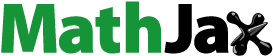
Abstract
In the present study, a group-acceptance sampling plan was presented when the lifetime of an item followed an alpha power transformation-inverted perks distribution. The median is used as a quality index to attain design parameters, such as the acceptance number and minimum group size, which are determined when the test termination and consumer risk are specified. The operating characteristic values are presented both graphically and in the form of tables. Two real-life datasets are used as examples to illustrate the tables.
1. Introduction
Group acceptance sampling plans (GASP) are a statistical method used in quality control in order to determine whether to accept or reject a batch of products or services based on the results of a sample taken from that batch. There is, however, a difference between a GASP and an acceptance sampling plan (ASP) in terms of the way samples are taken and analyzed (Al-Omari et al., Citation2019; Banihashemi et al., Citation2021). ASP involves selecting a random sample from a batch and inspecting it for defects or other quality issues. The sampling plan is typically based on a statistical model that considers batch size, sample size, and acceptable quality level (AQL). If the number of defects or issues in the sample is within the AQL, the batch is accepted; otherwise, it is rejected (Stern, Citation2004). On the other hand, GASP involves dividing the batch into subgroups or groups and then randomly selecting a sample from each group. The samples are then evaluated together, and if the number of defects or issues in the combined sample is within the AQL, the entire batch is accepted. If not, the entire batch is rejected (Moussawi-Haidar et al., Citation2013). GASP is used when it is difficult or costly to inspect each unit individually or when there is a risk of lot-to-lot variation. It can also be used when the product or service is complex and different parts of the batch have different levels of quality (Bouslah et al., Citation2013). In a nutshell, ASP is used to evaluate a batch based on a random sample, whereas GASP divides the batch into subgroups and evaluates the combined sample from each group. These methods are useful for quality control, and the choice of method depends on the specific circumstances and requirements of the product or service being evaluated (Mobley, Citation2002).
Advancements in technology have accelerated the production process, and as far as quality is concerned, 100% inspection cannot be highlighted in a statistical experiment owing to time and monetary limitations (Mohamed et al., Citation2015). Numerous statistical and sampling distribution tests have been conducted to ensure effective product quality (Ryan, Citation2011). In the area of quality control, ASP have gained attention for the life testing of different distributions that will help in the acceptance or rejection of lots taken from samples (Balakrishnan et al., Citation2007). ASP is a method that typically specifies the lot disposition criteria and the necessary sample size. It also determines the number of units used for screening, such as n, as well as the acceptance number c. Hence, if there are no more than c failures out of n items, the lot is accepted; otherwise, it is rejected. Consumer and producer risks are the possibility that a poor lot will be authorized and a good lot will be disallowed (Wang et al., Citation2021). Every sampling plan included information on consumer and producer risks (Wu et al., Citation2017).
Potential motivation for using the GASP is to increase the efficiency and reduce the costs of the quality control process. Instead of checking each item in a batch, which can be time-consuming and costly, GASP allows a representative sample of items to be tested. This provides a good idea of the overall quality of the batch. Another motivation provided by (Al-Nasser et al., Citation2018) for using GASP is to reduce the risk of accepting a batch with a high proportion of defective items. Batches with many flaws can be identified and rejected by testing a small number of items. This can help avoid expensive recalls, returns, or customer complaints (Aslam, et al., Citation2013). In addition, GASP can help increase confidence in the quality of the products being produced, which can be important for maintaining customer satisfaction and loyalty. By implementing a quality control process that includes GASP, organizations can demonstrate their commitment to producing high-quality products, which can help build trust and confidence in customers (Montgomery, Citation2020).
Researchers have been interested in the parameter induction strategy, which uses the composite approach and baseline distribution to introduce new distributions. Evans et al. (Citation2011) provided a detailed description of the generation of new distributions utilising additional factors. Elbatal et al. (Citation2022) presented an inverted perks distribution, which is the most important perspective in this research. To date, many scholars have also introduced many other transformed distributions, such as the alpha power transformation Lindley distribution (Dey et al., Citation2019), the APT Lomax distribution (ZeinEldin et al., Citation2020), the APT Weibull distribution (Ramadan & Magdy, Citation2018), the APT exponential distribution (Ijaz et al., Citation2021), the APT top-leone distribution (Hozaien et al., Citation2022), the APT log logistic distribution (Aldahlan, Citation2020), the APT-exponentiated T-X distribution (Klakattawi & Aljuhani, Citation2021), the APT Pareto distribution (Kinaci et al., Citation2019), and the APT Aradhana distribution (Mohiuddin & Kannan, Citation2021), Marshall–Olkin alpha power inverse exponential distribution (Basheer, Citation2022), generalization of Lindley distribution (Ahsan-Ul-Haq, Choudhary, et al., Citation2022), and power Lomax distribution (Al-Naseer & Haq, Citation2021). Many developments have been made by researchers on APT-inverted distributions; however, no work has been performed using the APTIP distribution. To date, information on performing the GASP technique to maintain the quality of products and improve the selection of products with minimum consumer and producer risk has been provided in this research article, along with some important features of the APTIP distribution are given;
APTIP distribution has a wide range of applications in the analysis of lifespan data.
In the APTIP model, pdf and hrf shapes are more feasible.
The APTIP model outperformed its competitors in terms of fitting.
GASP has already been studied and worked on by many authors and has developed numerous lifetime distributions: Marshall–Olkin extended to the Lomax distribution (Rao, Citation2009a), generalized exponential distribution (Rao, Citation2009b), gamma distribution (Gupta & Gupta, Citation1961), log logistic distribution (Aslam et al., Citation2009), inverse Rayleigh (Ramaswamy & Anburajan, Citation2012), Weibull distribution (Aslam & Jun, Citation2009), generalized inverted exponential (S. Singh et al., Citation2015), inverse power Lomax (Ashraf et al., Citation2022), exponentiated inverted Weibull (Suseela & Rao, Citation2021), skew-generalized inverse Weibull (N. Singh et al., Citation2022), and Odd generalized exponential log-logistic distribution (Sivakumar et al., Citation2019), Exponential Lifetime Distribution (Mughal, Citation2011), Marshall–Olkin Kumaraswamy Exponential (MOKw-E) Distribution by Almarashi et al. (Citation2021), Generalized Inverted Kumaraswamy Distribution by Alsultan and Khogeer (Citation2022), Burr Type X Percentiles (Aslam, Wu, et al., Citation2013), and Generalized Transmuted‐Exponential Distribution (Fayomi & Khan, Citation2022).
The structure of this research paper is as follows: We introduced GASP in section 1. The cumulative distribution function (cdf), probability distribution function (pdf), and quantile function (qf) are presented in section 2. We describe GASP under the APTIP model in section 3. In Section 4 we describe the tables. In Sections 5 (Application) and 6, a discussion is presented. In Section 7, conclusions are presented.
2. Alpha power transformed inverted perks distribution
The random variable Y has the inverted perks distribution with two parameters β and λ, and its cdf is given by
pdf is given by
The cdf of the APT is given below
The corresponding to their pdf is given as
Based on the alpha power transformation, many new distributions are liked APT by Mahdavi and Kundu (Citation2017), and cdf of APTIP is given, where α > 0, β > 0,
The corresponding pdf of APTIP distribution is
Thus, quantile function of APTIP as given,
3. GASP described under the APTIP model
In this study, we used the median as the quality index. As stated by (Shafiq et al., Citation2022), medians perform better for skewed types of distributions. However, the primary goal of this study is to provide a GASP when the lifetime of an item follows APTIP distributions with the specified parameters α, β, λ, and cdf in EquationEquation 5(5)
(5) . GASP provides valuable information about the lot by employing statistical techniques chosen at random to effectively test the overall performance of the product. Numerous steps were used to obtain the design parameters in GASP; the following steps are as follows:
The original group sampling inspection is carried out by randomly selecting n items and evenly distributing them into g groups, with r items and n = r × g in each group.
Determine the duration of the experiment, t0, and the acceptance number, c.
The lot is accepted if the total number of failures across all g groups is less than c within t0; otherwise, it is rejected.
The experiment is terminated when the total number of failures from all groups exceeds c or when the experiment time runs out, whichever comes first.
GASP is defined for the given hypothesized value of r under the two design parameters (g, c) for the APTIP model. In addition, the cdf defined in EquationEquation 5(5)
(5) is dependent on the parameters α, β, and λ. The median lifetime of the truncated life test is defined by EquationEquation 6
(7)
(7) , and the acceptance of the lot probability is expressed as:
Therefore, p represents the likelihood of an item in a group that has failed previously to. To insert EquationEquation 6(7)
(7) into EquationEquation 5
(5)
(5) . Based on EquationEquation 6
(7)
(7) , we set,
and
To substitute η in EquationEquation 8(8)
(8) , λ=
is obtained. Let λ=
and to =a1
, where the product quality can be expressed as a ratio and the median of its median life as the prescribed lifespan is μ/μo. Now substituting λ=
and to=a1μo in EquationEquation 5
(5)
(5) , probability of failure is expressed as
it can also be written as
EquationEquation 12(12)
(12) can be used to calculate p for a specified value of α, β, when a1 is given and r2= μ/μo; however, we have two failure probabilities, p1 and p2, and used to represent the consumer and producer risk, where producer risk refers to the probability of rejecting a good lot and consumer risk refers to accepting the probability of a bad lot. To satisfy the following two Equations simultaneously for a given value of α, β, a1, and r2, we must evaluate the values of g and c
and
However, r1 and r2 correspond to the median ratios of the consumer and producer risks, respectively. The probabilities in EquationEquation 13(13)
(13) and (EquationEquation 14
(14)
(14) ) are given by
Ensuring the constraints while minimizing g and c as min {g, c : (g, r, c)}, to justify the following Equations simultaneously for given values ,
, we evaluate the respective g and c values.
4. Description of tables with examples
For different design parameters under the GASP, Tables are demonstrated, where the values of β (0.2487, 0.455), ℽ (0.25, 0.50, and 0.01), r2 (2, 4, 6, and 8), with a1 (0.5, 1), and r (5,10), are respectively, where r1 is equal to 1, r2 is the ratio (2, 4, 6, 8), and r is the number of items in each group (5 and 10). In our findings with the minimization of consumer risk (ℽ), the number of groups g increases with constant c, and the probability of accepting a lot Pa(p) s also increases. However, in Tables , when we have r = 5, a1 = 0.5, ℽ = 0.25, and r2 = 4, 138 (69 × 2), the units are required to put a life test on it. As shown in Table , when we have r = 10, a1 = 1, ℽ = 0.25, and r2 = 2, 448 (112 × 4) units are required. According to the APTIP model, when the OC value increases, the number of groups decreases, the median life increases to account for GASP, and the median is utilised as a quality metric. As seen in Table , the true median values are, when r = 5 and a1 = 1, which are taken from Table , the true median life was found to rise with decreasing g and c beyond a certain point for the fixed (g, c) and Pa(p). Figure depicts this occurrence by plotting r2 values with respect to OC and g values from Tables , respectively. Figure clearly indicates that the true median lifetime grows, g decreases and remains constant, and OC values rise instantly. As a result, the inspected lot is accepted at these sites under the APTIP model. Accepting the lot for r = 5, as shown in Figure , enables the lowest number of groups to be tested, saving time and money.
Table 1. GASP under APTIP model, α = 0.125, β = 0.2487
Table 2. GASP under APTIP model, α = 1.5, β = 0.455487
Table 3. Optimized values taken from Tables 1 and 2
Now, we have illustrated Tables , with an example, and for the readers, provided additional details (Aslam et al., Citation2010). Let the lifespan of the ball bearing be tested following the APTIP model, with a value of β = 0.2487. However, the mean lifespan of the ball bearing is 2,000 cycles. The consumer and producer face 25% and 5% risks, respectively, when the mean life is 3,000 and 5,000 cycles, respectively. Therefore, if the investigator runs the experiment of 1,000 cycles with 10 units in each group to see that the ball bearing mean life is longer than the prescribed life, for this structure, we have μ0 = 3,000, β = 0.2487, α1 = 0.5, ℽ = 0.25, r = 10, r2 = 4, and producer risk 0.05. However, from Table , we have g = 8, with approval number c = 3, which implies that (8 × 3) = 24, three units will be required among the eight groups. If no more than one unit expires in all these groups before 1,000 cycles, the mean life of the ball bearing will be higher than the stated life. Therefore, if the investigator wants to check the hypothesis that ball bearings have a life span of 5,000 cycles, the true median lifetime is four times that, he can test eight groups of three units each; if higher than one unit fails in 1,000 cycles, as a1 = 0.5, and the mean life length is 1,000 cycles, the integrator will conclude that the life is higher than 5,000 cycles with a 95% level of certainty; consequently, the lot is accepted.
Figure depicts the aforementioned (g,c), and OC (probability values) phenomena by plotting the optimised values from Tables . Figure shows that as the median life increases, the value of g decreases and then remains constant, whereas the value of probability (OC values) increases rapidly. At these points, the under-investigation lot will be accepted under the APTIP model, saving both money and time.
5. Application
5.1. Dataset 1
Data reflects an aerial communication transceiver’s active repair time (hours) (Alyami et al., Citation2022). The information of data is as follows: 0.50, 0.60, 0.60, 0.70, 0.70, 0.70, 0.80, 0.80, 1.00, 1.00, 1.00, 1.00, 1.10, 1.30, 1.50, 1.50, 1.50, 1.50, 2.00, 2.00, 2.20, 2.50, 2.70, 3.00, 3.00, 3.30, 4.00, 4.00, 4.50, 4.70, 5.00, 5.40, 5.40, 7.00, 7.50, 8.80, 9.00, 10.20, 22.00, and 24.50
The maximum likelihood estimates (MLE) with their standard error for two parameters of APTIP are = 0.8132096 (1.376690),
= 1.7222587 (4.026261), and
= 1.9866405 (0.484034). The maximum distance between the fitted APTIP model as well as the data, according to the Kolmogorov –Smirnov test (k-s), is 0.082276 with a p-value = 0.9494. Figure depicts a data (a) P-PP–P Plot, (b) Q-–Q plot, (c) histogram with estimated pdf, (d) estimated cdf with empirical cdf, and (e) increased estimated hazard rate function (f) TTT plot.
5.2. Dataset second
The second dataset was initially given by (Ahsan-Ul-Haq, et al., Citation2022), and it depicts the maximum northern yearly flood flowsflow of Saskatchewan 1,000 cubic feet per second river near Edmonton over a 47-a calendar year. The data information is as follows: 19.885, 20.940, 21.820, 23.700, 24.888, 25.460, 25.760, 26.720, 27.500, 28.100, 28.600, 30.200, 30.380, 31.500, 32.600, 32.680, 34.400, 35.347, 35.700, 38.100, 39.020, 39.200, 40.000, 40.400, 40.400, 42.250, 44.020, 44.730, 44.900, 46.300, 50.330, 51.442, 57.220, 58.700, 58.800, 61.200, 61.740, 65.440, 65.597, 66.000, 74.100, 75.800, 84.100, 106.600, 109.700, 121.970, 121.970, and 185.560.
The maximum likelihood estimates (MLE) with their standard error for three parameters of APTIP are = 8.5678509 (6.094041),
= 0.5080798 (0.435501), and
= 31.9016069 (11.999767). The maximum distance between the fitted APTIP model and the data, as determined by the Kolmogorov –Smirnov test (k-s), is 0.21745, with a p-value of 0.02136.
Figure depicts a data probability plot (pp plot), quantile plot (QQ plot), histogram with estimated pdf, estimated cdf with empirical cdf, and increased estimated hazard rate function, TTT plot all of which demonstrate a better fit of failure times of deep groove ball bearings under APTIP model. The true median lifetimes, n (sample size), c, gg, and OC values for the two datasets are showedshown in Table . Assuming dataset I as shown in Table , with = 0.8132096,
= 1.7222587, and
= 1.9866405, r = 10, a1 = 1, ν = 0.050.05, and when c and g decreasedecrease, the probability increaseincreases, hence lot will be accepted. Similarly, consider dataset II as shown in Table , with
= = 8.5678509,
=
= 0.5080798, and
= = 31.9016069, r = 10, a1 = 1, ν = 0.05, as c and g decrease, the probability increases, and the lot is accepted. As can be seen from the above results for both data sets data setsdatasets I and II, lessfewer groups are tested at optimised cost and time.
Figure 3. Graphical representation of APTIP model for dataset I (a) P–P plot, (b) Q–Q plot, (c) estimated pdf, (d) empirical and theoretical cdf, (e) estimated hrf, and (f) TTT plot.

Table 4. GASP under APTIP model, = 0.8132096,
= 1.7222587,
= 1.9866405
Table 5. GASP under APTIP model,
= 8.5678509,
= 0.5080798, and
= 31.9016069
Table 6. The true median lifetimes, sample sizes, g, c, and OC values of the two datasets
From a practical perspective, we calculated descriptive statistics in Table ; however, due to the right-skewed nature of data, using the median as a quality index is the best option. Therefore, Figure depicts the box-plot of datasets I and II with maximum (Xm), minimum (Xo), mean (), median (
), mode (
), lower quartile (Q1), upper quartile (Q3), variance (S2), and standard deviation (S), respectively.
Table 7. Descriptive statistics
6. Discussion
Group acceptance sampling plans are important quality control tools used in many industries. They offer several advantages over individual sampling plans including cost-effectiveness, efficiency, and ease of use. GASP provide a statistically reliable method for ensuring that the quality of many products meets the required standards, while also reducing the time and resources required for inspection. These plans are widely used in manufacturing, aerospace, and healthcare industries, where quality and reliability are critical. These plans have been developed and refined over many years, and are recognized as an effective method for ensuring product quality and reliability. However, there are some limitations to group acceptance sampling plans that need to be considered. For instance, GASP can sometimes result in more defects being accepted in many or more good products being rejected. In addition, sampling plans cannot guarantee that all products in a lot will be of the same quality. Therefore, GASP is an effective and widely used method to ensure product quality and reliability. They offer several advantages over individual sampling plans, and are based on statistical principles that provide a reliable estimate of the quality of a large number of products. While there are limitations to these plans, they remain valuable tools in the quality control process and are likely to continue to be used in many industries in the future.
7. Conclusion
In this study, we present the GASP, which occurs when the lifetime of an item follows an alpha power transformation-inverted perks distribution. The median is used as a quality index for different constraint parameters, namely acceptance number, group size, predefined consumer risk, and test termination. We also noticed that the optimum value graphs when g and c tended to drop rather than the OC values tended to grow in the table. We visually displayed these optimized values and concluded that it is beneficial to accept the lot at such optimized values with little g and c because it optimizes the price and time involved in examining the items. These findings align with the most recent research by (Algarni, Citation2022), showing that such a tester would save time and money during testing.
Disclosure statement
No potential conflict of interest was reported by the author(s).
References
- Ahsan-Ul-Haq, M., Ahmed, J., Albassam, M., Aslam, M., & Cesarano, C. (2022). Power inverted Nadarajah–Haghighi distribution: Properties, estimation, and applications. Journal of Mathematics, 2022, 1–16. https://doi.org/10.1155/2022/9514739
- Ahsan-Ul-Haq, M., Choudhary, S. M., AL-Marshadi, A. H., & Aslam, M. (2022). A new generalization of Lindley distribution for modeling of wind speed data. Energy Reports, 8, 1–11. https://doi.org/10.1016/j.egyr.2021.11.246
- Aldahlan, M. A. (2020). Alpha power transformed log-logistic distribution with application to breaking stress data. Advances in Mathematical Physics, 2020, 1–9. https://doi.org/10.1155/2020/2193787
- Algarni, A. (2022). Group acceptance sampling plan based on new compounded three-parameter Weibull model. Axioms, 11(9), 438. https://doi.org/10.3390/axioms11090438
- Almarashi, A. M., Khan, K., Chesneau, C., & Jamal, F. (2021). Group acceptance sampling plan using Marshall–Olkin Kumaraswamy Exponential (MOKw-E) distribution. Processes, 9(6), 1066. https://doi.org/10.3390/pr9061066
- Al-Nasser, A. D., Al-Omari, A. I., Bani-Mustafa, A., & Jaber, K. (2018). Developing single-acceptance sampling plans based on a truncated lifetime test for an Ishita distribution. Statistics in Transition New Series, 19(3), 393–406. https://doi.org/10.21307/stattrans-2018-022
- Al-Nasser, A. D., & Haq, M. A. U. (2021). Acceptance sampling plans from a truncated life test based on the power Lomax distribution with application to manufacturing. Statistics in Transition New Series, 22(3), 1–13. https://doi.org/10.21307/stattrans-2021-024
- Al-Omari, A., Al-Nasser, A., & Ciavolino, E. (2019). Acceptance sampling plans based on truncated life tests for Rama distribution. International Journal of Quality & Reliability Management, 36(7), 1181–1191. https://doi.org/10.1108/IJQRM-04-2018-0107
- Alsultan, R., & Khogeer, H. (2022). Group acceptance sampling plan application for vinyl chloride data using generalized inverted Kumaraswamy distribution. Advances and Applications in Statistics, 75(1), 67–78. https://doi.org/10.17654/0972361722027
- Alyami, S. A., Elgarhy, M., Elbatal, I., Almetwally, E. M., Alotaibi, N., & El-Saeed, A. R. (2022). Fréchet binomial distribution: Statistical properties, acceptance sampling plan, statistical inference and applications to lifetime data. Axioms, 11(8), 389. https://doi.org/10.3390/axioms11080389
- Ashraf, R., Saeed, N., Saleem, K., & Aslam, M. (2022). Optimal design of multiple dependent state repetitive group sampling plan for inverse power Lomax distribution. Hacettepe Journal of Mathematics and Statistics, 51(2), 525–542. https://doi.org/10.15672/hujms.875794
- Aslam, M., Ahmad, M., & Mughal, A. R. (2010). Group acceptance sampling plan for lifetime data using generalized Pareto distribution. Pakistan Journal of Commerce and Social Sciences (PJCSS), 4(2), 185–193.
- Aslam, M., Azam, M., Lio, Y. L., & Jun, C. H. (2013). Two-stage group acceptance sampling plan for Burr type X percentiles. Journal of Testing and Evaluation, 41(4), 20120209. https://doi.org/10.1520/JTE20120209
- Aslam, M., & Jun, C. H. (2009). A group acceptance sampling plan for truncated life test having Weibull distribution. Journal of Applied Statistics, 36(9), 1021–1027. https://doi.org/10.1080/02664760802566788
- Aslam, M., Wu, C. W., Jun, C. H., Azam, M., & Itay, N. (2013). Developing a variables repetitive group sampling plan based on process capability index C pk with unknown mean and variance. Journal of Statistical Computation and Simulation, 83(8), 1507–1517. https://doi.org/10.1080/00949655.2012.663374
- Balakrishnan, N., Leiva, V., & López, J. (2007). Acceptance sampling plans from truncated life tests based on the generalized Birnbaum–Saunders distribution. Communications in Statistics - Simulation & Computation, 36(3), 643–656. https://doi.org/10.1080/03610910701207819
- Banihashemi, A., Nezhad, M. S. F., & Amiri, A. (2021). A new approach in the economic design of acceptance sampling plans based on process yield index and Taguchi loss function. Computers & Industrial Engineering, 159, 107155. https://doi.org/10.1016/j.cie.2021.107155
- Basheer, A. M. (2022). Marshall–Olkin alpha power inverse exponential distribution: Properties and applications. Annals of Data Science, 9(2), 301–313. https://doi.org/10.1007/s40745-019-00229-0
- Bouslah, B., Gharbi, A., & Pellerin, R. (2013). Joint optimal lot sizing and production control policy in an unreliable and imperfect manufacturing system. International Journal of Production Economics, 144(1), 143–156. https://doi.org/10.1016/j.ijpe.2013.01.031
- Dey, S., Ghosh, I., & Kumar, D. (2019). Alpha-power transformed Lindley distribution: Properties and associated inference with application to earthquake data. Annals of Data Science, 6(4), 623–650. https://doi.org/10.1007/s40745-018-0163-2
- Elbatal, I., Alotaibi, N., Almetwally, E. M., Alyami, S. A., & Elgarhy, M. (2022). On odd perks-G class of distributions: Properties, regression model, discretization, Bayesian and non-Bayesian estimation, and applications. Symmetry, 14(5), 883. https://doi.org/10.3390/sym14050883
- Evans, M., Hastings, N., Peacock, B., & Forbes, C. (2011). Statistical distributions. John Wiley & Sons. https://doi.org/10.1002/9780470627242
- Fayomi, A., & Khan, K. (2022). A group acceptance sampling plan for ‘another generalized transmuted‐exponential distribution’ based on truncated lifetimes. Quality and Reliability Engineering International Portico. https://doi.org/10.1002/qre.3246
- Gupta, S. S., & Gupta, S. S. (1961). Gamma distribution in acceptance sampling based on life tests. Journal of the American Statistical Association, 56(296), 942–970. https://doi.org/10.1080/01621459.1961.10482137
- Hozaien, H. E., Al Dayian, G. R., Yehia, E. G., & El-Helbawy, A. A. (2022). Topp-leone alpha power family of distributions. المجلة المصرية للدراسات التجارية, 46(3), 1–50.. https://doi.org/10.21608/alat.2022.260618
- Ijaz, M., Mashwani, W. K., Göktaş, A., & Unvan, Y. A. (2021). RETRACTED ARTICLE: A novel alpha power transformed exponential distribution with real-life applications. Journal of Applied Statistics, 48(11), I–XVI. https://doi.org/10.1080/02664763.2020.1870673
- Kinaci, İ., Coşkun, K. U. Ş., Karakaya, K., & Akdoğan, Y. (2019). APT-Pareto distribution and its properties. Cumhuriyet Science Journal, 40(2), 378–387. https://doi.org/10.17776/csj.469493
- Klakattawi, H. S., & Aljuhani, W. H. (2021). A new technique for generating distributions based on a combination of two techniques: Alpha power transformation and exponentiated TX distributions family. Symmetry, 13(3), 412. https://doi.org/10.3390/sym13030412
- Mahdavi, A., & Kundu, D. (2017). A new method for generating distributions with an application to exponential distribution. Communications in Statistics - Theory and Methods, 46(13), 6543–6557. https://doi.org/10.1080/03610926.2015.1130839
- Mobley, R. K. (2002). Predictive maintenance techniques. An Introduction to Predictive Maintenance, 99–113. https://doi.org/10.1016/b978-075067531-4/50006-3
- Mohamed, O. A., Masood, S. H., & Bhowmik, J. L. (2015). Optimization of fused deposition modeling process parameters: A review of current research and future prospects. Advances in Manufacturing, 3(1), 42–53. https://doi.org/10.1007/s40436-014-0097-7
- Mohiuddin, M., & Kannan, R. (2021). Alpha power transformed aradhana distributions, its properties and applications. Indian Journal of Science and Technology, 14(31), 2483–2493. https://doi.org/10.17485/ijst/v14i30.598
- Montgomery, D. C. (2020). Introduction to statistical quality control. John Wiley & Sons.
- Moussawi-Haidar, L., Salameh, M., & Nasr, W. (2013). An instantaneous replenishment model under the effect of a sampling policy for defective items. Applied Mathematical Modelling, 37(3), 719–727. https://doi.org/10.1016/j.apm.2012.02.030
- Mughal, A. R. (2011). A hybrid economic group acceptance sampling plan for exponential lifetime distribution. Economic Quality Control, 26(2). https://doi.org/10.1515/eqc.2011.016
- Ramadan, D. A., & Magdy, W. (2018). On the alpha-power inverse Weibull distribution. International Journal of Computer Applications, 181(11), 6–12. https://doi.org/10.5120/ijca2018917657
- Ramaswamy, A., & Anburajan, P. (2012). Group acceptance sampling plan using weighted binomial on truncated life tests for inverse Rayleigh and loglogistic distributions. IOSR Journal of Mathematics, 2(3), 33–38. https://doi.org/10.9790/5728-0233338
- Rao, G. S. (2009a). A group acceptance sampling plans based on truncated life tests for Marshall-Olkin extended Lomax distribution. Electronic Journal of Applied Statistical Analysis, 3(1), 18–27. https://doi.org/10.22237/jmasm/1304223060
- Rao, G. S. (2009b). A group acceptance sampling plans for lifetimes following a generalized exponential distribution. Economic Quality Control, 24(1). https://doi.org/10.1515/eqc.2009.75
- Ryan, T. P. (2011). Statistical methods for quality improvement. John Wiley & Sons. https://doi.org/10.1002/9781118058114
- Shafiq, S., Jamal, F., Chesneau, C., Aslam, M., Mendy, J. T., & Hashemi-Tabatabaei, M. (2022). On the odd perks exponential model: An application to quality control data. Advances in Operations Research, 2022, 1–10. https://doi.org/10.1155/2022/5502216
- Singh, N., Singh, G., Kanwar, A., & Singh, N. (2022). Repetitive acceptance sampling plan for lifetimes following a skew-generalized inverse Weibull distribution. Pesquisa Operacional, 42. https://doi.org/10.1590/0101-7438.2022.042.00249077
- Singh, S., Tripathi, Y. M., & Jun, C.-H. (2015). Sampling plans based on truncated life test for a generalized inverted exponential distribution. Industrial Engineering & Management Systems, 14(2), 183–195. https://doi.org/10.7232/iems.2015.14.2.183
- Sivakumar, D. C. U., Kanaparthi, R., Rao, G. S., & Kalyani, K. (2019). The odd generalized exponential log-logistic distribution group acceptance sampling plan. Statistics in Transition New Series, 20(1), 103–116. https://doi.org/10.21307/stattrans-2019-006
- Stern, R., ED. (2004). Good statistical practice for natural resources research. CABI. https://doi.org/10.1079/9780851997223.0003
- Suseela, V. B., & Rao, B. S. (2021). Group acceptance sampling plans for life tests based on exponentiated inverted Weibull distribution. European Journal of Molecular & Clinical Medicine, 8(2), 2713–2720.
- Wang, T. C., Wu, C. W., Hsu, B. M., & Shu, M. H. (2021). Process‐capability‐qualified adjustable multiple‐dependent‐state sampling plan for a long‐term supplier–buyer relationship. Quality and Reliability Engineering International, 37(2), 583–597. https://doi.org/10.1002/qre.2750
- Wu, C. W., Lee, A. H., & Chang Chien, C. C. (2017). A variables multiple dependent state sampling plan based on a one-sided capability index. Quality Engineering, 29(4), 719–729. https://doi.org/10.1080/08982112.2017.1288913
- ZeinEldin, R. A., Ahsan Ul Haq, M., Hashmi, S., & Elsehety, M. (2020). Alpha power transformed inverse Lomax distribution with different methods of estimation and applications. Complexity, 2020, 1–15. https://doi.org/10.1155/2020/1860813