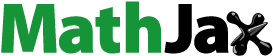
Abstract
The reliability assessment helps to determine the existing power system reliability. Equipment failures may decrease reliability and the major contributory factors for power system unreliability. Therefore, the objective of this study was reliability assessment for distribution with distribution generation units and without distribution generation units, Dangila substation from 2020 to 2021. The base case reliability indices were 774.93, 1231.48, 1.589, 85.96%, and 14.04 for system average interruption frequency index, system average interruption duration index, customer average interruption duration index, average service availability index and average service unavailability index respectively. These indices are below the standards of Ethiopian Electric power set indices values of 20 system average interruption frequency index and 25 system average interruption duration index. Therefore, the system needs improvement to achieve the minimum system reliability standard. Distribution generation was used to improve the system reliability in this paper. Distribution generation was placed at five suggested locations of feeder seven from substation, 66kilometer, 80kilometer, 100kilometer, 113.3kilometer, and 146kilometer distances. The results shows that as distribution generation was placed further from Supply point the reliability of the power system will be improved more. The assessment result of feeder seven shows that the feeder had more outages with the base cases of 636, 833, and 1.311 system average interruption frequency index, system average interruption duration index, customer average interruption duration index respectively. The size of distribution generation for feeder seven was 3.13 MW to supply 50% of feeder peak load. After distribution generation placed at feeder seven the system average interruption frequency index, system average interruption duration index, customer average interruption duration index, and average service unavailability index indices were 20.0868, 25.109, 1.25, and 99.71%. This shows that the system reliability is improved as compared to the base case and the reliability standards of the country were achieved.
PUBLIC INTEREST STATEMENT
There has been growing interest in electrical energy. Since electrical energy plays a vital role in the economic and social development of any country, a stable and reliable electric power supply system is essential for the technology and economic growth of any society. Integrating distributed generation with existing grid is one of the methods of reliability improvement that aims at increasing power system reliability. The effectiveness of system depends on the placement of distribution generation and the size distribution generation. These are considered to obtain optimal results in terms of system reliability. Integrating distribution generation with national grid is used to obtain most reliable power system. In Ethiopia, electric power interruption is a common incident. Thus, integrating distribution generation with national grid is a solution to improve power system reliability.
1. Introduction
Using electricity is becoming an essential part of our lives. Since electrical energy plays a vital role in the economic and social development of any country, a stable and reliable electric power supply system is essential for the technology and economic growth of any society. Electric power utilities should provide uninterrupted power supply to their customers.
The main problem facing in electric power utilities in the case study is the power interruption. However, there is no sufficient technical research has been carried out in the distribution network. This is due to lack of technical know-how in regarding system reliability assessment and improvement in the utility. It is not possible to prevent all component failures in the electric system. Due to this, reliability is a common problem in the power system. Therefore, the power system needs reliability assessment and improvement with distribution generation.
In Ethiopia, the economic and social effects of loss of electric service have significant impacts for both the Ethiopian electric power and the electric utilities supplying electric energy for end users of electric service. The major power outage cost confined to one state can be on the order of tens of millions of dollars. If a major power outage affects multiple states, then the cost can exceed a hundred million dollars. The power system is vulnerable to system abnormalities such as control failures, protection or communication system failures, and disturbances, such as lightning, and human operational errors (Tur, Citation2020; Jamshid & Abrar, Citation2020; Ostovar et al., Citation2021; Aruna et al., Citation2021; Prajapati & Mahajan, Citation2021).
The power distribution system was established mainly to provide adequate electricity supply to its customers as economically as possible with reasonable assurance of reliability. Nowadays, in Ethiopia, the power distribution networks have grown exponentially in terms of size and technology over the past few years. Therefore, the utility company must strive to ensure that the customer’s reliability requirements are met with optimum strategic planning and the lowest possible cost. The ability of the system to provide an adequate supply of electric energy determined by the term reliability (Kumar et al., Citation2020; Karngala & Singh, Citation2021; Gaur et al., Citation2021; Khan et al., Citation2019; Ghiasi et al., Citation2019; Liu et al., Citation2019).
The reason behind it is the generation and transmission systems are capital intensive and the inadequacy can have widespread consequences for both society and the environment. However, a distribution network is slightly cheaper compared to the generation and distribution because its effects are more localized. As analyzing the customer failure statistics of most utilities shows that the distribution system makes the greatest individual contribution to the unavailability of electrical supply to the customer (Ostovar, Citation2021; Karngala & Singh, Citation2021; Puri et al., Citation2020; Kannan et al., Citation2021; Ahmad, Citation2021).
Assessment and evaluation of the reliability of power system networks are the most accurate and effective way in decision making, especially in planning, operation, and maintenance. The historical assessment and predictive methods are normally used to evaluate the reliability of a distribution network. Most of the utilities focus on historical assessment rather than predictive methods. Therefore, the predictive methods are categorized into analytical and simulation methods. Reliability assessment method for distribution systems can be divided into two classes: simulation and analytical method (Prajapati & Mahajan, Citation2021; Mi et al., Citation2021; Zheng et al., Citation2012; Akhtar et al., Citation2021; de Sousa et al., Citation2019). The simulation methods is the most flexible but requires extensive time in computational and uncertainty in precision and analytical method can be fatally divided into two; network modeling and Markov modeling. This network modeling is the most popular technique for distribution system reliability analysis due to the simplicity of the method and the natural similarities between the network model and the distribution topology. The basic difference between simulation and analytical methods is the way in which the system reliability indices are evaluated (Karngala & Singh, Citation2021; Tiwary & Tiwary, Citation2021 Myhre et al., Citation2021; Li et al., Citation2019; Bae & Kim, Citation2008; Jirutitijaroen & Singh, Citation2008). By applying both analytical and simulation methods, the reliability indices for distribution systems will be determined such as System Average Interruption Frequency Index and System Average Interruption Duration Index. The probability distribution of System Average Interruption Frequency Index and System Average Interruption Duration Index, which give information about the variability of the indices, also needs to obtain to improve the reliability indices (Jamshid & Abrar, Citation2020; Tiwary & Tiwary, Citation2020; Memarzadeh & Keynia, Citation2020; Ahmed et al., Citation2021; Tiwary, Citation2019; Barzkar et al., Citation2022).
Monte Carlo methods are a class of computational methods that rely on repeated random sampling to compute the statistics. More broadly, Monte Carlo methods are useful for modeling phenomena with significant uncertainty in the systems. They are used for obtaining numerical solutions to problems which are too complicated to solve analytically. In an analytical method, unfortunately, assumptions are frequently required to simplify the problems. As complex systems and complex operating procedures have to be considered, analytical method is not even capable to achieve the correct solution. Therefore, the simulation techniques are very important in the reliability evaluation. Monte Carlo simulation is increasingly considered by system planners due to the capability of modeling system behavior more comprehensively and informatively. Sequential Monte Carlo method is typically applied for solving the uncertainty of a system in chronological order. If the operating life of the system is sufficiently simulated using Monte Carlo method, it is possible to conclude the behavior of the system and obtain a clear picture of the type of failure. Simulation aims to make estimates of unknown parameters which will serve as real experiments. Monte Carlo simulation methods estimate power system reliability indices by simulating the actual operations and random events in the system. The method treats the problem as a series of real experiments. The technique used in this study is sequential simulation that can comprehensively take into account the chronological behavior of the system. Therefore, the method treats the Problem as a series of experiments. Monte Carlo simulation requires a large amount of computing time as compared to analytical methods. A significant advantage of utilizing sequential Monte Carlo simulation in bulk electric system reliability analysis is its ability to provide reliability index probability distributions in addition to the expected values of these indices.
This study contributes to the existing knowledge by providing the integration of distribution generation with the existing national grid for reliability improvements. Distribution generation is used to improve the overall power system reliability.
2. Materials and methods
2.1 Study area and period
Reliability assessment is conducted in Dangila town from 14 January 2020 to 21 January 2021. Dangila is a town in Awi Zone in Amhara regional state. It is 76 kilometers away from Bahir Dar, which is the capital city of Amhara region. There is 66kVA substation in the town. Dangila substation serves to the town and the surrounding community.
Dangila is located in the North-Western parts of Ethiopia between 11.26° North latitude 36.85° East longitudes. There is electricity access to town and the surrounding community. However, system reliability is a common problem and the community suffer with this reliability problem.
2.2 Data collection
The data were obtained from Ethiopian Electric Utility and Electric power. The data obtained included fault statistics, outage consequences, the number of interruptions per year, interruption duration, and energy not supplied. The data regarding distribution network such as existing distribution system capacity, the capacity of power supplied to the network was collected. The data like the number of connected feeders, minimum, maximum demands per year, the distance from substation to the feeder was collected from Ethiopian Electric.
2.3 System modeling
The distribution generation system components were mathematically modeled with the perspective of reliability improvement. The system reliability indices were calculated with the following equations (Equations 1–7).
Ni is Total number of customers interrupted
NT is Total number of customers served
λi is number of interruptions
Ui is restoration time, minutes
Where, La (i) is the average load given by:
Where, Lp is peak load, Lf is load factor, Ed is average energy and T is time required.
DIgSILENT software was used to model the existing systems, and the collected data was entered to DIgSILENT software for the existing system reliability assessment. Distribution generation placement was modeled with DIgSILENT and reliability was analyzed.
2.3 Single line diagram system of substation
The single line of Bahir Dar 400kV substation II is shown (Figure ). At this substation the 66kV transmission line is stretched to 76.47 km to dangle substation. Then, the distribution system in the city has a primary voltage of 15kV and 33 kV. This voltage value was stepped down to 380 V/220 in three-phase and single phase respectively.
2.4 Sizing of distribution generation
In determining the size of distribution generation, the following were taken into consideration:
Distribution generation is placed at the most outages feeders.
Keeping the power factor of distribution generation constant
Maximum energy not supplied.
In this case study feeder two, feeder five, and feeder seven have more interruption duration and frequency. The corresponding peak loads are 3.742, 1.326, and 6.25 MW respectively. The size of the selected distribution generation is based on the peak load of feeder seven which is 6.25 mW. This feeder also have large values of energy not supplied. The size of distribution generation is 50% peak load of feeder seven. The selection of this 50% is for the reduction of the annual revenue loss from the substation. Therefore, size distribution generation is 3.25 mW.
2.5 Placement of distribution generation
The placement of distribution generation was contributory factor for reliability improvement. Average reliability indices of the distribution system were analyzed. Among the seven feeders, three have more interruption durations: feeder two, feeder five, and feeder seven. Therefore, the placements of distributed generation were on those three feeders to find optimal locations among three feeders (Table ). This assessment indicates that feeder seven has largest value of energy not supplied.
Table 1. Placements of distributed generation
2.6 Feeder seven reliability indices with distance perspectives
Distribution generation was placed at different points of the radial distribution system. At feeder seven, five locations were suggested for placing distribution. The following distances from the substation were considered for feeder reliability assessment.
Distribution generation located at substation
Distribution generation located at 6.6 km distance
Distribution generation located at 8 km distance
Distribution generation located at 10 km distance
Distribution generation located at 11.33 km distance
Distribution generation located at 14.6 km distance
In conclusion distribution generation placed at the start of the feeder barely improves the reliability indices. The placed distribution generation was not mitigated feeder at this distance. It acts as an additional source to supply bus. However, the distribution generation would be used to supply loads during grid fails. Thus, reliability indices were slightly increased and significant improvements as the distribution generation was placed at distance from the supply point. Therefore, the base reliability indices SAIFI, SAIDI, and ENS were decreased, but ASAI were increased while distribution generation was further from the substation. In this regard, distribution generation was placed at 14.6 km (Figure ) end of customers for more improvements.
3. Result and discussion
3.1 Result of reliability versus distance at feeder seven
The study proposed five locations for distribution generation placement to find optimal reliability improvements in power system on feeder seven.
3.1.1 Distribution generation supply point
As distribution generation was placed at supply point, there is no reliability improvement. The reliability indices were the same as the base case. The result obtained shows that the probability of interruption and duration occurring in the supply point happens rarely. Therefore, distribution generation placement at supply point is not mitigating the power system reliability. This distribution generation is used as an additional source in this regard.
3.1.2 Distribution generation location at line 6.6 km
When distribution generation is placed at 6.6 km from substation, distribution generation barely improves reliability indices. Therefore reliability improvement indices at 6.6 km of SAIFI, SAIDI, and ENS were 2.2%, 2.113% and 12.14% respectively with respect the base case.
3.1.3 Distribution generation location at line 8 km
As distribution generation is placed at 8 km, the reliability indices are improved more in the previous distances. The result obtained shows the reliability improvement indices at 8 km of SAIFI, SAIDI, and ENS were 40.88%, 39.97%, and 30.33% respectively.
3.1.4 Distribution generation location at line 10 km
As distribution generation is placed at 10 km from substation, the reliability indices are improved to 61.32% of SAIFI, 62.18% SAIDI, and 81.78% ENS.
3.1.5 Distribution generation location at line 11.33 km
As distribution generation is placed at 11.33 km from substation, the reliability indices are improved to 85% of SAIFI, 83.07% SAIDI, and 82.707% ENS.
3.1.6 Distribution generation location at line 14.6 km
Distributed generation placement at the starting point of the feeder barely improved the reliability indices. The distributed generation acts as an additional source to the supply bus as the power grid. As the grid fails to supply the distributed generation supply and reliability indices are slightly improved. However, placement of distributed generation at far point from supply improves the reliability significantly. There are different results as the same magnitude of distributed generation is placed at the end point of the supply due to distance effects.
As distribution generation is placed at line 14.6 km, the reliability indices (Figure ) results are improved to 99.99% of SAIFI, 96.998% SAIDI, 92.1% ENS, and 99.71% ASAI.
4. Conclusion
This work introduced the optimal placement and size of distribution generation for reliability improvement. The reliability assessment was conducted on the radial distribution systems of 15 kV and 33 kV. Feeder seven was selected as the optimal placement of distribution generation. Reliability indices were improved more as the distribution generation was placed further from the substation. As distribution generation was placed at feeder seven the average system available index was 99.71%. The average system availability index of this substation was improved by 13.77%. This is nearly the same as average system availability index of the standard values of the country. It is concluded that placing distribution generation at optimal place on national grid is a reasonable choice to improve the power system reliability. Therefore, using a distribution generation for system reliability improvement is important to overcome the reliability problem in Ethiopia.
Limitations
The modeling of this distribution system is done by placements of distribution generation in the system as the type of radial network and other types of distribution systems such as meshed and ring networks may be considered for reliability evaluation in the future.
Abbreviations
AC: Alternative current that depend at time t.
AENS: Average Energy Not Supplied Index.
ASAI: Average Service Availability Index.
ASUI: Average Service Unavailability Index.
CAIDI: Customer Average Interruption Duration Index.
CAIFI: Customer Average Interruption Frequency Index.
DG: Distributed generation
Disclosure statement
No potential conflict of interest was reported by the author(s).
Data availability statement
The date of this study will not be shared publicly.
Additional information
Funding
Notes on contributors
Addisu Mamo
Addisu Mamo lecturer at the faculty of electrical and computer engineering, Debre Tabour University Institute of Technology, Ethiopia. I obtained my first degree in Electrical Power Engineering in Debre Markos University and second degree in Electrical Power System Engineering in Bahir Dar University 2014 and 2020 respectively. My teaching experience in power system engineering alongside with my research background, m research interest is power system reliability.
Abraham Hizkiel
Abraham Hizkiel Nebey is an assistant professor at faculty of electrical and computer engineering, Bahir Dar Institute of Technology. He obtained his first degree in Electrical Power Engineering in Debre Markos University and second degree in Electrical Power System Engineering in Bahir Dar University.
References
- Ahmad, S. (2021). Reliability enhancement of electric distribution network using optimal placement of distributed generation. Sustainability, 13(20), 11407. https://doi.org/10.3390/su132011407
- Ahmed, K. M. U., Alvarez, M., and Bollen, M. H. (2021). A novel reliability index to assess the computational resource adequacy in data centers. IEEE Access, 9, 54530–10. https://doi.org/10.1109/ACCESS.2021.3070915
- Akhtar, I., Kirmani, S., and Jameel, M. (2021). Reliability assessment of power system considering the impact of renewable energy sources integration into grid with advanced intelligent strategies. IEEE Access, 9, 32485–32497. https://doi.org/10.1109/ACCESS.2021.3060892
- Aruna, S., Suchitra, D., Rajarajeswari, R., and Fernandez, S. G. (2021). A comprehensive review on the modern power system reliability assessment. International Journal of Renewable Energy Research (IJRER), 11(4), 1734–1747.
- Bae, I. -S. and Kim, J. -O. (2008). Reliability evaluation of customers in a microgrid. IEEE Transactions on Power Systems, 23(3), 1416–1422. https://doi.org/10.1109/TPWRS.2008.926710
- Barzkar, A., Najafzadeh, M., and Homaei, F. (2022). Evaluation of drought events in various climatic conditions using data-driven models and a reliability-based probabilistic model. Natural Hazards, 110(3), 1931–1952. https://doi.org/10.1007/s11069-021-05019-7
- de Sousa, R. S., Martins, C. C., & Sperandio, M. (2019, September). Distribution System Reliability Assessment Using Sequential Monte Carlo Simulation. In 2019 IEEE PES Innovative Smart Grid Technologies Conference-Latin America (ISGT Latin America) (pp. 1–6). IEEE.
- Gaur, V., Yadav, O. P., Soni, G., & Rathore, A. P. S. (2021). A literature review on network reliability analysis and its engineering applications. Proceedings of the Institution of Mechanical Engineers, Part O: Journal of Risk and Reliability, 235(2), 167–181.
- Ghiasi, M., Ghadimi, N., and Ahmadinia, E. (2019). An analytical methodology for reliability assessment and failure analysis in distributed power system. SN Applied Sciences, 1(1), 1–9. https://doi.org/10.1007/s42452-018-0049-0
- Jamshid, S., & Abrar, M. (2020, March). Evaluation of load point and customer point indices of a distribution generation system. In 2020 International youth conference on radio electronics, electrical and power engineering (REEPE) (pp. 1–5). IEEE.
- Jirutitijaroen, P. and Singh, C. (2008). Comparison of simulation methods for power system reliability indexes and their distributions. IEEE Transactions on Power Systems, 23(2), 486–493. https://doi.org/10.1109/TPWRS.2008.919425
- Kannan, D., Moazzeni, S., Mostafayi Darmian, S., and Afrasiabi, A. (2021). A hybrid approach based on MCDM methods and Monte Carlo simulation for sustainable evaluation of potential solar sites in east of Iran. Journal of Cleaner Production, 279, 122368. https://doi.org/10.1016/j.jclepro.2020.122368
- Karngala, A. K. and Singh, C. (2021). Reliability assessment framework for the distribution system including distributed energy resources. IEEE Transactions on Sustainable Energy, 12(3), 1539–1548. https://doi.org/10.1109/TSTE.2021.3053911
- Khan, B., Alhelou, H. H., and Mebrahtu, F. (2019). A holistic analysis of distribution system reliability assessment methods with conventional and renewable energy sources. AIMS Energy, 7(4), 413–429. https://doi.org/10.3934/energy.2019.4.413
- Kumar, S., Sarita, K., Vardhan, A. S. S., Elavarasan, R. M., Saket, R., and Das, N. (2020). Reliability assessment of wind-solar PV integrated distribution system using electrical loss minimization technique. Energies, 13(21), 5631. https://doi.org/10.3390/en13215631
- Liu, L., Wang, S., and Huang, R. (2019). An estimation method for marginal cost of distribution system reliability based on consumer-centric reliability assessment. Energy Procedia, 158, 3296–3301. https://doi.org/10.1016/j.egypro.2019.01.979
- Li, Z., Wu, W., Zhang, B., and Tai, X. (2019). Analytical reliability assessment method for complex distribution networks considering post-fault network reconfiguration. IEEE Transactions on Power Systems, 35(2), 1457–1467. https://doi.org/10.1109/TPWRS.2019.2936543
- Memarzadeh, G. and Keynia, F. (2020). A new index‐based method for optimal DG placement in distribution networks. Engineering Reports, 2(10), e12243. https://doi.org/10.1002/eng2.12243
- Mi, J., Lu, N., Li, Y. -F., Huang, H. -Z., and Bai, L. (2021). An evidential network-based hierarchical method for system reliability analysis with common cause failures and mixed uncertainties. Reliability Engineering & System Safety, 220, 108295. https://doi.org/10.1016/j.ress.2021.108295
- Myhre, S. F., Fosso, O. B., Gjerde, O., and Heegaard, P. E. (2021). Reliability assessment of distribution systems including microgrids. arXiv preprint arXiv, 2111(07674).
- Ostovar, S., Esmaeili-Nezhad, A., Moeini-Aghtaie, M., and Fotuhi-Firuzabad, M. (2021). Reliability assessment of distribution system with the integration of photovoltaic and energy storage systems. Sustainable Energy, Grids and Networks, 28, 100554. https://doi.org/10.1016/j.segan.2021.100554
- Prajapati, V. K. and Mahajan, V. (2021). Reliability assessment and congestion management of power system with energy storage system and uncertain renewable resources. Energy, 215, 119134. https://doi.org/10.1016/j.energy.2020.119134
- Puri, K. R., Subba, A. B., Pelden, K., and Chhetri, R. (2020). Reliability assessment of distribution system through cost analysis. International Journal of Scientific Research and Engineering Development (IJSRED), 3(Issue4), 560–565.
- Tiwary, A. (2019). Reliability evaluation of radial distribution system–a case study. Reliability: Theory & Applications, 14(4), 9–13.
- Tiwary, A. and Tiwary, S. (2020). Evaluation of customer orientated indices and reliability study of electrical feeder system. Reliability: Theory & Applications, 15(3), 36–43.
- Tiwary, A. & Tiwary, S. (2021). Evaluation of reliability indices of Roy billinton test system (RBTS) Bus-2 distribution system for educational purpose. Reliability: Theory & Applications. 16(1), 61.
- Tur, M. R. (2020). Reliability assessment of distribution power system when considering energy storage configuration technique. IEEE Access, 8, 77962–77971. https://doi.org/10.1109/ACCESS.2020.2990345
- Zheng, H., Cheng, Y., Gou, B., Frank, D., Bern, A., and Muston, W. (2012). Impact of automatic switches on power distribution system reliability. Electric Power Systems Research, 83(1), 51–57.