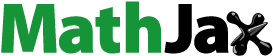
Abstract
This study focuses on implementing a group acceptance sampling plan (GASP) for simultaneous inspection of objects with complex lifespan patterns described by the odd exponential logarithmic (OEL) Fréchet distribution. The inspiration from the proposed model stems from the challenges posed by the objects that exhibited right-skewed, j-shaped, reversed j-shaped and nearly symmetric densities, along with decreasing and increasing hazard rates. A mathematical function is derived to identify key points in the distribution, crucial for designing the inspection process based on factors like testing duration and acceptable risk levels. Performance is assessed across various quality levels, with a simulation study providing insights into parameter changes. The results are clarified via visual representations. The minimum ratios of actual to expected lifespan at specific risk levels, utilizing real-world data to validate the approach are computed. Finally, a comparison is driven in the quest of highlighting the efficacy and versatility of the proposed acceptance sampling plan (ASP) under the inverse log-logistic and generalized exponential (GE) models.
1. Introduction
In the modern age, the advancement in technology has encouraged innovation in industrial sectors and also increased the process of production as well. When talking about bulk of experiments especially related to quality control, the hundred percent inspections cannot be highlighted due to monetary and time limitations. Therefore, for making quality control experiments as efficient as possible and evaluating the quality of the product, several sampling distributions and statistical methods are used. In the field of lifetime testing and quality control the acceptance sampling plan (ASP) has acquired some popularity. In ASP, the decision about accepting or rejecting a lot is made on the basis of life test or inspection which involves selection of a random sample from the lot. The ASP is a technique which describes the standards for allocating lots and the sample size that is used. It is also used to decide whether a lot of products meet predefined quality standards or not (Algarni, Citation2022).
It is widely recognized that nanotechnology has a profound impact on our daily lives. Suitable statistical techniques are indispensable in advancing this field. The application of nanotechnology requires careful statistical analysis, as was highlighted by Lu et al. (Citation2009). Sampling is a key method for evaluating the item quality. Many Researchers used different sampling plans, such as single sampling plan (SSPa) (Abd El-Raheem, Citation2020), where we randomly choose a sample from the lot and decide on the quality of the whole lot based on the sample. The decision is based on predefined criteria for what’s an acceptable quality. The second type is a double sampling plan (DASP) (Gui & Xu, Citation2015) with two inspection stages. In the first stage, a smaller initial sample is inspected, and based on its quality, a decision is made to accept, reject, or take a second larger sample. The final decision regarding the lot is then made based on this larger sample. A sequential sampling plan (SSPb) involves inspecting samples individually and deciding after each sample. The decision for the lot is based on the cumulative results of all the samples inspected sequentially. The inspection process continues until a decision is reached with sufficient confidence in the quality of the lot (Rasay et al., Citation2018). An attribute sampling plan (ASPc) involves assessing the specific characteristics or attributes of a product or process. These attributes are typically categorized as either conforming or non-conforming based on predetermined quality criteria. The decision to accept or reject a lot is based on the proportion of non-conforming items in the sample following established statistical principles (Rasay et al., Citation2020).
The ASP includes total number of units n which are used for screening and the acceptance number c, if the failure in n are more than c the predefined acceptance number, the lot will be rejected otherwise it will be accepted. The acceptance of bad lot and rejection of good lot depends on ASP, the acceptance of poor lot is consumer risk and rejection of good lot is producer risk. The extension of standard sampling plan is group acceptance sampling plan (GASP). The GASP was developed by Jun et al. (Citation2006). Several distributions have been proposed by numerous researchers in terms of GASP, such as GASP based on percentiles for weibull Fréchet model (Ahmed & Yousof, Citation2022), GASP based on compound weibull exponential model by Yiğiter et al. (Citation2023), a hybrid GASP based on Gumbel distribution by Cheepuri et al. (Citation2023), GASP for alpha power transformation inverted perks distribution by Ameeq et al. (Citation2023), GASP for resubmitted lots under odd generalized exponential (GE) log-logistic distribution by Sivakumar et al. (Citation2023), GASP under truncated life test for log logistic and inverse Rayleigh distributions were used by Aslam and Jun (Citation2009), GASP for Odd GE log logistic regression was used by Sivakumar et al. (Citation2019), time truncated GASP under GE distribution was reported by Aslam et al. (Citation2011), Shafiq et al. (Citation2022) proposed odd Perks exponential distribution in their research on GASP, Rao (Citation2009) also used Marshal Olkin extended Lomax distribution for ASP in their research, the GASP under exponentiated Bell exponential distribution proposed by Fayomi et al. (Citation2022), Marshal Olkin kumaraswamy exponential distribution was introduced for GASP by Almarashi et al. (Citation2021).
Researchers have shown a significant interest in a technique called parameter induction for many years (Tahir & Nadarajah, Citation2015). This technique involves creating new probability distributions by strategically combining the existing distributions. Tahir and Nadarajah (Citation2015) provided a comprehensive explanation of the formation of these new distributions by introducing additional parameters. In another study, Tahir et al. (Citation2015) proposed a specific type of distribution called odd GE class. Alizadeh et al. (Citation2015) introduced the Kumaraswamy Marshall–Olkin class of distribution. Handique and Chakraborty (Citation2017) utilized the Marshal Olkin Kumaraswamy distribution in their research. Jamal et al. (Citation2020) described the Marshal Olkin odd Lindley family of distribution. Alizadeh et al. (Citation2020) introduced the odd log-logistic generalized Gompertz distribution. Al-Marzouki et al. (Citation2020) developed Topp-Leone odd Fréchet distributions. Eliwa et al. (Citation2021) created the exponentiated odd Chen-G family of distributions. Chesneau et al. (Citation2022) brought forth the odd exponential Logarithmic (OEL) family of distribution, which serves as a critical theoretical foundation for this study.
The idea of the OEL family of distribution was generated from exponential logarithmic distribution proposed by Tahmasbi and Rezaei (Citation2008). The exponential logarithmic distribution has significant part in theory of reliability, in various fields such as economics, engineering, medical sciences and manufacturing. The primarily rationale for selecting the odd exponential logarithmic Fréchet (OELF) distribution for GASP is that there is no existing literature reporting GASP applied to the OELF distribution. Some of the important points for taking the OELF distribution by using (Chesneau et al., Citation2022) for this study are as follows:
In terms of fitting, the OELF model transcends its competitor model.
There is significant flexibility in the shapes of the probability density function (pdf) and hazard rate function (hrf) in the OELF model.
The OELF distribution excels for the GASP due to the skewed distribution and closed form formulations for the cumulative distribution function (cdf) and quantile function (qf).
The OELF distribution has various applications for analyzing various types of lifetime data.
The OELF distribution enhances the usefulness of the exponential distribution by employing a fundamental ratio approach and logarithmic approach as well as several well-selected scaling factors.
In the light of aforementioned remarks, we recommend the OELF distribution as having the best dynamic characteristics for GASP. As previous researches stated that for skewed data median shows better performance than mean (Gupta, Citation1962), so we take median as quality parameter in this study. At some specific quality levels to satisfy and
GASP was used for the OELF model. For a given
c and minimum number of g needed for test termination time and
are created. This study will be useful for researchers to use sampling plan for observing very small level of quality, when lifetime of objects follows various distributions under the OEL distribution family.
The rest of the article is structured as follows: Section 2 provides an overview of the theoretical foundation of the OELF model, detailing its qf, pdf and cdf. Section 3 establishes the GASP layout within the context of lifetime percentiles under a truncated life test. Section 4 offers an illustrative example of GASP applied to the OELF model. In Section 5, the proposed acceptance sampling technique is applied and summarized using a real-world data example. Lastly, Section 6 presents a succinct concluding summary of the study.
2. Theoretical background of the OELF model
Applications for the Fréchet distribution include accelerated life testing, rainfall and flooding, grocery store lines, sea currents, wind speeds and track race records, among others. According to Chesneau et al. (Citation2022), the pdf of the OELF model exhibits a distinctive shape with rightward skewness and inverted J-like patterns. This distribution, known as the OELF distribution having four parameters and
It is highly effective for analyzing various types of lifetime data. Previous research has confirmed that using the median as the quality parameter is optimal for the GASP (especially for generalized accelerated lifetime testing) approach, particularly when dealing with skewed distributions. Given the skewed nature of the OELF model, we introduce a new model specifically tailored for the GASP. First, we define the pdf, cdf and qf for the OEL-G family, as presented by Chesneau et al. (Citation2022), from which pdf, cdf and qf of the OELF distribution are derived. The cdf of the OEL-G family of distribution can be written as:
(1)
(1)
where
represents the cdf of any baseline model having parameter vector
and
The pdf of the OEL-G family of distribution can be written as:
(2)
(2)
The qf of the OEL-G model by EquationEquation (1)(1)
(1) is
(3)
(3)
where
is qf of the baseline model. Given the cdf of Fréchet distribution as
with pdf
and qf
then using EquationEquation (1)
(1)
(1) , we can write the cdf and pdf of the OELF distribution, respectively as:
(4)
(4)
and
(5)
(5)
The survival function (sf) and hrf of the OELF model are, respectively, expressed as:
(6)
(6)
and
provides different shapes of pdf like j-shape, reverse j-shape, nearly symmetric, positively skewed shape and reverses j-shape for hrf. provides 3D shapes for different values of parameters for the OELF model. The logarithmic increase in profile has been noted for in (a), further such increase is more prominent for smaller values of
The peak is increasing with values of
and
in , the peak occurs for
between 0 and 2.
As the qf of Fréchet distribution is
so the
qf of the OELF model using (Hussain et al., Citation2020) can be obtained from EquationEquation (3)
(3)
(3) as:
(7)
(7)
These functions are applied to further refine the GASP approach described below.
2.1. The related inference with simulation analysis
Let, be the random sample of size n from the OELF distribution with PDF
Then, the likelihood function
for the vector of parameters
for a OELF distribution using EquationEquation (5)
(5)
(5) is given by
(8)
(8)
The first derivatives of with respect to
and
are:
The aforementioned equations are maximized in the R software’s optimum method, which are used to assess the MLEs.
3. Navigating the GASP layout in life-time percentile under truncated life test
In this section, the design parameters for the GASP under the OELF model are delineated. Based on the insights from prior research, wherein the median exhibited superior performance compared to mean in the context of skewed distributions (Aslam & Jun, Citation2009), the quality parameter selected for this study is the median. The overarching objective is to formulate a GASP framework under the assumption that the lifetime of the object adhere to the OELF model, characterized by parameters and
with a cdf defined in EquationEquation (4)
(4)
(4) . GASP, as a statistical quality control tool, empowers a firm to scrutinize randomly selected samples, evaluating the overall performance of the lot through adept statistical techniques. The process of driving the design parameters and implementing GASP for the OELF, drawing from methodologies proposed by Almarashi et al. (Citation2021) and (Aslam & Jun, Citation2009), unfolds through the following steps:
Step 1
The sample size of the lot will be
when we select number of groups g and assign predefined
items to each group.
Step 2
Select acceptance number c and the experimental time
Step 3
Record number of failures d for each group g by performing simultaneously.
Step 4
If number of failures d are more than c, then terminate the experiment and reject the lot otherwise do not reject the lot.
This condition is specifying that, for each group g within the total batch t, the number of defective items d in each group should be less than or equal to a certain critical value c.
Thus, for a given r, design parameters define the preferred GASP. One can see from EquationEquation (4)
(4)
(4) that the cdf of the OELF model depends on
and
The median life of the OELF model can be obtained using EquationEquation (7)
(7)
(7) . It would be suitable to find for (termination time) because
where
is often referred to as the acceptance number or the tolerable number of defective items, the maximum allowable number of defective items in the sample for the entire lot to be accepted is represented by
and
refers to specific constant. If we take
the specified life is twice the experimental time. Using Shafiq et al. (Citation2022), we can obtained the probability of accepting the lot
It states that the
in a group ASP provides a quantitative measure of the likelihood of accepting a production lot based on the observed proportion of defective items. This information is crucial for companies to maintain quality standards, make informed decisions, and optimize their quality control processes and all the equations which are given below as:
(9)
(9)
where the failure of objects in the group before time
is represented by probability p, which can be obtained by substituting EquationEquation (7)
(7)
(7) in EquationEquation (4)
(4)
(4) . On the basis of EquationEquation (7)
(7)
(7) , we set,
Let
Now, we substitute and
in EquationEquation (4)
(4)
(4) , the probability of failure can be given as:
it can also be expressed as:
(10)
(10)
We can use EquationEquation (10)(10)
(10) to find p for specified value of
when
and
(where
is variability in determining the group size) are given. To describe the quality level of the product ratio
(the product’s mean lifespan to certain lifespan) can be used. The failure probabilities given below
and
can be used to describe
and
where
is the probability of accepting a bad lot and
is the probability of rejecting a good lot. For values given for
and
we would evaluate values for c and g which follow the following constraints
(11)
(11)
and
(12)
(12)
where the ratio at the
is
and the ratio at the
is
The failure probabilities to be used in EquationEquations (11)
(11)
(11) and Equation(12)
(12)
(12) are
(13)
(13)
and
(14)
(14)
To determine if the median lifespan has grown to the appropriate level, a statistical lifespan test must be performed. We can define the associated hypothesis as follows:
(15)
(15)
with
The best design would be GASP with smallest sample size which complies with the disparity restrictions stated in EquationEquations (11)
(11)
(11) and Equation(12)
(12)
(12) and formulation is given as:
(16)
(16)
where,
denoted the set of positive integers. The acceptable region in this case is calculated by
(17)
(17)
The g, c are the design variables for the prefixed values of r. Throughout the simulation, multiple mixtures of the plan parameters that satisfy the requirements of EquationEquation (17)(17)
(17) for the pre-defined values of r. The optimum values of g and c were chosen, with a 5% maximum tolerable producer risk.
4. Explanatory example of GASP for the OELF model
The specified parameters for GASP include varying values for and
and
and
and
and
and
and
as detailed in below. The results indicate that minimizing consumer risk
leads to an increase in the number of groups, while increasing
results in a decrease in the number of groups. The probability of accepting a lot
increases after reaching a certain point, holding g and c constant. For instance, when
and
(as shown in ), 55 units
are required for life testing. However, with all other values remaining constant and r increasing to 10, the units needed for life testing decrease to
In this context, choosing
items proves more advantageous. The utilization of the median as a quality parameter for GASP results in a reduction in the number of groups and an increase in the operating characteristic
values for the OELF model, attributable to the rise in the true median life. displays the median lifetime with values of c, g and OC derived from . The data indicates that as c and g values decrease, the true median life increases. Beyond a certain point, with constant c and g, OC values also rise.
Table 1. GASP displaying minimal g and c for
and
Table 2. GASP displaying minimal g and c for
and
Table 3. GASP displaying minimal g and c for
and
Table 4. Optimized values of g, c and when
and
Examining the results from using an example from Singh and Tripathi (Citation2017). Let us assume that lifespan of the ball bearing is 5000 cycles tested through the OELF model with The producer’s risk is
and the consumer’s risk is
for an average life of 10,000 cycles. In an experiment with 5000 cycles and 10 groups, where
the producer’s risk =0.05,
and
results from show
and 3. Thus 60 units
are drawn, allocating five units to each of the 12 groups. The median life statistically exceeds the prescribed life if no more than three units fail before 5000 cycles in these groups with 5 units each, a failure of more than three objects would lead the analyst to interpret that the lifetime exceeds 10,000 cycles with a
confidence interval, and the considered lot should be accepted.
shows the above-mentioned phenomena for g, c and OC (probability values) by plotting the optimized values obtained from and . From figure, it can be seen that with increase in median life the value of g began to decreases and then, after some points, it remains constant, but the value of probability (OC values) increase rapidly. At these points, the under investigation lot would be accepted under the OELF model and it will save cost and time.
5. Applications of GASP for the OELF model using real data sets
In this section, we take real dataset examples of GASP under the OELF model, which consists of failure times of 30 repairable equipments. The data were obtained from the study represented by Elbatal et al. (Citation2022). The failure times are given below in .
Table 5. Failure time of repairable equipments.
The maximum likelihood estimates of the OELF distribution for the above-mentioned data set for four parameters with their standard errors in parentheses like
and
are given in . According to Kolmogrove–Smirnove test the p value for the fitted the OELF model is 0.9998 and the highest distance between data and fitted OEFL distribution is 0.062991.
Table 6. GASP displaying minimal g and c for
and
represents the better fit of the data with histogram of the data with estimated pdf, estimated cdf with empirical cdf, probability–probability (P–P) plot and quantile–quantile (Q–Q) plot. It can be also be seen that total time on test (TTT) and also increased estimated hrf show the better fit of failure times of repairable equipment under the OELF model.
Figure 4. Graphical representation of the OELF model for data-set I (a) estimated pdf; (b) estimated cdf; (c) P–P plot; (d) Q–Q plot; (e) estimated hrf; (f) TTT plot.

Another real data set example for GASP under the OELF model was taken from article of Rasay et al. (Citation2020). The data set consists of failure times of 23 deep grove ball bearings (recorded in million of revolutions). The failure times are given in .
Table 7. Failure time of ball bearing.
The maximum likelihood estimates of the OELF distribution for second data set for four parameters with their standard errors in parentheses like
and
are given in . According to Kolmogrove–Smirnove test the p value for the fitted the OELF model is 0.8346 and the highest distance between data and fitted OELF distribution is 0.1296.
Table 8. GASP displaying minimal g and c for
and
represents histogram for the data having estimated pdf, estimated cdf with empirical cdf, P–P plot, Q–Q plot, TTT plot and also increased estimated hrf which show the better fit of failure times of deep grove ball bearings under the OELF model.
Figure 5. Graphical representation of the OELF model for data-set II (a) epdf (b) ecdf (c) P–P plot (d) Q–Q plot; (e) estimated hrf; (f) TTT plot.

The true median lifetimes, n (sample size), c, g and OC values taken from and for the two data sets are shown in . Assuming data set I with
given when c and g decrease the probability increase, hence lot will be accepted. Similarly, considering taking data set II with
given when c and g decrease the probability increase, hence lot will be accepted. It can be seen from the above results for both the data sets, at optimized cost and time, less number of groups are tested.
Table 9. True median lifetimes, sample sizes, g, c and values of the two data sets.
5.1. Comparative study of OSP and GASP
ASPs are used in the batch judgment process to assess whether the departing or arriving groups should be accepted or rejected based on a specified quality. The two variables that experienced experts should consider most carefully and maximize the length of the study and sample size. Although the OSP can assist with this optimization, only one object will be reviewed at a time in this plan. In contrast, when several items are examined by grouping the items together, a GASP may also attain the best cost and inconvenience.
The proposed GASP is an extension of the OSP for and
In this part, considering
we differentiate the recommended GASP with the OSP. For this objective, we offered a comparison of the proposed GASP under
and
with
and
Comparison of n and c in highlighted that the GASP is the ideal technique when contrasted to OSP because GASP holds minimal n and c and thus helpful in optimizing cost and time.
Table 10. n and c for GASP and OSP when
and
5.2. Comparison with plans used under different models
In this section, we compared the plan presented in this article with previous studies of Tripathi et al. (Citation2021) and Aslam et al. (Citation2011). The GASP for the inverse log logistic (INLL) model and GE model having and
are
and
respectively. So, to reach a same decision about the submitted product, the number of samples required for our plan is 30,
and while the number of samples required for comparative plans are 1230 for OPE distribution and 70 for GE distribution, respectively. Further comparison with number of samples required for each plan is given below in . represents comparison of the behavior of OC values for the OELF, INLL and GE models. In , the OC curve for the OELF model consistently exhibits higher values as compared to the INLL and GE models. Hence, the OELF model is better at correctly accepting lots or processes according to the specified criteria ().
Table 11. Comparison of sample sizes for GASP between different models having
6. Conclusion
This study highlights a GASP with the assumption that the lifespan of items follows an OELF distribution. The proposed approach involves equating the risks of consumers and producers, ensuring a balanced perspective and the determination of acceptance number c and the number of groups g. The operating characteristics values obtained through the proposed approach provide valuable insights into the efficiency and reliability of the proposed GASP. Graphical representations offered a clear visualization of how the acceptance number c and the number of groups g varied with changes in the true median life, demonstrating the adaptability and effectiveness of the proposed approach. Furthermore, a comprehensive set of tables presented the results obtained under various parametric conditions. Notably, as the true median life increases, the acceptance number c and the number of groups g tends to rise. This trend underscored the plan’s capability to accommodate longer-lasting items while maintaining stringent quality control. The proposed study goes beyond a mere presentation of numerical results. The risks associated with both consumers and producers, ensuring a balanced and fair evaluation of the sampling plan. This approach adds a layer of practicality to the findings of the study, making them applicable in real-world scenarios. Moreover, the comparative study conducted in this research highlights the superiority of the proposed GASP over traditional ordinary sampling plans. The GASP under OELF distribution performed best as compared to GASP under INLL model and GE model. The proposed study clearly concluded that to obtain optimum values of time and cost, an industrialist should use a GASP under the OELF model for the failure time data. The demonstrated advantages, coupled with the theoretical underpinnings and robust statistical analysis, reinforce the validity and practicality of the proposed approach. Future research should expand the study’s scope to encompass a wider range of industries and diverse operational conditions to ensure a more comprehensive evaluation of the plan’s effectiveness in various context. Collaborating with industries can lead in integrating the GASP into existing production processes and addressing industry-specific nuances.
Supplementary File of R codes.pdf
Download PDF (72.4 KB)Revised OELF copy changes in red.bbl
Download (9.3 KB)Disclosure statement
No potential conflict of interest was reported by the author(s).
References
- Abd El-Raheem, A. (2020). Optimal design of multiple accelerated life testing for generalized half-normal distribution under type-i censoring. Journal of Computational and Applied Mathematics, 368, 112539. https://doi.org/10.1016/j.cam.2019.112539
- Ahmed, B., & Yousof, H. (2022). A new group acceptance sampling plans based on percentiles for the Weibull fréchet model. Statistics, Optimization & Information Computing, 11(2), 409–421. https://doi.org/10.19139/soic-2310-5070-1320
- Al-Marzouki, S., Jamal, F., Chesneau, C., & Elgarhy, M. (2020). Topp-leone odd fréchet generated family of distributions with applications to Covid-19 data sets. Computer Modeling in Engineering & Sciences, 125(1), 437–458. https://doi.org/10.32604/cmes.2020.011521
- Algarni, A. (2022). Group acceptance sampling plan based on new compounded three-parameter Weibull model. Axioms, 11(9), 438. https://doi.org/10.3390/axioms11090438
- Alizadeh, M., Benkhelifa, L., Rasekhi, M., & Hosseini, B. (2020). The odd log-logistic generalized Gompertz distribution: Properties, applications and different methods of estimation. Communications in Mathematics and Statistics, 8(3), 295–317. https://doi.org/10.1007/s40304-018-00175-y
- Alizadeh, M., Tahir, M., Cordeiro, G. M., Mansoor, M., Zubair, M., & Hamedani, G. (2015). The Kumaraswamy marshal-olkin family of distributions. Journal of the Egyptian Mathematical Society, 23(3), 546–557. https://doi.org/10.1016/j.joems.2014.12.002
- Almarashi, A., Khan, K., Chesneau, C., & Jamal, F. (2021). Group acceptance sampling plan using marshall–olkin Kumaraswamy exponential (mokw-e) distribution. Processes, 9(6), 1066. https://doi.org/10.3390/pr9061066
- Ameeq, M., Tahir, M., Hassan, M. M., Jamal, F., Shafiq, S., & Mendy, J. T. (2023). A group acceptance sampling plan truncated life test for alpha power transformation inverted perks distribution based on quality control reliability. Cogent Engineering, 10(1), 2224137. https://doi.org/10.1080/23311916.2023.2224137
- Aslam, M., & Jun, C. H. (2009). A group acceptance sampling plans for truncated life tests based on the inverse rayleigh and log-logistic distributions. Pakistan Journal of Statistics, 25(2), 107–119. https://www.researchgate.net/publication/235971497_A_group_acceptance_sampling_plan_for_truncated_life_tests_based_the_inverse_Rayleigh_distribution_and_log-logistics_distribution
- Aslam, M., Kundu, D., Ahmad, M., Chi-Jun. (2011). Time truncated group acceptance sampling plans for generalized exponential distribution. Journal of Testing and Evaluation, 39(4), 671–677.
- Cheepuri, C., Rao, B. S., & Rosaiah, P. K. (2023). A hybrid group acceptance sampling plans for life tests based on gumbel distribution. https://doi.org/10.21203/rs.3.rs-2741373/v1
- Chesneau, C., Tomy, L., Jose, M., & Jayamol, K. V. (2022). Odd exponential-logarithmic family of distributions: Features and modeling. Mathematical and Computational Applications, 27(4), 68. https://doi.org/10.3390/mca27040068
- Elbatal, I., Alotaibi, N., Almetwally, E. M., Alyami, S. A., & Elgarhy, M. (2022). On odd perks-g class of distributions: Properties, regression model, discretization, bayesian and non-bayesian estimation, and applications. Symmetry, 14(5), 883. https://doi.org/10.3390/sym14050883
- Eliwa, M., El-Morshedy, M., & Ali, S. (2021). Exponentiated odd Chen-g family of distributions: Statistical properties, bayesian and non-bayesian estimation with applications. Journal of Applied Statistics, 48(11), 1948–1974. https://doi.org/10.1080/02664763.2020.1783520
- Fayomi, A., Tahir, M., Algarni, A., Imran, M., & Jamal, F. (2022). A new useful exponential model with applications to quality control and actuarial data. Computational Intelligence and Neuroscience, 2022, 2489927–2489998. https://doi.org/10.1155/2022/2489998
- Gui, W., & Xu, M. (2015). Double acceptance sampling plan based on truncated life tests for half exponential power distribution. Statistical Methodology, 27, 123–131. https://doi.org/10.1016/j.stamet.2015.07.002
- Gupta, S. S. (1962). Life test sampling plans for normal and lognormal distributions. Technometrics, 4(2), 151–175. https://doi.org/10.1080/00401706.1962.10490002
- Handique, L., Chakraborty, S., & Hamedani, G. G. (2017). The Marshall-Olkin-kumaraswamy-G family of distributions. Journal of Statistical Theory and Applications, 16(4), 427–447.
- Hussain, M. A., Tahir, M. H., & Cordeiro, G. M. (2020). A new Kumaraswamy generalized family of distributions: Properties and applications. Mathematica Slovaca, 70(6), 1491–1510. https://doi.org/10.1515/ms-2017-0429
- Jamal, F., Reyad, H., Chesneau, C., Nasir, M. A., & Othman, S. (2020). The Marshall-Olkin odd Lindley-g family of distributions: Theory and applications. Punjab University Journal of Mathematics, 51(7), 111–125.
- Jun, C. H., Balamurali, S., & Lee, S. H. (2006). Variables sampling plans for Weibull distributed lifetimes under sudden death testing. IEEE Transactions on Reliability, 55(1), 53–58. https://doi.org/10.1109/TR.2005.863802
- Lu, J. C., Jeng, S. L., & Wang, K. (2009). A review of statistical methods for quality improvement and control in nanotechnology. Journal of Quality Technology, 41(2), 148–164. https://doi.org/10.1080/00224065.2009.11917770
- Rao, G. S. (2009). A group acceptance sampling plans based on truncated life tests for Marshall-Olkin extended Lomax distribution. Electronic Journal of Applied Statistical Analysis, 3(1), 18–27.
- Rasay, H., Naderkhani, F., & Golmohammadi, A. M. (2020). Designing variable sampling plans based on lifetime performance index under failure censoring reliability tests. Quality Engineering, 32(3), 354–370. https://doi.org/10.1080/08982112.2020.1754426
- Rasay, H., Pourgharibshahi, M., & Fallahnezhad, M. S. (2018). Sequential sampling plan in the truncated life test for Weibull distribution. Journal of Testing and Evaluation, 46(2), 20170022. https://doi.org/10.1520/JTE20170022
- Shafiq, S., Jamal, F., Chesneau, C., Aslam, M., & Mendy, J. T. (2022). On the odd perks exponential model: An application to quality control data. Advances in Operations Research, 2022, 1–10. https://doi.org/10.1155/2022/5502216
- Singh, S., & Tripathi, Y. M. (2017). Acceptance sampling plans for inverse Weibull distribution based on truncated life test. Life Cycle Reliability and Safety Engineering, 6(3), 169–178. https://doi.org/10.1007/s41872-017-0022-8
- Sivakumar, D., Kalyani, K., Rao, G. S., & Rosaiah, K. (2023). Group acceptance sampling plans for resubmitted lots under odd generalized exponential log-logistic distribution. European Journal of Statistics, 3, 9–9. https://doi.org/10.28924/ada/stat.3.9
- Sivakumar, D. C. U., Kanaparthi, R., Rao, G. S., & Kalyani, K. (2019). The odd generalized exponential log-logistic distribution group acceptance sampling plan. Statistics in Transition New Series, 20(1), 103–116. https://doi.org/10.21307/stattrans-2019-006
- Tahir, M. H., Cordeiro, G. M., Alizadeh, M., Mansoor, M., Zubair, M., & Hamedani, G. G. (2015). The odd generalized exponential family of distributions with applications. Journal of Statistical Distributions and Applications, 2(1), 28. https://doi.org/10.1186/s40488-014-0024-2
- Tahir, M. H., & Nadarajah, S. (2015). Parameter induction in continuous univariate distributions: Well-established g families. Anais da Academia Brasileira de Ciencias, 87(2), 539–568. https://doi.org/10.1590/0001-3765201520140299
- Tahmasbi, R., & Rezaei, S. (2008). A two-parameter lifetime distribution with decreasing failure rate. Computational Statistics & Data Analysis, 52(8), 3889–3901. https://doi.org/10.1016/j.csda.2007.12.002
- Tripathi, H., Dey, S., & Saha, M. (2021). Double and group acceptance sampling plan for truncated life test based on inverse log-logistic distribution. Journal of Applied Statistics, 48(7), 1227–1242. https://doi.org/10.1080/02664763.2020.1759031
- Yiğiter, A., Hamurkaroğlu, C., & Danacıoğlu, N. (2023). Group acceptance sampling plans based on time truncated life tests for compound weibull-exponential distribution. International Journal of Quality & Reliability Management, 40(1), 304–315. https://doi.org/10.1108/IJQRM-07-2021-0201