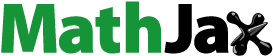
Abstract
Scheduling problems should not only focus on minimizing completion time, but other performances, such as minimizing energy consumption, are urgent to investigate. Many companies and researchers are competing to develop various methods to solve this problem. One problem that has received much attention lately is the energy-efficient Hybrid Flow Shop Scheduling Problem (HFSP). The HFSP problem is also popularly called the flexible flow shop problem. This research provides a comprehensive review of articles that discuss energy-efficient HFSP scheduling to determine the trend of energy-efficient HFSP research and research gaps for future research interests. This review uses a systematic literature review method. The total articles reviewed in this study are 90 articles from January 2008 - December 2022. The articles are classified by year, country, journal/conferences, publisher, objective function, and method. The analysis results show that the trend of energy-efficient HFSP research continues to increase. In addition, the multi-objective problem is the dominating problem in energy-efficient HFSP scheduling problems. The study also presents a gap research analysis and a review of future research directions.
1. Introduction
Scheduling is the management of production decisions that affect manufacturing activities, particularly the continuity of production (X. Li et al., Citation2010). Many researchers are interested in scheduling, and one of the most widely discussed cases in scheduling is flow shop scheduling (Han et al., Citation2022; Utama, Citation2021a). Scheduling is used to manage jobs completed on
machines with a single machine at each stage in the same sequence (Utama et al., Citation2020; Utama, Citation2021b; Widodo & Utama, Citation2020). The hybrid flow shop scheduling problem (HFSP)is an alternative to the traditional flow shop scheduling problem (Ruiz & Vázquez-Rodríguez, Citation2010). According to some sources, the HFSP problem is also a flexible flow shop (L. Tang et al., Citation2005). The HFSP problem is a flow shop scheduling problem with multiple machines at each stage (Behnamian & Ghomi, Citation2011; H. Qin et al., Citation2022; Qin, Han, Liu, et al., Citation2022). The HFSP is classified as a Non-Polynomial Hard (NP-Hard) problem (Fernandez-Viagas et al., Citation2019).
The depletion of fuel reserves and rising energy consumption has recently prompted practitioners and researchers to focus on reducing energy consumption in the manufacturing sector (Utama & Widodo, Citation2021; Utama et al., Citation2019). It cannot be separated from the manufacturing sector, which consumes the most electricity, emits the most pollutants, and uses the most fuel (Utama et al., Citation2018; Utama, Citation2019). Because they are related to regulating production jobs and machines, energy-efficiency problems in the manufacturing sector are closely related to scheduling. Industries with an HFSP production system employ many machines and workers (Fernandez-Viagas et al., Citation2019; Tosun et al., Citation2020). Consequently, the issue of energy consumption cannot be avoided, and the problem of efficient energy is becoming increasingly urgent in the industrial world (Utama, Citation2022; Utama, Ibrahim, et al., Citation2022). Hence, Companies can reduce energy consumption through proper HFSP scheduling management (Zuo et al., Citation2021).
Researchers have published several literature review articles addressing the HFSP scheduling issue. Ribas, et al (Ribas et al., Citation2010). reviewed articles on HFSP scheduling published from 1995 to 2010. The articles were categorized based on machine and job characteristics. Furthermore, Morais and Perassoli Boiko (Morais & Perassoli Boiko, Citation2013) reviewed 72 articles published between 1991 and 2013. HFSP Analysis with setup considerations is covered in their article. Tosun, et al (Tosun et al., Citation2020). reviewed 219 articles published between 2010 and Citation2019. Their article reviews HFSP scheduling articles, mainly mapping metaheuristic procedures in HFSP solutions. Several literature reviews on efficient energy scheduling have been published, including Gahm, et al (Gahm et al., Citation2016), which discuss three dimensions of energy coverage, energy supply, and energy demand. Gao, et al (Gao et al., Citation2020). examined production scheduling for intelligent manufacturing systems with various energy-related constraints and objectives. Fernandes, et al (Fernandes et al., Citation2022). Recently examined energy-efficient scheduling in the Job Shop Manufacturing System. Unfortunately, Since the article’s publication, no more comprehensive study has been conducted on energy-efficient HFSP.
Based on literature review articles that discuss comprehensive HFSP studies, to the best of our knowledge, there needs to be a comprehensive study on energy-efficient HFSP. Many researchers interested in the energy-efficient HFSP require more insight into this problem’s trends and research gaps of this problem. Therefore, this article provides a comprehensive review of energy-efficient HFSP. A systematic literature review (SLR) is applied to provide an analysis, summary, and information on research on energy-efficient HFSP scheduling. In addition, this research is expected to provide insight for researchers who want to expand the topic of energy-efficient HFSP. This study also presents opportunities and future research directions on energy-efficient HFSP problems.
The following structure of the paper is presented to answer the objectives of this study: The SLR methodology used in energy-efficient HFSP is presented in Section 2. Then, Section 3 presents an analysis of problem types, variants, optimization tools, and objective functions for the energy-efficient HFSP problem. This section also summarizes and discusses gaps in the literature and future research directions. Finally, the conclusions are presented in Section 4.
2. Methods
According to Kitchenham and Charters (Kitchenham & Charters, Citation2007), there are three primary stages to a systematic review: planning, conducting, and reporting (See Figure ). The planning stage is divided into three sub-phases. (a) assess the need for an SLR; (b) develop research questions; and (c) review protocol development.
Figure 1. SLR flow modified from Kitchenham and Charters (Kitchenham & Charters, Citation2007).

SLR is implemented in four sub-steps during the conducting stage. First, the review includes (a) finding the studies using the appropriate search technique, (b) selecting the studies, (c) extracting information from the studies, and (d) data analysis and discussion. Finally, the SLR stage was concluded with reporting presenting findings and conclusions. Each stage is described below.
The first sub-section in this section analyzes the need for an SLR. It is presented in the introduction, which discusses the context and importance of SLR energy-efficient HFSP problems. Determine Research Questions (RQ) are specified in the second sub-section so that the SLR focuses on presenting a review based on the RQ. The RQ defined in this SLR is shown in Table . This demonstrates that 4 RQs are used in the SLR.
Table 1. Research questions of the systematic review on energy efficient HFSP
The protocol review is the final stage of the planning process. This stage includes choosing the database to search for articles on the topic of energy-efficient HFSP. Because of its good reputation as an indexer, the Scopus database (https://www.scopus.com) was chosen for this SLR. In addition, this study also searches for open-access articles using the Google Scholar search engine (https://scholar.google.com).
For articles reviewed, several inclusion criteria are set, including only full-text articles in English published in journals and conferences. The inclusion criteria are met by research that describes or proposes a model or procedure for solving energy-efficient HFSP. Exclusion criteria for this study include studies that do not describe or propose a model or procedure for solving energy-efficient HFSP. The inclusion and exclusion criteria for energy-efficient HFSP SLRs are listed in Table .
Table 2. Inclusion/Exclusion criteria on energy efficient HFSP
The first sub-section of the Conducting SLR stage is the search strategy used to find significant articles on energy-efficient HFSP problems. Defining keywords is the first step in the search strategy to answer RQ. Next, these keywords are used to search the Scopus database and the Google Scholar search engine, as determined by the inclusion and exclusion criteria. The keywords used in the search strategy are “Green” OR “Energy”AND “Efficient” OR “Aware” OR “Consumption” AND “Hybrid” OR “Flexible” AND “Flow” AND “Shop” AND “Scheduling”.
The next stage is the selection of articles based on the search strategy stage. In phase 1, 465 articles were collected based on searches in the Scopus database and the Google Scholar search engine. However, 123 papers were duplicated, resulting in a total of 342 articles after the duplication was removed. Furthermore, after reading the title and publication abstract, 157 studies were discovered after the inclusion and exclusion criteria were applied. The final stage is eligibility based on full-text articles, showing that 90 articles were extracted. Figure shows the total number of publications chosen for each phase.
Based on selection papers, seventy-seven (86%) of the total articles were published in journals, with the remaining thirteen (14%) articles published in conferences. Table shows the distribution of papers published by the journal. The International Journal of Production Research, Swarm and Evolutionary Computation, and Journal of Cleaner Production contributed the most, with six articles. In addition, five articles are contributed by IEEE Access, and four papers from the Computers & Industrial Engineering.
Table 3. the distribution of papers published by the journal
The third sub-step is data extraction, based on 90 articles chosen in the identified studies and selection stage. The literature review was organized by country, year, journal article or conference type, type of optimization (single-objective and multi-objective), optimization tool, and objective function problem. This analysis is based on the RQ defined in Table , and Figure . depicts the classification scheme of the literature review on energy-efficient HFSP scheduling.
This sub-stage concludes with data analysis and discussion. This SLR data analysis uses the frequency distribution presented in each Literature Review classification scheme. This section also presents research mapping based on the established Literature Review classification scheme.
This stage of the SLR concludes with a description of the findings and conclusions. It is presented to answer the RQ that has been set. In addition, this section also presents gaps and further research for energy-efficient HFSP.
3. Results and discussion
3.1. Paper distribution based on year and country of publication
This paper reviews articles from January 2008 to December 2022, as shown in Figure . It demonstrates that research on energy-efficient HFSP problems is increasing yearly. Furthermore, it is the most published article on energy-efficient HFSP in 2022, with contributed 18 papers. It indicates that researchers are increasingly interested in the subject. Furthermore, in 2018–2021, 9, 15, 14 and 15 articles were published, respectively, whereas from 2008 to 2012, each year contributed 1 article.
This SLR presents the distribution of articles by country, as shown in Figure , to determine the distribution of research in each country. Researchers from various countries have contributed to the study of energy-efficient HFSP. A total of 16 countries have contributed to research on energy-efficient HFSP, with Chinese authors publishing the most articles in the last 14 years, with 64 papers. Furthermore, Germany has published the most articles on this topic, with five, followed by Taiwan with four, Malaysia with three papers, and Iran and Indonesia contributing two Articles. On the other hand, Belgium, France, Hong Kong, Italy, Japan, Mexico, Palestine, the United Kingdom, the United States, and Turkey are among the countries that have only published one article in the last 14 years.
3.2. Literature review
This study contains 90 articles, all classified into predefined categories using the classification scheme defined in section 2. Table breaks down the articles by problem, variant, and optimization tool, while Table details the objective function. We identified seven variations of energy-efficient HFSP based on 90 articles, including Energy-Efficient Hybrid Flow Shop Scheduling Problem Classic (EEHFSP), Energy-Efficient Reentrant Hybrid Flow Shop Scheduling Problem (EERHFSP), Energy-Efficient Resource-Constrained Hybrid Flow Shop Scheduling Problem (EERCHFSP), Energy-Efficient Fuzzy Hybrid Flow Shop Scheduling Problem (EEFHFSP), Energy-Efficient Distributed Hybrid Flow Shop Scheduling Problem (EEDHFSP), Energy-Efficient Distributed Reentrant Hybrid Flow Shop Scheduling Problem (EEDRHFSP), and Energy-Efficient Blocking hybrid flow shop scheduling (EEBHFSP).
Table 4. Classification of articles based on problem, variant, optimization tools
Table 5. Classification of articles by objective function
EEHFSP is an energy-efficient hybrid flow shop problem in which a set of jobs are processed in a series of stages in a manufacturing environment to optimize the energy problem (X. Liu et al., Citation2008). The characteristics of this problem are that the number of processing stages m is at least 2, each stage has at least one machine, at least one of the stages has machines in parallel, and all jobs are processed following the same production flow (Schulz, Citation2019; Tian et al., Citation2019). EERHFSP is an energy-efficient hybrid flow shop problem that combines EEHFSP and reentrant scheduling problems. In this problem, a set of jobs follow the same processing sequence at each stage and often repeat processing activities at the same stage and sequence (Geng et al., Citation2020).
In the EERCHFS variant, this problem is an extension of the EEHFSP problem that considers constraints such as limited resources and machine breakdowns (Tao et al., Citation2021). This variant cannot be separated from the various dynamic events that can occur in the actual production process in the manufacturing industry. The EEFHFSP problem variant is a variant of the EEHFSP problem that incorporates fuzzy processing time (M. Li et al., Citation2021). Furthermore, the EEDHFSP problem is an extension of the EEHFSP problem, which considers processing in a factory and the allocation of work to several factories (Ling-Li et al., Citation2009). This variant is also extended with a reentrant scheduling problem that repeats processing activities at the same stage and sequence, so this problem is called EEDRHFSP (Dong & Ye, Citation2022). The last variant is EEBHFSP which accommodates the EEHFSP problem with blocking characteristics that assume inter-machine buffers are unavailable and the storage capacity between stages is considered zero (Qin, Han, Zhang, et al., Citation2022).
The following subsection describes a literature review based on single and multi-objective problems. Some abbreviations used to identify the objective function of the energy-efficient HFSP problem are as follows:
3.2.1. Single-objective
This section presents a review of research with single-objective problems. All the studies (26 papers) used the objective function of minimizing total energy consumption. Several procedures are proposed to solve this problem. On heuristic procedures, Ding et al., (Citation2014). investigated the EEHFSP problem by proposing the Lagrangian Relaxation Algorithm procedure. The Iterative Greedy Algorithm is also proposed by Qin, et al (Citation2022). to solve the EEBHFSP problem.
On the exact procedure, four studies proposed the Mixed Integer Linear Programming procedure to solve the EEHFSP problem. These studies are presented by Bruzzone et al. (Citation2012), Xu, et al. (Citation2014), Schulz (Citation2018), and Wang, et al. (Citation2018). Researchers also offer hybrid procedures to solve EEHFSP problems. Liu, et al. (Citation2008). and Xing, et al. (Citation2019). have proposed a hybrid procedure that integrates a Mixed Integer Non-Linear Programming-Genetic Algorithm. The combination of Mixed Integer Linear Programming and an iterative greedy algorithm was also proposed by Qin, et al. (Citation2021). Utama and Primayesti (Citation2022) developed a hybrid procedure based on Aquila Optimizer Algorithm and Local search. Utama, et al. (Citation2022). also proposed An integrated procedure of Archimedes optimization and Local search.
The metaheuristic procedure is the most widely applied procedure for solving single-objective problems. In the EEHFSP problem, the Genetic Algorithm is researchers’ most widely used procedure. Researchers who utilize this procedure for EEHFSP are Schulz (Citation2019), Tian, et al. (Citation2019), Meng, et al. (Citation2019), Kong, et al. (Citation2020), Huang, et al. (Citation2017), Luo, et al. (Citation2019), Lu and Qiao. (Citation2022), and Chen, et al. (Citation2022). Other metaheuristic procedures were also proposed to solve the EEHFSP problem, such as Simulated Annealing proposed by Keller, et al. (Citation2015), and Memetic Algorithm proposed by Wang, et al. (Citation2020). Jabari and Hasan (Citation2021) applied Firefly Algorithm, and Li, et al. (Citation2023). developed the Cooperative Coevolutionary Algorithm to solve EEHFSP. Another variant of EEDHFSP was also investigated by Ling-Li, et al. (Citation2009). by proposing a Particle Swarm Optimization procedure.
3.2.2. Multi-objective
This section presents research on energy-efficient HFSP with multi-objective problems. Researchers have proposed a wide variety of energy-efficient HFSP problems and optimization procedures. In the heuristic procedure, the EEDHFSP problem was investigated by Lu, et al. (Citation2022). by applying a procedure based on an iterated greedy algorithm to minimize makespan and total energy consumption. Adaptive selection multi-objective optimization algorithm with preference was developed by Liu, et al. (Citation2022). to investigate the EEHFSP problem with the objective functions of minimizing makespan, minimizing total energy consumption, and maximizing the excess value of adjustment time.
Five Multi-objective research utilizes an exact procedure based on Mixed Integer Linear Programming to solve the EEHFSP problem. This procedure has been used by Liu, et al. (Citation2020), Chen, et al. (Citation2020), and Öztop, et al. (Citation2020). to minimize makespan and minimize total energy consumption. Biel, et al. (Citation2018). investigated the objective functions of minimizing energy cost and flow time with the Mixed Integer Linear Programming procedure. The same procedure was used by Schulz, et al. (Citation2020). to minimize tardiness and energy cost.
The metaheuristic procedure is a popular procedure used to solve this problem. In addition, the EEHFSP problem is a problem that dominates this research. Most of the research focuses on minimizing makespan and minimizing total energy consumption. Various metaheuristic procedures have been proposed to optimize these objective functions such as Ant Colony System (Du et al., Citation2011), Decomposition Algorithm (Zhang, Pan, Gao, Meng, et al., Citation2019), Artificial Bee Colony (Zhang, Pan, Gao, Li, et al., Citation2019), Differential evolution algorithm (L. Wang et al., Citation2021), Artificial immune Algorithm (B. -H. Zhou & Gu, Citation2021), and Evolutionary Algorithm (Gong et al., Citation2020). Other procedures such as Firefly Algorithm (Rashid & Osman, Citation2020), Genetic Algorithm (C. Lu et al., Citation2018; Cheng et al., Citation2020; J. Yan et al., Citation2014, Citation2016; Lian et al., Citation2021; Shi et al., Citation2019), Memetic Algorithm (Geng et al., Citation2021), Moth Flame Optimization (Rashid et al., Citation2022), Simulated Annealing (Dai et al., Citation2013), Particle Swarm Optimization (D. Tang et al., Citation2016), Scatter search algorithm (Zeng et al., Citation2022), and Non-dominated Sorting Genetic Algorithm II (Duan et al., Citation2022; J. -H. Yan et al., Citation2014; Y. Liu et al., Citation2018; Yaurima-Basaldua et al., Citation2018) have also been offered. Recently, advanced procedures such as the Whale optimization algorithm (Yankai et al., Citation2021), Teaching-learning based optimization (Song, Citation2021), and Tiki-Taka Algorithm (Ab Rashid & Mu’tasim, Citation2022) are also proposed to solve the EEHFSP problem with the objective functions of minimizing makespan and total energy consumption.
Meanwhile, Greywolf Optimization was developed by Lu, et al. (Citation2019). to minimize the makespan, minimize total energy consumption, and minimize noise pollution. Zhou, et al. (Citation2019). proposed the imperialist competitive Algorithm to minimize makespan and total energy consumption. Li, et al. (Citation2019). also proposed the same Algorithm to optimize three objective functions: minimizing makespan, minimizing tardiness, and minimizing total energy consumption. Minimized tardiness and total energy consumption are investigated with Novel Teaching-Learning based Optimization Algorithm (Lei et al., Citation2017), and Non-dominated Sorting Genetic Algorithm II (Nasiri et al., Citation2018). Non-dominated Sorting Genetic Algorithm II was also proposed by Liu and Huang (Citation2014) to minimize total energy consumption and minimize carbon footprint. Zeng, et al. (Citation2018). also used the same procedure to minimize total energy consumption and material wastage. The problem of minimizing tardiness and minimizing Non-processing energy was implemented by Jiang and Zhang (Citation2019) by applying the Evolutionary Algorithm procedure. The same procedure is also applied to the optimization of three objective functions: minimizing tardiness, minimizing total energy consumption, and minimizing Average sojourn time. Two procedures were offered to solve the EEHFSP problem to minimize total energy consumption and production cost. Hasani and Hosseini (Citation2020) developed Non-Dominated Sorting Genetic Algorithm II, and Zhang, et al (Citation2021). implemented the Genetic algorithm procedure.
On the EEDHFSP problem, Wang, et al. (Citation2021). and Jiang, et al. (Citation2021). have developed Evolutionary Algorithm procedures to minimize makespan and total energy consumption. The objective functions of minimizing tardiness and minimizing total energy consumption have also been investigated by Wang and Wang (Citation2022), and Shao, et al. (Citation2022a). by implementing the Memetic Algorithm. Shao, et al. (Citation2022b). also applied the Memetic algorithm procedure to solve three objective functions. The objective functions are to minimize tardiness, energy cost, and carbon emission. In the EEDRHFSP problem, metaheuristic procedures were also applied to solve the problem. Geng, et al. (Citation2022). used Artificial Bee Colony to minimize makespan and total energy consumption. Furthermore, Dong and Ye (Citation2022) used Salp Swarm Algorithm with three objective functions: minimizing the makespan, minimizing total energy consumption, and minimizing carbon emission.
Meanwhile, on the EEFHFSP problem, Li, et al. (Citation2021). applied the Bee Colony Algorithm procedure to minimize makespan and total energy consumption. In the EERHFSP problem, Geng, et al. (Citation2019). implemented the Multiverse algorithm with three objective functions: minimizing the makespan, tardiness, and total energy consumption. Geng, et al. (Citation2020). offered an Ant Lion Optimization procedure to minimize makespan and total energy consumption. With the same goal, Xu, et al. (Citation2022). developed the Moth Flame Optimization procedure. Meanwhile, in the EERCHFSP problem, Tao, et al. (Citation2021). developed the Imperialist competitive Algorithm to minimize makespan and total energy consumption.
Several hybrid procedures have also been proposed to solve this problem. In the hybrid procedures, all studies address the EEHFSP problem. The objective functions minimizing makespan and minimizing total energy consumption are the popular objective functions used in this research. The procedures proposed to solve this problem include Mixed Integer Programming-constructive heuristic-tabu search-ant colony optimization (S. Wang et al., Citation2020). Mixed Integer Linear Programming Model-Cooperative Memetic Algorithm (J. -J. Wang & Wang, Citation2021), and Mixed Integer Linear Programming-Simulated Annealing-Genetic Algorithm (Dai et al., Citation2014) procedures have also been proposed. Differential Evolution-NEH was proposed by Zhou and Liu (Citation2019) to minimize total energy consumption and minimize Total Delivery Penalty. Optimization of three objective functions was also investigated by Shen, et al. (Citation2021), including minimizing the makespan, minimizing energy cost, and minimizing labor cost. They proposed a Mixed Integer Linear Programming-Genetic Algorithm integration procedure.
3.3. Analysis
This section presents an analysis of the findings based on the assigned RQ. The results of this analysis were obtained by identifying previous studies presented in the literature review section. The details of the analysis and findings are presented in the following subsections.
3.3.1. Analysis of the type of problem in energy-efficient HFS
Based on RQ1, this section examines the problems encountered in energy-efficient HFSP research. Figure depicts the distribution of energy-efficient HFSP research by problem type, revealing that 73 percent (66 articles) of the 90 articles are a type of multi-objective problem. This study’s remaining 27% (24 articles) are about single-objective issues. These findings indicate that multi-objective problems are in higher research than single-objective problems. Multi-objective problems are widely investigated because the decision maker can select an optimal decision involving two or more objective functions. These decisions can accommodate trade-offs between the two or more objective functions under investigation. Unlike the single-objective problem, it only focuses on optimizing an objective function while ignoring other objective functions. Therefore, multi-objective problems have received much attention from researchers.
Figure shows the distribution paper for each year based on the type of problem. These findings indicate that there has been an increase in research on energy-efficient HFSP from 2008 to 2022. Even since 2018, the number of multi-objective problems has increased significantly compared to single-objective problems.
3.3.2. HFSP energy efficient variant analysis
This section examines the variant energy-efficient HFSP in order to answer RQ2. The distribution of the energy-efficient HFSP variant is shown in Figure , and it shows that 90 articles discuss EEHFSP problem variants, with 22 articles discussing single-objective problem types and 53 articles discussing multi-objective problem types. Furthermore, research on the EEDHFSP and EERHFSP variants each contributed seven and three articles. Problem EEDRHFSP contributes two papers. In contrast, EEBHFSP, EERCHFSP, and EEFHFSP variants each contributed one. This finding demonstrates that the EEHFSP variant dominates the energy-efficient HFSP problem.
3.3.3. HFSP energy efficient optimization tool analysis
Based on RQ3, this section examines the procedures used in research on energy-efficient HFSP. Figure depicts the distribution of research on the procedure for solving the energy-efficient HFSP problem. It demonstrates that the metaheuristics procedure dominates the procedure for solving the energy-efficient HFSP problem. Distribution optimization tools on single-objective problems are presented in Figure . The results show that 13 different procedures have been offered to solve this problem. The genetic Algorithm is the most widely used metaheuristic procedure by researchers to solve the energy-efficient HFSP problem. We recorded eight studies (34%) utilizing Genetic Algorithms to solve the problem of single-objectives. The exact procedure occupies the second position by contributing four papers (17%).
According to the review on the type of multi-objective problem in section 3.2.2, 36 procedures are proposed to solve multi-objective problems. The distribution of optimization tools on multi-objective problems is summarized in Figure . The results show that the metaheuristic procedure based on the Non-dominated Sorting Genetic Algorithm II procedure is the most applied procedure to solve the energy-efficient HFSP problem. We recorded ten studies (15%) utilizing this procedure to solve the energy-efficient HFSP problem. The Genetic Algorithm metaheuristic procedure comes second with seven studies (11%), and the Evolutionary Algorithm contributes six papers (9%). In addition, the exact procedure is also widely implemented by researchers to solve the energy-efficient HFSP problem. We note that five articles (8%) propose this procedure.
3.3.4. Analysis of objective function on energy-efficient HFS
This section examines which objective function is frequently used to answer RQ4 in energy-efficient HFSP. The distribution of objective function on energy-efficient HFSP is presented in Figure . In single-objective problems, solve the energy-efficient HFSP problem using the objective function of total energy consumption (TEC). We noted that 24 paper used this objective function. It shows that current studies still focus on minimizing total energy consumption. Previous studies did not investigate other objective functions, such as minimizing energy cost and peak power consumption.
Meanwhile, various objective functions for multi-objective problems have been presented (see Table ). The distribution of objective functions on multi-objective problems is presented in Figure . These results show that bi-objective problems with objective functions of minimizing makespan and total energy consumption are researchers’ most widely investigated objective functions. Thirty-nine papers (59%) discussed this objective function. Some studies that address this issue are Du, et al. (Citation2011). proposed the Ant Colony System procedure, and Tang, et al. (Citation2016). applied Particle Swarm Optimization. More recently, Duan, et al. (Citation2022). applied the Non-dominated sorting genetic algorithm II procedure to solve this problem. All three studies addressed the EEHFSP problem with the same objective but different procedures. These results show that researchers widely investigate the trend of utilizing advanced procedures.
The bi-objective problem with the objective functions of minimizing tardiness and total energy consumption is in second place by contributing four papers (6%). We record various proposed procedures such as Novel Teaching-Learning based Optimization Algorithm proposed by Lei, et al. (Citation2017), Non-dominated Sorting Genetic Algorithm II developed by Nasiri, et al. (Citation2018), and Memetic Algorithm applied by Wang and Wang (J. -J. Wang & Wang, Citation2022) and Shao, et al. (Citation2022a). As before, studies addressed the EEHFSP problem with the same objective but different procedures. Furthermore, the bi-objective minimizes makespan and Carbon Footprint, and the triple objective with the objective functions minimizes the makespan, minimizes tardiness, and minimizes total energy consumption, each accounting for two papers (3%).
3.4. Gaps and opportunities research
This section discusses research gaps in energy-efficient HFSP and future directions. Table depicts Characteristics of research on energy-efficient based on variants, optimization tools, single and multi-objective problem. It shows that multi-objective EEHFSP problems with metaheuristic procedures dominate the energy-efficient HFSP research of the published studies. This problem accounts for 44 papers (46%). The single-objective EEHFSP problem with metaheuristic procedures ranked second with 13 papers (14%). The multi-objective EEHFSP problem with metaheuristic procedures ranked third, contributing seven papers (7%).
Table 6. Characteristics research on energy efficient based on variants, optimization tools, single and multi-objective problem
Researchers are currently very excited about the problem of energy-efficient HFSP. Gaps and summaries of previous research on this topic are required to aid in developing new projects in future research. The gap analysis and future research plans are summarized below:
The EEHFSP problem dominates the energy-efficient HFSP problem. Unfortunately, researchers are paying less attention to the problems of EEDHFSP, EERHFSP, EERCHFSP, EEDRHFSP, EEBHFSP and EEFHFSP. As a result, more research into the issues of EEDHFSP, EERHFSP, EERCHFSP, EEDRHFSP, EEBHFSP and EEFHFSP are required.
In single-objective problems, it is necessary to explore objective functions such as cost because this objective function can be integrated with other objective functions such as energy cost, penalty cost, and waste cost.
Energy efficient HFSP currently models processing time and idle time as one of the parameters that affect energy consumption. However, when modeling energy-efficient HFSP, all aspects of production, including setup and removal times, must be considered. As a result, to reduce energy consumption, energy-efficient HFSP must model more comprehensive factors. The more aspects of energy-efficient HFSP that can be modeled, the more likely it is that energy efficiency and consumption can be improved.
The deterministic processing time dominates the energy-efficient HFSP challenge. Therefore, fuzzy and stochastic time characteristics are the focus of future research.
Due to sustainability concerns, researchers are encouraged to use objective functions considering economic, social, and environmental factors. In economics, minimizing total energy consumption (from an environmental standpoint) and turnaround time are the most critical considerations in the problem of energy-efficient HFSP. On the other hand, the issue of energy-efficient HFSP ignores social aspects. As a result, more research is required to address the three dimensions of sustainability (economic, social, and environmental) and HFSP issues.
Most academics provide multi-objective problems. Researchers are less concerned with the issue of energy-efficient single-objective HFSP. However, single-objective problems are less appealing. Several objective functions from economic aspects, such as minimizing turnaround time, delays, and idle time, as well as environmental aspects, such as energy and emission minimization, can be combined to minimize costs. In the case of the HFSP problem, the decision maker can use a single objective solution without seeking a non-dominant solution.
Regarding energy-efficient HFSP problems, the multi-objective problem rule is a hot topic among researchers. The most critical objectives of the energy-efficient HFSP problem are reducing total energy consumption and minimizing the makespan. Traditional objective functions, such as minimizing delays and flow times, are less critical in multi-objective problems. Other goals in multi-objective issues, such as lowering greenhouse gas emissions, were not investigated. Most researchers focus on developing a more effective method for solving the energy-efficient HFSP problem. The relationship between objective functions should be investigated and analyzed in greater depth in multi-objective problems. Furthermore, research into objective functions of more than two objectives is lacking. As a result, investigating more than two objectives should concern future research directions.
Based on the previous section’s analysis, the metaheuristic procedure in scheduling is an efficient and effective method for solving energy efficiency scheduling problems. Large-scale problems can be solved effectively and efficiently using this method. To find non-dominant solutions, it is, therefore, necessary to improve the development of high-quality advanced metaheuristic algorithms for single and multiple-purpose problems. Furthermore, several local search operators and heuristic procedures can be used to improve the Algorithm’s quality in solving the energy-efficient HFSP scheduling problem. As a result, developing a hybrid procedure that combines heuristic and metaheuristic procedures is required for the energy-efficient HFSP problem.
Research on energy-efficient HFSP is dominated by research considering new jobs arriving simultaneously. Dynamic job arrivals have received little attention. Further research into energy-efficient HFSP is required to address the future dynamic job problem.
Some industries have unique energy-efficient HFSP characteristics. As a result, decision-makers are more concerned with employing models that are both practical and simple to implement. As a result, research focusing on specific industries where models and algorithms can be applied is critical.
4. Conclusion and future work
This article attempts to review the research that addresses the energy-efficient HFSP problem that was published in 2008–2022. Ninety-six articles were comprehensively reviewed based on HFSP variants, problem types, objective functions, and optimization procedures described in this study. The review results show that researchers often investigate the multi-objective problem type of energy-efficient HFSP problem. Meanwhile, the EEHFSP variant is also a variant that researchers widely investigate. In addition, the metaheuristic procedure is the most proposed procedure to solve this problem. In single-objective problems, Genetic Algorithm is the most popular procedure used. Non-dominated Sorting Genetic Algorithm II is the most widely applied procedure to solve multi-objective problems. In the objective function, minimizing total energy consumption is the only procedure researchers use. Meanwhile, makespan minimization and total energy consumption are popular objective functions used in multi-objective problems.
Some future research directions are also presented in this paper. Future research directions that need to be explored are described as follows: (1) EEDHFSP, EERHFSP, EERCHFSP, EEDRHFSP, EEBHFSP and EEFHFSP problems need more attention because these problems are commonly encountered in manufacturing environments; (2) processing time characteristics need to consider fuzzy and stochastic data characteristics; (3) sustainability issues encourage further research considering objective functions involving social, economic and environmental aspects; (4) single-objective problems need to consider the cost function to accommodate the objective functions of energy cost, penalty cost, tardiness cost, and waste cost; (5) in multi-objective, research on objective functions of more than two objective function needs to be done; (6) the continuous development of computer technology encourages researchers to develop advanced procedures based on metaheuristics; and (7) research that considers dynamic job arrivals also needs to be investigated in energy efficient HFSP problems.
Disclosure statement
No potential conflict of interest was reported by the authors.
Data Availability statement
All data generated or analyzed during this study are included in this article.
Additional information
Funding
References
- Ab Rashid, M. F. F., & Mu’tasim, M. A. N. (2022). A novel Tiki-Taka algorithm to optimize hybrid flow shop scheduling with energy consumption. Engineering Applied Science Research, 49(2), 189–37.
- Behnamian, J., & Ghomi, S. F. (2011). Hybrid flowshop scheduling with machine and resource-dependent processing times. Applied Mathematical Modelling, 35(3), 1107–1123. https://doi.org/10.1016/j.apm.2010.07.057
- Biel, K., Zhao, F., Sutherland, J. W., & Glock, C. H. (2018). Flow shop scheduling with grid-integrated onsite wind power using stochastic MILP. International Journal of Production Research, 56(5), 2076–2098. https://doi.org/10.1080/00207543.2017.1351638
- Bruzzone, A. A., Anghinolfi, D., Paolucci, M., & Tonelli, F. (2012). Energy-aware scheduling for improving manufacturing process sustainability: A mathematical model for flexible flow shops. CIRP Annals, 61(1), 459–462. https://doi.org/10.1016/j.cirp.2012.03.084
- Chen, T. L., Cheng, C. Y., & Chou, Y. H. (2020). Multi-objective genetic algorithm for energy-efficient hybrid flow shop scheduling with lot streaming. Annals of Operations Research, 290(1), 813–836. https://doi.org/10.1007/s10479-018-2969-x
- Cheng, Q., Liu, C., Chu, H., Liu, Z., Zhang, W., & Pan, J. (2020). A new multi-objective hybrid flow shop scheduling method to fully utilize the residual forging heat. EEE Access, 8, 151180–151194. https://doi.org/10.1109/ACCESS.2020.3017239
- Chen, W., Wang, J., Yu, G., & Hu, Y. (2022). Energy-efficient hybrid flow-shop scheduling under time-of-use and ladder electricity tariffs. Journal, Type of Article, 12(13), 6456. https://doi.org/10.3390/app12136456
- Dai, M., Tang, D., Giret, A., Salido, M. A., & Li, W. D. (2013). Energy-efficient scheduling for a flexible flow shop using an improved genetic-simulated annealing algorithm. Robotics and Computer-Integrated Manufacturing, 29(5), 418–429. https://doi.org/10.1016/j.rcim.2013.04.001
- Dai, M., Tang, D., Zhang, H., & Yang, J. (2014, May). Energy-aware scheduling model and optimization for a flexible flow shop problem. Proceedings of the 26th Chinese Control and Decision Conference (2014 CCDC), Changsha, China (pp. 323–328). IEEE.
- Ding, X. L., Zhu, J., & Liu, C. (2014). Lagrangian relaxation algorithms for hybrid flow-shop scheduling problems with energy saving. In Wen-Pei S. (Ed.), Advanced Materials Research (Vol. 997, pp. 821–826). Trans Tech Publ.
- Dong, J., & Ye, C. (2022). Green scheduling of distributed two-stage reentrant hybrid flow shop considering distributed energy resources and energy storage system. Computers & Industrial Engineering, 169, 108146. https://doi.org/10.1016/j.cie.2022.108146
- Duan, J., Feng, M., & Zhang, Q. (2022). Energy-efficient collaborative scheduling of heterogeneous multi-stage hybrid flowshop for large metallic component manufacturing. Journal of Cleaner Production, 375, 134148. https://doi.org/10.1016/j.jclepro.2022.134148
- Du, B., Chen, H., Huang, G. Q., & Yang, H. (2011). Preference vector ant colony system for minimising make-span and energy consumption in a hybrid flow shop. In Lihui, W., Amos H. C., Ng., & Kalyanmoy, D. (Eds.), Multi-objective evolutionary optimisation for product design and manufacturing (pp. 279–304). Springer.
- Fernandes, J. M. R. C., Homayouni, S. M., & Fontes, D. B. M. M. (2022). Energy-efficient scheduling in job shop manufacturing systems: A literature review. Sustainability, 14(10), 6264. https://doi.org/10.3390/su14106264
- Fernandez-Viagas, V., Perez-Gonzalez, P., & Framinan, J. M. (2019). Efficiency of the solution representations for the hybrid flow shop scheduling problem with makespan objective. Computers & Operations Research, 109, 77–88. https://doi.org/10.1016/j.cor.2019.05.002
- Gahm, C., Denz, F., Dirr, M., & Tuma, A. (2016). Energy-efficient scheduling in manufacturing companies: A review and research framework. European Journal of Operational Research, 248(3), 744–757. https://doi.org/10.1016/j.ejor.2015.07.017
- Gao, K., Huang, Y., Sadollah, A., & Wang, L. (2020). A review of energy-efficient scheduling in intelligent production systems. Complex & Intelligent Systems, 6(2), 237–249. https://doi.org/10.1007/s40747-019-00122-6
- Geng, K., Liu, L., & Wu, Z. (2022). Energy-efficient distributed heterogeneous re-entrant hybrid flow shop scheduling problem with sequence dependent setup times considering factory eligibility constraints. Scientific Reports, 12(1), 18741. https://doi.org/10.1038/s41598-022-23144-6
- Geng, K., Ye, C., Cao, L., & Liu, L. (2019). Multi-objective reentrant hybrid flowshop scheduling with machines turning on and off control strategy using improved multi-verse optimizer algorithm. Mathematical Problems in Engineering, 2019, 1–18. https://doi.org/10.1155/2019/2573873
- Geng, K., Ye, C., Liu, L., & Liu, L. (2020). Bi-objective re-entrant hybrid flow shop scheduling considering energy consumption cost under time-of-use electricity tariffs. Complexity, 2020, 1–17. https://doi.org/10.1155/2020/8565921
- Geng, K., Ye, C., & Metawa, N. (2021). A memetic algorithm for energy-efficient distributed re-entrant hybrid flow shop scheduling problem. Journal of Intelligent & Fuzzy Systems, (Preprint), 1–21. https://doi.org/10.3233/JIFS-189838
- Gong, G., Chiong, R., Deng, Q., Han, W., Zhang, L., Lin, W., & Li, K. (2020). Energy-efficient flexible flow shop scheduling with worker flexibility. Expert Systems with Applications, 141, 112902. https://doi.org/10.1016/j.eswa.2019.112902
- Gui, L., Gao, L., & Li, X. (2020). Anomalies in special permutation flow shop scheduling problems. Chinese Journal of Mechanical Engineering, 33(1), 1–7. https://doi.org/10.1186/s10033-020-00462-2
- Han, X., Han, Y., Zhang, B., Qin, H., Li, J., Liu, Y., & Gong, D. (2022). An effective iterative greedy algorithm for distributed blocking flowshop scheduling problem with balanced energy costs criterion. Applied Soft Computing, 129, 109502. https://doi.org/10.1016/j.asoc.2022.109502
- Hasani, A., & Hosseini, S. M. H. (2020). A bi-objective flexible flow shop scheduling problem with machine-dependent processing stages: Trade-off between production costs and energy consumption. Applied Mathematics and Computation, 386, 125533. https://doi.org/10.1016/j.amc.2020.125533
- Huang, R. H., Yu, S. C., & Chen, P. H. (2017). Energy-saving scheduling in a flexible flow shop using a hybrid genetic algorithm. Journal of Environmental Protection, 8(10), 1037–1056. https://doi.org/10.4236/jep.2017.810066
- Jabari, A. N. A. A. K., & Hasan, A. J. J. T. I. (2021). Energy-aware scheduling in hybrid flow shop using firefly algorithm. Tsinghua Science and Technology, 22(1), 18–30.
- Jiang, E., Wang, L., & Wang, J. (2021). Decomposition-based multi-objective optimization for energy-aware distributed hybrid flow shop scheduling with multiprocessor tasks. Tsinghua Science and Technology, 26(5), 646–663.
- Jiang, S. L., & Zhang, L. (2019). Energy-oriented scheduling for hybrid flow shop with limited buffers through efficient multi-objective optimization. IEEE Access, 7, 34477–34487. https://doi.org/10.1109/ACCESS.2019.2904848
- Keller, F., Schönborn, C., & Reinhart, G. (2015). Energy-orientated machine scheduling for hybrid flow shops. Procedia CIRP, 29, 156–161. https://doi.org/10.1016/j.procir.2015.02.103
- Kitchenham, B., & Charters, S. (2007). Guidelines for performing systematic literature reviews in software engineering. Citeseer.
- Kong, L., Wang, L., Li, F., Wang, G., Fu, Y., & Liu, J. (2020). A new sustainable scheduling method for hybrid flow-shop subject to the characteristics of parallel machines. Institute of Electrical and Electronics EngineersAccess, 8, 79998–80009.
- Lei, D., Gao, L., & Zheng, Y. (2017). A novel teaching-learning-based optimization algorithm for energy-efficient scheduling in hybrid flow shop. IEEE Transactions on Engineering Management, 65(2), 330–340. https://doi.org/10.1109/TEM.2017.2774281
- Lian, X., Zheng, Z., Wang, C., & Gao, X. (2021). An energy-efficient hybrid flow shop scheduling problem in steelmaking plants. Computers Industrial Engineering, 162, 107683. https://doi.org/10.1016/j.cie.2021.107683
- Li, X., Deng, Z., Chen, Z., & Fei, Q. (2010). Analysis and simplification of three-dimensional space vector PWM for three-phase four-leg inverters. IEEE Transactions on Industrial Electronics, 58(2), 450–464. https://doi.org/10.1109/TIE.2010.2046610
- Li, M., Lei, D., & Cai, J. (2019). Two-level imperialist competitive algorithm for energy-efficient hybrid flow shop scheduling problem with relative importance of objectives. SwarmEvolutionary Computation, 49(September 2019), 34–43.
- Ling-Li, Z., Feng-Xing, Z., Xiao-Hong, X., & Zheng, G. (2009, June). Dynamic scheduling of multi-task for hybrid flow-shop based on energy consumption. Proceedings of the 2009 international conference on information and automation, Zhuhai/Macau, China (pp. 478–482). IEEE.
- Liu, Y., Farnsworth, M., & Tiwari, A. (2018). Energy-efficient scheduling of flexible flow shop of composite recycling. International Journal of Advanced Manufacturing Technology, 97(1–4), 117–127. https://doi.org/10.1007/s00170-018-1852-x
- Liu, C. H., & Huang, D. H. (2014). Reduction of power consumption and carbon footprints by applying multi-objective optimisation via genetic algorithms. International Journal of Production Research, 52(2), 337–352. https://doi.org/10.1080/00207543.2013.825740
- Liu, Z., Yan, J., Cheng, Q., Chu, H., Zheng, J., & Zhang, C. (2022). Adaptive selection multi-objective optimization method for hybrid flow shop green scheduling under finite variable parameter constraints: Case study. International Journal of Production Research, 60(12), 3844–3862. https://doi.org/10.1080/00207543.2021.1933239
- Liu, Z., Yan, J., Cheng, Q., Yang, C., Sun, S., & Xue, D. (2020). The mixed production mode considering continuous and intermittent processing for an energy-efficient hybrid flow shop scheduling. Journal of Cleaner Production, 246, 119071. https://doi.org/10.1016/j.jclepro.2019.119071
- Liu, M., Yang, X., Chu, F., Zhang, J., & Chu, C. (2020). Energy-oriented bi-objective optimization for the tempered glass scheduling. Omega, 90, 101995. https://doi.org/10.1016/j.omega.2018.11.004
- Liu, X., Zou, F., & Zhang, X. (2008, July). Mathematical model and genetic optimization for hybrid flow shop scheduling problem based on energy consumption. Proceedings of the 2008 Chinese control and decision conference, Yantai, Shandong (pp. 1002–1007). IEEE.
- Li, M., Wang, G. G., & Yu, H. (2021). Sorting-based discrete artificial bee colony algorithm for solving fuzzy hybrid flow shop green scheduling problem. Mathematics, 9(18), 2250. https://doi.org/10.3390/math9182250
- Li, C., Zhang, B., Han, Y., Wang, Y., Li, J., & Gao, K. (2023). Energy-efficient hybrid flowshop scheduling with consistent sublots using an improved cooperative coevolutionary algorithm. Journal, Type of Article, 11(1), 77.
- Lu, C., Gao, L., Li, X., Zheng, J., & Gong, W. (2018). A multi-objective approach to welding shop scheduling for makespan, noise pollution and energy consumption. Journal of Cleaner Production, 196, 773–787. https://doi.org/10.1016/j.jclepro.2018.06.137
- Lu, C., Gao, L., Pan, Q., Li, X., & Zheng, J. (2019). A multi-objective cellular grey wolf optimizer for hybrid flowshop scheduling problem considering noise pollution. Applied Soft Computing, 75, 728–749. https://doi.org/10.1016/j.asoc.2018.11.043
- Lu, C., Liu, Q., Zhang, B., & Yin, L. (2022). A Pareto-based hybrid iterated greedy algorithm for energy-efficient scheduling of distributed hybrid flowshop. Expert Systems with Applications, 204, 117555. https://doi.org/10.1016/j.eswa.2022.117555
- Luo, H., Du, B., Huang, G. Q., Chen, H., & Li, X. (2013). Hybrid flow shop scheduling considering machine electricity consumption cost. International Journal of Production Economics, 146(2), 423–439. https://doi.org/10.1016/j.ijpe.2013.01.028
- Luo, J., Fujimura, S., El Baz, D., & Plazolles, B. (2019). GPU based parallel genetic algorithm for solving an energy efficient dynamic flexible flow shop scheduling problem. Journal of Parallel and Distributed Computing, 133, 244–257. https://doi.org/10.1016/j.jpdc.2018.07.022
- Lu, H., & Qiao, F. (2022). An efficient adaptive genetic algorithm for energy saving in the hybrid flow shop scheduling with batch production at last stage. Expert Systems, 39(2), e12678. https://doi.org/10.1111/exsy.12678
- Meng, L., Zhang, C., Shao, X., Ren, Y., & Ren, C. (2019). Mathematical modelling and optimisation of energy-conscious hybrid flow shop scheduling problem with unrelated parallel machines. International Journal of Production Research, 57(4), 1119–1145. https://doi.org/10.1080/00207543.2018.1501166
- Morais, M. D. F., & Perassoli Boiko, T. J. (2013). Hybrid flow shop scheduling problems involving setup considerations: A literature review and analysis. International Journal of Industrial Engineering, 20(11–12), 614–630.
- Nasiri, M. M., Abdollahi, M., Rahbari, A., Salmanzadeh, N., & Salesi, S. (2018). Minimizing the energy consumption and the total weighted tardiness for the flexible flowshop using NSGA-II and NRGA. Journal of Industrial and Systems Engineering, 11(Special issue), 150–162. 14th International Industrial Engineering Conference.
- Öztop, H., Tasgetiren, M. F., Kandiller, L., Eliiyi, D. T., & Gao, L. (2020). Ensemble of metaheuristics for energy-efficient hybrid flowshops: Makespan versus total energy consumption. Swarm Evolutionary Computation, 54, 100660. https://doi.org/10.1016/j.swevo.2020.100660
- Qin, H. X., Han, Y., Li, J., Sang, H., Chen, Q., Meng, L., & Zhang, B. (2021, May). A quick and effective iterated greedy algorithm for energy-efficient hybrid flow shop scheduling problem with blocking constraint. Proceedings of the 11th International Conference on Information Science and Technology (ICIST), Chengdu, China (pp. 325–331). IEEE.
- Qin, H. X., Han, Y. Y., Liu, Y. P., Li, J. Q., Pan, Q. K., & Xue, H. (2022). A collaborative iterative greedy algorithm for the scheduling of distributed heterogeneous hybrid flow shop with blocking constraints. Expert Systems with Applications, 201, 117256. https://doi.org/10.1016/j.eswa.2022.117256
- Qin, H., Han, Y., Wang, Y., Liu, Y., Li, J., & Pan, Q. (2022). Intelligent optimization under blocking constraints: A novel iterated greedy algorithm for the hybrid flow shop group scheduling problem. Knowledge-Based Systems, 258, 109962. https://doi.org/10.1016/j.knosys.2022.109962
- Qin, H. X., Han, Y. Y., Zhang, B., Meng, L. L., Liu, Y. P., Pan, Q. K., & Gong, D. W. (2022). An improved iterated greedy algorithm for the energy-efficient blocking hybrid flow shop scheduling problem. Swarm and Evolutionary Computation, 69, 100992. https://doi.org/10.1016/j.swevo.2021.100992
- Rashid, M. F. F. A., Mohd Rose, A. N., & Nik Mohamed, N. M. Z. (2022). Hybrid flow shop scheduling with energy consumption in machine shop using moth flame optimization. In Ahmad, F. Ab. N., Ahmad, N. I., Ismayuzri, I., Nafrizuan, M. Y., Muhammad, A. Z., Anwar, P. P., & Abdul, M. (Eds.), Recent Trends in Mechatronics Towards Industry 4.0 (pp. 77–86). Springer Singapore.
- Rashid, M. F. F. A., & Osman, M. A. H. (2020, April). Optimisation of energy efficient hybrid flowshop scheduling problem using firefly algorithm. Proceedings of the 2020 IEEE 10th Symposium on Computer Applications & Industrial Electronics (ISCAIE), Malaysia (pp. 36–41). IEEE.
- Ribas, I., Leisten, R., & Framiñan, J. M. (2010). Review and classification of hybrid flow shop scheduling problems from a production system and a solutions procedure perspective. Computers Operations Research, 37(8), 1439–1454. https://doi.org/10.1016/j.cor.2009.11.001
- Ruiz, R., & Vázquez-Rodríguez, J. A. (2010). The hybrid flow shop scheduling problem. European Journal of Operational Research, 205(1), 1–18. https://doi.org/10.1016/j.ejor.2009.09.024
- Schulz, S. (2018). A multi-criteria MILP formulation for energy aware hybrid flow shop scheduling. Proceedings of the Operations Research Proceedings 2016: Selected Papers of the Annual International Conference of the German Operations Research Society (GOR),Helmut Schmidt University Hamburg, Germany (pp. 543–549). Springer International Publishing.
- Schulz, S. (2019). A genetic algorithm to solve the hybrid flow shop scheduling problem with subcontracting options and energy cost consideration. Proceedings of the Information Systems Architecture and Technology: Proceedings of 39th International Conference on Information Systems Architecture and Technology–ISAT 2018: Part III, Nysa, Poland (pp. 263–273). Springer International Publishing.
- Schulz, S., Buscher, U., & Shen, L. (2020). Multi-objective hybrid flow shop scheduling with variable discrete production speed levels and time-of-use energy prices. Journal of Business Economics, 90(9), 1–29. https://doi.org/10.1007/s11573-020-00971-5
- Schulz, S., Neufeld, J. S., & Buscher, U. (2019). A multi-objective iterated local search algorithm for comprehensive energy-aware hybrid flow shop scheduling. Journal of Cleaner Production, 224, 421–434. https://doi.org/10.1016/j.jclepro.2019.03.155
- Shao, W., Shao, Z., & Pi, D. (2022a). A multi-neighborhood-based multi-objective memetic algorithm for the energy-efficient distributed flexible flow shop scheduling problem. Neural Computing & Applications, 34(24), 22303–22330. https://doi.org/10.1007/s00521-022-07714-3
- Shao, W., Shao, Z., & Pi, D. (2022b). A network memetic algorithm for energy and labor-aware distributed heterogeneous hybrid flow shop scheduling problem. Swarm and Evolutionary Computation, 75, 101190. https://doi.org/10.1016/j.swevo.2022.101190
- Shen, K., De Pessemier, T., Martens, L., & Joseph, W. (2021). A parallel genetic algorithm for multi-objective flexible flowshop scheduling in pasta manufacturing. Computers Industrial Engineering, 161, 107659. https://doi.org/10.1016/j.cie.2021.107659
- Shi, L., Guo, G., & Song, X. (2019). Multi-agent based dynamic scheduling optimisation of the sustainable hybrid flow shop in a ubiquitous environment. International Journal of Production Research, 52(2), 576–597.
- Song, C. (2021). A hybrid multi-objective teaching-learning based optimization for scheduling problem of hybrid flow shop with unrelated parallel machine. Institute of Electrical and Electronics Engineers Access, 9, 56822–56835.
- Tang, D., Dai, M., Salido, M. A., & Giret, A. (2016). Energy-efficient dynamic scheduling for a flexible flow shop using an improved particle swarm optimization. Computers in Industry, 81, 82–95. https://doi.org/10.1016/j.compind.2015.10.001
- Tang, L., Liu, W., & Liu, J. (2005). A neural network model and algorithm for the hybrid flow shop scheduling problem in a dynamic environment. Journal of Intelligent Manufacturing, 16(3), 361–370. https://doi.org/10.1007/s10845-005-7029-0
- Tao, X. R., Li, J. Q., Huang, T. H., & Duan, P. (2021). Discrete imperialist competitive algorithm for the resource-constrained hybrid flowshop problem with energy consumption. Complex & Intelligent Systems, 7(1), 311–326. https://doi.org/10.1007/s40747-020-00193-w
- Tian, X. K., Ma, B. C., Zhang, J. W., Zhao, R. Y., & Wang, J. (2019, April). Research on multi-objective optimization and simulation to HFSSP hybrid flow-shop scheduling problem for energy saving. Proceedings of the IOP Conference Series: Materials Science and Engineering, Qingdao, China (Vol. 504, No. 1, pp. 012108). IOP Publishing.
- Tosun, Ö., Marichelvam, M. K., & Tosun, N. (2020). A literature review on hybrid flow shop scheduling. International Journal of Advanced Operations Management, 12(2), 156–194. https://doi.org/10.1504/IJAOM.2020.108263
- Utama, D. M. (2019). An effective hybrid sine cosine algorithm to minimize carbon emission on flow-shop scheduling sequence dependent setup. Jurnal Teknik Industri, 20(1), 62–72. https://doi.org/10.22219/JTIUMM.Vol20.No1.62-72
- Utama, D. M. (2021a, March). Hybrid grey wolf algorithm for energy-efficient scheduling with sequence-dependent setup times: A case study. Proceedings of the IOP Conference Series: Materials Science and Engineering (Vol. 1096, No. 1, pp. 012116). IOP Publishing.
- Utama, D. M. (2021b, March). Minimizing Number of Tardy Jobs in Flow Shop Scheduling Using a Hybrid Whale Optimization Algorithm. Proceedings of the Journal of Physics: Conference Series (Vol. 1845, No. 1, pp. 012017). IOP Publishing.
- Utama, D. M. (2022, July). An effective hybrid crow search algorithm for energy-efficient flow shop scheduling. Proceedings of the AIP Conference Proceedings, Malang, Indonesia (Vol. 2453, No. 1, pp. 020040). AIP Publishing LLC.
- Utama, D. M., Baroto, T., Maharani, D. M., Jannah, F. R., & Octaria, R. A. (2018). Algoritma ant-lion optimizer untuk meminimasi emisi karbon pada penjadwalan flow shop dependent sequence set-up. Jurnal Litbang Industri, 9(1), 69–78. https://doi.org/10.24960/jli.v9i1.4775.69-78
- Utama, D. M., Ibrahim, M. F., Wijaya, D. S., Widodo, D. S., & Primayesti, M. D. (2022, December). A Novel Hybrid Multi-Verse Optimizer Algorithm for Energy-Efficient Permutation Flow Shop Scheduling Problem. Proceedings of the Journal of Physics: Conference Series, Malang, Indonesia (Vol. 2394, No. 1, p. 012006). IOP Publishing.
- Utama, D. M., & Primayesti, M. D. (2022). A novel hybrid Aquila optimizer for energy-efficient hybrid flow shop scheduling. Results in Control and Optimization, 9, 100177. https://doi.org/10.1016/j.rico.2022.100177
- Utama, D. M., Salima, A. A. P., & Widodo, D. S. (2022). A novel hybrid Archimedes optimization algorithm for energy-efficient hybrid flow shop scheduling. International Journal of Advances in Intelligent Informatics, 8(2), 237–250. https://doi.org/10.26555/ijain.v8i2.724
- Utama, D. M., & Widodo, D. S. (2021). An energy-efficient flow shop scheduling using hybrid Harris hawks optimization. Bulletin of Electrical Engineering and Informatics, 10(3), 1154–1163. https://doi.org/10.11591/eei.v10i3.2958
- Utama, D. M., Widodo, D. S., Ibrahim, M. F., & Dewi, S. K. (2020). An effective hybrid ant lion algorithm to minimize mean tardiness on permutation flow shop scheduling problem. International Journal of Advances in Intelligent Informatics, 6(1), 23–35. https://doi.org/10.26555/ijain.v6i1.385
- Utama, D. M., Widodo, D. S., Wicaksono, W., & Ardiansyah, L. R. (2019). A new hybrid metaheuristics algorithm for minimizing energy consumption in the flow shop scheduling problem. International Journal of Technology, 10(2), 320–331. https://doi.org/10.14716/ijtech.v10i2.2194
- Wang, J., Du, H., Xing, J., Qiao, F., & Ma, Y. J. (2020). Novel energy-and maintenance-aware collaborative scheduling for a hybrid flow shop based on dual memetic algorithms. IEEE Robotics Automation Letters, 5(4), 5613–5620. https://doi.org/10.1109/LRA.2020.3005626
- Wang, B., Huang, K., & Li, T. (2018). Two-stage hybrid flowshop scheduling with simultaneous processing machines. Journal of Scheduling, 21(4), 387–411. https://doi.org/10.1007/s10951-017-0545-x
- Wang, L., Kong, L., Li, F., & Lv, X. (2021). A ranking-based differential evolution algorithm for hybrid flow shop sustainable scheduling. Research Square, https://doi.org/10.21203/rs.3.rs-175466/v1
- Wang, G., Li, X., Gao, L., Li, P. J. S., & Computation, E. (2021). Energy-efficient distributed heterogeneous welding flow shop scheduling problem using a modified MOEA/D. Swarm and Evolutionary Computation, 62, 100858. https://doi.org/10.1016/j.swevo.2021.100858
- Wang, J. J., & Wang, L. (2021). A cooperative memetic algorithm with learning-based agent for energy-aware distributed hybrid flow-shop scheduling. IEEE Transactions on Evolutionary Computation, 26(3), 461–475. https://doi.org/10.1109/TEVC.2021.3106168
- Wang, J. -J., & Wang, L. (2022). A cooperative memetic algorithm with feedback for the energy-aware distributed flow-shops with flexible assembly scheduling. Computers & Industrial Engineering, 168, 108126. https://doi.org/10.1016/j.cie.2022.108126
- Wang, S., Wang, X., Chu, F., & Yu, J. (2020). An energy-efficient two-stage hybrid flow shop scheduling problem in a glass production. International Journal of Production Research, 58(8), 2283–2314. https://doi.org/10.1080/00207543.2019.1624857
- Widodo, D. S. and Utama, D. M., “The hybrid ant lion optimization flow shop scheduling problem for minimizing completion time,” in International Conference on Science and Technology 2019 Surabaya, Indonesia, 2020, vol. 1569, no. 2, p. 022097: IOP Publishing.
- Wu, X., Shen, X., & Cui, Q. (2018). Multi-objective flexible flow shop scheduling problem considering variable processing time due to renewable energy. Sustainability, 10(3), 841. https://doi.org/10.3390/su10030841
- Wu, X., Xiao, X., & Cui, Q. (2020). Multi-objective flexible flow shop batch scheduling problem with renewable energy. International Journal of Automation Control, 14(5–6), 519–553. https://doi.org/10.1504/IJAAC.2020.110071
- Xing, J., Qiao, F., & Lu, H. (2019). A hybrid multi-objective algorithm for energy-efficient scheduling considering machine maintenance. Proceedings of the 2019 IEEE 15th International Conference on Automation Science and Engineering (CASE), Vancouver, BC, Canada (pp. 115–120). IEEE.
- Xu, F., Tang, H., Xun, Q., Lan, H., Liu, X., Xing, W., Zhu, T., Wang, L., & Pang, S. (2022). Research on green reentrant hybrid flow shop scheduling problem based on improved moth-flame optimization algorithm. Processes, 10(12), 2475. https://doi.org/10.3390/pr10122475
- Xu, F., Weng, W., and Fujimura, S. (2014). Energy-efficient scheduling for flexible flow shops by using MIP. IIE Annual Conference. Proceedings, San Antonio, Texas, USA (pp. 1040). Institute of Industrial and Systems Engineers (IISE).
- Yankai, W., Shilong, W., Dong, L., Chunfeng, S., & Bo, Y. (2021). An improved multi-objective whale optimization algorithm for the hybrid flow shop scheduling problem considering device dynamic reconfiguration processes. Expert Systems with Applications, 174, 114793. https://doi.org/10.1016/j.eswa.2021.114793
- Yan, J., Li, L., Zhao, F., Zhang, F., & Zhao, Q. (2016). A multi-level optimization approach for energy-efficient flexible flow shop scheduling. Journal of Cleaner Production, 137, 1543–1552. https://doi.org/10.1016/j.jclepro.2016.06.161
- Yan, J., Wen, J., and Li, L. (2014). Genetic algorithm based optimization for energy-aware hybrid flow shop scheduling. Proceedings on the international conference on artificial intelligence (ICAI), Las Vegas, USA (pp. 1). The Steering Committee of The World Congress in Computer Science, Computer
- Yan, J. H., Zhang, F. Y., Li, X., Wang, Z. M., & Wang, W. (2014). Modeling and multiobjective optimization for energy-aware hybrid flow shop scheduling. Proceedings of 2013 4th International Asia Conference on Industrial Engineering and Management Innovation (IEMI2013), China (pp. 741–751). Springer.
- Yaurima-Basaldua, V. H., Tchernykh, A., Villalobos-Rodríguez, F., & Salomon-Torres, R. (2018). Hybrid flow shop with unrelated machines, setup time, and work in progress buffers for bi-objective optimization of tortilla manufacturing. Algorithms, 11(5), 68. https://doi.org/10.3390/a11050068
- Zeng, Z., Gao, L., & Hong, Z. (2022). Energy-aware scheduling of two-stage flexible flow shop based on group technology: Consideration of the Inconsistency of Process differences. International Journal of Computational Intelligence Systems, 15(1), 60. https://doi.org/10.1007/s44196-022-00124-2
- Zeng, Z., Hong, M., Man, Y., Li, J., Zhang, Y., & Liu, H. (2018). Multi-object optimization of flexible flow shop scheduling with batch process—Consideration total electricity consumption and material wastage. Journal of Cleaner Production, 183, 925–939. https://doi.org/10.1016/j.jclepro.2018.02.224
- Zhang, H., Li, J., Hong, M., & Man, Y. (2021). Artificial intelligence algorithm-based multi-objective optimization model of flexible flow shop smart scheduling (pp. 447–472).
- Zhang, B., Pan, Q. K., Gao, L., Li, X. Y., Meng, L. L., & Peng, K. K. (2019). A multiobjective evolutionary algorithm based on decomposition for hybrid flowshop green scheduling problem. Computers & Industrial Engineering, 136, 325–344. https://doi.org/10.1016/j.cie.2019.07.036
- Zhang, B., Pan, Q. K., Gao, L., Meng, L. L., Li, X. Y., & Peng, K. K. (2019). A three-stage multiobjective approach based on decomposition for an energy-efficient hybrid flow shop scheduling problem. IEEE Transactions on Systems, Man, and Cybernetics: Systems, 50(12), 4984–4999. https://doi.org/10.1109/TSMC.2019.2916088
- Zhang, M., Yan, J., Zhang, Y., & Yan, S. (2019). Optimization for energy-efficient flexible flow shop scheduling under time of use electricity tariffs. Procedia CIRP, 80, 251–256. https://doi.org/10.1016/j.procir.2019.01.062
- Zhou, B. H., & Gu, J. (2021). Energy-awareness scheduling of unrelated parallel machine scheduling problems with multiple resource constraints. International Journal of Operational Research, 41(2), 196–217.
- Zhou, R., Lei, D., & Zhou, X. (2019). Multi-objective energy-efficient interval scheduling in hybrid flow shop using imperialist competitive algorithm. IEEE Access, 7, 85029–85041. https://doi.org/10.1109/ACCESS.2019.2924998
- Zhou, B. & Liu, W. (2019). Energy-efficient multi-objective scheduling algorithm for hybrid flow shop with fuzzy processing time Proceedings of the Institution of Mechanical Engineers, Part I: Journal of Systems and Control Engineering, China (Vol. 233, no. 10, pp. 1282–1297).
- Zuo, Y., Fan, Z., Zou, T., & Wang, P. (2021). A novel multi-population artificial bee colony algorithm for energy-efficient hybrid flow shop scheduling problem. Symmetry, 13(12), 2421. https://doi.org/10.3390/sym13122421