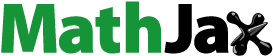
Abstract
This study investigates the relationship between four welding input parameters (voltage, current, heat input, and welding speed) and the ultimate tensile strength (UTS) and yield strength (YS) of a welded joint. The work joined two super-duplex stainless-steel pipes of outside diameter 0.630 inches (16.002 mm) and a thickness of 0.065 (1.651 mm), using Gas Tungsten Arc Welding. Factorial design was used to ascertain the main and interaction effects of the welding input parameters on the UTS and YS of the welded joint. Results revealed a significant interaction between voltage, current, welding speed and heat input for UTS, and a significant interaction between the welding speed and heat input for YS. Results further revealed that as voltage increases from 9.3 V to 11.6 V, the UTS decreases by 2.69 MPa, while YS increases significantly by 24.25 MPa. When the current increases from 30 A to 38 A, the UTS increases by 10.81 MPa, while the YS increases by 4.25 MPa. In the case of the welding speed, an increase from 0.46 to 0.71 mm/s results in a decrease of the UTS by 7.94 MPa and a significant increase of the YS by 33.25 MPa. When the heat input increases from 0.54 to 0.70 kJ/mm, the UTS decreases by 15.06 MPa, whereas the YS decreases significantly by 35.25 MPa. Also, the optimum voltage, current, welding speed and heat input for UTS are 11.60 V, 30.0 A, 0.71 mm/s, and 0.70 kJ/mm, respectively, and those of YS are 9.30 V, 30.0 A, 0.46 mm/s, and 0.70 kJ/mm, respectively.
1. Introduction
Super duplex stainless steel (SDSS) is a type of stainless steel that has exceptional mechanical properties due to its ferrite and austenite compositions (Eghlimi et al., Citation2014). SDSS has in recent years gained popularity in major industries like oil and gas, marine, petrochemical, chemical processing, and equipment manufacturing industries owing to its enhanced chemical composition, consisting mainly of austenite and ferrite (Yousefieh et al., Citation2011). These constituents produce extraordinary mechanical properties like high corrosion resistance, good formability and weldability, stress corrosion cracking resistance, and relative affordability when compared to superior performance alloys like nickel-based alloys and super-austenitic stainless steels. SDSSs are produced in various shapes and forms, such as tubes, sheets, bars, angles, wires, plates, rods, and pipes for different applications. SDSS pipes are used for high-pressure applications to transport fluids in various industries. Transportation of fluids to relatively long distances requires the joining of the pipes which come in standard lengths. Joining is predominantly by welding, and engineers are usually faced with the challenge of pipes failure at the welded joints (Ramkumar et al., Citation2015). Various welding techniques exist, such as gas metal arc welding (GMAW), submerged arc welding (SAW), gas tungsten arc welding (GTAW) and flux-cored arc welding (FCAW). Among the various methods, gas tungsten arc welding (GTAW) is one of the most preferred welding procedures for SDSS pipes because it affords welders control over heat input and dilution, and also high reliability, versatility, high quality, affordability, and productivity (Madadi et al., Citation2011).
GTAW requires certain input parameters to achieve desirable mechanical properties after weld (Baghel & Gupta, Citation2022). Several researchers have indicated that the input parameters of the GTAW process have a great influence on mechanical properties like hardness, ultimate tensile strength, yield strength, and corrosion resistance of the SDSS (Bhatt, Citation2018; Prabaharan et al., Citation2014; Rajendra & Arakerimath, Citation2015; Yousefieh et al., Citation2011). For instance, Rajendra and Arakerimath (Citation2015) found that the current has the greatest influence on hardness among three parameters: current (44.60%), voltage (38.59%) and gas flow rate (16.63%). Yousefieh et al. (Citation2011) found pulsed current to have the greatest effect on corrosion resistance among four parameters: pulsed current (66.28%), background current (25.97%), % on time (2.71%) and pulse frequency (5.04%). Inaccurate selection of welding input parameters on the part of welders leads to the failure of SDSS under high pressure, resulting in an unfavorable rise in the energy, and cost of the welding process (Yang et al., Citation2014). Kutelu et al. (Citation2018) reported that welded joints of steels could experience mechanical failure and corrosion vulnerability when the input welding parameters are not optimally combined. Consequently, optimizing GTAW process parameters becomes an important area of focus that is essential for obtaining high-quality and durable welds in the manufacturing industry. Important mechanical properties that should be maximized to attain high-quality weld are the tensile strengths of the material. Several researchers have studied the influence of welding process parameters on the ultimate tensile strength of the welded material. Patil and Waghmare (Citation2013) studied the effect of current, voltage, and welding speed on the ultimate tensile strength of AISI 1030 mild steel material using Orthogonal array and analysis of variance. They found out that current and welding speed were a major influence on the UTS of the welded joint. Bhatt (Citation2018) studied the effect of current and gas flow rate on the UTS of aluminium alloy. The methodology deployed non-destructive testing including visual inspection and dye penetration test and found that current and gas flow rate has a direct bearing on the UTS of the aluminium alloy. Yousefieh et al. (Citation2012) reported on the effect of pulse current and background current on the UTS of SDSS in pulsed current gas tungsten arc welding. Taguchi method was used to optimize the pulse current. Their study revealed that current has a great influence on the UTS of the weld. Baloyi et al. (Citation2021) investigated the effect of voltage, current and gas flow rate on the UTS of AISI1008 mild steel plates using Taguchi method and adaptive neurofuzzy inference system. The study revealed that voltage had the strongest influence on UTS, followed by current and gas flow rate. Roth et al. (Citation2020) studied the effect of heat input in a welding process on the heat-affected zone of the weld specimen. Moon et al. (Citation2013) studied the effect of heat input/treatment of GTAW on SDSS (UNS S32750). Mechanical properties studied were pitting corrosion resistance, sigma change precipitation and microstructural change. The study showed that varying heat input affects the listed mechanical properties of the SDSS material. For instance, the pitting corrosion resistance improved as the heat treatment was increased from 930°C to 1080°C to 1230°C. Paulraj and Garg (Citation2015) investigated the impact of heat input, shielding gas temperature and interpass temperature on the tensile strength, hardness and impact toughness of SDSS (UNS S32750). They found that an increase in heat input and interpass temperature decreased the tensile strength, hardness and impact toughness of the SDSS while an increase in Nitrogen content of shielding gas caused an increase effect. Judging from the above review, it can be said that; (1) voltage, current, speed, heat input and gas flow rate influence the tensile strength of the welded material, (2) further studies need to be done to study the interaction/combined effects of the welding parameters on the tensile strength of the steel materials. Factorial design has been successfully used by researchers to expose interaction effects of input parameters on process responses (Andoh et al., Citation2013, Citation2022; Davis et al., Citation2007). The objective of this study is to investigate the relationship between the welding parameters and the strength of the welded material in order to: (1) determine the main and interaction/combined effects of GTAW process input parameters (voltage, current, welding speed and heat input) on the UTS and YS of superduplex stainless steel using factorial design, (2) generate a quantitative model to predict the UTS and YS of welded material, and (3) optimize the input parameters of the gas tungsten arc welding process.
2. Materials and methods
2.1. Material
ASTM A789-UNS S32750 super-duplex tube with an outside diameter of 0.630 inches (16.002 mm) and a wall thickness of 0.065 inches (1.651 mm) was employed as the specimen to be joined by welding and the strength of the welded joint studied. The SDSS material is selected due to its wide application in chemical processes that require corrosion resistance. The filler metal used for this experiment was exaton 25.10.4.L. This filler rod was selected from the welding procedure specification (WPS) ORS-SDP-BW−001. This is due to its corrosion resistance and mechanical strength for binding the ASTM A789-UNS S32750 SDSS tube in the GTAW process. A WPS is a formally written document that guides the welding process to meet standard requirement for welding (Blavatsky, Citation2019). Tables present the mechanical properties and chemical composition of the welding and welded metal.
Table 1. Mechanical properties of superduplex stainless steel (UNS S32750)
Table 2. Chemical composition of superduplex stainless steel (UNS S32750)
2.2. Selection of parameters
Current, voltage, weld speed and heat input were selected as input parameters to the GTAW equipment. The WPS ORS-SDP-BW−001 manual defines acceptable ranges of voltage, current, weld speed, and heat input that are selected as input parameters for the GTAW process. The WPS is generated by a welding procedure qualification process that is legally backed by Article II of Section IX of the ASME Boiler and Pressure Vessel Code (Blavatsky, Citation2019). The study employed a 24 factorial design in analysing the welding conditions of voltage, current, weld speed and heat input. Each parameter had two levels that are coded as high (+) and low (-). Table displays the levels of welding input parameters as derived from the WPS ORS-SDP-BW−001 manual. The 24 factorial design was run as a completely randomized design.
Table 3. Values of welding input parameter
2.3. Experimental method
The experimental work was carried out in two stages. The first stage was the welding process, where two superduplex stainless steel tubes were welded together. The second stage was the testing process, which included non-destructive tests (NDTs) and destructive tests (DTs). The non-destructive test consisted of an x-ray radiography test, to ensure that there were no defects at the weld joint. For the destructive test, the welded joints were taken through tensile tests to determine the ultimate tensile strength and yield strength using a tensile testing machine. Data was extracted from an attached computer as the equipment was computer-controlled and interfaced with a software to facilitate the collection of data.
2.3.1. Sample preparation
The preparation of the sample starts with placing two superduplex stainless steel tubes in a screw jack (Figure ). The round edges of both tubes to be welded together are prepped to form a butt-v joint. This prepping enables the weld bead to sit well, thereby ensuring a solid weld. The combination of heat input of 0.54 kJ/mm, welding speed of 0.46 mm/s at a voltage of 9.3 V and a current of 30 A were used as the first set of welding input parameters from the 16 factorial points (Table ) and keyed into the GTAW equipment. The weld is then carefully executed at the selected weld speed parameter using exaton 25.10.4.L as filler material as depicted in Figure .
Figure 1. (a) Schematic of the welding setup (b) Photograph of gas tungsten arc welding of super-duplex stainless-steel pipe.

Table 4. Factorial points of welding input parameters
The procedure was repeated for the remaining fifteen (15) factorial points. To avoid bias, the remaining fifteen (15) points were randomly selected. In all thirty-two (32) samples were prepared representing two sets of run for each factorial point. The thirty-two (32) welded samples are then subjected to destructive testing. Some of the other parameters used in the welding process are as presented in Table .
Table 5. Other parameters of the welding process
2.3.2. Mechanical testing
Two sets of tests were performed on the samples to ascertain the ultimate tensile strength and yield strength. Response data were recorded from the mechanical test using a tensile testing instrument. The data collected were then used to generate the mathematical model that evaluates the UTS and YS of welded superduplex stainless steel. Figure presents a pictorial view of the mechanical testing setup.
The tensile testing procedure involves a mechanical test in which a tensile force is applied to both ends of a material specimen. This test is used to determine a material’s tensile strength and other tensile properties. Tensile tests were conducted at room temperature utilizing the tensile testing instrument, interfaced with a computer software. The specimen to be tested is held between the machine’s two crossheads as depicted in Figure . The wedge grip type that is selected is dependent on the material’s geometry and dimensions. The force is gradually increased, and the specimen elongates during the testing process. It is repeated until the specimen fractures. The applied crosshead speed and the initial strain rate were set to 5 mm/min and 0.1/min, respectively, on the computer to determine the specimens’ extension during testing. The resulting ultimate tensile strength and yield strength are determined and recorded. The procedure is repeated for the remaining 15 specimens which are selected at random to avoid bias.
2.3.3. Experimental results
Table presents the average response of ultimate tensile strength and yield strength for the 16 factorial points in which the joint welds were tested in the tensile testing machine. Sixteen factorial points because of four input parameters (heat input, welding speed, voltage, current) conducted at two levels, making 24 = 16 experimental points. The Run 1 and Run 2 columns represent the first and second set of results, respectively, for both UTS and YS, while the mean column computes the average of Run 1 and Run 2 set of results. Execution of weld per factorial point was randomized to eliminate bias, as shown in column “Run Order”.
Table 6. Average response for the ultimate tensile strength and yield strength
3. Analysis and discussion of results
3.1. Non-destructive tests
Table presents the results of X-ray radiography conducted during non-destructive testing of the welded specimen. It can be observed from all the x-ray radiographs that there were no effects detected for the 16 weld specimens. This suggests that the range of values selected for the various welding parameters produced good welded joints. It is necessary to ensure that the weld specimen had no defects or fractures to obtain credible tensile testing results.
Table 7. X-ray radiography tests
3.2. Calculation of effects
Four variables, namely, heat input (a in kJ/mm), welding speed (b in mm/sec), voltage (c in volts) and current (d in amps) were used to investigate the effects of TIG welding on superduplex stainless steel specimen (ASTM A789-UNS S32750). Table present coded level of input parameters. The minus sign represents the low level and a plus sign the high level for both the UTS and YS responses. Levels and their corresponding values are already presented in Table .
Table 8. Coded level with average UTS and YS response
3.2.1. Computation of effects and standard error
There are four main effects, six 2-factor interaction effects, four 3-factor interaction effects, and a 4-factor interaction effect. The main effect of each of the process factors reflects the changes of the respective responses as the process factors change between low level and high level. Equations for computation of effects and standard error are referenced from Box et al. (Citation1979).
The main effect of voltage is:
The main effect of current is:
The main effect of welding speed is:
The main effect of heat input is:
The combined effect of two or more factors is referred to as interaction effects. The interaction effects of the six 2- factor interactions are given as follows.
The interaction effect of voltage-current is defined as:
The interaction effect of voltage-welding speed is defined as:
The interaction effect of voltage-heat input is defined as:
The interaction effect of current-welding speed is defined as:
The interaction effect of current-heat input is defined as:
The interaction effect of welding speed-heat input is defined as:
The interaction effects of the four 3- factor interactions are given as follows.
The interaction effect of voltage-current-welding speed is defined as:
The interaction effect of voltage-current-heat input is defined as:
The interaction effect of voltage-welding speed-heat input is defined as:
The interaction effect of current-welding speed-heat input is defined as:
The interaction effect of the 4- factor interactions is given below.
The interaction effect of voltage-current-welding speed-heat input is defined as:
The mean of the runs is defined as:
where are the responses (ultimate tensile strength and yield strength).
When replicates are made under a particular set of experimental conditions, the variation between the related observations is utilized to calculate the standard deviation of a single observation and thus the standard deviation of the findings. In general, if sets of experimental parameters are repeated and the
replicate runs made at the
set generates an estimated
having
degree (s) of freedom (Box et al., Citation1979), the run variance is estimated as
With only replicates at each of the
sets of conditions, the formula for the
variance reduces to:
With , where
is the difference between the duplicate observations for the
set of conditions. Thus, Equationequation 17
(17)
(17) becomes:
If a total of N runs is made when conducting a replicated factorial design, the variance of an effect is given as:
And the standard error of the effect is given as:
There were four main effects, voltage (V), current (I), welding speed (S), and heat input (Q), six 2-factor interaction effects, four 3-factor interaction effects, and one 4-factor interaction effect. The findings are tabulated and presented in Table .
Table 9. Coefficient of analysis for ultimate tensile strength and yield strength
The first column presents a list of welding parameters (both individual and combined), while their corresponding various effects on UTS and YS are shown in column two. The third and fourth columns present coefficients and standard error coefficients of the parameters in the predicted model.
3.2.2. Identification of main and interaction effects
Figure presents the plot of the main effects of voltage, current, welding speed, and heat input on UTS. The main effect plot shows the degree of effect within the low and high levels. It can be seen from the graph that UTS increased with increasing current and decreased with increasing voltage, welding speed and heat input. UTS increased from 740.5 MPa to 751.312 MPa when current increased from 30 A to 38 A. UTS decreased from 747.25 MPa to 744.562 MPa when voltage increased from 9.3 V to 11.6 V. UTS decreased from 749.875 MPa to 741.937 MPa when welding speed increased from 0.46 mm/s to 0.71 mm/s. UTS decreased from 753.437 MPa to 738.375 MPa when heat input increased from 0.54 kJ/mm to 0.70 kJ/mm. The implication of the above results is that varying the welding input parameters (voltage, current, welding speed and heat input) causes a significant change in the UTS of the welded joint. This is consistent with Patil et al., (2013).
Figure presents the plot of the main effects of voltage, current, welding speed, and heat input on YS. It can be seen from the graph that YS increased with increasing voltage and welding speed and decreased with increasing welding speed and heat input. YS increased from 432.125 MPa to 456.375 MPa when voltage increased from 9.3 V to 11.6 V. YS decreased from 446.375 MPa to 442.125 MPa when current increased from 30 A to 38 A. YS increased from 427.625 MPa to 460.875 MPa when welding speed increased from 0.46 mm/s to 0.71 mm/s. YS decreased from 461.875 MPa to 426.625 MPa when heat input increased from 0.54 kJ/mm to 0.70 kJ/mm. The implication of the above results is that there is a significant difference in the YS of the welded joint when current, voltage, welding speed, and heat input are varied within their respective ranges.
The two-way interactions evaluated in Figures demonstrate how interactions of independent variables affect the dependent variable. The broken lines reflect low values of the independent variables, while the solid lines reflect high values. From Figures , the combined effect of welding speed and heat input has the greatest influence on UTS and YS. This is because the lines of interaction deviate substantially from being parallel. It can be seen from the interaction plots of UTS that all the two-factor interactions are nonparallel, revealing that there is an interaction between the parameters. In the interaction plot for YS, all the two-factor interactions are nonparallel except current-heat input. This implies that only current-heat input do not interact under the YS plot.
Figures show a Pareto chart of the standardized effects of voltage, current, welding speed, and heat input, with their interactions on the UTS and YS, respectively, at a significance level of 95 %. The Pareto chart is used to verify the significance of the effects of the independent variable on the dependent variable. The effect is deemed significant when it crosses the reference standardized effect of 2.12, and insignificant when it does not cross the line. It could be seen from Figure that every other effect on the UTS Pareto chart is significant except BCD (current-welding speed-heat input) and A (voltage), having absolute standardized effects of 2.09 and 1.84 respectively. The highest standardized effect (19.80) on UTS was that of the 4-factor interaction, ABCD (voltage-current-welding speed-heat input). In Figure , every other effect on YS is significant except for B, BCD, ABCD, and BD, having absolute standardized effects of 2.09, 1.48, 0.99, and 0.74, respectively. The highest effect on YS was CD (welding speed-heat input), with an absolute standardized effect of 33.30.
3.3. Generation of prediction model
The prediction model involves the main effects and the interaction effects of the input parameters under consideration. A full 24 factorial design consists of: four main effects, six 2-way interaction effects, four 3-way interaction effects, and one 4-way interaction effect, resulting in 15 equation terms as depicted in Equationequation 22(22)
(22) . Where y is the predicted response,
to
are the coefficients for the various effects and
is the mean response value as derived in Table .
Also; ;
;
;
;
;
;
;
;
;
;
;
;
;
;
and is the experimental error. The experimental error is given by the relation
Where N is the number of runs of the experiment, t is the distribution, is the confidence level, v is the n – 1 degree of freedom, and
is the pool variance, defined as:
Where is the difference between the duplicate observations for the set of conditions, and n is the number of experimental sets of conditions. The significant effects and interactions are used to develop the empirical models for the response with the deployment of Equationequations 22
(22)
(22) , Equation23
(23)
(23) and Table .
Thus, using the corresponding coefficients in Table , the predictive model for ultimate tensile strength (UTS) and yield strength (YS) are given as Equationequations 25(25)
(25) and Equation26
(26)
(26) respectively.
For 2 replicates at each of the sets of conditions, N is 32 and v is 1. Substituting the values into Equationequation 24(24)
(24) , the pool variance is 4.13. Applying 95 % confidence level,
is 12.7. Substituting the values into Equationequation 23
(23)
(23) , the experimental error was calculated to be
3.27 for UTS and
4.55 for YS. Coefficient of determination (R2) analysis was used to test the predictive models. The higher the R2 value, the better the model fits the experimental data (Chicco et al., Citation2021). A high R2 value suggests that the models are accurate and can be used to make further predictions of UTS and YS. For the data used in this study, the R2 value obtained for UTS and YS were 98.63 % and 99.43% respectively. These R2 values indicate that; both the UTS and YS models provide good fits for the data. Predicted models were validated to determine if the experimental results fall within the predicted values of the UTS and the YS. A predicted model is deemed accurate when the experimental values fall within the range of the predicted values (Box et al., Citation1979). The results obtained for both the experimental and predicted models of UTS and YS are presented in Tables respectively.
Table 10. Comparison of experimental values with predicted values of ultimate Tensile strength
From Table , it can be seen that 87.5% of the experimental values fall within the predicted values. This implies that the predictive model is accurate in predicting the UTS. It can also be seen from Table that 93.75% of the experimental values fall within the predicted values, making the predictive model of YS accurate in predicting the YS.
Table 11. Comparison of experimental values with predicted values of Yield Strength
3.3.1. Optimization of ultimate tensile strength and yield strength
To find the optimum conditions for UTS and YS, the experimental data were fed into the Minitab software to generate optimization plots for the UTS and YS as presented in Figures respectively. The optimization programme displays the optimum conditions in red for each input parameter. It can be seen from Figure that the optimal welding input parameters are 11.60 V (voltage), 30.0 A (current), 0.71 mm/s (welding speed), and 0.70 kJ/mm (heat input), yielding in an optimum UTS of 707.5 MPa as displayed in blue on the plot. Also, Figure reveals optimal input parameters of 9.30 V (voltage), 30.0 A (current), 0.46 mm/s (welding speed), and 0.70 kJ/mm (heat input), generating an optimum YS of 329.0 MPa.
4. Conclusion
This study performed gas tungsten arc welding of UNS S32750 and subjected it to non-destructive x-ray radiography test and destructive tensile test. The objectives were to: (1) determine the effects of GTAW process input parameters (voltage, current, welding speed and heat input) on the UTS and YS of welded superduplex stainless steel using factorial design, (2) generate a quantitative model to predict the UTS and YS of the welded material, and (3) optimize the input parameters of the gas tungsten arc welding process. The main and interaction effects of the input parameters on the welded material have been identified using factorial design. The study has developed quantitative models to predict the UTS and YS of welded SDSS. Optimization of the input parameters has also been accomplished. Results revealed that voltage, current, welding speed and heat input have effects on the UTS and YS of the welded SDSS. The following conclusions are drawn based on the range of welding input parameters considered.
The range of welding parameters selected produced a welded joint that had no fractures and defects.
The UTS of the welded joint revealed a significant interaction between the voltage, current, welding speed and heat input and a little interaction between the current, weld speed and heat input.
The YS of the welded joint revealed a significant change when the welding input parameters were varied within their respective ranges.
The YS of the welded joint revealed a significant interaction between the welding speed and heat input and a little interaction between the current and heat input.
The quantitative models accurately predict the UTS and YS of the welded SDSS with R-squared values of 94.5% and 97.72%, respectively.
The optimum welding input parameters required to achieve the best UTS of the welded SDSS are 11.60 V (voltage), 30.0 A (current), 0.71 mm/s (welding speed), and 0.70 kJ/mm (heat input), whereas that of YS are 9.30 V (voltage), 30.0 A (current), 0.46 mm/s (welding speed), and 0.70 kJ/mm (heat input).
Disclosure statement
No potential conflict of interest was reported by the authors.
References
- Andoh, P. Y., Davis, F., & Adablah, G. (2022). Research paper selection of optimum pouring conditions for improvement of Aluminum Alloy. Castings in Ghana, 41(2), 1–21.
- Andoh, P. Y., Davis, F., & Fiagbe, Y. A. K. (2013). Monitoring of Carbon Dioxide production in a Carbonated beverage company using factorial design technique. International Journal of Scientific & Technology Research, 2(10). https://www.ijstr.org/paper-references.php?ref=IJSTR-1013-7418
- Baghel, P. K., & Gupta, T. (2022). Optimization of parameters of pulse current gas tungsten arc welding using non conventional techniques. Journal of Advanced Joining Processes, 6(July), 100124. https://doi.org/10.1016/j.jajp.2022.100124
- Baloyi, P., Akinlabi, S. A., Madushele, N., Adedeji, P. A., Hassan, S., Mkoko, Z., & Akinlabi, E. T. (2021). Materials Today: Proceedings Two-staged technique for determining ultimate tensile strength in MIG welding of mild steel. Materials Today: Proceedings, 44, 1227–1234. https://doi.org/10.1016/j.matpr.2020.11.244
- Bhatt, H. (2018). Study of effect of process parameters of welding during TIG welding of AA 7075 and its optimization. International Journal of Applied Engineering Research, 13(12), 10658–10663. http://www.ripublication.com/
- Blavatsky, H. P. (2019). Section IX welding brazing and fusion qualification. The Secret Doctrine, 91–97. https://doi.org/10.1017/cbo9780511978371.011
- Box, G. E. P., Hunter, W. G., Hunter, J. S., & Hunter, J. S. (1979). Statistics for experimenters: An introduction to design, data analysis, and model building. Journal of Marketing Research, 16(2), 291. https://doi.org/10.2307/3150696
- Chicco, D., Warrens, M. J., & Jurman, G. (2021). The coefficient of determination R-squared is more informative than SMAPE, MAE, MAPE, MSE and RMSE in regression analysis evaluation. Peer Journal Computer Science, 7, 1–24. https://doi.org/10.7717/PEERJ-CS.623
- Davis, F., Ansong, L. E., Andoh, P. Y., & Owusu-Ofori, S. P. (2007). Quasi-automatic monitoring system for turning operation using acoustic emission signal response and factorial design method. Journal of Science and Technology (Ghana), 27(3). https://doi.org/10.4314/just.v27i3.33063
- Eghlimi, A., Shamanian, M., & Raeissi, K. (2014). Effect of current type on microstructure and corrosion resistance of super duplex stainless steel claddings produced by the gas tungsten arc welding process. Surface and Coatings Technology, 244, 45–51. https://doi.org/10.1016/j.surfcoat.2014.01.047
- Kutelu, B. J., Seidu, S. O., Eghabor, G. I., & Ibitoye, A. I. (2018). Review of GTAW welding parameters. Journal of Minerals and Materials Characterization and Engineering, 06(5), 541–554. https://doi.org/10.4236/jmmce.2018.65039
- Madadi, F., Ashrafizadeh, F., & Shamanian, M. (2011). Optimization of pulsed TIG cladding process of stellite alloy on carbon steel using RSM. Journal of Alloys and Compounds, 510(1), 71–77. https://doi.org/10.1016/j.jallcom.2011.08.073
- Moon, I., Jang, B., & Koh, J. (2013). Heat treatment effect on pitting corrosion of super duplex stainless steel UNS S32750 GTA welds. Advanced Materials Research, 746, 467–472. https://doi.org/10.4028/www.scientific.net/AMR.746.467
- Patil, S. R., & Waghmare, C. A. (2013). Optimization of Mig Welding Parameters for Improving Strength of Welded Joints Address for Correspondence. International Journal of Advanced Engineering Research and Studies, II(July–Sept.2013), 14–16. http://www.technicaljournalsonline.com/ijaers/index.html
- Paulraj, P., & Garg, R. (2015). Effect of welding parameters on mechanical properties of GTAW of UNS S31803 and UNS S32750 weldments. Manufacturing Review, 2, 29. https://doi.org/10.1051/mfreview/2015032
- Prabaharan, C., Venkatachalam, P., Suresh Kumar, K., & Lenin, K. (2014). Parametric optimization of gas tungsten arc welding processes by using factorial design approach. Journal of Scientific and Industrial Research, 73(6), 415–420.
- Rajendra, S., & Arakerimath, R. R. (2015). Parameter optimization of tig welding using austenitic stainless steel. International Journal of Innovative and Emerging Research in Engineering, 2(1), 120–124.
- Ramkumar, D., Mishra, D., Ganesh Raj, B., Vignesh, M. K., Thiruvengatam, G., Sudharshan, S. P., Arivazhagan, N., Sivashanmugam, N., & Rabel, A. M. (2015). Effect of optimal weld parameters in the microstructure and mechanical properties of autogeneous gas tungsten arc weldments of super-duplex stainless steel UNS S32750. Materials and Design, 66(PA), 356–365. https://doi.org/10.1016/j.matdes.2014.10.084
- Roth, S., Hezler, A., Pampus, O., Coutandin, S., & Fleischer, J. (2020). Influence of the process parameter of resistance spot welding and the geometry of weldable load introducing elements for FRP/metal joints on the heat input. Journal of Advanced Joining Processes, 2(June), 100032. https://doi.org/10.1016/j.jajp.2020.100032
- Yang, J., Wang, Q., Wei, Z., & Guan, K. (2014). Weld failure analysis of 2205 duplex stainless steel nozzle. Case Studies in Engineering Failure Analysis, 2(2), 69–75. https://doi.org/10.1016/j.csefa.2014.05.001
- Yousefieh, M., Shamanian, M., & Arghavan, A. R. (2012). Analysis of design of experiments methodology for optimization of pulsed current GTAW process parameters for ultimate tensile strength of UNS S32760 welds. Metallography, Microstructure, and Analysis, 1(2), 85–91. https://doi.org/10.1007/s13632-012-0017-9
- Yousefieh, M., Shamanian, M., & Saatchi, A. (2011). Optimization of the pulsed current gas tungsten arc welding (PCGTAW) parameters for corrosion resistance of super duplex stainless steel (UNS S32760) welds using the Taguchi method. Journal of Alloys and Compounds, 509(3), 782–788. https://doi.org/10.1016/j.jallcom.2010.09.087