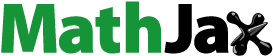
Abstract
This study intended to investigate the injury severity of animal-vehicle crashes (AVCs) and identify the contributing factors from the perspective of animals. To achieve this objective, Bayesian random parameter probit regression model with endogenous variable was proposed with a case study application on two places in Inner Mongolia, China. First, from the perspective of animals, pooled probit regression model with endogenous variable was presented to address the endogeneity issue. Next, panel data model integrated with probit regression model with endogenous variable was presented in order to accommodate the heterogeneity issue due to unobserved factors within Bayesian framework, and to identify the significant influencing factors of injury severity. Results revealed that the endogenous variable (number of casualty) and vehicle type are significant factors for the animal injury severity. Meanwhile, it is demonstrated that there exists an endogeneity issue between injury severity and the number of casualty; while the panel data model integrated with Bayesian probit regression model accommodates the heterogeneity due to unobserved factors. The findings can contribute to the implementation of more reliable countermeasures to prevent the injury severity from drivers and animals in pastoral areas.
1. Introduction
With the improvement of ecological environment in China, more and more wild animals have become active, whereas with the gradually refined transportation system, more and more automobiles have invaded their territories, thus the animal-vehicle crashes (AVCs) have been increasing in recent years, and economic loss, animals and human injury and fatality due to this have becoming worse. Coincidentally, because of the camel-vehicle crash during traveling at night in Inner Mongolia, last month (August 2021) both one famous Chinese actress and the camel were dead, which rang the alarm again. Therefore, it is necessary to concentrate on decreasing the AVCs and reducing the injury severity.
In China, AVCs mostly occur in pastoral or mountainous areas, e.g. Inner Mongolia Autonomous Region, where vehicle collisions with horses, cows and sheep happen frequently, and the property damage and fatality due to AVCs are countless. Consequently, based on those data of AVCs cases in recent years, the estimation and prediction of AVCs may provide valuable references by considering the roadway, weather and environment conditions, as well as providing collision avoidance testing for connected vehicles with actual scenarios.
So far there have been some works and studies focusing on the injury severity analysis of AVCs, but either the analysis is too simple in depth or too complicated in modeling to implement the countermeasures, thus it’s inappropriate to put in practice. Therefore, the objective of this study is to (1) construct a animal-vehicle crash model to identify the influencing factors associated with injury severity of AVCs, and (2) use this model to explore the endogeneity and heterogeneity issues and identify implementable countermeasures in real life.
The main contribution of this work lies in the two aspects: (1) the Bayesian random parameter probit regression model with endogenous variable is proposed to address the influencing factors associated with injury severity of AVCs; (2) There exists an endogeneity issue between injury severity and the number of casualties, while the panel data model integrated with Bayesian probit regression model accommodates the heterogeneity due to unobserved factors.
2. Literature review
A variety of methods and approaches have been employed to detect and analyze the animal-vehicle crashes, mainly including descriptive analysis, econometric modeling and technology application.
From the perspective of human beings, Khattak (Citation2003) focused on human fatalities in animal-related vehicular crash frequencies and rates, and it was found human fatalities occurred when animals were more active and unrestrained persons were more often killed. Due to human being sustained large animal-related injuries, Tremelling et al. (Citation2017) explored the similarities between large animal-related and motor vehicle crash related injuries in USA. Identical study by Niemi et al. (Citation2017) verified that moose-vehicle collisions with personal injuries may address driver awareness and attitudes during summer. Byard (Citation2021) examined vehicle fatalities involving collisions with kangaroos in Australia, and motorcycle riders were verified vulnerable.
At early research time, Conn et al. (Citation2004) analyzed the nonfatal motor-vehicle animal crash-related injuries from United States, which revealed that the risk for motor-vehicle animal crash-related injury can vary among states and local areas. Consequent studies were performed by Wilkins et al. (Citation2019), Ang et al. (Citation2019) in Texas and in Australia, respectively. Rowden et al. (Citation2008) analyzed animal-related road crashes in Australia, which showed night-time travel was significant risk factor, and motorcyclists accounted for a higher proportion in serious crashes. Continuously, Sullivan (Citation2011) examined the AVC trends and characteristics in the United States, and it was found that seasonal variation and posted speed limit played significant role in AVCs. Cherry et al. (Citation2019) described the characteristics of animal-related motor vehicle crashes in United States, and November was found to be the peak month for animal-vehicle crashes.
From the perspective of econometric modeling, Murphy and Xia (Citation2016) investigated the risk of animal-vehicle crashes on a segmented highway in Western Australia. A hierarchical Bayesian model with multivariate Poisson lognormal regression was employed, and the results showed farming, forest roadside vegetation and roadside vegetation had positive effect while speed limits and horizontal curves had negative impact. Al-Bdairi et al. (Citation2020) explored the determinants of driver injury severity in animal-vehicle collisions from Washington State with mixed logit models. It was found that freeway/expressway, daylight crashes, dry road surface and clear weather condition may increase the likelihood of severe injuries.
With latest smart detection technology, Huijser et al. (Citation2006) presented animal detection systems to mitigate animal-vehicle collisions. Warning signs were activated to alert drivers to reduce the travel speed. Khalilikhah and Heaslip (Citation2017) improved the performance of animal crossing warning signs while Druta and Alden (Citation2020) employed a buried cable animal detection system (BCADS) to detect animals. Both provided mitigation countermeasures in critical areas against AVCs.
To sum up, with descriptive analysis, econometric modeling and technology application, animal-vehicle crashes emphasize on the influencing factors and detection of collisions, which provides fundamentals for our analysis. Currently, not many studies have concentrated on animal-related crashes, so the purpose of this study is to take the attempt of investigating animal-vehicle crashes from Inner Mongolia, China, and to explore the endogeneity and heterogeneity issues. The findings may provide meaningful insights for the reduction of animal-vehicle crashes and improvement of roadway safety.
3. Methodology
In this study, we investigate the AVCs from the perspective of injured and fatal animals, so the animal injury severity and number of casualties are both considered. However, there exists endogeneity issue between injury severity and number of casualties, i.e. the more number of casualties, the more severe the animals suffer likely, and vice versa, thus a structural probit equation is required, in which the injury severity is considered as dependent variable, and the number of casualties is regarded as endogenous variable, thus probit regression model with endogenous variable can be expressed as:
where is a latent variable,
is its observable indicator,
is an endogenous and observable variable,
and
are
and
vectors of exogenous variables,
,
,
and
are parameter vectors to be expected,
and
are error terms with mean zero, variance
and
, respectively. For
, Cov(
= Cov(
= 0. Specifically,
represents the injury severity,
denotes the number of casualties,
and
are influencing factors of AVCs. The joint normal distribution of error terms
and
are assumed to be:
Generally, probit regression model can be completed by maximum likelihood estimation, and a two-step method can be employed to estimate the probit regression model with endogenous variable.
In order to address the heterogeneity issue due to unobserved variables, panel data model is integrated with probit regression model, that is, random-effects, mixed effects or random parameter probit model with endogenous variable. Since random parameter model has been demonstrated to be better in performance, similar to the model above, the random parameter probit model with endogenous variable can be described as:
where all the variables represent the same meaning, whereas here includes two parts, fixed and error terms. The panel here includes the IDs and year of animals’ injury severity.
Identically, the two-step method can be employed to estimate the random parameter probit regression model with endogenous variable. However, in this study, Bayesian estimation approach is employed due to the following reasons: (1) the uncertainty is considered in estimating parameters by simulating posterior distribution; (2) compared with the asymptotic maximum likelihood method, it is valid in small samples, which is the main reason here. Bayesian estimation approximates the posterior density of y, where
is the prior distribution of
and
is the posterior density of
given y. Therefore, the posterior density of y given
is the product of the prior distribution of
and the likelihood of the observed data as follows:
In this study, non-information priori is adopted because prior information does not exist, while Markov Chain Monte Carlo (MCMC) is implemented to compute the joint posterior distribution. Different from 95% confidence interval of maximum likelihood estimation, the results present Bayesian credible interval (BCI) as a probability statement about the parameter itself, i.e. a 95% BCI contains the true parameter value with ~ 95% certainty. If the 95% BCI of the posterior mean does not include 0, it implies that this effect is statistically significant at the 95% level.
For model comparison, as provided by many other studies under the Bayesian (Xu et al., Citation2016; Yuan et al., Citation2020; Zeng et al., Citation2017), the Deviance Information Criterion (DIC) is employed to compare the models abovementioned:
where is the deviance evaluated at
, the posterior mean of the parameter of interest,
is the effective number of parameter in the model, and
is the posterior mean of the deviance statistic
.
4. Data description
In this study, the AVCs data were collected in two places Otog Banner and Darhan Muminggan Joint Banner, Inner Mongolia, China and provided by the relevant traffic police teams. The two locations were selected because the AVCs in the two places rank front in the Inner Mongolia region, which is shown in Figure . In Otog Banner area, from 2017 to 2021, there were 92 AVCs, while in Darhan Muminggan Joint Banner area there were 80 AVCs, totally 172 observations. Four main aspects were involved, injury features, driver characteristics, vehicle profiles, roadway and environment attributes. Figure shows four typical real-world AVC scenes from the cases above.
Since the animal perspective is chosen as the injury selection, animal injury severity and number of casualties were collected, but only injured and dead animals were considered as injury source, thus the dependent variables in the proposed model were regarded as binary, in which the response of interest is referred to death, and injured was treated as the contrast, that is, binary probit model. Due to the endogeneity between the injury severity and number of casualties, binary probit model with endogenous variable was proposed. Moreover, by integrating panel data model with Bayesian framework, Bayesian random parameter binary probit model with endogenous variable was presented to make the comparison.
Besides the animal injury severity and the number of casualties, the injury features collected involve animal type, crash time, location and reason. The driver characteristics include the driver age (classified according to Wong et al. (Citation2007)), gender and driving behavior. In vehicle profiles, vehicle color, type and vehicle conditions are collected. Due to the limitation, roadway conditions contain the roadway class and speed limit while environment conditions involve the weather and light.
In order to make the description uniform in two locations, some variables were removed. Because crash time and lighting, roadway class and speed limit reflect the similar parameters, crash time and roadway class were eliminated to avoid the co-linearity. Therefore, in Table , only the necessary parameters description and proportion are given.
Table 1. Summary of Variables Selected
5. Results
Based on the variables selected from the four aspects, the correlation among independent variables needs to be examined before running the model. The Pearson correlation test was conducted in STATA 16 to avoid the co-linearity. Shown from the test result, light is highly related to time, thus, in the final results the two variables may not occur at the same time.
Since the variables belong to categorical outcomes, multinomial probit regression model was supposed to be the best match, however, after the model running, the results can’t reflect the endogenous relation, and hence probit regression model with endogenous variable was turned to. Both the Bayesian binary probit regression model with endogenous variable and Bayesian random parameter binary probit regression model with endogenous variable were conducted to evaluate the injury severity of AVCs, and to make the comparison and examine whether the panel data model can address the heterogeneity issue. Table gives the results of both models.
Table 2. Results for Bayesian binary probit and Bayesian random parameter binary probit models with endogenous variable
As shown from Table , the following observations can be noted. First, the significant variables of both models are identical, and the only difference lies in the mean values. Second, the endogenous variable is significant for both models, indicating that there exists endogeneity between injury severity and number of casualty, and may lead to estimation bias when ignored. Third, the DIC values (156.79 and 155.56) from both models are pretty close, and the difference is less than 5, which indicates the models are not statistically different, but the random parameter probit regression model with endogenous variable performs a little better, thus the following explanation would focus on the Bayesian random parameter probit regression model with endogenous variable.
6. Discussion
As predicted, number of casualty is positively significant for animal injury severity, meaning that the more animal casualties, the higher the probability of injury severity. This is quite natural because if more animal casualties are involved in AVCs, the chances of running into severe injury are higher, thus leading to severe injury with high probability. On the other hand, it demonstrates that there exists an endogeneity issue between injury severity and number of casualty as stated above.
Vehicle type plays a significant role in the injury severity of animals. Compared to regular vehicles, the probability of injury severity is increased with large vehicles (e.g. truck, pickup/van), which makes sense. Usually the injury severity by large vehicles is more severe than regular cars because of the shocking force and size, and some previous studies (Haq et al., Citation2020; Islam & Hernandez, Citation2013) have verified this.
The reason that only two variables are significant lies in two aspects: One is the data limitation. Because AVCs collection is difficult, the coverage of longer time may provide more potential unobserved factors; the other is the impact of extremely adverse weather. Since some of the areas in Inner Mongolia are a vast expanse of Gobi or desert, under bad weather conditions AVCs may occur, but nobody can be found until certain time period, thus the evidence may vanish partially, which causes the factors to be collected prejudicially.
According to the results above, empirically, first, at the high density risk locations in Otog Banner and Darhan Muminggan Joint Banner, corresponding measures should be taken to alert drivers to prevent the animals from confliction, e.g. some flashing sign can be mounted at the feet to remind drivers in advance, or electronic screen, traffic signs, labels and marks can be set up at those segments where the animals occur frequently; meanwhile animals should be paid more attention, for instance, electronic fences can be established to avoid them to approach to the roadways. Next, drivers with large vehicles, especially heavy trucks, should be limited since they are slow in maneuver control, which might lead to severe injury; At last, more education should be conducted for the drivers crossing those regions regularly to guarantee that the drivers are kept alert all the time.
7. Conclusions
In this study, Bayesian random parameter probit regression model with endogenous variable was proposed to identify the significant influencing factors of injury severity for AVCs. The conclusions are as follows:
The results show that the number of casualty and vehicle type are significant factors for the animal injury severity. Meanwhile, it is demonstrated that there exists an endogeneity issue between injury severity and the number of casualty; while the panel data model integrated with Bayesian probit regression model accommodates the heterogeneity due to unobserved factors.
In this study, in order to address the potential relationship between independent variable and independent variable, and some unobserved factors leading to injury severity, the Bayesian random parameter probit regression model with endogenous variable is proposed, in which the endogeneity issue between injury severity and the number of casualty is addressed since they are intrinsically related, while the heterogeneity issue due to unobserved variables is accommodated by the panel data model integrated. This provides the main finding of the work.
Some drawbacks may need to be strengthened in the future study. Since it’s the initial attempt in pastoral areas, more variables relevant to the injury severity need to be collected in the future, such as driver personal status, physical and psychological status, education level, driving habits (passive or aggressive), etc., and with those variables the injury severity can be reflected more completely. Another issue is that the results of the work are founded on the dataset from two locations, and it is worthy of employing different data sources to ascertain the findings and transferability in the future. Further study may consider the space and longer time, so that spatial and temporal issues can be addressed more accurately.
Acknowledgments
The authors gratefully acknowledge the real-world crash cases provided by Baotou Public Security Bureau Traffic Management Detachment and Erdos Public Security Bureau Traffic Management Detachment. And we also acknowledge Wenbin Gao and Jingbo Wang for their participation in crash case collection. This study was supported by State Key Program of National Natural Science Foundation (No.72131008), National Natural Science Foundation of China (No: 52072214), and National Key Research and Development Program (No:2022YFC3800103-03).
Disclosure statement
No potential conflict of interest was reported by the authors.
Additional information
Funding
Notes on contributors

Quan Yuan
Dr. Quan Yuan received the Ph.D. degree on vehicle engineering in 1997. He was a postdoctoral research fellow in Tsinghua University from 2000 to 2003 and he was a visiting scholar in the University of Washington from 2013 to 2014. He is currently the associate director of the Institute of Intelligent Mobility, School of Vehicle and Mobility, Tsinghua University. His research focuses on traffic safety, accident analysis, intelligent vehicle and human factors engineering. He has completed over 30 research projects, published over 100 papers in journals and conferences, and analyzed over 5000 traffic crashes in Beijing, China.
References
- Al-Bdairi, N. S. S., Behnood, A., & Hernandez, S. (2020). Temporal stability of driver injury severities in animal-vehicle collisions: A random parameters with heterogeneity in means (and variances) approach. Analytic Methods in Accident Research, 26, 100120. https://doi.org/10.1016/j.amar.2020.100120
- Ang, J. Y., Gabbe, B., Cameron, P., & Beck, B. (2019). Animal–vehicle collisions in Victoria, Australia: An under-recognised cause of road traffic crashes. Emergency Medicine Australasia, 31(5), 851–9. https://doi.org/10.1111/1742-6723.13361
- Byard, R. W. (2021). Vehicle fatalities involving collisions with kangaroos. Medicine, Science, and the Law, 61(1), 23–26. https://doi.org/10.1177/0025802420962684
- Cherry, C. C., Dietz, S., Sauber-Schatz, E., Russell, S., Proctor, J., & Buttke, D. (2019). Characteristics of animal-related motor vehicle crashes in select National Park Service units-United States, 1990-2013. Traffic Injury Prevention, 20(1), 58–63. https://doi.org/10.1080/15389588.2018.1508835
- Conn, J. M., Annest, J. L., & Dellinger, A. (2004). Nonfatal motor-vehicle animal crash-related injuries—United States, 2001–2002. Journal of Safety Research, 35(5), 571–574. https://doi.org/10.1016/j.jsr.2004.10.002
- Druta, C., & Alden, A. S. (2020). Preventing animal-vehicle crashes using a smart detection technology and warning system. Transportation Research Record, 2674(10), 680–689. https://doi.org/10.1177/0361198120936651
- Haq, M. T., Zlatkovi, M., & Ksaibati, K. (2020). Investigating occupant injury severity of truck-involved crashes based on vehicle types on a mountainous freeway: A hierarchical Bayesian random intercept approach. Accident Analysis & Prevention, 144, 105654. https://doi.org/10.1016/j.aap.2020.105654
- Huijser, M. P., McGowen, P. T., & Camel, W. (2006). Animal vehicle crash mitigation using advanced technology phase I: Review, design, and implementation. Final Report, Federal Highway Administration,
- Islam, M., & Hernandez, S. (2013). Large truck-involved crashes: Exploratory injury severity analysis. Journal of Transportation Engineering, 139(6), 596–604. https://doi.org/10.1061/(ASCE)TE.1943-5436.0000539
- Khalilikhah, M., & Heaslip, K. (2017). Improvement of the performance of animal crossing warning signs. Journal of Safety Research, 62, 1–12. https://doi.org/10.1016/j.jsr.2017.04.003
- Khattak, A. J. (2003). Human fatalities in animal-related highway crashes. Transportation Research Record, 1840(1), 158–166. https://doi.org/10.3141/1840-18
- Murphy, A., & Xia, J. (2016). Risk analysis of animal-vehicle crashes: A hierarchical Bayesian approach to spatial modeling. International Journal of Crashworthiness, 21(6), 614–626. https://doi.org/10.1080/13588265.2016.1209823
- Niemi, M., Rolandsen, C. M., Neumann, W., Kukko, T., Tiilikainen, R., Pusenius, J., Solberg, E. J., & Ericsson, G. (2017). Temporal pattern of moose-vehicle collisions with and without personal injuries. Accident Analysis & Prevention, 98, 167–173. https://doi.org/10.1016/j.aap.2016.09.024
- Rowden, P., Steinhardt, D., & Sheehan, M. (2008). Road crashes involving animals in Australia. Accident Analysis & Prevention, 40(6), 1865–1871. https://doi.org/10.1016/j.aap.2008.08.002
- Sullivan, J. M. (2011). Trends and characteristics of animal-vehicle collisions in the United States. Journal of Safety Research, 42(1), 9–16. https://doi.org/10.1016/j.jsr.2010.11.002
- Tremelling, A. M., Marley, R. A., Marley, M. B., Woofter, C. M., Docherty, C., Salvator, A. E., & Muakkassa, F. F. (2017). Similarities between large animal-related and motor vehicle crash-related injuries. Wilderness & Environmental Medicine, 28(3), 213–218. https://doi.org/10.1016/j.wem.2017.05.004
- Wilkins, D. C., Kockelman, K. M., & Jiang, N. (2019). Animal-vehicle collisions in Texas: How to protect travelers and animal on roadways. Accident Analysis & Prevention, 131, 157–170. https://doi.org/10.1016/j.aap.2019.05.030
- Wong, S. C., Sze, N. N., & Li, Y. C. (2007). Contributory factors to traffic crashes at signalized intersections in Hong Kong. Accident Analysis & Prevention, 39(6), 1107–1113. https://doi.org/10.1016/j.aap.2007.02.009
- Xu, X., Xie, S., Wong, S. C., Xu, P., Huang, H., & Pei, X. (2016). Severity of pedestrian injuries due to traffic crashes at signalized intersections in Hong Kong: A Bayesian spatial logit model. Journal of Advanced Transportation, 50(8), 1025–2028. https://doi.org/10.1002/atr.1442
- Yuan, Q., Xu, X., Xu, M., Zhao, J., & Li, Y. (2020). The role of striking and struck vehicles in side crashes between vehicles: Bayesian bivariate probit analysis in China. Accident Analysis & Prevention, 134, 105324. https://doi.org/10.1016/j.aap.2019.105324
- Zeng, Q., Wen, H., Huang, H., & Abdel-Aty, M. (2017). A Bayesian spatial random parameters Tobit model for analyzing crash rates on roadway segments. Accident Analysis & Prevention, 100, 37–43. https://doi.org/10.1016/j.aap.2016.12.023