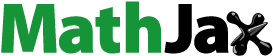
Abstract
The work aims to prepare and characterize polyester nanoclay nanocomposite (PNNCs) with various nanoclay weight percentages (0, 2, and 4). Nanoclay and polyester resin are blended using a mechanical stirrer followed by a sonicator. The blend is molded as specimens as per ASTM standards. The addition of nanoclay improved tensile strength by 12% to 16% and flexural strength by 4% to 10%. After 60 days of soaking in, the tensile strength retention rate of pure PE, 2PNNC, and 4PNNC are 84.9%, 89.8%, and 90.6%, respectively. At the same time, flexural strength retention rates of pure PE, 2PNNC, and 4PNNC are 86.7%, 90.2%, and 91.9%, respectively. SEM images are analyzed to know the reasons for specimen failure under tensile load. ExpDec1 (“One-phase exponential decay function with time constant parameter”) model is used on the experimental data to determine the composite’s durability. The experimental values and data produced by the ExpDec1 model are relatively close to one another. In all specimens, the error percentage of experimental and predicted values during 80 and 100 days of water soaking varies very little (less than 1%). The study proposes CS-ANN (Cuckoo Search-Artificial Neural Network) architecture to predict mass loss. Test results prove that the CS-ANN predicted values are much closer to the experimental results. Cuckoo Search Algorithm (CSA) is used along with the ANN model to optimize and fine-tune the hyperparameters according to the data. The loss curves substantially prove the proposed model to be the best fit for the experimental data.
1. Introduction
The ever-growing performance need in the modern market for sophisticated materials has recently focused on the advancements in polymer nanocomposites that exhibit improved physicochemical properties. The substantial improvement in the interfacial area caused by the introduction of nanoscale fillers, which raises the interfacial polymer chain’s volume fraction and filler–polymer interactions, gives nanocomposites their superior properties (Daglar et al., Citation2021).
Polyester resins make excellent candidates for creating nanocomposites due to the flexibility of their synthesis, which enables the creation of polyesters with exceptional chemical, thermal, and mechanical properties (Bhat et al., Citation2019). Polyester resin reinforced with fibers or micro-sized and nano-sized particles is broadly used in many industries involving water sports and the construction of pleasure boats and windsurfers. Due to its superior performance and versatile use, a revolution in the shipping sector is centered on polyester. Polyester resins are widely used in the automobile sector due to their design flexibility, lightweight, low costs, and excellent mechanical strength. Additionally, this material is used in the construction industry, specifically in producing bathroom vanities, pipes, tunnels, and drums (Saharudin et al., Citation2016; Shettar et al., Citation2020).
The most popular and extensively studied nanoparticle in polymer nanocomposites is nanoclay (Nemati Giv et al., Citation2020). Because of its ease of usage, advantages for the environment, and intricate chemistry, nanoclay has gained favor over other nanoparticles. Nanoclay is more affordable, less dense, and has a larger surface area. It also has a high elastic modulus. The aforementioned properties greatly enhance nanocomposites’ durability, wear resistance, fracture toughness, and strength (Merah et al., Citation2022; Merzah et al., Citation2022). A small amount of nanoclay, typically less than 5 wt.%, can enhance various properties. The characteristics of polyester resin are improved by adding nanoclay, according to numerous studies (Abdul Hanan et al., Citation2022; Megahed et al., Citation2022). Seetharaman and Narayanan (Seetharaman & Narayanan, Citation2022) investigated the effect of adding 5 wt.% bentonite nanoclay to polyester resin. The results show that adding nanoclay improves tensile, flexural, impact, and ILSS properties by 16%, 15%, 25%, and 26%, respectively. This improvement could be related to the nanoclay’s stiffening feature, which minimizes the mobility of polymeric molecules.
Temperature, humidity, fluctuating loads, and their combinations can cause polymers to degrade in the environment. Most polymer-based composites absorb moisture, and the water molecules act as plasticizers. Therefore, it is important to comprehend how polymers behave under adverse environmental conditions throughout their service life. Even though there are numerous investigations on water immersion of polymer-based composites in literature, it is very challenging to comprehend the mechanism. Kini et al. (Kini et al., Citation2022) investigated the short-term water exposure effect on the polyester-based nanocomposite. The polyester matrix’s susceptibility to water exposure has been shown, and adding nanoclay has mitigated its effects. Merah and Mohamed (Merah & Mohamed, Citation2019) studied the impact of varying wt.% of nanoclay and water uptake on polyester resin properties. The addition of nanoclay enhances the mechanical properties and reduces the water uptake (%). The water barrier property of nanoclay has minimized the plasticization effect of the moisture and thus reduced the polymer’s flexural strength degradation.
The material’s wear property is just as significant as its mechanical properties for any specified application. Wear is the progressive loss of material from the operating surface of a solid occurring as a result of relative motion between two surfaces. The five main types of wear normally affecting polymer composites are fretting, fatigue wear, adhesion, erosion, and abrasive wear (Jumahat et al., Citation2016). Material, load, speed, and time are a few variables that affect wear properties (Shettar et al., Citation2021).
Simulation of material properties can be helpful in parameter optimization for various applications. It is not easy to comprehend the foundation of particle-reinforced composites without reliable ways to anticipate material properties. Additionally, the nonlinear nature of the problem, like in the wear process, makes this task even more difficult. Thus, simulating the mechanical and physical properties of engineering materials has been extensively followed in material science using Artificial Neural Networks (ANNs) in recent years (Mutuk et al., Citation2020). ANN-based models are able to predict the material properties with high accuracy and even outperform many adaptive neuro-fuzzy inference system (ANFIS) models in this regard (Alas et al., Citation2020; Boga & Koroglu, Citation2021). Considering the strong learning capacity of ANN models, they have been employed as an effective technique to solve various real-world problems (Srinivas, Shankar, et al., Citation2022; Srinivas, Sharma, et al., Citation2022). Most of the literature presents the selection of hyperparameters of the ANN model specific to the experimental data and application, which essentially necessitates automation for optimizing its selection. A wide range of metaheuristic algorithms is commonly used for parameter optimization for various applications (Abdel-Basset et al., Citation2018). Prior empirical studies are conducted to choose the Cuckoo Search Algorithm (CSA) (Yang & Deb, Citation2010) for parameter tuning of the proposed ANN model for wear property analysis of the material.
The current work investigates the impact of water soaking and nanoclay wt.% on the tensile and flexural strength of polyester nanoclay nanocomposites (PNNCs). It also aims to examine the retention of property and “durability prediction analysis” by mathematical modeling. Fracture surfaces under tensile load are also examined to identify the reasons for specimen failure. This study also proposes the CS-ANN model, which uses the Cuckoo Search Algorithm (CSA) for hyperparameters optimization of the ANN model to predict the wear property, i.e., mass loss of PNNCs under each factor.
Despite several pieces of literature reported earlier, very few works report the effect of varying wt.% of nanoclay and water soaking on the properties of nanoclay-reinforced polyester composites. Moreover, to the best of the author’s knowledge, no work has been reported on the “durability prediction analysis” of nanoclay-reinforced polyester composites by mathematical modeling and application of the ANN model for wear prediction.
2. Methodology
2.1. Materials and specimen preparation
Surface-modified nanoclay (Montmorillonite clay) is utilized as reinforcement and supplied by “Sigma Aldrich”. Nanoclay has a density of between 0.2 and 0.5 g/cm3 and sheet-like structure. Each nanoclay sheet is just a few nanometers thick and has lateral dimensions ranging from 200 to 600 nanometers. The features of nanoclay include barrier, chemical resistance, flame retardancy, and a greater modulus than the matrix. A possible reinforcement for polymers, nanoclay has a high aspect ratio and a sheet-like form.
The matrix material is polyester with curing agents, cobalt accelerators, and MEKP catalysts (“1 kg: 12 ml: 15 ml”). Three sets of specimens are prepared using various nanoclay weight percentages, as presented in Table . Polyester and nanoclay are mechanically stirred together for 2 h. After mechanical stirring, sonication is performed for 0.5 h to ensure that the nanoclay and polyester are adequately mixed. Before being put into the molds, the nanoclay-polyester mixture is thoroughly mixed with the cobalt accelerator and MEKP catalyst. The specimen molds are created following ASTM Standards D790 and D638. Demoulded specimens’ rough surfaces are smoothed with emery paper.
Table 1. Different sets of specimens
2.2. Water soaking
PNNC specimens are submerged in water for 10, 20, 30, 40, 50, 60, 80, and 100 days. The ASTM D570–98 standard is followed when conducting water uptake tests. Digital balances are used to weigh dry samples at first. The specimens are then submerged in water at ambient temperature for the aforementioned periods.
Samples are taken out of the water at the end of 10, 20, 30, 40, 50, 60, 80, and 100 days. A paper towel dries up the samples and then weighed. The water uptake is assessed based on sample weight and soaking time variations.
The following Equation is used to calculate the percentage of water uptake:
where, Wws—Water soaked specimen weight; Wam—As-made weight of the specimen
2.3. Mechanical testing
According to ASTM D638, tensile testing is performed using a “Universal Testing Machine.” At least five specimens of each type of polyester-nanoclay nanocomposites and each case, i.e., end of each water soaking period, are made into dumbbell-shaped shapes (Figure ). The crosshead motion test speed is held constant at 5.0 mm/min.
As per ASTM D790, the three-point flexural tests are conducted. Each set is molded into specimens (140 mm x 15 mm x 3 mm in size – Figure ). At least five specimens of each type of polyester-nanoclay nanocomposites and each case, i.e., end of each water soaking period. The flexural characteristics of polyester-nanoclay nanocomposites are investigated using a “ZWICK-ROELL Z020, LOADCELL 20 kN” machine. Flexural tests are conducted with a span length of 48 mm and a constant test speed of 1.28 mm/min.
2.3.1. Durability prediction analysis
Comparing the composite’s performance under different test conditions offers an indirect estimate of the composite’s durability. It is required to use some extrapolation techniques on the experimental values to determine the composite’s durability. With the aid of experimental data, the durability prediction Equation Equation11
1 is constructed using nonlinear exponential regression. To create an equation for the durability of composite, the ExpDec1 model (“One-phase exponential decay function with time constant parameter”) and Equation Equation1
1
1 are utilised (He et al., Citation2021; Kowshik et al., Citation2021).
Where A1, t1, and y0 - Constant values generated by graph
x—x-axis value of graph (number of days)
y—y-axis predicted value based on x-axis value
2.3.2. Failure mode analysis
A “Scanning Electron Microscope (ZEISS, Model: EVO18)” is used to capture the SEM images of the specimen’s fractured surface. To fit in the specimen holder of the microscope, samples must be sliced to the appropriate sizes. For efficient imaging, the sample surface must be conductive. Utilizing a small sputter coater (“Model: SC7620”) machine, a tinny layer of conductive material is applied to the specimen’s surface for 10 min. The sputter coater device uses the gold-palladium (at the ratio of 80:20) sputtering object.
2.4. Wear testing
According to ASTM G-99 standard, wear testing of PNNCs is carried out on “pin-on-disc” equipment. The equipment uses a steel disc with a nominal roughness of 5 microns. A fixture is used to hold the specimen perpendicular to the rotating disc. The load is applied via a lever attachment. The time, load, and speed are also carefully modified for each test repetition. Input parameters with different levels in this study are nanoclay (0, 2, and 4 wt.%), speed (100, 200, and 300 rpm), load (1, 2, and 3 kg), and time (5, 10, and 15 min). All three sets of specimens are subjected to wear test by varying aforesaid input parameters with varying levels. Each specimen’s mass loss (wear property) is obtained by weighing the specimen before and after the wear test. An average of five tests is taken for each sample, parameter, and level.
2.4.1. Artificial neural network
ANN is an effective method used for data prediction for a wide range of applications, which is a computer model built by interconnecting numerous processing units called neurons. Considering its numerous applications, a multi-layered feed-forward backpropagation neural network architecture is used in this study to predict mass loss by wear test. The model consists of one input layer, followed by a maximum of two hidden layers and a fully connected output layer, as shown in Figure . The number of hidden layers is limited to two as a denser network will not effectively help in learning the feature set in our study. The four input parameters, viz. nanoclay, speed, load, and time (), are normalized and fed to the input neuron layer. The output layer consists of a single neuron indicating the predicted output (y’).
Neurons are interconnected between layers, weighed by the respective weight factor, indicating the importance of each feature. Activation functions describing a nonlinear relationship between input and output neurons are preferred in modeling the network. The input and output data have been normalized in the range [0,1] to enhance and stabilize ANN training.
The final predicted output (y’), thus, can be mathematically modeled as Equation Equation22
2
Where represents the number of neurons in the input layer, hidden layer 1 and 2, respectively.
represents the inputs, with i ranging from 1 to n = 4 for the model in Figure .
and
are the interconnecting weight factors between each layer, and
and
represents the corresponding bias in each layer of the network. The activation functions,
and
are optimized using Cuckoo Search Algorithm (CSA) (Yang & Deb, Citation2010) for each layer and multiplied with the weighted sum of feature values with the corresponding bias to generate the predicted value. In the proposed model, the number of hidden layers, neurons in each of the hidden layer, the nonlinear activation function to be used, and the learning rate are optimized using CSA, which is one among the recent, computationally efficient metaheuristic approach used for parameter optimization. The constraint limits are prefixed as [Equation1
1
1 , Equation2
2
2 ] for the number of hidden layers, 1 to 10 for neurons in each hidden layer, 0.01 to 0.99 for the learning rate, and the activation function is chosen from the feasible set, which includes rectified linear unit (ReLU), Sigmoid, Tanh and Tansig. These bounding limits are set by performing an empirical study based on the experimental data.
The initial weights and bias sets are generated at random. To ensure that the ANN’s outputs accurately fit the real data, these values are then updated and improved using CSA. The neural network is validated and tested with 20% of the experimental data after training, with the remaining 80%. The maximum number of epochs is set at 300, and mean square error (MSE), perhaps the most widely used error measure, is used as the fitness function of the CSA to evaluate the performance of the ANN model.
2.4.2. Cuckoo Search Algorithm (CSA)
CSA is a meta-heuristic optimization algorithm developed by Yang and Deb (Yang & Deb, Citation2010) inspired by the intriguing reproductive strategy of certain Cuckoo species. To improve the likelihood of their eggs getting hatched, these cuckoos lay eggs in the nest of other birds and even take host eggs out of the nest. The three primary forms of blood parasitism that these birds display are (1) intra-specific (2) cooperative breeding (3) nest takeover. Some host bird species toss out cuckoo eggs or even abandon their nest and build a new one when foreign eggs are found. The odds of getting captured are decreased since certain species of cuckoo are cunning enough to replicate the colour and texture of the host bird’s egg. The following three conditions are considered to streamline the entire procedure.
Each cuckoo will lay only one egg at a time in any nest they choose at random.
The best-egg-laying nests are passed down to the following generation.
The total number of nests is fixed, and the likelihood of the host species finding the cuckoo’s egg is fixed within the range
[0, 1].
An initial solution space is randomly generated following Equation Equation33
3 . Each egg represents a possible solution, and the fitness value of each egg is computed by evaluating the MSE. Each iteration updates the possible solution set using Levy flight strategy as modeled in Equation Equation4
4
4 .
Where is the population size
,
denotes the number of parameters to be optimized and [
,
] indicates the lower and upper bounds of each parameter, and
is the iteration count. The values for
and
(maximum no of iterations) are fixed as 30 and 50, respectively.
Where is the step size and
The host species will abandon the nest or discard the cuckoo’s egg once it is found, which is represented in the algorithm by replacing of the total number of nests with new ones.
Compared to linear models, the nonlinear relationship of the variance of Levy flight, as indicated in Equation Equation55
5 , aids in a more effective exploration of the solution space.
The algorithm iterates, improving the solution set following the Levy flight strategy till it reaches the termination condition. This optimal exploitation phase eventually helps in reducing the chances of getting stuck in an optimal local solution, which frequently occurs in many other metaheuristic algorithms of a similar kind.
2.4.3. Proposed CS-ANN model
The proposed CS-ANN model to predict the mass loss is shown in Figure . As explained in section 2.4.2, the ANN architecture is built by optimizing the parameters using CSA for the test case. CSA algorithm helps in deciding the optimum number of hidden layers, neurons in each of the hidden layer, the nonlinear activation function, and the learning rate.
3. Results and discussions
3.1. Water uptake
Figure shows that the total water uptake of nanocomposites continuously reduces as the wt.% of nanoclay increases. All nanocomposites have been shown to exhibit the normal water absorption behaviour of polymers. It is also obvious that the water uptake curves are flat after 60 days, indicating the specimens have reached saturation level. Pure PE, which is thought to have the water-affinity functional groups “hydroxyl and amino-methyl,” is shown to have the highest total water uptake. Lower water uptake is the outcome of the pure PE network having less mean free path for water molecules to travel due to the nanoclay addition. The main factor enhancing water uptake resistance is that the nanoclay’s increased aspect ratio gives water molecules tortuous routes (Kini et al., Citation2022; Merah & Mohamed, Citation2019).
3.2. Tensile strength
As shown in Table , adding nanoclay increases the tensile strength of polyester resin by 12% to 16%. The higher load transfer between the nanoclay and matrix, as well as the better nanoclay platelet dispersion in the polyester resin, are the causes of the improved tensile strength of 2PNNC. The tensile strength of the 4PNNC marginally reduces compared to 2PNNC. A similar trend is observed in (Shettar et al., Citation2021), i.e., nanoclay addition enhances the tensile strength of polyester by 14% and 11% at 2 and 4 wt.% of nanoclay, respectively.
Table 2. Tensile strength (MPa) of polyester and nanocomposites with number of days of water soaking
The water soaking condition significantly negatively impacts the tensile strength of pure PE and PNNC, as shown in Table . The specimens’ tensile strength decreases as the number of soaking days increases. The tensile strength retention rate of pure PE is very low compared to 2PNNC and 4PNNC in all the intervals. After 60 days of soaking in, the retention rate of pure PE, 2PNNC, and 4PNNC are 84.9%, 89.8%, and 90.6%, respectively. A similar trend is observed by Kini et al. (Kini et al., Citation2022), after 84 days of water soaking, the retention rate of tensile strength of neat polyester, 2 and 4 wt.% nanoclay reinforced polymer composites are 80%, 84%, and 88%, respectively.
The tensile strength of pure PE is decreased under water soaking conditions due to engrossed water acting as the plasticizer. In comparison to pure PE, the tensile strengths of 2PNNC and 4PNNC are less affected. The smaller percentage of decrease in the tensile strength shows how adding nanoclay has improved the resistance to plasticization. Additionally, nanoclay delays the total water soaking effect by providing “tortuous paths” for water molecules to enter PNNCs (Kusmono et al., Citation2020; Saxena et al., Citation2020). In addition, the nanoclay’s higher aspect ratio offers protection against polymer-chain agility in water-absorbing polymers (Rafiq & Merah, Citation2019), resulting in less tensile strength loss.
3.2.1. SEM analysis
The effect of adding nanoclay on the morphology of fractured surfaces for pure PE is shown in Figure . Comparing pure PE and PNNCs, there is a distinct difference in the fracture surface. Pure PE in Figure has a completely smooth, featureless surface that is typical of glassy materials, indicating low fracture toughness and rapid crack propagation.
Figure 5. SEM images of fracture surface of as-made specimens under tensile load (a) pure PE (b) 2PNNC (c) 4PNNC.

The SEM images show that the morphology of the fractured surfaces becomes rougher as the nanoclay weight percentage increases (Figure ). The nanoclay presence upfront of crack paths facilitates the developing microcracks, presumably causing the PNNCs’ rough fractured surfaces. The fracture surface area and fracture toughness are increased as the crack propagation path is forced to turn out to be convoluted (Chan et al., Citation2011). As shown in Table , the nanoclay behaves as a blockade to prevent crack propagation, increasing the tensile strength of 2PNNC.
A gluey and sluggish mixture results when 4 wt.% nanoclay is mixed with polyester in 4PNNCs. Inevitable nano or micro-air bubbles appear in the cured composites as a result of the PNNCs’ later incorporation of a cobalt accelerator and an MEKP catalyst. The drop in the tensile strength of the nanocomposite, as shown in Table , may be caused by the air bubbles and micro-voids (Figures , shown with an arrow (Yellow color)).
Figure 6. SEM images of fracture surface of water soaked specimens under tensile load (a) pure PE (b) 2PNNC (c) 4PNNC.

For the pure PE specimen in the water-soaking condition (Figure ), a multifaceted nexus of microcracks on the fracture surface is evident due to engrossed water, which encourages the plasticization effect of water and speeds up craze initiation and propagation in the polyester (Hu et al., Citation2020). At different stages of crack propagation, high enough stress to create subsequent local cracks causes the rougher area to develop near the fracture front. The crazes that start close to the crack front do not have adequate time to spread because of how quickly the crack develops (Hamim & Singh, Citation2014).
In contrast to the as-made specimen (Figure ), the water-soaked PNNCs specimen (Figure exhibits shear leaps and a lesser rough surface, suggesting poorer fracture toughness. When compared to PNNC, SEM images demonstrate that water soaking has a significant impact on pure PE. This may help to explain why PNNC’s tensile strength declined less noticeably than that of polyester. Nanoclay addition to polyester boosts the cross-linking density and hinders the water molecule movement. Compared to pure PE, nanoclay inclusion reduces the effects of water soaking, improving the tensile strength retention rate of PNNCs.
3.2.2. Durability prediction
Figure plots experimental and ExpDec1 model-generated data using the Origin 2022 in relation to tensile strength vs. number of days of water soaking. Since the curves of experimental values and data produced by the ExpDec1 model are relatively close to one another, it is possible to estimate the values based on the number of days of water soaking using Equation Equation11
1 and constant values, such as A1, t1, and y0. Tensile tests are carried out on all specimens (pure PE, 2PNNC, and 4PNNC) for longer periods of water soaking, i.e., 80 and 100 days, to verify the accuracy of Equation Equation1
1
1 and its values. The experimental and predicted values for each of the three set specimens are shown in Table . In all specimens, the error percentage during 80 and 100 days of water soaking varies very little. With the use of prediction models, composites’ durability may be estimated.
Table 3. Experimental values (EV) and predicted values (PV) of tensile strength (MPa)
3.3. Flexural strength
Table shows that, in comparison to pure PE (52 MPa), the flexural strengths of 2PNNC and 4PNNC increase to 57 and 54 MPa, respectively. Stress transfer, elastic deformation, and the improved flexural strength of PNNC result from the strengthened interfacial bonding between nanoclay and matrix. The surrounding matrix is strengthened and stiffened by nanoclay, which prevents the polymer chain from sliding and makes the matrix less elastic (Guo et al., Citation2018). The polymer chains get harder to separate and move with an increase in the weight % of nanoclay. A similar trend is observed in (Shettar et al., Citation2022), i.e., the flexural strength of epoxy is improved due to the addition of nanoclay by 4% and 9% at respective nanoclay weight percentages of 4 and 2.
Table 4. Flexural strength (MPa) of polyester and nanocomposites with number of days of water soaking
Because water soaking negatively impacts the composite’s mechanical properties, it is evident from Table that all specimens lost strength when soaked in water. As the number of soaking days increases, the flexural strength of every specimen diminishes. In all intervals, the retention rate of pure PE’s flexural strength is much lower than that of 2PNNC and 4PNNC. The retention rates of pure PE, 2PNNC, and 4PNNC are 86.7%, 90.2%, and 91.9%, respectively, after 60 days of water soaking. A similar trend is observed by Merah and Mohamed (Merah & Mohamed, Citation2019), after 90 days of water soaking, the retention rate of flexural strength of neat polyester, 2 and 4 wt.% nanoclay reinforced polymer composites are 60%, 80%, and 90%, respectively.
As shown in Table , the flexural strength of the pure PE and PNNC specimens decreases after soaking in water compared to the original as-prepared specimens. This is partly because the presence of water inside the polyester system increases its ductility. Due to nanoclay, which works as a blockade to water molecules’ flow, PNNCs are less affected by water soaking (Merah et al., Citation2022). The greater aspect ratio of the nanoclay platelets in water-absorbed PNNCs provides endurance to the polymer chain’s movement, resulting in less flexural strength loss than pure PE.
3.3.1. Durability prediction
Figure depicts the relationship between flexural strength vs. number of days of water soaking for experimental data as well as data produced by the ExpDec1 model. The ExpDec1 model fit and experimental data curves are quite near to each other. Experimental values (EV) and predicted values (PV) of flexural strength (MPa) are shown in Table . Flexural tests are conducted for all the specimens for soaking periods of 80 and 100 days in order to evaluate the accuracy of EquationEquation 11
1 and its values. The percentage of error for 80 and 100 days of soaking varies little in all three groups of nanocomposites. Prediction models enable the estimation of the durability of composite.
Table 5. Experimental values (EV) and predicted values (PV) of flexural strength (MPa)
3.4. Wear property
Our previously published work discusses the effect of individual input parameters, i.e., nanoclay, speed, load, and time, on the wear property, i.e., mass loss of PNNCs (Shettar et al., Citation2021). Hence, this study concentrates on the ANN application for predicting the wear property, i.e., mass loss of PNNCs.
3.4.1. ANN model performance
The design parameters for the model, tuned and optimized using CSA, are shown in Table . The number of neurons in each hidden layer has been optimized to be 5 and 6 neurons, respectively, using CSA. The activation function is set to “rectified linear unit (ReLU),” and the learning rate is optimized to be 0.52. ReLU is the simplest and widely used activation function that introduces non-linearity into the neural network’s output. It is mathematically modeled as , where
is the input to the function. In other words, if the input is greater than zero, the output is equal to the input value itself and if the input is less than or equal to zero, the output is zero. The simple thresholding operations involved makes it computational efficient and faster compared with other activation functions. Figure depicts the plot between the best fitness values (MSE) versus number of iterations using the Cuckoo Search Algorithm. The curve is clearly found to be converging from the 33rd iteration/epoch, as shown in Figure , yielding the optimal set of values for the parameters to train the ANN for the experimental data.
Table 6. Optimized design parameters and specifications for ANN
The loss curves shown in Figure indicate the proposed model best fit for the data. According to the loss function graph, the network parameters are appropriate for this kind and quantity of data. The positive correlation between training loss and validation loss may be observed in Figure . After 100 epochs, both training and validation losses are at their lower points and remain at a constant value, indicating that the model is properly trained and a satisfactory fit is attained.
The proposed CS-ANN model is evaluated for the test cases indicated in Table . Confirmation tests are performed under identical conditions to ensure the established model is adequate. Table lists the new levels for experimental and CS-ANN-predicted mass loss for the input parameters.
Table 7. Confirmation test results mass loss
The variance in mass loss among the experimental and CS-ANN predicted values is much less, indicating that the CS-ANN model is acceptable in correlating input parameters to the PNNCs wear property, i.e., mass loss.
4. Conclusions
The total water uptake (%) of nanocomposites continuously reduces as the wt.% of nanoclay increases compared to pure PE.
Adding nanoclay increases the tensile and flexural strength of polyester resin. The tensile strength of the 4PNNC marginally reduces compared to 2PNNC, but is higher than the pure PE.
The retention rate of pure PE is very low compared to 2PNNC and 4PNNC in all the intervals. After 60 days of soaking in, the tensile strength retention rate of pure PE, 2PNNC, and 4PNNC are 84.9%, 89.8%, and 90.6%, respectively. At the same time, flexural strength retention rates of pure PE, 2PNNC, and 4PNNC are 86.7%, 90.2%, and 91.9%, respectively.
The smoother surface in the SEM images of the pure PE indicated rapid crack propagation and low fracture toughness. However, the fracture surface of PNNCs is noticeably rougher, as seen in the SEM micrograph. Nanoclay prevents and slows the spread of cracks in 2PNNCs. The existence of air bubbles and micro-voids may be the reason for the 4PNNCs’ decreased tensile strength. SEM images also show how the fracture surface might change as a result of absorbed water.
The mathematical model for prediction provides good congruence among experimental and predicted values. The error percentage for long-term soaking varies very slightly. Consequently, a composite’s durability may be estimated fairly.
The study proposes CS-ANN architecture to predict the mass loss, and test results prove that the CS-ANN predicted values are very much closer to the experimental results.
Cuckoo Search Algorithm (CSA) is used along with the ANN model to optimize and fine-tune the hyperparameters according to the data. The loss curves substantially prove the proposed model to be the best fit for the experimental data.
Disclosure statement
No potential conflict of interest was reported by the authors.
References
- Abdel-Basset, M., Abdel-Fatah, L., & Sangaiah, A. K. (2018). Metaheuristic algorithms: A comprehensive review. In Computational intelligence for multimedia big data on the cloud with engineering applications (pp. 185–20). Elsevier. https://doi.org/10.1016/B978-0-12-813314-9.00010-4
- Abdul Hanan, U., Abu Hassan, S., Uzir Wahit, M., Binoj, J. S., Mansingh, B. B., & Goh, K. L. (2022, Sep). Experimentation, optimization, and predictive analysis of compressive behavior of montmorillonite nano‐clay/unsaturated polyester composites. Polymer Composites, 44(1), 241–251. https://doi.org/10.1002/pc.27041
- Alas, M., Ali, S. I. A., Abdulhadi, Y., & Abba, S. I. (2020, Oct). Experimental evaluation and modeling of polymer nanocomposite modified asphalt binder using ANN and ANFIS. Journal of Materials in Civil Engineering, 32(10), https://doi.org/10.1061/(ASCE)MT.1943-5533.0003404
- Bhat, R., Mohan, N., Sharma, S., Pratap, A., Keni, A. P., & Sodani, D. (2019). Mechanical testing and microstructure characterization of glass fiber reinforced isophthalic polyester composites. Journal of Materials Research and Technology, 8(4), 3653–3661. https://doi.org/10.1016/j.jmrt.2019.06.003
- Boga, C., & Koroglu, T. (2021, Oct). Proper estimation of surface roughness using hybrid intelligence based on artificial neural network and genetic algorithm. Journal of Manufacturing Processes, 70, 560–569. https://doi.org/10.1016/j.jmapro.2021.08.062
- Chan, M., Lau, K., Wong, T., Ho, M., & Hui, D. (2011, Sep). Mechanism of reinforcement in a nanoclay/polymer composite. Composites Part B: Engineering, 42(6), 1708–1712. https://doi.org/10.1016/j.compositesb.2011.03.011
- Daglar, O., Çakmakçi, E., Gunay, U. S., Hizal, G., Tunca, U., & Durmaz, H. (2021, Jul). All in one: The preparation of polyester/silica hybrid nanocomposites via three different metal-free click reactions. European Polymer Journal, 154, 110532. https://doi.org/10.1016/j.eurpolymj.2021.110532
- Guo, F., Aryana, S., Han, Y., & Jiao, Y. (2018, Sep). A review of the synthesis and applications of polymer–nanoclay composites. Applied Sciences, 8(9), 1696. https://doi.org/10.3390/app8091696
- Hamim, S. U., & Singh, R. P. (2014). Effect of hygrothermal aging on the mechanical properties of fluorinated and nonfluorinated clay-epoxy nanocomposites. International Scholarly Research Notices, 2014, 1–13. https://doi.org/10.1155/2014/489453
- He, X., Zhang, H., Qiu, L., Mao, Z., & Shi, C. (2021, Apr). Hygrothermal performance of temperature-humidity controlling materials with different compositions. Energy & Buildings, 236, 110792. https://doi.org/10.1016/j.enbuild.2021.110792
- Hu, Y., Ji, W. M., & Zhang, L. W. (2020, Sep). Water-induced damage revolution of the carbon nanotube reinforced poly (methyl methacrylate) composites. Composites Part A, Applied Science and Manufacturing, 136, 105954. https://doi.org/10.1016/j.compositesa.2020.105954
- Jumahat, A., Talib, A. A. A., & Abdullah, A. (2016). Wear properties of nanoclay filled epoxy polymers and fiber reinforced hybrid composites (pp. 247–260). https://doi.org/10.1007/978-981-10-1953-1_11
- Kini, A., Shettar, M., Kowshik, S., Nikhil, R., & Chate, G. (2022). Water soaking and re-drying effect on mechanical and wear properties of nanoclay-polyester nanocomposites. Materials Research, 25. https://doi.org/10.1590/1980-5373-mr-2021-0478
- Kowshik, S., M C, G., Shettar, M., Bhat, R., B M, G., & Gupta, M. (2021, Jan). Durability prediction analysis on mechanical properties of GFRP upon immersion in water at ambient temperature. Cogent Engineering, 8(1), https://doi.org/10.1080/23311916.2021.1956869
- Kusmono, Hestiawan, H., Jamasri. (2020, May). The water absorption, mechanical and thermal properties of chemically treated woven fan palm reinforced polyester composites. Journal of Materials Research and Technology, 9(3), 4410–4420. https://doi.org/10.1016/j.jmrt.2020.02.065
- Megahed, M., Agwa, M., & Megahed, A. (2022, Jun). Effect of ultrasonic parameters on the mechanical properties of glass fiber reinforced polyester filled with nano-clay. Journal of Industrial Textiles, 51(2_suppl), 2944S–2959S. https://doi.org/10.1177/1528083720918348
- Merah, N., Ashraf, F., & Shaukat, M. M. (2022, Apr). Mechanical and moisture barrier properties of epoxy–nanoclay and hybrid epoxy–nanoclay glass fibre composites: A review. Polymers (Basel), 14(8), 1620. https://doi.org/10.3390/polym14081620
- Merah, N., & Mohamed, O. (2019, Jan). Nanoclay and water uptake effects on mechanical properties of unsaturated polyester. Journal of Nanomaterials, 2019, 1–11. https://doi.org/10.1155/2019/8130419
- Merzah, Z. F., Fakhry, S., Allami, T. G., Yuhana, N. Y., & Alamiery, A. (2022, Jan). Enhancement of the properties of hybridizing epoxy and nanoclay for mechanical, industrial, and biomedical applications. Polymers (Basel), 14(3), 526. https://doi.org/10.3390/polym14030526
- Mutuk, T., Gürbüz, M., & Mutuk, H. (2020, Aug). Prediction of wear properties of graphene-Si 3 N 4 reinforced titanium hybrid composites by artificial neural network. Materials Research Express, 7(8), 086511. https://doi.org/10.1088/2053-1591/abaac8
- Nemati Giv, A., Rastegar, S., & Özcan, M. (2020, Jan). Influence of nanoclays on water uptake and flexural strength of glass–polyester composites. Journal of Applied Biomaterials & Functional Materials, 18, 228080002093018. https://doi.org/10.1177/2280800020930180
- Rafiq, A., & Merah, N. (2019, Jan). Nanoclay enhancement of flexural properties and water uptake resistance of glass fiber-reinforced epoxy composites at different temperatures. Journal of Composite Materials, 53(2), 143–154. https://doi.org/10.1177/0021998318781220
- Saharudin, M. S., Wei, J., Shyha, I., & Inam, F. (2016). The degradation of mechanical properties in halloysite nanoclay-polyester nanocomposites exposed in seawater environment. Journal of Nanomaterials, 2016, 1–12. https://doi.org/10.1155/2016/2604631
- Saxena, D., Soundararajan, N., Katiyar, V., Rana, D., & Maiti, P. (2020, Jul). Structural, mechanical, and gas barrier properties of poly(ethylene terephthalate) nanohybrid using nanotalc. Journal of Applied Polymer Science, 137(27), 48607. https://doi.org/10.1002/app.48607
- Seetharaman, A., & Narayanan, V. (2022, Oct). Effect of aluminosilicate nanoclay on polyester composites’ static and dynamic mechanical properties. Polymer Composites, 43(10), 7290–7302. https://doi.org/10.1002/pc.26794
- Shettar, M., Doshi, M., & Rawat, A. K. (2021, Sep). Study on mechanical properties and water uptake of polyester-nanoclay nanocomposite and analysis of wear property using RSM. Journal of Materials Research and Technology, 14, 1618–1629. https://doi.org/10.1016/j.jmrt.2021.07.034
- Shettar, M., Kowshik, S., Gowrishnakar, G., Hiremath, P., & Sharma, S. (2022, Mar). Water sorption-desorption-resorption effects on mechanical properties of epoxy-nanoclay nanocomposites. International Journal of Automotive and Mechanical Engineering, 19(1), 9478–9486. https://doi.org/10.15282/ijame.19.1.2022.11.0730
- Shettar, M., Shettigar, P., Manjunath, M., & Rao, U. S. (2020, Jul). Study on effect of water soaking conditions on properties and morphology of glass fiber–cement–polyester composites. Journal of Materials Research and Technology, 9(4), 8697–8704. https://doi.org/10.1016/j.jmrt.2020.05.117
- Srinivas, D., Shankar, G., Sharma, S., Shettar, M., & Hiremath, P. (2022). Artificial neural network for predicting hardness of multistage solutionized and artificially aged LM4 + TiB2 composites. Materials Research, 25. https://doi.org/10.1590/1980-5373-mr-2021-0557
- Srinivas, D., Sharma, S., Nayak, R., Kumar, N., & Shettar, M. (2022, Oct). Optimization and prediction of the hardness behaviour of LM4 + SI3N4 composites using RSM and ANN - a comparative study. Journal of Applied Engineering Science, 20(4), 1–12. https://doi.org/10.5937/jaes0-38109
- Yang, X. -S., & Deb, S. (2010). Engineering optimisation by cuckoo search. International Journal of Mathematical Modelling and Numerical Optimisation, 1(4), 330. , May, [Online]. Available. https://doi.org/10.1504/IJMMNO.2010.035430