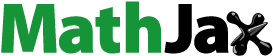
Abstract
Sustainability has become a major industry concern in the last decade to maintain the prosperity of current and future generations. A closed-loop supply chain (CLSC) is considered in line with sustainability because it involves the return of used products in manufacturing, which will reduce end-of-life product waste and the use of raw materials. This research has two contributions to the CLSC literature. First, demand and the quantity of returned products are estimated using the Bass model with a certain return rate, which will become the input of the product mix decision model. Second, carbon caps and trade policy is considered in the model to achieve sustainability. The analysis showed that carbon bought and sold are significantly affected by carbon cap threshold, manufacturing cost, and remanufacturing cost in both low- and high-return cases. The profit was not sensitive to the change in carbon cap threshold and remanufacturing cost but was significantly impacted by the change in manufacturing cost.
1. Introduction
Sustainability has become a major concern in the manufacturing industry in the last decade. The manufacturing company has to shift its focus from single consideration in terms of economics to triple bottom lines. Those additional considerations consist of social and environmental. Due to the rise of global environmental consciousness, manufacturing company has to increase their efficiency in raw material uses and energy consumption and develop strategies to decrease carbon emission, which has been acknowledged as the major source of climate change. According to the results of COP 26, the participant countries of the United Nations Climate Change Conference have agreed to limit the rise of the earth’s temperature to 1.5°C in 2060. In this context, the closed-loop supply chain (CLSC) is considered an effective way to lower the impact of industrial activities on the environment.
In a CLSC, once a product enters its end of life, it will be returned by consumers to the manufacturers for further reconditioning, repairing, or remanufacturing (Ijomah, Citation2009). Industries that fulfill demand from manufacturing and remanufacturing production are known as the hybrid manufacturing-remanufacturing production system. Carbon emission policies are also often used to achieve sustainability. Three carbon emission policies are widely adopted in developed countries: cap and trade, strict cap, and carbon tax. The government sets a carbon cap as the allowable limit on carbon emissions in a cap and trade policy. Then, the companies can sell the surplus of their carbon emissions or buy the carbon when the emitted carbon exceeds the cap.
One of the important decisions that should be made when an industry implements a hybrid manufacturing-remanufacturing system is how to determine the quantities of new and remanufactured products. In this paper, we developed an optimization model to determine the optimal product mix that maximizes the company’s net profit. The model can be used for an industry that implements a hybrid manufacturing-remanufacturing system by considering carbon caps and trade policy. The Bass model is used in this research to estimate the product demand and the quantity of returned products from consumers. The output resulting from the Bass model will be used as the input data for the mathematical optimization model.
The rest of this paper is organized as follows. Section 2 presents a literature review on the hybrid manufacturing-remanufacturing systems model and carbon emission policies. In Section 3, we describe the system description. Section 4 presents the model assumptions, notations, and model formulation. In Section 5, a numerical example is given to illustrate the models’ implementation along with the sensitivity analysis results. Lastly, the conclusions are drawn in Section 6.
2. Literature review
Many previous studies have discussed the implementation of a CLSC. In recent years, there has been a growing interest in CLSC issues in terms of reverse logistics, recycling, remanufacturing, and reusing of products or components due to the environmental concerns, economic issues, and legal obligations so that companies should take into account the recovery options, such as recycling and remanufacturing, while in the same time preparing their tactical plans (Subulan & Tasan, Citation2013). Remanufacturing is a viable way to prolong the useful life of an end-of-use product or its components. Despite its economic, environmental, and social benefits, remanufacturing is associated with many challenges related to core (used product or its components) availability, timing, and quality (Kurilova-Palisaitiene et al., Citation2018). The configuration of the reverse logistics network is complex comprising the determination of the optimal sites and capacities of collection centers, inspection centers, remanufacturing facilities, and recycling plants, including the product price and advertising decisions (Alumur et al., Citation2012; Asghari et al., Citation2022). Another aspect that makes the configurations more complex is the reshoring decisions.(Kim & Chung, Citation2022). The model helps a decision-maker to decide whether the production facilities must be relocated to the home country or still in the host country.
A CLSC model has been developed in Jeihoonian et al. (Citation2017) with remanufacturing for a single modular product, and a fuzzy multi‐objective mixed integer linear programming (FMOMILP) model was proposed in Pourjavad & Mayorga (Citation2018) to simultaneously minimize cost and environmental effects and maximize social impacts for a multi‐echelon and multi‐period CLSC network. In a multi-product closed-loop system environment, a model has been developed by Shi et al. (Citation2011) where the manufacturer has two channels for supplying products: producing brand-new products and remanufacturing the returned products into as-new ones. In a more complex problem with a similar environment (Shi et al., Citation2011), a model has been developed by incorporating uncertainty in the case of tire and shrimp products (Fatthollahi-Fard et al., Citation2021; Mosallanezhad et al., Citation2021). Another research by Subulan & Tasan (Citation2013) developed a mathematical model to focus primarily on integrating remanufacturing as a recovery option into the tactical planning process. A product mix optimization model was developed by Bagalagel & ElMaraghy (Citation2020) in Industry 4.0 with a hybrid manufacturing-remanufacturing system. The research aims to determine the optimal number of the product mix in such a manufacturing-remanufacturing system to maximize profit (Bagalagel & ElMaraghy, Citation2020).
An integrated optimization model for CLSC, which produces new and reconditioned products, has been developed by Gaur et al. (Citation2017) with a real-world case study of a battery manufacturer. Zhong et al. (Citation2017) established a hybrid manufacturing-remanufacturing production decision model for the product in a stable market environment by considering the update cycle of the product’s life cycle. An optimization model has been proposed to solve the problem of manufacturing-remanufacturing—transport–warehousing CLSC (Turki et al., Citation2017). The company is assumed to have two machines for manufacturing and remanufacturing, manufacturing stock, purchasing warehouse, transport vehicle, and recovery inventory. Three models have been developed by Su et al. (Citation2018) to study the decision mechanism in a CLSC with different players (retailer, a third-party service, or retain it exclusively) that were selected to collect the used product.
Commonly, the quality and quantity of the returned products in a CLSC are considered as the input parameters (Zeballos et al., Citation2018). A model was proposed by Wang et al. (Citation2017a, Citation2017b) to determine the number of components used in remanufacturing using Bass’ model, a product diffusion dynamics approach widely used to estimate the demand for a new product. The model has been used to predict the demand quantity of fashion (Tashiro, Citation2016), automotive (Fan et al., Citation2017), and solar water heaters (da Silva et al., Citation2020). In Rosyidi (Citation2021), a framework was proposed to implement the models of Wang et al. (Citation2017a, Citation2017b) in predicting the demand and the returned product. Hence, their quantity has a quantitative justification rather than being treated as a given input parameter.
According to Avenyo & Tregena (Citation2022), China emitted the highest carbon dioxide compared to other countries such as Russia, South Africa, and India. This condition was resulted from the large population of China and the used of high level intensive productions. In average, the carbon emissions from the countries under study were more than 200,000 kilotons. Based on the data total Green House Gas emissions of Indonesia in 2012 were estimated at 1,454 million metric tons of carbon dioxide equivalent MtCO2e. Those study showed that many efforts must be made to globally reduce the carbon emissions. The research of Lamba & Singh, (Citation2019) and Lamba et al. (Citation2019) strengthened the importance of minimizing carbon emissions in a supply chain by determining the optimal combination of suppliers and their corresponding lot sizes. Using the same policies, Fareeduddin et al. (Citation2015) developed an optimization model in a CLSC. A model was developed to design a logistics network in an environmentally friendly hybrid manufacturing-remanufacturing system industry by considering carbon emissions that include carbon emissions during the manufacturing/remanufacturing process and carbon emissions in transportation (Wang et al., Citation2013). In this research, we develop an optimization model to determine the product mix of a hybrid manufacturing-remanufacturing system in a single time horizon. The model is formulated using linear programming to maximize the profit. The quantity of the returned products will be estimated using the Bass model. This model also considers the carbon cap and trade policy. The decision variables in this model include the production quantity of the products by regular manufacturing, the number of products by remanufacturing, the quantity of discarded products, and the quantity of carbon emission, either bought or sold.
Other researchers in this area developed meta-heuristics and algorithms to solve the model efficiently. Several researchers implemented a hybrid algorithm to solve the models. Some of the interesting hybrid algorithms are the hybrid genetic algorithm and simulated annealing (Li et al., Citation2019), the combination of the red deer algorithm and whale optimization algorithm with genetic algorithm and simulated annealing (Fatthollahi-Fard et al., Citation2021), and the extension of particle swarm optimization using crowd learning theory (Asghari et al., Citation2022).
3. System description
The mathematical model proposed in this paper addresses a manufacturing-remanufacturing production planning for a hybrid manufacturing-remanufacturing system to fulfill the company’s demands. The company follows a carbon cap and trade policy in its production process. Demand and the quantity of returned products are estimated using the Bass model with a certain return rate. Figure shows the system under consideration. The company’s demands are fulfilled by manufacturing and remanufacturing products. In the manufacturing process, the manufacturer buys raw materials from suppliers and then processes them into finished products and sold to customers through distribution dealers. In remanufacturing, customers return their used products to the company through distribution dealers. Those used products will be disassembled and inspected at the company facility and then processed with raw materials obtained from suppliers to produce remanufacturing products, which are finally sold to customers through distribution dealers. Meanwhile, the used products that do not pass the quality inspection will be disposed of with no salvage values.
4. Model development
4.1. Model assumption
The assumptions of the model are as follows:
The model covers production planning for the hybrid manufacturing-remanufacturing system, with the demand for each period and the quantity of the used products estimated using the Bass’ diffusion model approach with a certain return rate.
Product demand is fulfilled either by manufacturing or remanufacturing production.
Remanufactured products are produced using one component from the returned product.
Product materials are purchased from a single supplier.
The new products, as well as remanufactured products, are sent to a single distribution dealer who is the collector of returned products from customers.
The unit transportation cost from the manufacturer to the distribution dealer has the same value as the transportation cost of the returned products back to the disassembly-inspection facility.
The disassembly-inspection facility is located at the manufacturer’s site.
4.2. Model notations
Table
4.3. Model formulation
First, we have to determine the demand for each period, which is estimated using the Bass’ diffusion model approach as suggested by (Zhong et al., Citation2017):
In EquationEquation (1)(1)
(1) , p denotes the coefficient of innovation (external influence), and q denotes the coefficient of imitation (internal influence). The values of both p and q may be obtained from historical data.
After some delay times (τ) representing the product use time, the product will be returned by customers to the company with a certain return rate (r). Hence, the quantity of the returned product for each period can be calculated as follows (Zhong et al., Citation2017):
The value of r will determine the quantity of . There are two possibilities in
, namely low return and high return. A low return will occur if the value of
and a high return will occur if the value of
. Those two possibilities will be used as the basic scenarios in the model development. The results of the above estimations constitute the product demand at each period and the quantity of the returned product
will be used as the input data for the optimization model.
The model’s objective function is to maximize the net profit of the manufacturing-remanufacturing activities on the manufacturer side. The net profit can be expressed simply by EquationEquation (3)(3)
(3) :
The complete model can be formulated as follow:
s.t.
The model’s objective function is to maximize the net profit as formulated in EquationEquation (4)(4)
(4) . EquationEquation (5)
(5)
(5) ensures that the total number of remanufactured products and the disposed of products equals the number of returned products. The sum of manufactured and remanufactured products should equal the market demand as indicated by EquationEquation (6)
(6)
(6) . The capacity constraints for the manufacturing facility, remanufacturing facility, disassembly and inspection facility, and distribution dealer facility are given by EquationEquations (7)
(7)
(7) , (Equation8
(8)
(8) ), (Equation9
(9)
(9) ), (Equation10
(10)
(10) ), and (Equation11
(11)
(11) ) respectively. EquationEquation (12)
(12)
(12) limits the number of carbon emissions from the company activities and determines how much carbon should be bought or can be sold by the manufacturer. The last constraint in Equation (13) ensures that all product and carbon emission quantities are non-negative.
5. Numerical example and analysis
The numerical example in this research is adopted from Pourjavad & Mayorga (Citation2018), which presented a case of washing machine products with some adjustments from the related literature. The values of p and q used in this research were set at the values of 0.03 and 0.38, as suggested by Sultan et al. (Citation1990) as the average values of both p and q. We assume that the potential market of the product is 150,000 units. The transportation cost is assumed to depend on the traveled distance, unit size, and weight. The parameters of the model are taken from the most relevant research (Bagalagel & ElMaraghy, Citation2020; Wang et al., Citation2017b; Wang et al., Citation2013) and shown in Table . The demand data that the manufacturer should fulfill are shown in Table . The demand data result from the Bass model. We forecast the demand for 20 years, assuming that the product is still marketable for at least the next 20 years and the delay time is set at 10 years, meaning that the customers return their used products after 10 years of use. Hence, the returned products with low- and high-return rates are shown in Table . The value of r represents the low return rate, and the high-return rate is set at 0.01 and 0.2, respectively.
Table 1. Parameters of the model
Table 2. Demand on time horizon
Table 3. Quantity of returned product in each period
The optimization results for low- and high-return cases are shown in Tables , which shows the optimal product mix production planning of manufactured and remanufactured products with the maximum profit. The optimization model resulted profit in low-return and high-return cases of $39,526,560 and $39,256,230, respectively. The last two columns of the table show the carbon sold and bought in both low return and high return case. The manufacturer experiences the deficit in carbon emission only in the first 2 years of operation. The high return gives lower deficit of carbon emission compared to the low return. For the rest of periods, the manufacturer experiences surplus of carbon emission so the emission can be sold to other manufacturer who needs it. On the other side, the profit of low return is 0.6% higher than high return. Hence, with sustainability concern, the manufacturer has to make decision regarding which scenario will be implemented (i.e. the low or high return scenario) and trading off between the profit and carbon emission.
Table 4. Results of the optimization model
A sensitivity analysis was performed to study the model’s behavior toward the uncertainty of its parameters. In this research, we study the effect of the cap threshold, manufacturing cost, and remanufacturing cost on the carbon bought, sold, and company profit. The results of such analysis are shown in Figures . Figures show the result of sensitivity analysis of carbon threshold on high and low returns, respectively. From the figure, we can see that both scenarios result in the same linear pattern in which the increasing or decreasing threshold of the carbon cap affects the carbon bought and sold significantly. The increase of the threshold will decrease the amount of money that the company must spend in buying the carbon while at the same time increasing the company earnings from selling the carbon quota. Conversely, the decrease of the threshold will increase the company’s spending on buying carbon while at the same time decreasing the company’s earnings from selling its carbon quota. The change in the carbon threshold does not significantly affect the resulting profit.
Figures show the sensitivity of manufacturing cost on carbon bought, carbon sold, and company profit both for low- and high-return scenarios. From the figure, the carbon bought and profit have a similar pattern as the impact of manufacturing cost. The higher the manufacturing cost, the lower carbons bought and the company’s profit. While for carbon sold, in low return, the manufacturing cost reduction will decrease the carbon sold, while in high return, the manufacturing reduction cost will make the carbon sold higher. Figures show the effect of remanufacturing cost on carbon bought, carbon sold, and company profit in low-return and high-return scenarios. The figures show the same pattern for the sensitivity effect of remanufacturing cost. The magnitude of the effects looks higher in the high return scenario compared to the low return.
Besides the carbon cap and trade, several developed countries have implemented other carbon policies. They can be incorporated into future research, namely carbon cap and trade, carbon tax, carbon cap, and carbon cap offset. The adoption of those four carbon policies has been discussed in the research of Wang et al. (Citation2013). The research concluded that carbon tax and carbon cap and trade are effective in reducing carbon emissions but give a great challenge to the economic development of the company. In the case of the other two policies, the cap and trade performed better than the cap offset. Hence, it is interesting to incorporate the other carbon policies and compare the results to determine the most effective policy regarding the closed-loop model in this research.
6. Conclusions
In this research, we developed a product mix optimization model for a hybrid manufacturing-remanufacturing system to maximize profit. The product demand is estimated using the Bass model, while the returned product is represented as the proportions of the demand. Optimization results showed that the model could determine the optimal manufacturing-remanufacturing product mix planning by considering the carbon cap and trade policy. A sensitivity analysis was performed on three parameters: carbon cap threshold, manufacturing cost, and remanufacturing cost. The sensitivity analysis results showed that the threshold in both the low- and high-return cases significantly affected carbon bought and sold. Manufacturing cost also significantly affected the carbon bought and sold and the company profit for both low- and high-return cases. While remanufacturing cost resulted from the same pattern in both low- and high-return cases, the magnitude of the effect was higher in high-return cases. As the extensions of this work, we can consider parameters uncertainty, multi-products and components, sources of carbon emission from other activities in manufacturer operations such as inventory and delivery. Other future research can be directed in the development of meta-heuristics methods and the implementation of artificial intelligence to tackle the uncertainty of demand. Lastly, future research can be directed to consider other carbon policies such as carbon tax, carbon cap, and carbon cap offset.
Disclosure statement
No potential conflict of interest was reported by the authors.
Additional information
Notes on contributors
Cucuk Nur Rosyidi
Cucuk Nur Rosyidi is a professor in Industrial Engineering Department of Universitas Sebelas Maret. He received doctoral degree in 2010 from Industrial Engineering Department of Institut Teknologi Bandung. Currently, he serves as the Doctoral Program in Industrial Engineering of Universitas Sebelas Maret and also the head of Production System Laboratory. He is one of the Members of Center for Research in Manufacturing Systems (CRiMS), a Research Group at Faculty of Engineering Universitas Sebelas Maret which focusing on the research of problem solving and mathematical modeling in make or buy decision, inventory management, quality engineering, product design and development, and other related research.
Rachmi Mustika Sari
Rachmi Mustika Sari is the alumni of Undergraduate Program of Industrial Engineering Universitas Sebelas Maret. She served as one of the Production System Laboratory assistant and graduated in 2022. During her position as the assistant of the laboratory, she was responsible for several laboratory practices such as Production Planning and Control and also held several webinars conducted by the laboratory. Currently, she works at the Financial Services Authority (OJK) Republic of Indonesia.
References
- Alumur, S. A., Nickel, S., Saldanha-da-Gama, F., & Verter, V. (2012). Multi-period reverse logistics network design. European Journal of Operational Research, 220(1), 67–14. https://doi.org/10.1016/j.ejor.2011.12.045
- Asghari, M., Afshari, H., Al-E-Hashem, S. M. J., Fathollahi-Fard, A. M., & Dulebenets, M. A. (2022). Pricing and advertising decisions in a direct-sales closed-loop supply chain. Computers & Industrial Engineering, 171(1), 108439. https://doi.org/10.1016/j.cie.2022.108439
- Avenyo, E. K., & Tregena, F. (2022). Greening manufacturing: Technology intensity and carbon dioxide emissions in developing countries. Applied Enrgy, 324, 119726. https://doi.org/10.1016/j.apenergy.2022.119726
- Bagalagel, S., & ElMaraghy, W. (2020). Product mix optimization model for an Industry 4.0-enabled manufacturing-remanufacturing system. Procedia CIRP, 93, 204–209. https://doi.org/10.1016/j.procir.2020.03.029
- da Silva, H. B., Uturbey, W., & Lopes, B. M. (2020). Market diffusion of household PV systems: Insight using the Bass model and solar water heaters market data. Energy for Sustainable Development, 55, 210–220. https://doi.org/10.1016/j.esd.2020.02.004
- Fan, Z.-P., Che, Y.-J., & Chen, Z.-Y. (2017). Product sales forecasting using online reviews and historical sales data: A method combining the Bass model and sentiment analysis. Journal of Business Research, 74, 90–100. https://doi.org/10.1016/j.jbusres.2017.01.010
- Fareeduddin, M., Hassan, A., Syed, M. N., & Selim, S. Z. (2015). The impact of carbon policies on closed-loop supply chain network design. Procedia CIRP, 26, 335–340. https://doi.org/10.1016/j.procir.2014.07.042
- Fatthollahi-Fard, A. M., Dulebenets, M. A., Hajiaghaei-Keshteli, M., Tavakkoli-Moghaddam, R., Safaeian, M., & Mirzahosseinian, H. (2021). Two hybrid meta-heuristic algorithm for a dual channel closed-loop supply chain network design problem in the tire industry under uncertainty. Advanced Engineering Informatics, 50, 101418. https://doi.org/10.1016/j.aei.2021.101418
- Gaur, J., Amini, M., & Rao, A. K. (2017). Closed-loop supply chain configuration for new and reconditioned products: An integrated optimization model. Omega, 66, 212–223. https://doi.org/10.1016/j.omega.2015.11.008
- Ijomah, W. L. (2009). Addressing decision making for remanufacturing operations and design-for-remanufacture. International Journal of Sustainable Engineering, 2(2), 91–102. https://doi.org/10.1080/19397030902953080
- Jeihoonian, M., Zanjani, M. K., & Gendreau, M. (2017). Closed-loop supply chain network design under uncertain quality status: Case of durable products. International Journal of Production Economics, 183, 470–486. https://doi.org/10.1016/j.ijpe.2016.07.023
- Kim, Y. G., & Chung, B. D. (2022). Closed-loop supply chain network design considering reshoring drivers. Omega, 109, 102610. https://doi.org/10.1016/j.omega.2022.102610
- Kurilova-Palisaitiene, J., Sundin, E., & Poksinska, B. (2018). Remanufacturing challenges and possible lean improvements. Journal of Cleaner Production, 172, 3225–3236. https://doi.org/10.1016/j.jclepro.2017.11.023
- Lamba, K., & Singh, S. P. (2019). Dynamic supplier selection and lot-sizing problem considering carbon emission in a big data environment. Technological Forecasting & Social Change, 144, 573–584. https://doi.org/10.1016/j.techfore.2018.03.020
- Lamba, K., Singh, S. P., & Mishra, N. (2019). Integrated decisions for supplier selection and lot-sizing considering different carbon emission regulations in big data environment. Computers & Industrial Engineering, 128, 1052–1062. https://doi.org/10.1016/j.cie.2018.04.028
- Li, L., Yang, Y., & Qin, G. (2019). Optimization of integrated inventory routing problem for cold chain logistics considering carbon footprint and carbon regulations. Sustainability, 11(17), 4628. https://doi.org/10.3390/su11174628
- Mosallanezhad, B., Hajiaghaei-Keshteli, M., & Triki, C. (2021). Shrimp closed-loop supply chain network design. Soft Computing, 25(11), 7399–7422. https://doi.org/10.1007/s00500-021-05698-1
- Pourjavad, E., & Mayorga, R. V. (2018). Optimization of a sustainable closed loop supply chain network design under uncertainty using multi-objective evolutionary algorithms. Advances in Production Engineering & Management, 13(2), 216–228. https://doi.org/10.14743/apem2018.2.286
- Rosyidi, C. N. (2021). A framework of integrated sustainable make or buy decision model, Proceedings of ICIMECE International Conference, IOP Conference Series: materials Science and Engineering, Solo, Indonesia, 1096, 012004.
- Shi, J., Zhang, G., & Sha, J. (2011). Optimal production planning for a multi-product closed loop system with uncertain demand and return. Computers & Operations Research, 38(3), 641–650. https://doi.org/10.1016/j.cor.2010.08.008
- Subulan, K., & Tasan, A. S. (2013). Taguchi method for analyzing the tactical planning model in a closed-loop supply chain considering remanufacturing option. International Journal of Advanced Manufacturing Technology, 66(1–4), 251–269. https://doi.org/10.1007/s00170-012-4322-x
- Su, J., Li, C., Tsai, S. B., Lu, H., Liu, A., & Chen, Q. (2018). A sustainable closed-loop supply chain decision mechanism in the electronics sector. Sustainability, 10(4), 1295. https://doi.org/10.3390/su10041295
- Sultan, F., Farley, J. U., & Lehman, D. R. (1990). A meta-analysis of applications of diffusion model. Journal of Marketing Research, 27(1), 70–77. https://doi.org/10.1177/002224379002700107
- Tashiro, T. (2016). Hierarchical Bass model: A product diffusion model considering a diversity of sensitivity fashion. Physica A Statistical Mechanics & Its Applications, 461, 824–832. https://doi.org/10.1016/j.physa.2016.06.032
- Turki, S., Didukh, S., Sauvey, C., & Rezg, N. (2017). Optimization and analysis of a manufacturing–remanufacturing–transport–warehousing system within a closed-loop supply chain. Sustainability, 9(4), 561. https://doi.org/10.3390/su9040561
- Wang, W., Wang, Y., Mo, D., & Tseng, M. M. (2017a). Component reuse in remanufacturing across multiple product generations. Procedia CIRP, 63, 704–708. https://doi.org/10.1016/j.procir.2017.02.033
- Wang, W., Wang, Y., Mo, D., & Tseng, M. M. (2017b). Managing component reuse in remanufacturing under product diffusion dynamics. International Journal of Production Economics, 183, 551–560. https://doi.org/10.1016/j.ijpe.2016.06.010
- Wang, Y., Zhu, X., Lu, T., & Jeeva, A. (2013). Eco-efficient based logistics network design in hybrid manufacturing/remanufacturing system in low carbon economy. Journal of Industrial Engineering & Management, 6(1), 200–214. https://doi.org/10.3926/jiem.665
- Zeballos, L. J., Méndez, C. A., & Barbosa-Povoa, A. P. (2018). Integrating decisions of product and closed-loop supply chain design under uncertain return flows. Computers & Chemical Engineering, 112, 211–238. https://doi.org/10.1016/j.compchemeng.2018.02.011
- Zhong, J., Guo, J., & Zhu, W. (2017). Optimal manufacturing/remanufacturing production decision for short life-cycle products with uncertain of product update-cycle. Proceedings of International Conference on Industrial Informatics-Computing Technology, Intelligent Technology, Industrial Information Integration (ICIICII), Wuhan, Hubei, China (pp. 190–193).