour matching’, ‘Kernel matching’ and ‘Calliper matching’ are 8.840, 10.56 and 9.22, respectively, which is significant at 1% and greater than 1.96. According to the extant literature, the T-stat of any of these techniques must be greater than 1.96, suggesting a significant level at 5%. This implies that the adoption of SATS increased smallholder tea farmers’ productivity. This result is consistent with the income levels of adopters in the socio-economic characteristics.
Table 5. Average treatment effect (ATT) estimates.
4.6. The distribution of propensity scores
The study graphically showed the propensity score distribution to evaluate the common characteristics between SATs’ adopters and non-adopters. The distribution graph i.e. depicts a normally distributed relationship between adopters and non-adopters. The undispersed graph supports the assumption that adopted and non-adopted farmers have similar characteristics.
4.7. The indices of matching quality
The study checked for matching quality. According to the literature, to ensure matching quality the Ps R2 should be significant. The LR chi2 is expected to reduce significantly after matching. Furthermore, the p > chi2 is supposed to be insignificant as presented in the table with 0.205 and 0.968 for Unmatched and Matched, respectively. Finally, the Mean Bias and Med Bias are expected to reduce. shows that Ps R2 between Unmatched and Matched is 0.023 and 0.003, respectively which falls at a 1% significant level. The result also shows LR chi2 of 10.94 and 2.36 for Unmatched and Matched, respectively. From the table, the Mean Bias reduced from 8.5 to 4.1 and Med Bias reduced from 6.3 to 3.8 for Unmatched and Matched, respectively. This result indicates a good match. The results also suggest that the matching of adopters and non-adopters had a robust result. Hence, the study relies on the result and attributes the increase in productivity to the adoption of SATs.
Table 6. Indices of the matching quality.
5. Discussion
Sustainable agriculture is a growing topic for agricultural stakeholders due to the alarming environmental issues and the effects of agricultural activities on human and natural ecosystems. The study discusses the perception, and determinants of SATs adoption, as well as the impact of the adoption on productivity.
5.1. The demographics of respondents
In analysing social science research, the demographics of the respondents exert profound impacts on respondents ‘ behaviour and decisions (Anuhya et al., Citation2022). Gender shows similar trends between adopters and non-adopters. The findings suggest that there is a gender-based difference in the SATs’ adoption and rejection. This finding is backed by the decision-making theory and the utility expectation, which stipulate a clear difference between males and females’ decision-making. The theory states tha, men are quick in decision-making compared to women (Frederick et al., Citation2005). This can be explained using the purchasing behaviour of males and females (Tian et al., Citation2022). For example, men prioritize products that emphasize functionality, practicality and performance. Hence, men will be more inclined to adopt items related to technology such as SAT over females. The finding aligns with (Venkatesh et al., Citation2000) and recently (Bannor et al., Citation2020), who found that female farmers sometimes prefer to follow their male counterparts in making new varieties and technologies’ decisions. A similar result was found in (Teeken et al., Citation2018)’s study where women were not quick in choosing cassava trait preferences in Nigeria but were more willing to do so if their male counterparts chose to do so. Also, the result on the educational level shows that farmers with higher education rejected SAT adoption. This could imply that SATs may not have adequately been tailored to the specific needs, conditions and expectations. For example, rejection may occur if the technology does not align with their expectations and goals, or if they perceive SAT as incompatible with their knowledge, skills and expectations. The result is, however, contrary to (Anang et al., Citation2020)’s study which found education to be significant in the adoption of modern agricultural technology in Ghana. This difference could be due to differences in socio-economic status in both countries. Marital status significantly impacted farmers’ adoption decisions. This could be possible in group decision-making. For instance, couples compared with singles will take more time to make decisions This finding supports the fact that individual decision-making is sometimes faster than group decision-making (Chavas & Nauges, Citation2020). The off-farm employment shows a significantly positive difference between adopters and non-adopters. Most adopters do not have off-farm employment and thus may be willing to adopt SATs with the expectation of increasing productivity. It is possible that these farmers’ principal source of revenue comes from their agricultural endeavours. Their SATs adoption may have been spurred by a desire to increase the output and profitability of farming, which directly impacts their capacity for livelihood, as found in (Anuhya et al., Citation2022).
There is a negatively significant relationship between adopters and non-adopters in farmer cooperatives. This suggests that cooperatives do not currently have much influence on farmers’ adoption decisions. This can be due to power balance and the resource endowment of cooperative societies. There are also instances in which cooperatives may work against farmers’ efforts to practice sustainability. For instance, cooperatives that do not adequately train and educate members on sustainable farming practices may unintentionally promote unsustainable practices or deter members from implementing novel, and eco-friendly practices. It may be difficult for members of smaller cooperatives to switch to more environment-friendly practices since they lack the infrastructure, support and training required.
The awareness of SATs did not have an impact on the decisions of adopters and non-adopters. This implies that there is a significant distinction among farmers’ awareness, knowledge level and practices or adoption of SAT. This is evident in Rogers (Citation1995) ’s innovation processes where he purported that innovation knowledge does not necessarily result in innovation adoption even though innovation knowledge and innovation adoption share the same individual characteristics. This suggests that more education in the form of information availability, and transformative education is needed to sell the concept and necessity for SATs adoption to farmers. This is relevant as the theory of selective exposure argues that individuals with a ‘need’ for innovation actively search for the knowledge that can solve their problem (LI et al., Citation2017; Rogers, Citation1995).
Age was positive but not significant in the SAT adoption decision. This could be due to no significant difference found between adopters and non-adopters. The average age for adopters and non-adopters is 48 years. This implies that most farmers are within the youthful age but tilted towards old age. The age range may have influenced their SAT’s adoption decision. This result is, however, contrary to Kumar et al. (Citation2018) who found that age is significant to technology adoption decisions. The differences in the studies might be associated with issues like household size, labour availability, etc. For instance, the household size of adopters was slightly higher than non-adopters and showed a positive significant level towards adoption. This suggests that families with large family sizes adopted SAT over farmers with smaller family sizes. This could be because of the need to meet family demand as large family size comes with more socio-economic responsibilities, as found in (Pattnaik et al., Citation2020). The annual income of adopters was slightly higher than non-adopters. This implies that the income of SAT-adopted farmers changed relatively over the period. This may be due to many factors such as the adoption of inputs, farming experience and skill to make the SAT efficient. The finding is similar to (Chuang & Wang, Citation2020) findings for smart agricultural technology adoption in Taiwan. In addition, farm size shows no significance between adopters and non-adopters. The average farm size of adopters is 4.2 acres compared with 4.58 acres for non-adopters. This implies that farmers with relatively small land adopted the SAT to ensure production efficiency and increase productivity. The farming experience of adopters is on average 15 years compared to 13 years of non-adopters. Again, the off-farm income of adopters is relatively low compared with non-adopters and shows a positive significant level in the adoption process. This implies that farmers who adopted SATs have no off-farm employment.
5.2. Perception of farmers on SATs’ adoption
Perception can be described as how something is understood, regarded or interpreted (Chuang & Wang, Citation2020). According to the study results, farmers exhibited mixed perceptions of SATs. This suggests evidence of uncertainties surrounding the outcome of the SAT’s adoption decision. The variation in perceptions also implies a lack of information about the SAT such as the perceived use, cost and efficiency of SAT (Mottaleb, Citation2018). This could be attributed to previous experience with the SAT. As perceptions form attitudes, farmers’ mixed perceptions are reflected in adoption decisions (Li et al., Citation2020). The finding relates to Weersink and Fulton (Citation2020) who found that some farmers may show reluctance to adopt a technology when they supposedly think it will increase their workload. This study also aligns with Cullen and White (Citation2013) who demonstrated that among the several types of sustainable innovations proposed, wine producers in New Zealand mostly chose what they consider (perceived) to be cheapest and riskless to limit any potential economic loss. The findings relate to Locke’s theory of perception which stipulates that perception is more of a theory of knowledge, in that experience is the true source as opposed to the reason (Abdusalam et al., Citation2020). It can be said that adequate knowledge and a good perception of the SAT will positively impact adoption and vice versa. Again, the findings suggest that the decision to adopt SAT on farms is complex, even though farmers agreed that SAT has several advantages. There is, therefore, the need for information and education to motivate farmers on SATs’ adoption and clear the uncertainties surrounding SATs’ adoption (Mottaleb, Citation2018). For example, (Bui & Nguyen, Citation2021) argued that farmers need to understand what exactly they stand to gain by adopting new technologies. This can be applied to SATs’ adoption and what possible challenges could occur during this process. Consequently, enhancing farmers’ perception will translate into higher adoption rates. This will work effectively, particularly with farmers who have family members as extension officers. Because, they have built more trust in them and are willing to practice what they tell them.
5.3. Determinant of SATs adoption
From the technology adoption literature, many factors including institutional, technological, individual and socio-economic influence technology adoption. The study analysed the determinants of SATs’ adoption. The results show that age has a positive and significant impact on adoption decisions. This reflects the fact that as farmers grow older, they tend to look out for solutions that will reduce workload and increase productivity. The result depicts a similar outcome to (Dhraief et al., Citation2018) who found that older farmers turn to adopt technologies with the view of helping them reduce their workload. Also, education had different impacts on farmers’ adoption decisions at different levels. Given that, farmers with relatively low education such as upper secondary, and degree adopted SAT compared with farmers with higher education such as master’s and above who did not adopt SATs. This could happen when farmers with higher education have alternative jobs and sources of money and they tend not to commit much to farming relatively low education level is not a sign of absence of education, it can, therefore, be said that education has a positive influence with adoption, but higher education at some point may impede adoption. The results align with those of (Anang et al., Citation2020; Teeken et al., Citation2018) who found that education positively influences farmers’ likelihood to adopt new technologies. This implies that having educated youthful farmers would have positive SATs’ adoption behaviour. The result aligns with (Candemir et al., Citation2021; Dhraief et al., Citation2018; Shaygan & Daim, Citation2019) who all found education to have a positive relationship with adoption.
Moreover, farm size is an important element in the adoption decision. According to the extant literature, farm size has both negative and positive impacts on adoption. In this study, farm size negatively impacted farmers’ decisions. This implies that farmers with relatively small farmland were less likely to adopt SATs. This could be due to farmers’ expectation of the cost of SAT over the piece of land the SAT is used on (Pattnaik et al., Citation2020). This may not be economically viable for some farmers. For example, farmers with a small piece of land and arge family size may not consider the adoption of SATs beneficial since they already have a source of labour (Kumar et al., Citation2018). The result is in line with (Anang et al., Citation2020) who found that farmers with large farm sizes adopted fertilizer for sustainable rural livelihood over farmers with small farmland. Similar to the study results is (Dhraief et al., Citation2018) who found that farm size and land typology influenced farmers’ decision to adopt soil and water conservation practices.
Furthermore, income (log) has a positive influence on farmers’ adoption decisions. Income is important in farmers’ choices because the decision to adopt SAT might be immediately implemented or held back for implementation due to financial difficulties. As seen in the results, the adopters had a slightly higher income than non-adopters. The results align with (Chavas & Nauges, Citation2020) that, farmers with more stable incomes are more likely to adopt SATs compared with farmers who do not have a stable income. However, besides the income of farmers, other factors prompt farmers’ adoption behaviour, including credit access, extension service, etc. Credit access works as an alternative source of income for farmers who do not have a good financial position (Mariyono, Citation2019). It serves as a guarantee for farmers’ SAT adoption (Mottaleb, Citation2018). The findings align with (Li et al., Citation2020) who found that, credit access impacted farmers’ agricultural technology adoption. Furthermore, there is a positive relationship between extension services and adoption decisions. Family members being extension officers have a positive impact on adoption decisions. This is because family members are often more eager to offer farmers individualized support and direction that considers their unique needs and situations. This individualized care can be quite successful in addressing certain issues and promoting adoption. This also suggests a high level of trust and associations from family members which might have influenced farmers’ decisions. This result aligns with (Teeken et al., Citation2018) who indicated a positive relationship between the extension and adoption of improved cassava varieties by farmers in rural Nigeria. Interestingly, contrary to other studies such as (Chavas & Nauges, Citation2020; Teeken et al., Citation2018) visits by extension service has no impact on farmers’ adoption decisions. That is, the ‘rarely’, ‘sometimes’ and ‘often’ visits of extension officers to farmers had no impact on the farmer adoption decisions. This implies that irrespective of how many times farmers were visited by the extension officer, it did not impact positively their adoption decision. This may be possible as sometimes extension officers may provide generic recommendations during their visits that do not address the specific needs, constraints and conditions of individual farmers. Farmers may require personalized guidance and solutions tailored to their unique circumstances. This could also be explained using the theory of constraints. Though farmers might have wanted to do the right thing or practice, they are limited or face several constraints which impact their adoption decisions and practices. This could also be due to farmers already having family members as extension officers so they may have underrated the activities of extension services as farmers were not new to the activities and advice of extension services.
5.4. Impact of SATs’ adoption on productivity
Farmers as rational beings would like to see the positive impact of technology adoption decisions on productivity. The impact is usually measured as the difference between the annual output and productivity after adoption compared to the previous year(s) of non-adoption. The overall results of the study suggest that the SATs’ adoption improved farmers’ productivity by 16.6%. The indices of matching quality suggest that the matching of adopters and non-adopters for SATs’ adoption in tea production had a robust result. Given that, the adopters and non-adopters have similar socio-economic characteristics but different adoption decisions. Additionally, the propensity score distribution graph shows a normal distribution between adopters and non-adopters. Hence, the study can rely on the result and attribute the increase in productivity to SATs’ adoption. The positive impact of SATs’ adoption on farmers’ productivity is also consistent with the annual income of adopters and non-adopters. Adopters received slightly higher revenue than non-adopters. This finding is in line with (Bannor et al., Citation2020) who found the adoption of modern rice varieties impacted the productivity and poverty levels of farmers in India. The results also align with (Dhraief et al., Citation2018) who found that the adoption of innovative technologies by livestock farmers in arid areas of Tunisia impacted positively their productivity.
The study also analysed the factors that impacted the productivity of farmers. The findings show that the farmer cooperative is one of the important variables that impacted positively productivity. Farmer cooperatives serve as opinion leaders in the community and are pivotal in influencing the decisions and activities of farmers. For example, in technology adoption, farmer cooperatives can influence the decision phase, where a technology is adopted or rejected. The cooperative may reject the technology either actively or passively. The active rejection comes when they advise farmers to consider the innovation and take part in trials but later decide not to adopt it. On the other hand, passive rejection comes when cooperation discourages farmers from considering using the technology at all. For instance, cooperatives might be aware of substitute techniques or technologies that are better suited to the unique requirements and circumstances of their members. They might advise avoiding the technology in these situations in favour of better alternatives. The current findings suggest that cooperatives positively impactfarmers’ productivity. This aligns with (Anuhya et al., Citation2022; Candemir et al., Citation2021) findings on the positive impact of cooperatives on farmers’ decisions. Also, access to credit is an important element which impacts positively on farmer productivity. Access to credit serves as motivation to farmers and helps improve productivity. The result is consistent with (Mariyono, Citation2019) who found that, in areas where farmers have access to credit, farmers can take many initiatives including the adoption of new technologies and the use of farming equipment that can improve productivity. Equally, access to extension services impacted positively farmer productivity. This is interesting as the access to extension service did not influence farmers’ adoption decisions but rather impacted positively on productivity. The result is consistent with the prior expectation as extension agents are the primary means by which smallholders receive information on modern production practices and technologies. This could also mean that, although extension services did not influence farmers’ SATs’ adoption, they trust extension services for technical difficulties and problems with farming. Consequently, Rogers (Citation1995) suggested that extension agents need to provide information and technical assistance to help adopters at any stage of the process. The study is in line with (Anang et al., Citation2020) who found that extension office activities increased farmer income.
The theoretical implication of the study shows that SATs’ adoption is a continuous process as opposed to a one-time occurrence. This is in line with theories like the innovation diffusion theory and the technology adoption model (TAM), which highlight that adoption is a series of actions impacted by different factors over time. Also, the theory of constraints is brought up to clarify that even though farmers may want to implement appropriate technologies, several barriers (such as lack of education, credit availability and confidence in extension services) may affect their choices. This viewpoint highlights the difficulties and constraints farmers encounter when implementing new technologies. Practically, the study shows that, in contrast to some farmers’ unfavourable perceptions, SATs had a positive effect on productivity. This difference between perceived and actual impact is consistent with theories (such as TAM) about perceived usefulness versus actual innovation performance, indicating that perceptions do not always match objective results.
As a recommendation, China's organic tea production may advance towards achieving sustainability if it incorporates farmers’ perceptions and actively involves farmers in the development and use of SAT. To do this, cooperation between the government, businesses and farmers is crucial. The study helps in policy and decision-making regarding farmers’ understanding and perception of SATs. It also helps in determining the factors that will promote or influence farmers’ decisions on SATs . Considering farmers’ perception of SATs, it is recommended that, aside from promoting farmers’ formal education, the technological need and the unique values of SATs should be the core values communicated to farmers. The government must help to remove possible and feasible constraints that inhibit the adoption of SATs by providing interventions such as subsidies, credit facilities, technical support and strengthening activities of farmer cooperatives through award schemes to promote SATs adoption. Efforts to improve SATs’ adoption should consider the ties between farmers’ families and farmer-based associations when disseminating technological information to farmers. Government policies on agriculture technologies should include tea growers in the initial development and selection of technologies. Government must ensure technologies are in line with farmers’ requirements and priorities by getting their opinions, preferences and input. Foster communication among tea growers. Farmersm who have successfully incorporated sustainable technologies into their operationsm can educate and inform their colleagues by sharing their experiences and expertise, which fosters credibility. Stakeholders must tailor sustainable technologies to suit local conditions, including pests, soil types and climates. Ensure that SATs are adaptable and can be customized to meet the unique needs of different tea-growing regions in China.
6. Conclusion
The study investigated how the integration of farmers’ perceptions towards SATs’ adoption could help in the development of sustainable tea production in China. Since technology adoption is not a one-time event but rather a process which lasts over time and involves a series of actions that flow in a sequence, the study assessed farmers’ socio-economic characteristics, their perception and determinants of adoption, as well as the impact of SAT on productivity. The study concludes that the effectiveness of SAT adoption is influenced by various variables, including farmer education, age, family member as an extension officer and farmers’ income. Realizing the advantage of increased roductivity through SAT adoption, it is conclusive that ensuring long-term sustainable tea production in China depends heavily on encouraging and assisting farmers to adopt SATs through education, financial incentives and technical support.
Specifically, farmers’ demographics such as marital status, off-farm employment, farmer cooperatives, household size, farming experience and off-farm income play important roles in the SAT adoption decisions. In a similar manner, farmers’ productivity is impacted by variables such as age, credit access and family members as an extension officer. Interestingly, the frequency of visits by extension services had no impact on the adoption of SAT but positively impacted armers’ productivity. This is explained by the theory of constraints which suggests that although farmers may have the desire or willingness to do the right thing (adopt) the right technology, they may have constraints that impact their decisions. Farmers’ attitudes and behaviour towards visits by extension services could also be described as distrust in extension services since farmers acted upon family extension officers’ advice over unknown extension officers.
External services including credit access, cooperative and extension services are essential factors facilitating the increase in farmers’ productivity. However, education and farmer cooperatives can at times be impeding factors hindering adoption decisions. This is important when the expectations of highly educated farmers are not met, or cooperative activities and goals do not foster sustainable practices. Also, participating in cooperatives shows how farmers appreciate social networks and influence their decisions and activities. Since social networks are complex webs of human relationships, interactions and connections; considering their significance in the context of tea farmers and sustainable agriculture practices can offer insightful influence and a more complete understanding of the factors influencing technology adoption. For example, farmers often trust the opinions and experiences of their peers more than external sources. Thus, social networks can facilitate peer learning and allow farmers to learn from others’ successes and challenges in adopting sustainable practices. Also, influential people within a social network may have the power to influence the views and actions of farmers. This power can have a domino effect, pushing farmers to use the SAT. It is conclusive that farmer’s social networks such as cooperatives impact farmers’ decisions and practices. In real terms, the average treatment effects show that SATs’ adoption had a significant and positive impact on productivity despite a few farmers’ negative perceptions about SATs.
Disclosure statement
No potential conflict of interest was reported by the author(s).
Correction Statement
This article has been corrected with minor changes. These changes do not impact the academic content of the article.
Additional information
Funding
References
- Abdusalam, A., Zhang, Y., Abudoushalamu, M., Maitusun, P., Whitney, C., Yang, X.-f., & Fu, Y. (2020). Documenting the heritage along the silk road: An ethnobotanical study of medicinal teas used in Southern Xinjiang, China. Journal of Ethnopharmacology, 260, Article 113012. https://doi.org/10.1016/j.jep.2020.113012
- Abideen, A. Z., Sundram, V. P. K., Pyeman, J., & Othman, A. K. (2021). Food supply chain transformation through technology and future research directions—A systematic review. Logistics, 5(4), Article 83. https://doi.org/10.3390/logistics5040083
- Adesina, A. A., & Baidu-Forson, J. (1995). Farmers’ perceptions and adoption of new agricultural technology: Evidence from analysis in Burkina Faso and Guinea, West Africa. Agricultural Economics, 13(1), 1–9. https://doi.org/10.1111/j.1574-0862.1995.tb00366.x
- Ali, A., & Abdulai, A. (2010). The adoption of genetically modified cotton and poverty reduction in Pakistan. Journal of Agricultural Economics, 61(1), 175–192. https://doi.org/10.1111/j.1477-9552.2009.00227.x
- Anang, B. T. (2018). Farm technology adoption By smallholder farmers in Ghana. Review of Agricultural and Applied Economics, 21(2), 41–47. https://doi.org/10.15414/raae.2018.21.02.41-47
- Anang, B. T., Bäckman, S., & Sipiläinen, T. (2020). Adoption and income effects of agricultural extension in northern Ghana. Scientific African, 7, Article e00219. https://doi.org/10.1016/j.sciaf.2019.e00219
- Anuhya, P., Kisku, U., & Khare, N. K. (2022). A study on correlates of profile characteristics and adoption behaviour of Rythu Bharosa Kendra (RBK) beneficiaries in Anantapur District, Andhra Pradesh. Current Journal of Applied Science and Technology, 41(24), 39–45. https://doi.org/10.9734/cjast/2022/v41i2431768
- Arellanes, P., & Lee, D. R. (2003, August 16–22). The determinants of adoption of sustainable agriculture technologies: Evidence from the hillsides of Honduras. Proceedings of the 25th international conference of agricultural economists, Durban, South Africa (pp. 693–699).
- Arhin, I., Li, J., Mei, H., Amoah, M., Chen, X., Jeyaraj, A., Li, X., & Liu, A. (2022). Looking into the future of organic tea production and sustainable farming: A systematic review. International Journal of Agricultural Sustainability, 20(5), 942–954. https://doi.org/10.1080/14735903.2022.2028398
- Arhin, I., Mei, H., Li, J., Gyamfi, E., Antwi-Boasiako, A., Chen, X., Li, X., & Liu, A. (2023). Analysis of the determinants of sustainable agricultural technologies adoption in tea production in China: A systematic review. International Journal of Agricultural Sustainability, 21(1), Article 2239047. https://doi.org/10.1080/14735903.2023.2239047
- Asiedu-Ayeh, L. O., Zheng, X., Agbodah, K., Dogbe, B. S., & Darko, A. P. (2022). Promoting the adoption of agricultural green production technologies for sustainable farming: A multi-attribute decision analysis. Sustainability, 14(16), Article 9977. https://doi.org/10.3390/su14169977
- Asiedu-Darko, E. (2014). Farmers’ perception on agricultural technologies a case of some improved crop varieties in Ghana. Agriculture, Forestry and Fisheries, 3(1), Article 13. https://doi.org/10.11648/j.aff.20140301.13
- Atieno, M., Herrmann, L., Nguyen, H. T., Phan, H. T., Nguyen, N. K., Srean, P., Than, M. M., Zhiyong, R., Tittabutr, P., Shutsrirung, A., Bräu, L., & Lesueur, D. (2020). Assessment of biofertilizer use for sustainable agriculture in the great Mekong region. Journal of Environmental Management, 275, Article 111300. https://doi.org/10.1016/j.jenvman.2020.111300
- Azman, A., D Silva, J. L., Samah, B. A., Man, N., & Shaffril, H. A. M. (2013). Relationship between attitude, knowledge, and support towards the acceptance of sustainable agriculture among contract farmers in Malaysia. Asian Social Science, 9(2), 99–105. https://doi.org/10.5539/ass.v9n2p99
- Bannor, R. K., Kumar, G. A. K., Oppong-Kyeremeh, H., & Wongnaa, C. A. (2020). Adoption and impact of modern rice varieties on poverty in eastern India. Rice Science, 27(1), 56–66. https://doi.org/10.1016/j.rsci.2019.12.006
- Baumgart-Getz, A., Prokopy, L. S., & Floress, K. (2012). Why farmers adopt best management practice in the United States: A meta-analysis of the adoption literature. Journal of Environmental Management, 96(1), 17–25. https://doi.org/10.1016/j.jenvman.2011.10.006
- Biggs, E. M., Gupta, N., Saikia, S. D., & Duncan, J. M. A. (2018). Tea production characteristics of tea growers (plantations and smallholdings) and livelihood dimensions of tea workers in assam, India. Data in Brief, 17, 1379–1387. https://doi.org/10.1016/j.dib.2018.02.056
- Bu, X., Nguyen, H. V., Chou, T. P., & Chen, C.-P. (2020). A comprehensive model of consumers’ perceptions, attitudes and behavioral intention toward organic tea: Evidence from an emerging economy. Sustainability, 12(16), Article 6619. https://doi.org/10.3390/su12166619
- Bui, H. T. M., & Nguyen, H. T. T. (2021). Factors influencing farmers’ decision to convert to organic tea cultivation in the mountainous areas of northern Vietnam. Organic Agriculture, 11(1), 51–61. https://doi.org/10.1007/s13165-020-00322-2
- Calicioglu, O., Flammini A., Bracco S., Bellù L., & Sims R. (2019). The future challenges of food and agriculture: An integrated analysis of trends and solutions. Sustainability, 11(1), Article 222. https://doi.org/10.3390/su11010222
- Candemir, A., Duvaleix, S., & Latruffe, L. (2021). Agricultural cooperatives and farm sustainability – a literature review. Journal of Economic Surveys, 35(4), 1118–1144. https://doi.org/10.1111/joes.12417
- Chang, S. E. (2013). Blue magpie TEAgriculture: Eco-tea cultivation and participatory farming in pinglin satoyama, Taiwan. Procedia - Social and Behavioral Sciences, 101, 14–22. https://doi.org/10.1016/j.sbspro.2013.07.174
- Chavas, J. P., & Nauges, C. (2020). Uncertainty, learning, and technology adoption in agriculture. Applied Economic Perspectives and Policy, 42(1), 42–53. https://doi.org/10.1002/aepp.13003
- Chuang, J., & Wang, J. (2020). Farmers’ knowledge, attitude, and adoption of smart agriculture technology in Taiwan. International Journal of Environmental Research and Public Health, 17, 7236–7238. https://doi.org/10.3390/ijerph17197236
- Cullen, R., & White, P. C. (2013). Prioritising and evaluating biodiversity projects. Wildlife Research, 40(2), 91–93.
- de Mello Brandão Vinholis, M., Macchione Saes M. S., Carrer M. J., & Meirelles de Souza Filho H. (2021). The effect of meso-institutions on adoption of sustainable agricultural technology: A case study of the Brazilian low carbon agriculture plan. Journal of Cleaner Production, 280, Article 124334. https://doi.org/10.1016/j.jclepro.2020.124334
- Dhraief, M. Z., Bedhiaf-Romdhania, S., Dhehibib, B., Oueslati-Zlaouia, M., Jebali, O., & Ben Youssef, S (2018). Factors affecting the adoption of innovative technologies by livestock farmers in arid area of Tunisia. FARA Research Report, 3(5), 23. https://doi.org/10.13140/RG.2.2.15795.27686
- Dong, H., & Liang, Q. (2023). Agro-food quality certification, agricultural organizations, and farm performance: Evidence from vegetable farmers in China. International Journal of Agricultural Sustainability, 21(1), Article 2205772. https://doi.org/10.1080/14735903.2023.2205772
- Dunn, L., Latty T., Van Ogtrop F. F., & Tan D. K. Y. (2023). Cambodian rice farmers’ knowledge, attitudes, and practices (KAPs) regarding insect pest management and pesticide use. International Journal of Agricultural Sustainability, 21(1), Article 2178804. https://doi.org/10.1080/14735903.2023.2178804
- Frederick, D. A., Fessler, D. M., & Haselton, M. G. (2005). Do representations of male muscularity differ in men's and women's magazines? Body Image, 2(1), 81–86.
- Gaitán-Cremaschi, D., Klerkx, L., Duncan, J., Trienekens, J. H., Huenchuleo, C., Dogliotti, S., Contesse, M. E., Benitez-Altuna, F. J., & Rossing, W. A. H. (2020). Sustainability transition pathways through ecological intensification: An assessment of vegetable food systems in Chile. International Journal of Agricultural Sustainability, 18(2), 131–150. https://doi.org/10.1080/14735903.2020.1722561
- Gerasimova, K., Sheng, J., & Zhao, J. (2021). COVID-19 and other challenges: A case study of certified organic green tea producers in China. Critical Sociology, 47(4–5), 591–607. https://doi.org/10.1177/0896920520975843
- Gołaś, M., Sulewski P., Wąs A., Kłoczko-Gajewska A., & Pogodzińska K. (2020). On the way to sustainable agriculture—eco-efficiency of Polish commercial farms. Agriculture, 10(10), 438–424. https://doi.org/10.3390/agriculture10100438
- Hu, H., Cao A., Chen S., & Li H. (2022). Effects of risk perception of pests and diseases on tea famers’ green control techniques adoption. International Journal of Environmental Research and Public Health, 19(14), Article 8465. https://doi.org/10.3390/ijerph19148465
- Koner, N., & Laha, A. (2021). Economics of alternative models of organic farming: Empirical evidences from zero budget natural farming and scientific organic farming in West Bengal, India. International Journal of Agricultural Sustainability, 19(3–4), 255–268. https://doi.org/10.1080/14735903.2021.1905346
- Kothari, C. R. (2004). Research methodology: Methods and techniques. New Age International.
- Kumar, G., Engle, C., & Tucker, C. (2018). Factors driving aquaculture technology adoption. Journal of the World Aquaculture Society, 49(3), 447–476. https://doi.org/10.1111/jwas.12514
- Lampach, N., Nguyen-Van, P., & To-The, N. (2017, September 15). Robustness analysis of organic technology adoption: Evidence from northern Vietnamese tea production. http://doi.org/10.2139/ssrn.3037389
- Li, H. Q., Zheng, F., & Zhao, Y. Y. (2017). Farmer behavior and perceptions to alternative scenarios in a highly intensive agricultural region, south central China. Journal of Integrative Agriculture, 16(8), 1852–1864. https://doi.org/10.1016/S2095-3119(16)61547-2
- Li, J., Feng, S., Luo, T., & Guan, Z. (2020). What drives the adoption of sustainable production technology? Evidence from the large scale farming sector in East China. Journal of Cleaner Production, 257, Article 120611. https://doi.org/10.1016/j.jclepro.2020.120611
- Liu, J., Zhang, C., Hu, R., Zhu, X., & Cai, J. (2019). Aging of agricultural labor force and technical efficiency in tea production: Evidence from Meitan county, China. Sustainability, 11(22), 6246–6216. https://doi.org/10.3390/su11226246
- Loos, J., & Wehrden, H. V. (2018). Beyond biodiversity conservation: Land sharing constitutes sustainable agriculture in European cultural landscapes. Sustainability, 10(5), Article 1395. https://doi.org/10.3390/su10051395
- López-Felices, B., Aznar-Sánchez, J. A., Velasco-Muñoz, J. F., & Mesa-Vázquez, E. (2023). Farmers’ profiles and attitudes towards the implementation of rainwater harvesting systems in intensive agriculture. International Journal of Agricultural Sustainability, 21(1), Article 2189402. https://doi.org/10.1080/14735903.2023.2189402
- Lu, H., & Xie, H. (2018). Impact of changes in labor resources and transfers of land use rights on agricultural non-point source pollution in Jiangsu province, China. Journal of Environmental Management, 207, 134–140. https://doi.org/10.1016/j.jenvman.2017.11.033
- Mahama, A., Awuni, J. A., Mabe, F. N., & Azumah, S. B. (2020). Modelling adoption intensity of improved soybean production technologies in Ghana - A generalized poisson approach. Heliyon, 6(3), Article e03543. https://doi.org/10.1016/j.heliyon.2020.e03543
- Mariyono, J. (2019). Microcredit and technology adoption: Sustained pathways to improve farmers’ prosperity in Indonesia. Agricultural Finance Review, 79(1), 85–106. https://doi.org/10.1108/AFR-05-2017-0033
- Mottaleb, K. A. (2018). Perception and adoption of a new agricultural technology: Evidence from a developing country. Technology in Society, 55(July), 126–135. https://doi.org/10.1016/j.techsoc.2018.07.007
- Munasinghe, M., Deraniyagala, Y., Dassanayake, N., & Karunarathna, H. (2017). Economic, social and environmental impacts and overall sustainability of the tea sector in Sri Lanka. Sustainable Production and Consumption, 12(July), 155–169. https://doi.org/10.1016/j.spc.2017.07.003
- Pattnaik, I. P., Jena, S. J., & Kanungo, A. P. (2020). Assessment of factors affecting adoption of potato production technology in odisha. Journal of Pharmacognosy and Phytochemistry, 9(5), 2886–2889.
- Pello, K., Okinda, C., Liu, A., & Njagi, T. (2021). Factors affecting adaptation to climate change through agroforestry in Kenya. Land, 10(4), 371.
- Qiao, Y., Halberg, N., Vaheesan, S., & Scott, S. (2016). Assessing the social and economic benefits of organic and fair trade tea production for small-scale farmers in Asia: A comparative case study of China and Sri Lanka. Renewable Agriculture and Food Systems, 31(3), 246–257. https://doi.org/10.1017/S1742170515000162
- Rogers, E. M. (1995). Diffusion of Innovations: Modifications of a model for telecommunications. In Die diffusion von innovationen in der telekommunikation (pp. 25–38). Springer.
- Sarkar, D., Kar, S. K., Chattopadhyay, A., Rakshit, A., Tripathi, V. K., Dubey, P. K., & Abhilash, P. C. (2020). Low input sustainable agriculture: A viable climate-smart option for boosting food production in a warming world. Ecological Indicators, 115(April), Article 106412. https://doi.org/10.1016/j.ecolind.2020.106412
- Shaygan, A., & Daim, T. (2019, August). Technology management maturity assessment model: An exploratory multi-criteria approach for healthcare organizations. In 2019 Portland International Conference on Management of Engineering and Technology (PICMET) (pp. 1–9). IEEE.
- Silva, D. S., Arima, E. Y., dos Reis, T. N. P., & Rattis, L. (2023). Temperature effect on Brazilian soybean yields, and farmers’ responses. International Journal of Agricultural Sustainability, 21(1), Article 2173370. https://doi.org/10.1080/14735903.2023.2173370
- Sitthisuntikul, K., Yossuck, P., & Limnirankul, B. (2018). How does organic agriculture contribute to food security of small land holders?: A case study in the north of Thailand. Cogent Food & Agriculture, 4(1), Article 1429698. https://doi.org/10.1080/23311932.2018.1429698
- Teeken, B., Olaosebikan, O., Haleegoah, J., Oladejo, E., Madu, T., Bello, A., Parkes, E., Egesi, C., Kulakow, P., Kirscht, H., & Tufan, H. A. (2018). Cassava trait preferences of men and women farmers in Nigeria: Implications for breeding. Economic Botany, 72(3), 263–277. https://doi.org/10.1007/s12231-018-9421-7
- Tey, Y. S., & Brindal, M. (2012). Factors influencing the adoption of precision agricultural technologies: A review for policy implications. Precision Agriculture, 13(6), 713–730. https://doi.org/10.1007/s11119-012-9273-6
- Tian, H., Siddik, A. B., & Masukujjaman, M. (2022). Factors affecting the repurchase intention of organic tea among millennial consumers: An empirical study. Behavioral Sciences, 12(2), Article 50. https://doi.org/10.3390/bs12020050
- Ul Haq, S., Boz, I., & Shahbaz, P. (2022). Measuring tea farmers’ perceptions of sustainable agriculture and factors affecting this perception in rize province of Turkey. Journal of Agricultural Science and Technology, 24(5), 1043–1056.
- Vabi Vamuloh, V., Panwar, R., Hagerman, S. M., Gaston, C., & Kozak, R. A. (2019). Achieving sustainable development goals in the global food sector: A systematic literature review to examine small farmers engagement in contract farming. Business Strategy & Development, 2(4), 276–289. https://doi.org/10.1002/bsd2.60
- van Hulst, F., Ellis R., Prager K., & Msika J. (2020). Using co-constructed mental models to understand stakeholder perspectives on agro-ecology. International Journal of Agricultural Sustainability, 18(2), 172–195. https://doi.org/10.1080/14735903.2020.1743553
- Venkatesh, V., Morris, M. G., & Ackerman, P. L. (2000). A longitudinal field investigation of gender differences in individual technology adoption decision-making processes. Organizational Behavior and Human Decision Processes, 83(1), 33–60. https://doi.org/10.1006/obhd.2000.2896
- Viatte, G. (2001). Adopting technologies for sustainable farming systems: An OECD perspective. In Adoption of technologies for sustainable farming systems Wageningen workshop proceedings (Vol. 14, pp. 14–23). OECD.
- Wang, N., Gao Y., Wang Y., & Li X. (2016). Adoption of eco-friendly soil-management practices by smallholder farmers in shandong province of China. Soil Science and Plant Nutrition, 62(2), 185–193. https://doi.org/10.1080/00380768.2016.1149779
- Wang, X., Ma Y., Li H., & Xue C. (2022). How does risk management improve farmers’ green production level? Organic fertilizer as an example. Frontiers in Environmental Science, 10(1), 1–11. https://doi.org/10.3389/fenvs.2022.946855
- Weersink, A., & Fulton, M. (2020). Limits to profit maximization as a guide to behavior change. Applied Economic Perspectives and Policy, 42(1), 67–79.
- Williamson, S. (2002). Challenges for farmer participation in integrated and organic production of agricultural tree crops. BiocontrolNews and Information, 23(1), 25–36.
- Zheng, R. Zhan J., Liu L., Ma Y., Wang Z., Xie L., & He D. (2019) Factors and minimal subsidy associated with tea farmers’ willingness to adopt ecological pest management, Sustainability, 11(22), Article 6190. https://doi.org/10.3390/su11226190.