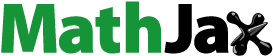
Abstract
From works in in-situ seepage through dams and laboratory experiments using Layered Heterogeneous Porous Media (LHPM), it has been noted that a refraction-like phenomenon, such as that experienced in light, affects fluids’ flowlines when crossing the contact interface of layers characterised by different porosity viz-a-viz permeability. This concept has many applications in fluid dynamics, such as the dispersion process in stratified media. Currently, no study exists that models and analyses the relationship between the porosities of two layers in contact and the resulting flowline refraction using validated LHPM data. Hence, this work aims to establish a relationship between the porosity ratio of stratified media made up of two layers and the refraction angle
of the maximum volume flux
. Volume flux data from the flow of a single-phase fluid through five LHPMs with
ranging from 0.8325 to 0.9524 were used in the modelling. The flow was oriented from the lower to the higher porosity vis-à-vis permeability layer. It was found that
which is also the refraction angle of the peak solute plume flux, refracts away from the normal as
reduces. This indicates an increase in the dilution rate vis-à-vis spread of plumes with the reduction in homogeneity between the two layers. Also,
does not correlate with the stratification inclination
, but the
which is also the peak solute plume flux correlates with
. Furthermore, an efficient model, which is the best-unbiased estimator, with
was derived. Findings from this work can help better understand solute plume dispersion and the general fluid flow dynamics in stratified media such as capillary barrier effect covers for pollution control and hydrocarbon reservoirs.
1. Introduction
When fluid flow through any medium, rocks and soil inclusive, its flow path and rate are influenced by several petrophysical properties such as tortuosity, pore size distribution, cracks, fissures, porosity, permeability, and inclination, which determine volume flux (q) at every instant, hence making in-situ flow dynamics complex (Wei et al., Citation2021; Alabi & Sedara, Citation2020; H. H. Wang et al., Citation2020; X. Li et al., Citation2020; Sun & Zhang, Citation2020; Govindarajan, Citation2019; Cao et al., Citation2016). Therefore, Intermediate Scale Experiments (ISEs) carried out in the laboratory are used to study the specific properties of interest, e.g., Preferential Flow Features (PFF) and Barrier Flow Features (BFF) and how they affect the flow dynamics (Solnyshkina et al., Citation2021; X. Li et al., Citation2020; Sebben & Werner, Citation2016b; Silliman et al., Citation1998). One of such ISEs is Layered Heterogeneous Porous Media (LHPM), a proxy used to study the effect of stratifications on flow dynamics (Alabi & Akanni, Citation2021, Citation2022a; Ku et al., Citation2021; X. Li et al., Citation2020; Liang et al., Citation2019). LHPMs have found application in geotechnics, CO2 sequestration, enhanced recovery of oil and gas, landfills’ leachate collection systems, and pollution control systems (Alabi & Akanni, Citation2022b; Banihashem & Karrabi, Citation2020; Hosking et al., Citation2018; Hu et al., Citation2020; March et al., Citation2018; Sai et al., Citation2020; Salimzadeh & Khalili, Citation2014; Zhang & Yuan, Citation2019).
From works in in-situ seepage through dams and laboratory experiments using LHPMs, it has been noted that a refraction-like phenomenon, such as that experienced in light, affects fluids’ flowlines when crossing the contact interface of layers characterised by different porosity viz-a-viz permeability (Casagrande, Citation1937; Cedergren, Citation1997; Eagleman & Jamison, Citation1962). However, unlike Snell’s law which is a sine law, groundwater refraction obeys tangential law (Sebben & Werner, Citation2016a).
Figure illustrates the refraction schematic diagram for an LHPM of increasing layer hydraulic conductivity (K) in the flow direction. The flow originates from the top layer (L1) of , into the bottom layer (L2) of
, where
. This flow regime is also known as Ascending Flow (AF) system. In such a system, the flowline refracts from the normal in L2 (Alabi & Akanni, Citation2022a). The observed refraction is due to the increased fluid velocity in L2 as the K increases (Pavlovskaya et al., Citation2018; Sebben & Werner, Citation2016b). It results in dispersion towards a higher refraction angle from the normal because the fluid flow field shows the instantaneous and free stream velocity (Ferdows et al., Citation2022; Sebben & Werner, Citation2016b). Figure illustrates the reverse flow, i.e. flow from *L1 into *L2, where
. This flow system is also known as Descending Flow (DF). In the DF system, the flowline refracts towards the normal in *L2 (Alabi & Akanni, Citation2022a). Since this study focuses on the AF system, more focus would be placed on it.
Figure 1. a) Flownet showing groundwater refraction in a heterogeneous medium. Areas with the same K have equal-sized “squares.” b) A simplified flownet refraction in an AF system (heterogeneous isotropic medium). The flow is from L1 into L2. It is characterised by two flowlines (yellow dash lines), two different equipotential lines (red dash lines), as porosity for L1,
as hydraulic conductivity for L1,
as porosity for L2, and
as hydraulic conductivity for L2 (Adapted from Bos, Citation2006; Freeze & Cherry, Citation1979; Leliavsky, Citation1955).

Figure 2. Refraction in a DF system (heterogeneous isotropic medium). The flow is from *L1 into *L2. It is characterised by two flowlines (yellow dash lines), two different equipotential lines (red dash lines), as porosity for L1,
as hydraulic conductivity for *L1,
as porosity for *L2, and
as hydraulic conductivity for L2 (Adapted from Bos, Citation2006; Freeze & Cherry, Citation1979; Leliavsky, Citation1955).

From Figure , the tangential law of groundwater refraction can be derived by first considering the mass continuity equation. It states that the discharge in both layers must be equal irrespective of the K value of each layer (Bos, Citation2006; Y. Li et al., Citation2022). Therefore,
where is the volumetric discharge that flows between the flowlines (F1 and F2),
is the hydraulic gradient of L1, is the hydraulic conductivity of L1,
is the unit area between two fluid particles following two different flowlines (F1 and F2),
is the entry angle,
is the hydraulic gradient of L2,
is the hydraulic conductivity of L2, and
is the refraction angle.
The i for the two layers can be expressed as (Bos, Citation2006),
where is equipotential line 1,
is equipotential line 2, and all other notations retain their meaning.
Substituting EquationEqn. 2(2)
(2) into EquationEqn. 1
(1)
(1) results in
Solving EquationEqn. 3(3)
(3) , using trigonometry results in the tangential law for groundwater flow (Bos, Citation2006; Lu et al., Citation2013);
where all notations retain their meaning.
Furthermore, recent studies have explored the flow dynamics associated with the contact interface in stratifications. Dejam and Hassanzadeh (Citation2022), using analytical and numerical methods, reported the presence of tensorial dispersion and advection at the contact interfaces of LHPMs characterised by different Darcy numbers . They reported that such dispersion tensor and advection become symmetric once hydraulic parameters like porosity, thickness, and molecular diffusion become equal, i.e. when the LHPM becomes isotropic and homogeneous. Using numerical analysis, Sebben and Werner (Citation2016b) found that as the L2 width increases, dispersion also increases in AF systems. Kurasawa et al. (Citation2020) reported that for a similarly scaled heterogeneity in LHPMs and randomly porous media, the longitudinal dispersivity of the former would always be more significant. This dispersivity could be enhanced nonmonotonically with the aid of velocity fluctuations as a stochastic spike phenomenon, where the statistically obtainable maximum dispersion is at a correlation length
, where y is the total layer thickness (Xu & Tartakovsky, Citation2017). Chen et al. (Citation2022), from ISEs and numerical models, reported the presence of directionally dependent asymmetric Breakthrough Curves (BTCs) in LHPMs with sharp and transition interfaces. The transition interfaces were reported to be associated with more pronounced BTCs, due to more accumulation at such interfaces. These transition interfaces are known to occur naturally or from man-made activities like contamination from nanoparticle-enhanced drilling fluids (L. Bég et al., Citation2018; L. Wang et al., Citation2022). Chen et al. (Citation2022) further reported the Mobile and Immobile (MIM) model as being optimally better than the Advection-Dispersion Equation (ADE) model in capturing the directionally dependent transport of solutes. Y. Li et al. (Citation2022) extended the works of (Sebben & Werner, Citation2016a) and (Sebben & Werner, Citation2016b) to non-Darcian dispersion of solute plumes in the PFF (faults and fractures) using the finite-element model COMSOL Multiphysics. Such dispersion occurs due to variable porosity and turbulent flow (Usman, Shaheen et al., Citation2022). Y. Li et al. (Citation2022) reported a 160% increase in solute concentration compared to Darcian models due to the non-Darcian dispersion, resulting from a reduction in interface refraction and specific discharge. This reduced refraction implies that EquationEqn. 4
(4)
(4) is invalid in non-Darcian conditions. Y. Li et al. (Citation2022) also found that non-Darcian effects are generally negligible in systems where PFFs are filled with porous media, except in rare situations.
All the earlier reviewed works of literature used numerical models to visualise the interface’s refraction and associated solute plume dispersion. For instance, Chen et al. (Citation2022) made use of the Galerkin finite-element software package COMSOL Multiphysics (COMSOL Inc., Burlington, MA, USA), while Dejam and Hassanzadeh (Citation2022) made use of GBAND solver for the resultant fully implicit Finite Difference (FD) discretised equation. Limited literature has reported observations from ISEs, designed and constructed to prove EquationEqn. 4(4)
(4) (the tangential groundwater law of refraction) based on the experimental results alone. Of the limited literature, we intend to focus on Popoola et al. Citation2010a, Popoola et al. Citation2010b and Popoola et al. Citation2009, whose full ISE results we have been granted access. The authors reported that the refraction angle
of the flowline is associated with the peak volume flux (
, denoted by
deflects from the normal for a single-phase fluid flowing from a lower to higher porous vis-à-vis permeable layer (AF system). The reported result aligns with Sebben and Werner (Citation2016a), and it validates the ISE setup as being in line with EquationEqn. 4
(4)
(4) . Figure illustrates the reported flow dynamics, where
.
More recent works have also been done using the ISE’s data (data obtained from Popoola et al., Citation2010a, Popoola et al., Citation2010b and Popoola et al., Citation2009). These subsequent works have further validated the ISE setup and its data. The works show that other flow dynamics deductions from the ISE’s data align with the findings of different numerical studies on AF systems. Using part of the data from the ISE setup as input parameters for 3D numerical analysis, Alabi and Akanni (Citation2021) reported AF systems’ interfaces as points of flow convergence. The reported result agrees with other authors like Ng et al. (Citation2015) and Aubertin et al. (Citation2009), whose studies were based on different data sources for AF system. Alabi and Akanni (Citation2021) further reported the suitability of the LHPM arrangement as capillary barrier effect (CBE) covers for groundwater protection, which aligns with the result of Huo et al. (Citation2022). Furthermore, Alabi & Akanni, (Citation2022a) inferred from applying statistical analysis on the ISE’s data and DF system data (Alabi, Citation2015; Alabi & Olaleye, Citation2016) that irrespective of the flow direction, the higher the difference between the hydraulic properties of the layers in contact, the higher the flow turbulence induced on a flowline crossing the interface. This aligns with the numerical simulation result Cherblanc et al. (Citation2007) reported.
Generally, flowline refraction is comparable to solute plume dispersion only in cases where mechanical dispersion and solute molecular diffusion can be ignored, such as cases of single-phase flow being studied (Sebben & Werner, Citation2016b). Considering that the fluid used in the experiment is single-phase, the can also be referred to as the refraction angle of the peak solute plume flux. This is because, since the fluid is of single-phase type, the
gives the most volume of the solute plume passing through a unit area of the interface per unit of time. Hence, its refraction
dictates the dispersion magnitude (Dawe & Marcelle-de Silva, Citation2005; Govindarajan, Citation2019). Understanding such dispersion dynamics would offer vital benefits such as a better understanding of nanofluids flooding processes for increasing Enhanced Oil Recovery (EOR) efficiency and dilution processes in other stratified media (Dejam & Hassanzadeh, Citation2022; Kurasawa et al., Citation2020; Magnusson et al., Citation2004; Shamshuddin et al., Citation2019).
Using the reviewed literature, the LHPM data for this study have been validated. Also, the data have been extended to include dispersion dynamics. To our knowledge, the available works have presented findings on the volume flux and solute plume dispersion dynamics in stratified media using numerical methods based on hydraulic properties like Darcy number and K of the layers in contact (Dejam & Hassanzadeh, Citation2022; Sebben & Werner, Citation2016a, Citation2016b). However, findings based on the porosities of the layers in contact have not been reported from purely experimental data. In addition, no model has been reported that relates the porosities to as a measurement parameter for volume flux and solute plume dispersion dynamics. Therefore, in this work, an analysis and model between the porosities of the layers and
would be presented. This could have applications in many areas, such as increasing the efficiency of the flooding processes in EOR, optimising CBE covers, and improving the knowledge of solute dispersal dynamics in other stratified media.
We aim to analyse and model the relationship between the of a two-layer stratified medium and the
. In addition, we extend the
to include dispersion scenarios as it relates to
. Hence, with the results to be presented, the flow dynamics in L2 of a two-layer stratified medium of known can be described in terms of peak flux and dispersion refraction. As an advantage, our findings could find application in predicting the peak solute plume dispersion when the CBE interface of a pollution control system breaks down (Huo et al., Citation2022). In addition, incorporating the model into the coding algorithm of reservoir simulators could increase their efficiency and effectiveness in predicting displacement patterns, particularly in smart injection wells management systems for EOR. This could cater for stratification zones of limited hydraulic properties’ knowledge, i.e. zones where only porosity is known (Brouwer et al., Citation2001; Dawe & Marcelle-de Silva, Citation2005; Obibuike et al., Citation2022; Shahrokhi et al., Citation2014). Other broad application areas include environments where stratifications are often encountered, such as geothermal energy extraction, geological storage of hydrogen and carbon dioxide, chemical separation of mixtures and drug delivery in living organisms (Dejam & Hassanzadeh, Citation2022).
The subsequent sections of this paper are organised as follows. The Methodology section discusses the data source, the ISE-LHPM setup, the data obtained and the analysis methods applied. After which, the Results and Discussion are presented, followed by the Summary and Conclusions.
2. Methodology
Figure shows the flowchart of the methodology applied in this study. It starts with obtaining the data from the ISEs (section 2) and terminates with summary and conclusions (section 4).
The data used in this work were obtained from Popoola, Adegoke, Alabi et al. (Citation2010a), Popoola et al. (Citation2010b) and Aubertin et al. (Citation2009). The setup is illustrated in Figure . Each of the five LHPMs comprises two layers of different soil samples of known ϕ yielding of 0.8325, 0.8667, 0.9100, 0.9148, and 0.9523, respectively. The experiment setup comprised a 108.5 cm long inlet pipe of radius 2.23 cm and five equal outlet pipes of radii 0.3 cm each. The outlet pipes were joined to the inlet pipe at outlet angles (θ) of 0°, 20°, 50°, 70°, and 90° from the normal. As a control experiment, water was allowed to flow through the empty pipes for 60 s. The discharged water from each outlet was collected separately and used to estimate Q and q, based on Eqn. 5 and Equation6
(6)
(6) , respectively (Alabi et al., Citation2019; H. Wang et al., Citation2020).
Figure 4. Schematic diagram of the experiment setup (Popoola et al., Citation2010a).

where Q is the volumetric flow rate, v is the volume, and 60 s is the amount of time allowed.
where q is the volume flux, A is the pipe’s cross-sectional area, K is the hydraulic conductivity, i is the hydraulic gradient, and all other notations retain their meaning.
This process was repeated at different inclination angles (α) in order to observe the effect on the flow process. The process described above was done with each of the LHPMs.
2.1. Data
Table gives the obtained q at different ranging from 0
to 20
for
0.8325–0.9524. From the tables, it was observed that the
are obtained at lower θ (refraction angles from the normal) as the
increases.
Table 1. Volume flux at different inclination angles and outlet angles for
Table 2. Volume flux at different inclination angles and outlet angles for
Table 3. Volume flux at different inclination angles and outlet angles for
Table 4. Volume flux at different inclination angles and outlet angles for
2.2. Data analysis
Table shows the summary data from Tables of the at each α, for each of the five LHPMs. Since it was observed that at
,
, the whole row was omitted in Table .
Table 5. Volume flux at different inclination angles and outlet angles for
Table 6. and their associated
at each
level
From Table , four plots (Fig. ) and associated models were generated at each α. Furthermore, a combined plot (Fig. A1—shown in appendix A) and model (EquationEqn. 1A)(1A)
(1A) valid at all the α was generated. The residuals of EquationEqn. 1A
(1A)
(1A) were checked for the satisfaction of Ordinary Least Squares (OLS) regression assumptions using the internally standardised residual (Gray & Woodall, Citation1994). The internally standardised residual checks for homoskedasticity, as shown in Figure .
However, the variance observed in Figure was unsatisfactory, indicating one outlier’s presence. Hence, weighted regression was carried out based on EquationEqn. 7(7)
(7) (Romano & Wolf, Citation2017; Suárez et al., Citation2017). This was done to prevent the removal of the outlying data point, as such points are known to have undue influence on the regression line, leading to an inaccurate model (Dhakal, Citation2017).
(Davidian & Haaland, Citation1989)
where is the
residual,
is the
weight,
is the
the observed value, and
is the
predicted value.
Since only one iteration of the weighted regression yielded improvement in the residual plots, no further iteration was attempted. This resulted in the weighted combined model of EquationEqn. 12(12)
(12) . Figure shows the weighted model superimposed on the scatter plot, while show the internally standardised residual plot and normality plot, respectively.
are only visual tests. Therefore, more formal tests were conducted to ensure that the OLS assumptions were truly satisfied. The tests conducted are the Shapiro–Wilk test for normality (Table ), at a significance level of 0.05, and the Bartlett test for variance homogeneity at a confidence level of 95% (Devore, Citation2011; Freund et al., Citation2010; King & Eckersley, Citation2019; Odoi et al., Citation2022). As a precaution, it was ensured that the Shapiro–Wilk test was carried out to verify that the residuals were genuinely normally distributed before the Bartlett test was conducted. The precaution was taken because the Bartlett test only performs optimally on normally distributed data (King & Eckersley, Citation2019; Odoi et al., Citation2022).
Table 7. Shapiro–Wilk test (confidence level = 0.05) result summary for the residual
3. Result and discussion
From Table , it can be observed that there is a decreasing trend in with increasing
, except at
, with a minor difference in the trend at
. Of course, this is expected since the tendency of the flow path to be disturbed increases with a decrease in porosity viz-a-viz permeability. It can be inferred that with an increasing
(
held constant while
reduces, or
held constant while
increases)
will tend to refract towards the normal, i.e., the
flowline will tend towards the normal. This agrees with the numerical analysis findings of Sebben and Werner (Citation2016a, Citation2016b) based on K, where they also reported that a less concentrated peak solute plume is more displaced than a more considerably concentrated type. Therefore, in the waterflooding scenario, the flowlines will maintain
in zones located closer to the contact interface’s normal. At the same time, the peak solute plume flux will undergo a lower dispersal or dilution rate due to the refraction towards the normal (Sebben & Werner, Citation2016a). In comparison, with a decreasing
(held constant while
increases, or
held constant while
decreases)
will refract from the normal, i.e., the peak fluid flux flow will refract from the normal. Hence, in a waterflooding scenario, the flowlines will maintain
in zones located away from the contact interface’s normal as long as the
of the layers in contact is low enough. On a similar note, for fluids containing solute plumes (single-phase), e.g., EOR fluids containing nanoparticles or other chemicals, the peak solute plume flux
will get dispersed towards a higher refraction angle from the normal. This would lead to a faster dilution of the solutes in the L2. If the
is low enough, up to 100 times concentration lesser than the concentration in L1 could be observed in L2 (Sebben & Werner, Citation2016a). These propositions remain valid as long as the flow occurs in an AF system or arrangement.
Furthermore, from Tables , it is worth noting that there is no definite trend between and
. But,
increases as
increases. These trends indicate that the refraction angle of the peak solute plume, which causes the spread in L2, does not depend on the layer inclination. Therefore, the refraction angle is purely an interfacial property, as reported in the generalisation of Taylor dispersion theory by Dejam and Hassanzadeh (Citation2022). However, the layer inclination does affect the flux rate (volume of the solute plume per unit area per second) through L2. The latter trend is believed to be due to the known sine relationship of
, where a is the acceleration of the fluid particles, g is the acceleration due to gravity, and
retains its meaning (Ceccarelli et al., Citation2018).
3.1. Individual models
From Figure , at , it is observed that with increasing
,
decreases. The fitted model is:
where is the refraction angle of the peak solute plume concentration, and
is the porosity ratio of the layers in contact.
EquationEqn. 8(8)
(8) has a coefficient of determination (R2) of 0.82. It implies that 82% of the dependent variable
can be explained by the independent variable
.
From Figure , at , it is observed that with increasing
,
decreases. The fitted model is:
where all notations retain their meaning.
Eqn. 9ʹs . It can then be said that 89% of
can be explained by
, at
As observed in Figures , in , decreases with increasing
. However, a trend reversal is observed at about
. Also, compared to the decrease in
observed in , the decrease observed in is steeper. The model generated is:
where all notations retain their meaning.
Eqn. 10ʹs . It is of better fit based on the
value when compared to those obtained in Figures , since 92% of the
can be explained by the
data.
From , EquationEqn. 11(11)
(11) was obtained as the model:
where all notations retain their meaning.
Eqn. 11ʹs . It is the best model based on
value
As observed from EquationEqns. 8(8)
(8) –Equation11
(11)
(11) , the lowest R2 being approximately 0.82, all the models are of good fit. However, R2 can be biased in small sample size conditions like we have (Akossou & Palm, Citation2013). Nevertheless, in comparison to other statistical alternatives like the Index of Agreement (d) and the Maximal Information Coefficient (MIC), it provides a better result (Valbuena et al., Citation2019). In addition, to have a reasonable reliance on the models, it is necessary to verify the suitability of the models’ form for our context of use (Non-Darcian flow; Howarth, Citation2017; Oloro, Citation2020; Valbuena et al., Citation2019). Deng and Li (Citation2021) and Hansbo (Citation2001, Citation1960) agree with their suitability and conclude that second-order polynomials have smaller Mean Square Errors (MSE) and a high R2 with experimental data.
3.2. Combined model
From Figure gradual decrease in with increase in
is observed. It follows the same trend as the individual plots (Figures ). The superimposed weighted model line is:
where all notations retain their meaning.
Eqn. 12ʹs . The
value
for the weighted model (EquationEqn. 12)
(12)
(12) is an improvement over the unweighted model’s (EquationEqn. 1A
(1A)
(1A) )
value
. Also, Eqn. 12ʹs internally standardised residuals plot (Figure ) shows improved residual variance homoscedasticity compared to Eqn. 1A’s (Figure ). As seen in Figure , there are no outliers (data points beyond −2 or 2), which indicates the absence of any data point having undue influence on the regression line (Peck et al., Citation2008)
Figure shows the normal probability plot of the residuals. It indicated some divergence from normality. However, from Table , the Shapiro–Wilk test statistically confirmed the residuals as normally distributed. From the Bartlett test for homogeneity, a statistic of 2.95 was obtained, giving an associated P-value of 0.229. The Bartlett test result indicates that there is insufficient statistical evidence to reject the null hypothesis of the residuals being homoscedastic at the chosen P-value of 0.05. Hence, based on the satisfaction of OLS assumptions, the weighted model (EquationEqn. 12)(12)
(12) is efficient and the Best Linear Unbiased Estimator (BLUE; Pötscher & Preinerstorfer, Citation2022).
4. Summary and conclusions
A study has been carried out to highlight the flow dynamics as it relates to the refraction angle or dispersion of the peak volume or solute plume flux of a single-phase fluid flowing through the contact interface of two layers of Layered Heterogeneous Porous Media (LHPM) characterised by different porosities. The LHPM is an idealised representation of a stratified medium. The flow was orientated so that it originates from a lower porosity vis-à-vis permeability layer (top layer—L1) and flows into a higher porosity vis-à-vis permeability layer (bottom layer—L2).
From the analysis of the data, it can be inferred that:
Flowlines will maintain
(peak volume or solute plume flux) in zones located away from the contact interface’s normal, as long as the porosity ratio
of the layers that make up such a contact interface is low enough. This would lead to a higher dispersal angle and fast rate of dilution of solute plumes in the bottom layer. On the other hand, with
tending to unity,
flowline will tend towards the normal. In this case, a lower dispersal angle and slow rate of dilution of solute plumes in the bottom layer would be observed.
The inclination angle
of the stratification units or layers does not correlate with
. However,
correlates with
For an AF system, the best-unbiased estimator model that could be used to predict
based on
is:
where is the refraction angle of the
or the peak solute plume concentration and
is the porosity ratio of the contact interface.
(4)The results of this work could find application in any porous environment characterised by stratification with an AF system flow regime. Such instances include the mapping of
and probable dilution rate of flooded fluids in optimised smart injection well management systems for EOR. In addition, the model could optimise CBE covers used for groundwater protection by predicting the path of the peak solute flux, which could then be considered to improve the covers’ performance.
This study was based on a single-phase fluid undergoing a one-way directional flow in an AF system. However, in most cases, such dynamics do not restrain flow in nature. Occurrence of the reverse flow, i.e. DF, is possible. Flow could also be in multi-phase like those experienced in hydrocarbon reservoirs. Hence, these limitations present possible future research opportunities.
Nomenclature
Porosity ratio
K Hydraulic conductivity
V Volume of fluid
i Hydraulic gradient
Peak or maximum volume flux of solute plume
q Volume flux (m/s)
Q Volumetric flow rate (m3s−1)
A Cross-sectional area perpendicular to the line offlow (m2)
L1 Layer 1 or the top layer for the AF system whereflow originates
L2 Layer 2 or the bottom layer for the AF system where flow terminates
*L1 Layer 1 or the top layer for the DF system where flow originate
*L2 Layer 2 or the bottom layer for the DF system where flow terminates
Greek symbols
α Inclination angle
θ Flowline deflection angle from the normal (Outlet angle) or refraction angle of solute plume flux
Entry angle
Refraction angle
Refraction angle from the normal of the flowline associated with or refraction angle of peak solute plume flux
L1 porosity
L2 porosity
*L1 porosity
*L2 porosity
ϕ Porosity
Porosity ratio
Disclosure statement
No potential conflict of interest was reported by the author(s).
Additional information
Funding
References
- Akossou, A. Y. J., & Palm, R. (2013). Impact of data structure on the estimators r-square and adjusted r-square in linear regression. International Journal of Mathematics and Computation, 20, 84–18. https://www.researchgate.net/publication/289526309
- Alabi, O. O. (2015). Velocity profile of fluid flow in heterogeneous porous media. Electronic Journal of Geotechnical Engineering, 20(3), 943–952. https://doi.org/10.15242/iicbe.c0315085
- Alabi, O. O., & Akanni, I. A. (2021). 3D fluid transport in layered heterogeneous porous media. In K. O. Alawode, R. B. Ibrahim, & A. U. Adebanjo (Eds.), 2nd International Conference on Engineering and Environmental Sciences (ICEES) (November 23-25, 2021): Re-thinking engineering and technology for environmental sustainability in the face of global pandemic (pp. 346–355). Faculties of Engineering and Environmental Sciences, Osun State University.
- Alabi, O. O., & Akanni, I. A. (2022a). Fluid velocity profile in ascending and descending layer heterogeneous porous media. UNIOSUN Journal of Engineering and Environmental Sciences, 4(1). https://doi.org/10.36108/ujees/2202.40.0180
- Alabi, O. O., & Akanni, I. A. (2022b). Single-phase fluid’s volume flux modelling in a layered heterogeneous porous media. In F. Chitea, I. Munteanu, L. Matenco, R. Dinescu, & I. Stanciu (Eds.), (1) 16th workshop of the international lithosphere program task force sedimentary basins and 7th Geoscience symposium of Romanian society of applied geophysics (pp. 93). Romanian Society of Applied Geophysics.
- Alabi, O. O., Ojurongbe, T. A., & Sedara, S. O. (2019). Gradients of seepage velocity model for contaminant transport prediction. Scientific African, 4, e00087. https://doi.org/10.1016/j.sciaf.2019.e00087
- Alabi, O. O., & Olaleye, K. O. (2016). Modifying Hagen-Poiseuille's equation for an inclined porous media with varying porosity. Journal of Indian Geophysical Union, 20(20), 231–240.
- Alabi, O. O., & Sedara, S. O. (2020). Validity of series and parallel bed layer permeability equations from experimental and numerical modeling. Scientific African, 10, e00579. https://doi.org/10.1016/j.sciaf.2020.e00579
- Aubertin, M., Cifuentes, E., Apithy, S. A., Bussière, B., Molson, J., & Chapuis, R. P. (2009). Analyses of water diversion along inclined covers with capillary barrier effects. Canadian Geotechnical Journal, 46(10), 1146–1164. https://doi.org/10.1139/T09-050
- Banihashem, S., & Karrabi, M. (2020). Investigation of suspended particle size effects on clogging of soil filters under laminar flow. European Journal of Environmental and Civil Engineering, 1–10. https://doi.org/10.1080/19648189.2020.1761455
- Bég, O. A., Espinoza, D. E. S., Kadir, A., Shamshuddin, M. D., & Sohail, A. (2018). Experimental study of improved rheology and lubricity of drilling fluids enhanced with nano-particles. Applied Nanoscience, 8(5), 1069–1090. https://doi.org/10.1007/s13204-018-0746-4
- Bos, M. G. (2006). Basics of groundwater flow. In H. P. Ritzema (Ed.), Drainage principles and applications (3rd), pp. 225–261). International Institute for Land Reclamation and Improvement. https://www.researchgate.net/publication/40105995
- Brouwer, D. R., Jansen, J. D., van der Starre, S., van Kruijsdijk, C. P. J. W., & Berentsen, C. W. J. 2001 May 21. Recovery Increase through Water Flooding with Smart Well Technology. All Days. https://doi.org/10.2118/68979-MS
- Cao, R., Wang, Y., Cheng, L., Ma, Y. Z., Tian, X., & An, N. (2016). A new model for determining the effective permeability of tight formation. Transport in Porous Media, 112(1), 21–37. https://doi.org/10.1007/s11242-016-0623-0
- Casagrande, A. (1937). Seepage through dams. New England Water Works Association, LI(2), 131–172.
- Ceccarelli, F., la Scaleia, B., Russo, M., Cesqui, B., Gravano, S., Mezzetti, M., Moscatelli, A., d’Avella, A., Lacquaniti, F., & Zago, M. (2018). Rolling motion along an incline: Visual sensitivity to the relation between acceleration and slope. Frontiers in Neuroscience, 12, 12. https://doi.org/10.3389/fnins.2018.00406
- Cedergren, R. H. (1997). Seepage, drainage, and flow nets (3rd) ed., Vol. 16). John Wiley & Sons.
- Chen, Z., Ma, X., Zhan, H., Dou, Z., Wang, J., Zhou, Z., & Peng, C. (2022). Experimental investigation of solute transport across transition interface of porous media under reversible flow directions. Ecotoxicology and Environmental Safety, 238, 113566. https://doi.org/10.1016/j.ecoenv.2022.113566
- Cherblanc, F., Ahmadi, A., & Quintard, M. (2007). Two-domain description of solute transport in heterogeneous porous media: Comparison between theoretical predictions and numerical experiments. Advances in Water Resources, 30(5), 1127–1143. https://doi.org/10.1016/j.advwatres.2006.10.004
- Davidian, M., & Haaland, P. (1989). The relationship between transformation and weighting in regression, with application to biological and physical science.
- Dawe, R. A., & Marcelle-de Silva, J. (2005). Viscous and permeability effects on miscible displacement in heterogeneous porous media. West Indian Journal of Engineering, 28(1), 69–84.
- Dejam, M., & Hassanzadeh, H. (2022). Dispersion tensor in stratified porous media. Physical Review E, 105(6), 065115. https://doi.org/10.1103/PhysRevE.105.065115
- Deng, H., & Li, L. (2021). Polynomial to non-Darcian flow study. IOP Conference Series: Earth and Environmental Science, 634(1), 012016. https://doi.org/10.1088/1755-1315/634/1/012016
- Devore, J. L. (2011). Probability & statistics for engineering and the sciences (8th) ed.). Cengage Learning.
- Dhakal, C. P. (2017). Dealing with outliers and influential points while fitting regression. Journal of Institute of Science and Technology, 22(1), 61–65. https://doi.org/10.3126/jist.v22i1.17741
- Eagleman, J. R., & Jamison, V. C. (1962). Soil layering and compaction effects on unsaturated moisture movement. Soil Science Society of America Journal, 26(6), 519–522. https://doi.org/10.2136/sssaj1962.03615995002600060004x
- Ferdows, M., Shamshuddin, M. D., Salawu, S. O., & Sun, S. (2022). Thermal cooling performance of convective non-Newtonian nanofluid flowing with variant power-index across moving extending surface. Scientific Reports, 12(1), 8714. https://doi.org/10.1038/s41598-022-12333-y
- Freeze, A. R., & Cherry, J. A. (1979). Groundwater (1st) ed.). Prentice Hall.
- Freund, R. J., Wilson, W. J., & Mohr, D. L. (2010). Inferences for two or more means. In Statistical Methods (pp. 245–320). Elsevier. https://doi.org/10.1016/B978-0-12-374970-3.00006-8
- Govindarajan, S. (2019). An overview on extension and limitations of macroscopic darcy’s law for a single and multi-phase fluid flow through a porous medium. International Journal of Mining Science, 5(4). https://doi.org/10.20431/2454-9460.0504001
- Gray, J. B., & Woodall, W. H. (1994). The maximum size of standardized and internally studentized residuals in regression analysis. The American Statistician, 48(2), 111–113. https://doi.org/10.1080/00031305.1994.10476035
- Hansbo, S. (1960). Consolidation of clay, with special reference to influence of vertical sand drains [ Ph.D.]. Chalmers Tekniska Høgskola.
- Hansbo, S. (2001). Consolidation equation valid for both darcian and non-Darcian flow. Géotechnique, 51(1), 51–54. https://doi.org/10.1680/geot.51.1.51.39357
- Hosking, L. J., Thomas, H. R., & Sedighi, M. (2018). A dual porosity model of high-pressure gas flow for geoenergy applications. Canadian Geotechnical Journal, 55(6), 839–851. https://doi.org/10.1139/cgj-2016-0532
- Howarth, R. J. (2017). Dictionary of mathematical geosciences. Springer International Publishing. https://doi.org/10.1007/978-3-319-57315-1
- Hu, J., Ke, H., Lan, J.-W., Chen, Y.-M., & Meng, M. (2020). A dual-porosity model for coupled leachate and gas flow to vertical wells in municipal solid waste landfills. Géotechnique, 70(5), 406–420. https://doi.org/10.1680/jgeot.18.P.193
- Huo, W., Zhu, Z., Hao, J., Zhang, W., & Peng, Y. (2022). Experimental study and numerical simulation on effectiveness of different capillary barriers in silt low subgrade. Bulletin of Engineering Geology and the Environment, 81(6), 246. https://doi.org/10.1007/s10064-022-02742-8
- King, A. P., & Eckersley, R. J. (2019). Inferential Statistics IV: Choosing a Hypothesis Test. In Statistics for Biomedical Engineers and Scientists (pp. 147–171). Elsevier. https://doi.org/10.1016/B978-0-08-102939-8.00016-5
- Ku, C.-Y., Hong, L.-D., Liu, C.-Y., Xiao, J.-E., & Huang, W.-P. (2021). Modeling transient flows in heterogeneous layered porous media using the space–time trefftz method. Applied Sciences, 11(8), 3421. https://doi.org/10.3390/app11083421
- Kurasawa, T., Suzuki, M., & Inoue, K. (2020). Experimental assessment of solute dispersion in stratified porous media. Hydrological Research Letters, 14(4), 123–129. https://doi.org/10.3178/hrl.14.123
- Leliavsky, S. (1955). Irrigation and hydraulic design: General principles of hydraulic design. In yjyy (Vol. 1). Chapman Hall.
- Liang, X., Zhang, Y., Liu, J., Ma, E., & Zheng, C. (2019). Solute transport with linear reactions in porous media with layered structure: A semianalytical model. Water Resources Research, 55(6), 5102–5118. https://doi.org/10.1029/2019WR024778
- Li, X., Ke, T., Wang, Y., Zhou, T., Li, D., Tong, F., & Wen, J. (2020). Hydraulic conductivity behaviors of karst aquifer with conduit-fissure geomaterials. Frontiers in Earth Science, 8. https://doi.org/10.3389/feart.2020.00030
- Li, Y., Werner, A. D., Wen, Z., & Zhu, Q. (2022). Solute transport in permeable porous media containing a preferential flow feature: Investigation of non-Darcian flow effects. Journal of Hydrology, 604, 127210. https://doi.org/10.1016/j.jhydrol.2021.127210
- Lu, C., Chen, Y., Zhang, C., & Luo, J. (2013). Steady-state freshwater–seawater mixing zone in stratified coastal aquifers. Journal of Hydrology, 505, 24–34. https://doi.org/10.1016/j.jhydrol.2013.09.017
- Magnusson, B. M., Anissimov, Y. G., Cross, S. E., & Roberts, M. S. (2004). Molecular size as the main determinant of solute maximum flux across the skin. Journal of Investigative Dermatology, 122(4), 993–999. https://doi.org/10.1111/j.0022-202X.2004.22413.x
- March, R., Doster, F., & Geiger, S. (2018). Assessment of CO 2 storage potential in naturally fractured reservoirs with dual‐porosity models. Water Resources Research, 54(3), 1650–1668.
- Ng, C. W. W., Liu, J., Chen, R., & Xu, J. (2015). Physical and numerical modeling of an inclined three-layer (silt/gravelly sand/clay) capillary barrier cover system under extreme rainfall. Waste Management, 38, 210–221. https://doi.org/10.1016/j.wasman.2014.12.013
- Obibuike, U. J., Kerunwa, A., Udechukwu, M. C., & Ekwueme, S. T. (2022). Simulation studies on comparative evaluation of waterflooding and gas injection in niger delta thin-bed reservoir. Open Journal of Yangtze Oil and Gas, 07(1), 65–83. https://doi.org/10.4236/ojogas.2022.71005
- Odoi, B., Twumasi-Ankrah, S., Samita, S., & Al-Hassan, S. (2022). The efficiency of bartlett’s test using different forms of residuals for testing homogeneity of variance in single and factorial experiments-a simulation study. Scientific African, 17, e01323.
- Oloro, O. J. (2020). Application of R-squared in the analysis of estimated water encroachment into a reservoir. Nigerian Journal of Environmental Sciences and Technology, 4(1), 70–76. https://doi.org/10.36263/nijest.2020.01.0155
- Pavlovskaya, G. E., Meersmann, T., Jin, C., & Rigby, S. P. (2018). Fluid flow in a porous medium with transverse permeability discontinuity. Physical Review Fluids, 3(4), 044102. https://doi.org/10.1103/PhysRevFluids.3.044102
- Peck, R., Olsen, C., & Devore, J. (2008). Introduction to statistics and data analysis (3rd) ed.). Thomson Brooks/Cole.
- Popoola, O. I., Adegoke, J. A., & Alabi, O. O. (2009). The effects of porosity and angle of inclination on the deflection of fluid flow in porous media. Glob. J. of Pure and Appl. Sci, 15(3–4), 401–416. https://doi.org/10.4314/gjpas.v15i3-4.48568
- Popoola, O. I., Adegoke, J. A., & Alabi, O. O. (2010a). The relationship between the angle of deflection and porosity ratio of stratified homogenous porous media. Journal of Applied and Environmental Sciences, 6(2), 35–41.
- Popoola, O. I, Adegoke, J. A., Alabi, O. O., Akinluyi, F. O., & Fayemiwo, K. A. (2010b). Controlling contaminated fluid from polluting groundwater using porous media. Journal of American Science, 6(9).
- Pötscher, B. M., & Preinerstorfer, D. (2022). A modern Gauss-Markov theorem? Really?
- Romano, J. P., & Wolf, M. (2017). Resurrecting weighted least squares. Journal of Econometrics, 197(1), 1–19. https://doi.org/10.1016/j.jeconom.2016.10.003
- Sai, Y., Priya, J., Anil Kumar, P., & Rao, B. R. S. (2020). Modelling recovery process in dual porosity and dual permeability reservoirs. International Research Journal of Engineering and Technology. www.irjet.net
- Salimzadeh, S., & Khalili, N. (2014). Double porosity behaviour and application in geotechnical problems. In Numerical methods in geotechnical engineering (pp. 1031–1036). CRC Press. https://doi.org/10.1201/b17017-183
- Sebben, M. L., & Werner, A. D. (2016a). A modelling investigation of solute transport in permeable porous media containing a discrete preferential flow feature. Advances in Water Resources, 94, 307–317. https://doi.org/10.1016/j.advwatres.2016.05.022
- Sebben, M. L., & Werner, A. D. (2016b). On the effects of preferential or barrier flow features on solute plumes in permeable porous media. Advances in Water Resources, 98, 32–46. https://doi.org/10.1016/j.advwatres.2016.10.011
- Shahrokhi, O., Fatemi, M., Sohrabi, M., Ireland, S., & Ahmed, K. (2014) Assessment of three phase relative permeability and hysteresis models for simulation of water-alternating-gas WAG injection in water-wet and mixed-wet systems. All Days. https://doi.org/10.2118/169170-MS
- Shamshuddin, M. D., Mishra, S. R., Kadir, A., & Bég, O. A. (2019). Unsteady chemo-tribological squeezing flow of magnetized bioconvection lubricants: Numerical study. Journal of Nanofluids, 8(2), 407–419. https://doi.org/10.1166/jon.2019.1587
- Silliman, S. E., Zheng, L., & Conwell, P. (1998). The use of laboratory experiments for the study of conservative solute transport in heterogeneous porous media. In Hydrogeology Journal (Vol. 6). Springer-Verlag.
- Solnyshkina, O. A., Batyrshin, E. S., & Pityuk, Yu, A. (2021). Investigation of hydrodynamic flows in micromodels of double porosity media. Fluid Dynamics, 56(4), 451–459. https://doi.org/10.1134/S001546282104011X
- Suárez, E., Pérez, C., Rivera, R., & Martínez, M. (2017). Weighted least-squares linear regression. In Applications of regression models in epidemiology (pp. 117–128). John Wiley & Sons, Inc. https://doi.org/10.1002/9781119212515.ch8
- Sun, S., & Zhang, T. (2020). Recent progress in multiscale and mesoscopic reservoir simulation. In Reservoir simulations (pp. 205–258). Elsevier. https://doi.org/10.1016/B978-0-12-820957-8.00005-8
- Usman, Shaheen, S., Arain, M. B., Nisar, K. S., Albakri, A., & Shamshuddin, M. D. (2022). A case study of heat transmission in a Williamson fluid flow through a ciliated porous channel: A semi-numerical approach. Case Studies in Thermal Engineering, 102523. https://doi.org/10.1016/j.csite.2022.102523
- Valbuena, R., Hernando, A., Manzanera, J. A., Görgens, E. B., Almeida, D. R. A., Silva, C. A., & García-Abril, A. (2019). Evaluating observed versus predicted forest biomass: R-squared, index of agreement or maximal information coefficient? European Journal of Remote Sensing, 52(1), 345–358. https://doi.org/10.1080/22797254.2019.1605624
- Wang, L., Liu, B., Guo, Y., Li, J., Yang, H., Liu, H., & Heng, S. (2022). Experimental investigation of the cementation and tensile properties of cement to formation interface considering surface irregularity and drilling mud residue. Journal of Petroleum Exploration and Production Technology. https://doi.org/10.1007/s13202-022-01585-z
- Wang, H., Qian, H., & Gao, Y. (2020). Non-darcian flow in loess at low hydraulic gradient. Engineering Geology, 267. https://doi.org/10.1016/j.enggeo.2020.105483
- Wei, W., Xia, Y., & Cai, J. (2021). Tortuosity in two-dimensional and three-dimensional fractal porous media: A numerical analysis. In Modelling of Flow and Transport in Fractal Porous Media (pp. 25–36). Elsevier. https://doi.org/10.1016/B978-0-12-817797-6.00007-5
- Xu, Z., & Tartakovsky, A. M. (2017). Method of model reduction and multifidelity models for solute transport in random layered porous media. Physical Review E, 96(3), 033314. https://doi.org/10.1103/PhysRevE.96.033314
- Zhang, W., & Yuan, S. (2019). Characterising preferential flow in landfilled municipal solid waste. Waste Management, 84, 20–28.