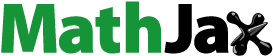
Abstract
Gait quality is of significant interest in studies investigating interventions for individuals with gait pathology. A primary challenge in gait analysis is selecting an appropriate metric which adequately reflects aberrant deviations and provides an assessment of gait quality in individuals with gait pathology. Metrics vary in definition of gait quality, and there is lack of consensus on an objective outcome measure for assessing gait; while three-dimensional gait analysis is the gold standard, metrics operationalizable in the form of wearable sensors would provide important information to clinicians and researchers without requiring a laboratory. In this study, we investigated and compared the ability of four metrics to detect aberrant gait through systematically applied joint constraint: Prosthetic Observational Gait Score (POGS), Impulse Asymmetry, Lateral Sway, and Gait Deviation Index (GDI). We analyze these metrics to understand their sensitivity and ability to detect systematic perturbed gait with an eye toward future operationalization in the form of a wearable sensor suite. We systematically applied four unilateral lower limb joint constraint conditions to nine able-bodied participants walking at three speeds creating four distinct gait patterns with variations from the baseline. Notably, POGS and GDI together distinguished five of six joint constraint comparisons, with each metric able to distinguish four joint constraint comparisons. Lateral Sway distinguished three joint constraint conditions and two speed conditions, while Impulse Asymmetry distinguished three constraint conditions. No single metric distinguished every condition. A single metric may be adequate for assessing specific gait features; however, multiple metrics are recommended for comprehensive assessment of pathological gait.
Reviewing Editor:
Background
Gait quality is of high interest in studies investigating wearable assistive technologies for individuals with gait impairment. However, a primary challenge in gait analysis is a selection of an appropriate metric which adequately reflects deviations from normal and provides a comprehensive assessment of gait quality (Roberts et al., Citation2017). Additionally, the gait metric of choice may vary depending on the expertise and background of the investigator. Various approaches to assessing gait quality have been used on individuals with gait impairment, including observational scores (Hillman et al., Citation2010; Read et al., Citation2017), spatiotemporal parameters (Balasubramanian et al., Citation2009; Highsmith et al., Citation2010; Lewek et al., Citation2014), kinematic and kinetic analyses (Kark et al., Citation2016; Zmitrewicz et al., Citation2006), overall gait scores (Eshraghi et al., Citation2014; Kark et al., Citation2012), clinical outcome measures (Kark et al., Citation2016; Lythgo et al., Citation2010), and patient-reported measures (Kark et al., Citation2016; Kark & Simmons, Citation2011). Advanced artificial intelligence methods have also recently been proposed for assessing gait quality and movement (Kaczmarczyk et al., Citation2009; Sayeed et al., Citation2022; Wang et al., Citation2023).
In typical clinical settings, gait quality is assessed through observation and patient reported feedback due to its simplistic and cost-effective nature (Brunnekreef et al., Citation2005; Rathinam et al., Citation2014; Toro et al., Citation2003). Although observational gait analysis is quick, it can be highly subjective (Rathinam et al., Citation2014; Toro et al., Citation2003), dependent on evaluator experience (Brunnekreef et al., Citation2005), and difficult to quantitatively report. Alternatively, advanced motion analysis systems are considered the gold standard of gait analysis and can provide objective and quantitative kinematics and kinetics data (Hutabarat et al., Citation2021). However, gait labs are not ubiquitously available especially to clinicians or researchers who want to understand the value of their interventions in real world environments (Kaczmarczyk et al., Citation2009; Toro et al., Citation2003). Further, a gait lab is not representative of a patient’s natural environment in regards to terrain, cognitive attention or changes in clinical presentation and physical condition (Del Din, Godfrey, et al., Citation2016; Del Din, Hickey, et al., Citation2016; Kiani et al., Citation1997; Rast & Labruyère, Citation2020). Indeed, there is great value in understanding pathological gait performance outside of the lab. However, for a gait metric to be useful, sensitivity to changes in a patient’s gait with quantifiable distinctions between improvement or decline is needed. As Toro, et al. (Citation2003) states, many gait analysis tools have ‘grown out of clinical need rather than scientific scrutiny of pathological gait’ (Toro et al., Citation2003). Therefore, it is critical that gait quality metrics are assessed via the gold standard of gait monitoring, the three-dimensional gait analysis laboratory, such that their sensitivity and specificity may be fully understood (Toro et al., Citation2003). Additionally, a metric which could be operationalized into a wearable sensor would enhance gait monitoring in clinical and community settings (Hutabarat et al., Citation2021; Jourdan et al., Citation2021) and be meaningful for both clinicians and researchers without access to advanced gait laboratories or a desire to test interventions in real-world scenarios (Del Din, Hickey, et al., Citation2016). With this motivation, we systematically tested four common gait quality metrics representing a range of approaches on their ability to detect obvious and systematic changes in gait quality. To our knowledge, a formal, systematic comparison of these common gait quality metrics has never before been performed.
We investigated four gait quality metrics that each represent a different analytical approach which we review herein: an observational scoring method, the Prosthetic Observational Gait Score (POGS) (Hillman et al., Citation2010); a quantitative method for assessing whole body kinetics, Impulse Asymmetry (IA) (Zmitrewicz et al., Citation2006); an easily automated, kinematic method of assessing balance, truncal Lateral Sway (LS); and a computational method for assessing kinematics, the Gait Deviation Index (GDI) (Schwartz & Rozumalski, Citation2008).
POGS
POGS is a visual metric with good intra-observer repeatability (Hillman et al., Citation2010) useful to clinicians without specialized equipment. While it has been used to assess gait of prosthesis users (Duffy et al., Citation2019) it is not limited to the evaluation of gait in those with lower limb amputation. Thus, POGS as a gait assessment tool has implications for individuals with general gait impairment as well.
Impulse Asymmetry
While observational gait analysis is useful in clinical settings (Brunnekreef et al., Citation2005), instrumented gait analysis is widely recognized as the preferred method for objective gait assessment in pathological populations (Rathinam et al., Citation2014). Force platforms are used in gait analysis laboratories to collect kinetics information which bolsters assessment capabilities. For example, ground force impulse is significantly different in children with cerebral palsy and hemiparetic stroke survivors than in control groups (Balasubramanian et al., Citation2007; White et al., Citation2005). Impulse differences are also seen between individuals with transfemoral and transtibial amputations, and inter-limb IA has been noted to improve with use of more advanced prosthetic technology (Cutti et al., Citation2018; Zmitrewicz et al., Citation2006).
Lateral Sway
Balance is a critical gait metric for clinical populations with mobility limitations given the high probability for falls and their subsequent detrimental impacts (Wong et al., Citation2015). LS is used as a simple measure of balance capability in individuals with amputation (Lamoth et al., Citation2010) and in aging populations (Gill et al., Citation2001). Lateral trunk position variability is correlated with step width, which is used as a gait stability metric (J. A. Perry & Srinivasan, Citation2017). Therefore, LS can provide quantitative insight into functional locomotor capability with relatively simple instrumentation requirements.
Gait Deviation Index
Finally, GDI is a computational method that utilizes 3-dimensional kinematics in comparison to an able-bodied control group. GDI has been used to understand disability and the impacts of medical interventions in a range of individuals with gait impairment including children with cerebral palsy (Kark et al., Citation2012), stroke survivors (Guzik & Drużbicki, Citation2020) and prostheses users (Eshraghi et al., Citation2014). GDI is correlated with simpler outcome measures commonly used in clinical settings such as step length, cadence, self-selected walking speed and distance traversed during the 6-min walk (Guzik & Drużbicki, Citation2020; Kark et al., Citation2016).
While all four metrics share the goal of providing some overall assessment of a person’s gait, they differ from each other in important ways: some of these metrics are more biomechanically comprehensive than others, some require more computationally intensive methods, and others are much simpler in approach. With these fundamental differences in mind, we tested the ability of these four representative gait quality metrics to detect systematically applied lower limb constraint in an able-bodied population. A recent study observed that similarly applied ankle and knee joint constraint can be an effective means to systematically affect locomotor compensatory strategies, as well as metabolic cost (McCain et al., Citation2021). The goal of this work is to provide clinicians and researchers working in gait analysis a more holistic approach to selecting a gait quality metric for individuals with pathological gait. While the selected metrics are widely used in the broader gait community, each has a distinct approach to gait analysis requiring specialized expertise and intrinsic biases that can result in both advantages and disadvantages over other metrics. POGS is visually assessed with minimal instrumentation and easily implemented clinically but is prone to observer subjectivity. IA provides information about how the legs impact whole-body function but requires specialized equipment and may miss gait impairments not asymmetrical in nature. LS can provide a sense of whole-body function using a range of technologies (video camera, wearable sensor or motion capture system), but may have limited ability for assessing localized deficits. GDI presents a highly precise, objective, and computational approach to gait analysis, but requires specialized equipment and analytical expertise. We hypothesized that the most computationally intensive and comprehensive metric, GDI, would distinguish the highest number of tested conditions.
Methods
Participants
Inclusion criteria for participants were as follows: between 18 and 69 years of age, no history of major muscle or bone injuries and no history of major nerve injuries. Participants were excluded based on the following criteria: Presence of dementia or inability to give informed consent, presence of significant or chronic loss of hip, knee or ankle joint motion, history of dizziness and/or balance problems, currently pregnant and presence of diabetes.
Experimental procedures
Participants walked on a custom dual-belt instrumented treadmill at three speeds (0.7 m/s, 0.85 m/s, and 1.0 m/s). These speeds were selected to distinguish between limited (<0.8 m/s) and full community ambulation (> 0.8 m/s) which is a distinguishing feature for determining ability and independence in clinical populations (J. Perry et al., Citation1995). Four joint constraint conditions were imposed on the left lower extremity for a total of 12 different conditions. We collected three walking trials at each condition. The four unilateral joint constraint conditions consisted of a baseline (no joint constraint), ankle constraint, knee constraint and a knee + ankle constraint combination. With the ankle constraint, the participant wore an orthopedic ankle boot (SideKICK Walker, DJO, Lewisville, TX) locked in a 90-degree ankle alignment. The knee constraint consisted of a knee orthosis (Formfit Post-op Knee, Ossur, Reykjavik, ICE) locked in 180 degrees of extension with the addition of a 4-buckle knee pad to reduce knee flexion through deflection of the knee orthosis uprights. The knee + ankle constraint was a combination of both the knee constraint and ankle constraint. All devices were fit to each participant by a certified orthotist to ensure proper fit for inhibiting motion and maximizing comfort. Participants wore their normal walking footwear for the entirety of the experiment except on the ipsilateral leg in conditions with the ankle boot. Additionally, while in conditions wearing the ankle boot, participants were fit with an adjustable external lift (Evenup, OPED Medical, Buford, GA) attached to their contralateral shoe to ensure equal leg lengths. The order of speed conditions was randomized for each participant. To minimize refitting time and ensure consistent constraint across speeds, the four joint constraint conditions always occurred in the following order: baseline, ankle, knee, knee + ankle.
We collected lower body and trunk kinematics using an 8-camera motion capture system (100 and 200 Hz; Vicon, Centennial, CO; Visual 3D, C-Motion, Germantown, MD). Ground reaction forces (960 and 1080 Hz) were recorded using force plates (AMTI, Watertown, MA) embedded within a custom dual-belt treadmill.(Kram et al., Citation1998; Toney & Chang, Citation2013) Retro-reflective markers were placed on anatomical landmarks using a modified Helen Hayes marker set.(Collins et al., Citation2009) Following a three-minute acclimation period, three walking trials were collected for each of the 12 conditions. Synchronized, optical video data were recorded in sagittal and frontal planes (Vicon Bonita cameras) for scoring POGS. Visual 3D was used to filter data (fourth-order Butterworth with lowpass cut-off frequency at 6 Hz for force and marker data), and to calculate joint angles and force impulses. The effects of the imposed joint constraint were characterized by calculating range of motion (ROM) in the sagittal plane of the ankle, knee, and hip joints for each condition. Data were exported to RStudio (Boston, MA) and MATLAB (R2017a, Mathworks, Inc., Natick, MA) for additional processing.
Gait metrics
Prosthetic observational gait score (POGS)
Video footage was reviewed following each trial and scored by an experienced, certified prosthetist/orthotist according to the POGS scale (Hillman et al., Citation2010) with the aid of an on-screen digital goniometer for improved accuracy. The maximum score of 32 is indicative of poorer gait quality and a score of 0 indicative of better gait quality (Hillman et al., Citation2010). Scoring involves systematic analysis of 16 aspects of gait including arm swing, vaulting in stance, lateral and anterior/posterior trunk lean, hip extension and flexion in stance and swing, knee extension in stance, knee flexion in terminal stance, initial swing and terminal swing, step symmetry, first ankle rocker, foot rotation at initial contact, width of the base of support, circumduction and whips. Scores of 0 for each aspect are considered normative movement patterns, whereas scores of 1 or 2 are indicative of pathological movement. The 16 aspects are then summed for a total score.
Impulse Asymmetry (IA)
IA was calculated as the absolute value of the difference between the right and left steps of the vertical ground reaction force impulse for each complete gait cycle and then averaging the difference across all steps.
Impulse asymmetry was calculated using the following equation (Robinson et al., Citation1987):
(1)
(1)
Lateral Sway (LS)
LS was calculated for each stride by taking the difference in the maximum and minimum values of the mediolateral trajectory of a sternal chest marker cluster in the coronal plane as in the following equation:
(2)
(2)
Gait Deviation Index (GDI)
GDI was calculated using the method of Schwartz and Rozumalski (Citation2008). Kinematics data from the pelvis and hip (sagittal, frontal, and transverse planes), sagittal plane data from the knee and ankle joints, and foot progression data in the transverse plane were computed at 2% increments of the gait cycle to arrive at an overall gait quality score that reflects a comparison to an able-bodied control group.
In short, a raw GDI for a given subject’s constraint was first calculated based on the natural logarithm of the Euclidean distance between the feature component vector from the subject’s constraint condition and the mean feature component vector from the baseline condition across all subjects (EquationEquation 3(3)
(3) ). The feature component vectors were derived from performing a singular value decomposition of a matrix containing all the kinematic data points at all the time increments. The GDI, due to a given constraint for a subject, is thus based on a scaled z-score of the raw GDI of the constraint condition from the single subject with respect to the raw GDI of the baseline condition across all subjects (EquationEquation 4
(4)
(4) ).
(3)
(3)
(4)
(4)
For the GDI, scores equal to or greater than 100 indicate a normal gait pattern (Schwartz & Rozumalski, Citation2008).
Data analysis
Two-way (Speed × Joint Constraint) repeated measures ANOVAs were conducted in SPSS (IBM Corp., v22, Armonk, NY) to characterize the effect of each joint constraint on ROM and to determine significant differences between the sensitivity of the different gait metrics to the various constraint-speed conditions. A Greenhouse-Geisser correction was used if Mauchly’s test of sphericity was not met. If a main effect of speed or joint constraint was observed, subsequent pair-wise comparisons were performed using Bonferroni corrections. Since the GDI metric is based on a z-score, statistical significance for comparisons to the baseline condition were defined as a GDI score lower than 80.4/100, which represents a difference greater than 1.96 standard deviations (95% confidence level); z-scores were subsequently converted into p-values based on a normal distribution curve. For comparisons between the remaining constraint-speed conditions for the GDI metric, we used the same repeated measures ANOVA statistical analysis described above. We defined significance at α = 0.05 throughout our analysis. To compare the ability of each gait metric to distinguish across different walking conditions, we computed the number of significant comparison detections tallied across all nine possible comparisons.
Results
Participants
Nine able-bodied individuals (39.3 ± 16.7 years, 82 ± 30.8 kg, height 1.7 ± 0.08 m) provided informed, written consent prior to participating in this study approved by the Georgia Institute of Technology Institutional Review Board under protocol H17290.
Effect of constraint on range of motion
The combined effect of changes in speed and joint constraint led to significant changes in ROM of all joints of the lower limbs. A main effect of joint constraint was observed for the hip (p=.017), knee (p<.001), and ankle (p<.001) ipsilateral to the imposed constraints, as well as the hip of the contralateral leg (p=.006). A main effect of speed was observed for all six joints. The ankle constraint led to an average reduction of ankle ROM by 83.1 ± 7.1% and the knee constraint led to a reduction in knee ROM by 64.1 ± 12.8%, indicating that the imposed joint constraints functioned as intended to systematically alter the gait pattern (ta 1) ().
Figure 1. Sagittal plane kinematic trajectories of the hip, knee and ankle for a representative participant walking at 1.0 m/s in the four different joint constraint conditions indicating that the constraints functioned as intended to alter gait.

Each metric was able to detect different levels of joint constraint (). No significant interaction effects were observed between joint constraint and speed.
Table 1. Statistical comparisons between conditions showing p-values, and significant (p ≤ 0.05) differences between constraint condition comparisons and speed condition comparisons are in bolded text with an asterisk*.
POGS
Overall, POGS () increased with more severe joint constraint indicating poorer gait quality and detected differences in four of the six different joint constraint comparisons. POGS distinguished the Knee condition from the Baseline condition and Ankle condition (p < 0.001 and p = 0.002, respectively). POGS also distinguished the Knee + Ankle condition from the Baseline condition and Ankle condition (p = 0.001 and p = 0.002, respectively).
Figure 2. Results of Prosthetic Observational Gait Score indicating differences between baseline and knee and knee + ankle constraint conditions as well as between ankle and knee + ankle and knee + ankle. Error bars represent the standard deviation across participants. † indicates significant difference compared to baseline condition, ‡ indicates significant difference compared to ankle condition.

Impulse Asymmetry
IA () increased indicating poorer gait quality with more severe joint constraint and detected differences between three of the six different joint constraint comparisons. IA distinguished the Knee + Ankle condition from the Baseline condition, the Ankle condition and the Knee condition (p = 0.020, p = 0.014, and p = 0.019, respectively).
Figure 3. Results of Impulse Asymmetry indicating significant differences seen between knee + ankle constraint and every other constraint condition. Error bars represent the standard deviation across subjects. † indicates significant difference compared to baseline condition, ‡ indicates significant difference compared to ankle condition, ⋄ indicates significant difference from knee condition.

Lateral Sway
LS () increased with more severe joint constraint and distinguished differences between three of six possible joint constraint comparisons and two of the three speed comparisons. LS distinguished the Baseline condition from the Knee condition (p = 0.017) and the Ankle condition from the Ankle + Knee condition (p = 0.004). Additionally, LS distinguished the 0.7 m/s speed condition from the 0.85 m/s and 1.0 m/s speed conditions (p = 0.006 and p = 0.002, respectively).
Figure 4. Results of Lateral Sway metric indicating differences seen in speed and joint constraint comparisons between the baseline and ankle constraint and knee + ankle conditions as well as between the ankle constraint and knee + ankle conditions. Error bars represent the standard deviation across participants. *indicates significant difference compared to 0.7 m/s speed, † indicates significant difference compared to baseline condition, ‡ indicates significant difference compared to ankle condition.

Gait Deviation Index
GDI () decreased with more severe joint constraint, indicating poorer gait quality and distinguished four of the six different joint constraint comparisons. GDI distinguished the Baseline condition from the Ankle condition, Knee condition and Knee + Ankle condition (p < 0.001, p < 0.001, and p < 0.001, respectively). GDI also distinguished the Ankle condition from the Knee + Ankle condition (p = 0.045).
Figure 5. Results of Gait Deviation Index indicating significant differences between the baseline (i.e., GDI = 100) and every other constraint condition as well as differences between the ankle constraint and knee + ankle conditions. Error bars represent the standard deviation across participants. † indicates significant difference compared to baseline condition, ‡ indicates significant difference compared to ankle condition.

Overall detection rate
We observed that GDI and POGS performed better than LS and IA at detecting the most significant differences between the various joint constraint conditions (). Both GDI and POGS could each distinguish differences in four of six joint constraint comparisons with overlap of distinguishing 3 of the same comparisons and 2 distinct comparisons. In contrast, LS and IA could only distinguish 3/6 and 2/6 joint constraint comparisons, respectively. When we also consider the ability to detect changes due to speed, Lateral Sway distinguished 5/9 comparisons. GDI and POGS performed similarly detecting 4 out of 9 comparisons each, and IA distinguished 3/9 comparisons. The observed power for each of the four metrics was high (>0.95) when assessing the main effect of joint constraint, but LS was the only metric with sufficient power to detect the main effect of speed ().
Table 2. Post hoc power analysis indicating high power (>0.95) when assessing the main effect of joint constraint and Lateral Sway as the only metric with sufficient power to detect the main effect of speed.
Discussion
There is a known need for metrics in the gait analysis community that are concise and allow for an objective measure of an individual’s gait quality without the complexities and time considerations required with three-dimensional gait analysis (Cimolin & Galli, Citation2014). However, these metrics should still be validated using the gold standard of three-dimensional gait analysis in the lab prior to their implementation outside of the lab (Toro et al., Citation2003). In this study, we anticipated that the most comprehensive and computationally intensive metric requiring use of three-dimensional gait analysis methods, GDI, would outperform the other metrics in distinguishing joint-constrained gait. However, our data did not support this hypothesis, as we found that the detection of constrained gait is not necessarily dependent on metric complexity and is more nuanced, depending on what aspects of gait the investigator aims to detect. For example, in Lee et al.’s (Citation2023) work on children with walking impairment, kinematics were specifically targeted and improved with use of the wearable intervention, but spatiotemporal metrics did not improve. An investigation into either spatiotemporal aspects of gait quality or kinematics alone would have missed that while one aspect of gait quality improved, another did not- underscoring the importance of global gait quality monitoring in clinical populations.
In this work, we found that POGS outperformed some of the more computationally intensive metrics. Both POGS and GDI performed equally in detecting constraint comparisons, and GDI outperformed all other selected metrics in distinguishing differences between the baseline condition and each joint constraint condition, which is particularly compelling for an automated system intended to objectively assess gait quality. Both POGS and GDI consider multiple joint angles in their score calculation, which perhaps explains their superior performance over the other metrics. While POGS evaluates some spatiotemporal aspects of gait, both POGS and GDI are largely kinematic-based assessments and may miss deviations that are kinetic in nature. While POGS considers all anatomical segments of the participant (Hillman et al., Citation2010), GDI focuses strictly on the pelvis and lower limbs.(Schwartz & Rozumalski, Citation2008) POGS is a noted metric in the orthotics and prosthetics clinical profession for its ability to lead to more objective and reliable methods than visual gait analysis alone (Simon et al., Citation2021). However, POGS’ clinical basis may limit automation, and scoring may vary depending on experience and clinical specialty (Watelain et al., Citation2005). In this study, the subjective POGS scoring was assisted by the use of a digital goniometer and video playback, which likely reduced subjectivity of gait analysis more than visual observation alone (Fatone & Stine, Citation2015). This use of the digital goniometer likely represents an upper bound of performance for the POGS metric compared to other scorers with less experience or access to the tools used here. Given its high detection rate and relatively easy scoring system, further exploration to implement markerless machine learning tools (Mathis et al., Citation2018) or inertial measurement unit sensors (Angelini et al., Citation2019) to automate and detect the characteristics of the more sensitive aspects of POGS (i.e. vaulting or trunk lean) may be worthwhile and provide a means toward future operationalization in the form of a wearable sensor. It is important to note that a limitation of POGS in the scope of this study is that it could not be blinded as the evaluator could discern the experimental condition due to the observational nature of the metric. However, the obvious nature of the employed joint constraint (reducing ROM to minimal levels) makes it difficult to imagine a scenario where such an analysis could be successfully blinded. Despite initial development for assessing prosthetic gait, POGS was able to distinguish gait of several different lower limb orthotic joint constraints in this study.
Only LS was able to distinguish any of the speed comparisons. Specifically, LS was able to detect differences between speeds that characterize ‘limited community ambulation’ (<0.8 m/s) and ‘full community ambulation’ (≥ 0.8 m/s). While we did not measure preferred walking speed, all participants were able-bodied, healthy community ambulators, and it is likely that the 0.7 m/s speed condition represented a significant deviation from their typical preferred walking speed. So, it is of particular interest that a relatively simple metric like LS could be used to identify individuals with limited community ambulation ability. Speed is known to be an important measure of community mobility and correlated with overall health and predictive of functional dependence, frailty, mobility disability and falls (Middleton et al., Citation2015; Wonsetler & Bowden, Citation2017). Slower walking speeds are also correlated with reduced quality of life, decreased participation and symptoms of depression (Middleton et al., Citation2015). Thus, LS may serve as a means to proactively detect change to gait speeds in clinical populations that may indicate greater risk for falls or depression, warranting new or additional intervention.
The two relatively simple measures, LS and IA, each detected differences in three of six joint constraint comparisons. LS distinguished conditions which could not be distinguished by IA, and vice-versa. It is easy to imagine using small, independent wearable sensors to non-intrusively quantify these gait metrics. For example, detailed motions of the trunk have been measured using the SwayStar System (Balance Int. Innovations GmbH, Iseltwald, CHE), which is worn around the lower trunk (Adkin et al., Citation2005; Van de Warrenburg et al., Citation2005). Neumann et al. used a load cell in the distal aspect of a prosthesis user’s socket to detect moments on the residual limb when walking on a curved path (Neumann et al., Citation2014), and Chen et al. used load cells in a shoe-like system to study walking in stroke survivors (H.-C. Chen et al., Citation2007) If miniaturized further, IA could be operationalized in the form of load cells embedded within an individual’s regular shoes or insoles and subsequently used to detect force impulse (Bhakta et al., Citation2020). Automatically fusing IA and LS through these two different sensor inputs may allow for the assessment of gait quality across a range of function at different walking speeds.
IA performed the worst in detecting our joint constraint comparisons indicating its reduced ability to discriminate across the conditions tested here. Similar to this finding, Seeley et al. also found no differences in IA between the dominant and non-dominant limbs in able-bodied participants walking at preferred speed (Seeley et al., Citation2008). This highlights one potential limitation relating to IA in this study in that our joint constraints were applied to the left leg in all participants without determining leg dominance. Since each participant served as their own control in this study, this influence of leg dominance on our general conclusions is likely minimal. In contrast, clinical literature suggests notable differences in ground reaction forces between limbs during walking in stroke survivors (Allen et al., Citation2011; Balasubramanian et al., Citation2007; C.-L. Chen et al., Citation2001), and other clinical populations (Cutti et al., Citation2018; White et al., Citation2005; Zmitrewicz et al., Citation2006), indicating that the perturbation applied herein may not have been disruptive enough to be detected by IA. Therefore, we would conclude that IA may be better suited for detecting more severe gait pathology and less well suited for mild to moderate gait impairments.
Given the varying ability of these four metrics to detect changes in gait quality, a single sensor and single metric alone may not suffice for assessing overall gait quality. There is precedent in the literature which shows fusion of multiple sensors as opposed to a single sensor improved performance of a powered knee and ankle prosthesis (Bhakta et al., Citation2020). Notably, we were able to detect differences between every single experimental condition when using all four gait metrics simultaneously. The use of these metrics in the form of future sensors in community settings has great potential because they will reflect gait of daily life versus constrained, and likely unrealistic, gait in the lab (Puthoff et al., Citation2008; Suri et al., Citation2021). Our study is limited by a lower sample size, which is commonly seen in studies investigating gait outcomes of wearable interventions in populations with movement disabilities (Bailes et al., Citation2010; Giest & Chang, Citation2016; Herrin et al., Citation2023) as we attempt to simulate here. Nonetheless, a larger sample size may have yielded additional differences observed between conditions for the four selected metrics.
In this study, we compared the ability of four commonly used metrics, representing varying approaches to gait assessment, to detect changes in gait quality when an able-bodied individual is challenged with systematically applied lower limb joint constraint. We were able to draw general conclusions about how each gait metric might perform when applied to someone with a pathological impairment, even though the intervention did not precisely represent the deficits of an individual with gait impairment. These findings provide broad insights into how limitations in joint ROM can affect gait and how we may best detect the resulting changes in gait quality.
Conclusion
Improving the ability to objectively quantify and automatically detect gait quality outside of the gait analysis laboratory may significantly improve a researcher or clinician’s ability to prescribe, administer, and monitor treatment of individuals with gait pathology for re-establishing a more normal gait pattern. Based on our results and observations, comprehensive metrics such as GDI and POGS are recommended for researchers interested in detecting kinematic gait deviations relative to an able-bodied, unconstrained baseline. LS and IA could be implemented together to detect clinically relevant changes in walking speed and more severe disruptions to gait quality. We note that all the metrics systematically tested herein may be operationalized in the form of future wearable sensors that would allow for the monitoring of gait quality without supervision in the lab or clinic, which is meaningful for both clinicians and researchers alike. While this work sought to test whether a single metric was capable of detecting differences in gait and speed, it highlights (1) that more computationally intensive methods, which often require specialized equipment may not be necessary for detecting certain types of gait deficits, and (2) that fusion of multiple gait metrics can more comprehensively assess an individuals’ gait quality. When designing a study investigating gait quality, we recommend clinicians and researchers include multiple gait metrics to avoid the potential bias that using only a single metric can induce.
Acknowledgements
The authors gratefully acknowledge the Georgia Tech EPIC and PoWeR labs for use of their laboratory space for this experiment. Additionally, the authors acknowledge the efforts of Margaret Berry and Navya Katragadda in processing the Vicon data.
Disclosure statement
No potential conflict of interest was reported by the author(s).
Data availability statement
The data that support the findings of this study are available from the corresponding author, KH, upon reasonable request.
Additional information
Funding
References
- Adkin, A., Bloem, B., & Allum, J. (2005). Trunk sway measurements during stance and gait tasks in Parkinson’s disease. Gait & Posture, 22(3), 240–249. https://doi.org/10.1016/j.gaitpost.2004.09.009
- Allen, J. L., Kautz, S. A., & Neptune, R. R. (2011). Step length asymmetry is representative of compensatory mechanisms used in post-stroke hemiparetic walking. Gait & Posture, 33(4), 538–543. https://doi.org/10.1016/j.gaitpost.2011.01.004
- Angelini, L., Carpinella, I., Cattaneo, D., Ferrarin, M., Gervasoni, E., Sharrack, B., Paling, D., Nair, K. P. S., & Mazzà, C. (2019). Is a wearable sensor-based characterisation of gait robust enough to overcome differences between measurement protocols? A multi-centric pragmatic study in patients with multiple sclerosis. Sensors, 20(1), 79. https://doi.org/10.3390/s20010079
- Bailes, A. F., Greve, K., & Schmitt, L. C. (2010). Changes in two children with cerebral palsy after intensive suit therapy: A case report. Pediatric Physical Therapy, 22(1), 76–85. https://doi.org/10.1097/PEP.0b013e3181cbf224
- Balasubramanian, C. K., Bowden, M. G., Neptune, R. R., & Kautz, S. A. (2007). Relationship between step length asymmetry and walking performance in subjects with chronic hemiparesis. Archives of Physical Medicine and Rehabilitation, 88(1), 43–49. https://doi.org/10.1016/j.apmr.2006.10.004
- Balasubramanian, C. K., Neptune, R. R., & Kautz, S. A. (2009). Variability in spatiotemporal step characteristics and its relationship to walking performance post-stroke. Gait & Posture, 29(3), 408–414. https://doi.org/10.1016/j.gaitpost.2008.10.061
- Bhakta, K., Camargo, J., Donovan, L., Herrin, K., Young, A., Valdastri, P., & Rouse, E. (2020). Machine learning model comparisons of user independent & dependent intent recognition systems for powered prostheses. IEEE Robotics and Automation Letters, 5(4), 5393–5400. https://doi.org/10.1109/LRA.2020.3007480
- Brunnekreef, J. J., Van Uden, C. J., van Moorsel, S., & Kooloos, J. G. (2005). Reliability of videotaped observational gait analysis in patients with orthopedic impairments. BMC Musculoskeletal Disorders, 6(1), 1–9. https://doi.org/10.1186/1471-2474-6-17
- Chen, C.-L., Chen, H.-C., Wong, M.-K., Tang, F.-T., & Chen, R.-S. (2001). Temporal stride and force analysis of cane-assisted gait in people with hemiplegic stroke. Archives of Physical Medicine and Rehabilitation, 82(1), 43–48. https://doi.org/10.1053/apmr.2001.18060
- Chen, H.-C., Chen, C.-L., Chen, Y.-M., Wong, A. M., & Lee, J. (2007). Instrumented shoes for measuring ground-reaction force of persons with stroke in level walking, stair ascending and descending [Paper presentation]. 3rd Kuala Lumpur International Conference on Biomedical Engineering 2006: Biomed 2006, 11–14. December 2006 Kuala Lumpur, Malaysia.
- Cimolin, V., & Galli, M. (2014). Summary measures for clinical gait analysis: A literature review. Gait & Posture, 39(4), 1005–1010. https://doi.org/10.1016/j.gaitpost.2014.02.001
- Collins, T. D., Ghoussayni, S. N., Ewins, D. J., & Kent, J. A. (2009). A six degrees-of-freedom marker set for gait analysis: repeatability and comparison with a modified Helen Hayes set. Gait & Posture, 30(2), 173–180. https://doi.org/10.1016/j.gaitpost.2009.04.004
- Cutti, A. G., Verni, G., Migliore, G. L., Amoresano, A., & Raggi, M. (2018). Reference values for gait temporal and loading symmetry of lower-limb amputees can help in refocusing rehabilitation targets. Journal of Neuroengineering and Rehabilitation, 15(Suppl 1), 61. https://doi.org/10.1186/s12984-018-0403-x
- Del Din, S., Godfrey, A., Mazzà, C., Lord, S., & Rochester, L. (2016). Free‐living monitoring of Parkinson’s disease: Lessons from the field. Movement Disorders, 31(9), 1293–1313. https://doi.org/10.1002/mds.26718
- Del Din, S., Hickey, A., Woodman, S., Hiden, H., Morris, R., Watson, P., Nazarpour, K., Catt, M., Rochester, L., & Godfrey, A. (2016). Accelerometer-based gait assessment: Pragmatic deployment on an international scale [Paper presentation]. 2016 IEEE Statistical Signal Processing Workshop (SSP). https://doi.org/10.1109/SSP.2016.7551794
- Duffy, C., Dolan, M., & Buis, A. (2019 Comparison of function and mobility pre and post prescription of a microprocessor-controlled knee joint [Paper presentation]. British Association of Prosthetists and Orthotists Conference.
- Eshraghi, A., Osman, N. A. A., Karimi, M., Gholizadeh, H., Soodmand, E., & Abas, W. A. B. W. (2014). Gait biomechanics of individuals with transtibial amputation: Effect of suspension system. PloS One, 9(5), e96988. https://doi.org/10.1371/journal.pone.0096988
- Fatone, S., & Stine, R. (2015). Capturing quality clinical videos for two-dimensional motion analysis. JPO Journal of Prosthetics and Orthotics, 27(1), 27–32. https://doi.org/10.1097/JPO.0000000000000051
- Giest, T. N., & Chang, Y.-H. (2016). Biomechanics of the human walk-to-run gait transition in persons with unilateral transtibial amputation. Journal of Biomechanics, 49(9), 1757–1764. https://doi.org/10.1016/j.jbiomech.2016.04.004
- Gill, J., Allum, J., Carpenter, M., Held-Ziolkowska, M., Adkin, A., Honegger, F., & Pierchala, K. (2001). Trunk sway measures of postural stability during clinical balance tests: effects of age. The Journals of Gerontology. Series A, Biological Sciences and Medical Sciences, 56(7), M438–M447. https://doi.org/10.1093/gerona/56.7.m438
- Guzik, A., & Drużbicki, M. (2020). Application of the Gait Deviation Index in the analysis of post-stroke hemiparetic gait. Journal of Biomechanics, 99, 109575. https://doi.org/10.1016/j.jbiomech.2019.109575
- Herrin, K., Upton, E., & Young, A. (2023). Towards meaningful community ambulation in individuals post stroke through use of a smart hip exoskeleton: A preliminary investigation. Assistive Technology, 2023 Aug 4, 1–11. https://doi.org/10.1080/10400435.2023.2239555
- Highsmith, M. J., Schulz, B. W., Hart-Hughes, S., Latlief, G. A., & Phillips, S. L. (2010). Differences in the spatiotemporal parameters of transtibial and transfemoral amputee gait. JPO Journal of Prosthetics and Orthotics, 22(1), 26–30. https://doi.org/10.1097/JPO.0b013e3181cc0e34
- Hillman, S. J., Donald, S. C., Herman, J., McCurrach, E., McGarry, A., Richardson, A. M., & Robb, J. E. (2010). Repeatability of a new observational gait score for unilateral lower limb amputees. Gait & Posture, 32(1), 39–45. https://doi.org/10.1016/j.gaitpost.2010.03.007
- Hutabarat, Y., Owaki, D., & Hayashibe, M. (2021). Recent advances in quantitative gait analysis using wearable sensors: a review. IEEE Sensors Journal, 21(23), 26470–26487. https://doi.org/10.1109/JSEN.2021.3119658
- Jourdan, T., Debs, N., & Frindel, C. (2021). The contribution of machine learning in the validation of commercial wearable sensors for gait monitoring in patients: A systematic review. Sensors, 21(14), 4808. https://doi.org/10.3390/s21144808
- Kaczmarczyk, K., Wit, A., Krawczyk, M., & Zaborski, J. (2009). Gait classification in post-stroke patients using artificial neural networks. Gait & Posture, 30(2), 207–210. https://doi.org/10.1016/j.gaitpost.2009.04.010
- Kark, L., Odell, R., McIntosh, A. S., & Simmons, A. (2016). Quantifying prosthetic gait deviation using simple outcome measures. World Journal of Orthopedics, 7(6), 383–391. https://doi.org/10.5312/wjo.v7.i6.383
- Kark, L., & Simmons, A. (2011). Patient satisfaction following lower-limb amputation: The role of gait deviation. Prosthetics and Orthotics International, 35(2), 225–233. https://doi.org/10.1177/0309364611406169
- Kark, L., Vickers, D., McIntosh, A., & Simmons, A. (2012). Use of gait summary measures with lower limb amputees. Gait & Posture, 35(2), 238–243. https://doi.org/10.1016/j.gaitpost.2011.09.013
- Kiani, K., Snijders, C., & Gelsema, E. (1997). Computerized analysis of daily life motor activity for ambulatory monitoring. Technology and Health Care, 5(4), 307–318. https://doi.org/10.3233/THC-1997-5404
- Kram, R., Griffin, T. M., Donelan, J. M., & Chang, Y. H. (1998). Force treadmill for measuring vertical and horizontal ground reaction forces. Journal of Applied Physiology, 85(2), 764–769. https://doi.org/10.1152/jappl.1998.85.2.764
- Lamoth, C. J., Ainsworth, E., Polomski, W., & Houdijk, H. (2010). Variability and stability analysis of walking of transfemoral amputees. Medical Engineering & Physics, 32(9), 1009–1014. https://doi.org/10.1016/j.medengphy.2010.07.001
- Lee, D., Shepherd, M. K., Mulrine, S. C., Schneider, J., Moore, K., Eggebrecht, E., Rogozinski, B., Herrin, K., & Young, A. J. (2023). Reducing knee hyperextension with an exoskeleton in children and adolescents with genu recurvatum: A feasibility study. IEEE Transactions on Bio-Medical Engineering, 70(12), 3312–3320. https://doi.org/10.1109/TBME.2023.3282165
- Lewek, M. D., Bradley, C. E., Wutzke, C. J., & Zinder, S. M. (2014). The relationship between spatiotemporal gait asymmetry and balance in individuals with chronic stroke. Journal of Applied Biomechanics, 30(1), 31–36. https://doi.org/10.1123/jab.2012-0208
- Lythgo, N., Marmaras, B., & Connor, H. (2010). Physical function, gait, and dynamic balance of transfemoral amputees using two mechanical passive prosthetic knee devices. Archives of Physical Medicine and Rehabilitation, 91(10), 1565–1570. https://doi.org/10.1016/j.apmr.2010.07.014
- Mathis, A., Mamidanna, P., Cury, K. M., Abe, T., Murthy, V. N., Mathis, M. W., & Bethge, M. (2018). DeepLabCut: Markerless pose estimation of user-defined body parts with deep learning. Nature Neuroscience, 21(9), 1281–1289. https://doi.org/10.1038/s41593-018-0209-y
- McCain, E. M., Libera, T. L., Berno, M. E., Sawicki, G. S., Saul, K. R., & Lewek, M. D. (2021). Isolating the energetic and mechanical consequences of imposed reductions in ankle and knee flexion during gait. Journal of Neuroengineering and Rehabilitation, 18(1), 21. https://doi.org/10.1186/s12984-021-00812-8
- Middleton, A., Fritz, S. L., & Lusardi, M. (2015). Walking speed: The functional vital sign. Journal of Aging and Physical Activity, 23(2), 314–322. https://doi.org/10.1123/japa.2013-0236
- Neumann, E. S., Brink, J., Yalamanchili, K., & Lee, J. S. (2014). Use of a load cell and force–moment curves to compare transverse plane moment loads on transtibial residual limbs: A preliminary investigation. Prosthetics and Orthotics International, 38(3), 253–262. https://doi.org/10.1177/0309364613497048
- Perry, J., Garrett, M., Gronley, J. K., & Mulroy, S. J. (1995). Classification of walking handicap in the stroke population. Stroke, 26(6), 982–989. https://doi.org/10.1161/01.str.26.6.982
- Perry, J. A., & Srinivasan, M. (2017). Walking with wider steps changes foot placement control, increases kinematic variability and does not improve linear stability. Royal Society Open Science, 5(1), 172000. https://doi.org/10.1098/rsos.160627
- Puthoff, M. L., Janz, K. F., & Nielson, D. (2008). The relationship between lower extremity strength and power to everyday walking behaviors in older adults with functional limitations. Journal of Geriatric Physical Therapy, 31(1), 24–31. https://doi.org/10.1519/00139143-200831010-00005
- Rast, F. M., & Labruyère, R. (2020). Systematic review on the application of wearable inertial sensors to quantify everyday life motor activity in people with mobility impairments. Journal of Neuroengineering and Rehabilitation, 17(1), 148. https://doi.org/10.1186/s12984-020-00779-y
- Rathinam, C., Bateman, A., Peirson, J., & Skinner, J. (2014). Observational gait assessment tools in paediatrics–A systematic review. Gait & Posture, 40(2), 279–285. https://doi.org/10.1016/j.gaitpost.2014.04.187
- Read, F. A., Boyd, R. N., & Barber, L. A. (2017). Longitudinal assessment of gait quality in children with bilateral cerebral palsy following repeated lower limb intramuscular Botulinum toxin-A injections. Research in Developmental Disabilities, 68, 35–41. https://doi.org/10.1016/j.ridd.2017.07.002
- Roberts, M., Mongeon, D., & Prince, F. (2017). Biomechanical parameters for gait analysis: a systematic review of healthy human gait. Physical Therapy and Rehabilitation, 4(1), 6. https://doi.org/10.7243/2055-2386-4-6
- Robinson, R. O., Herzog, W., & Nigg, B. M. (1987). Use of force platform variables to quantify the effects of chiropractic manipulation on gait symmetry. Journal of Manipulative and Physiological Therapeutics, 10(6), 295–299.
- Sayeed, M. S., Min, P. P., & Bari, M. A. (2022). Deep learning based gait recognition using convolutional neural network in the COVID-19 pandemic. Emerging Science Journal, 6(5), 1086–1099. https://doi.org/10.28991/ESJ-2022-06-05-012
- Schwartz, M. H., & Rozumalski, A. (2008). The Gait Deviation Index: A new comprehensive index of gait pathology. Gait & Posture, 28(3), 351–357. https://doi.org/10.1016/j.gaitpost.2008.05.001
- Seeley, M. K., Umberger, B. R., & Shapiro, R. (2008). A test of the functional asymmetry hypothesis in walking. Gait & Posture, 28(1), 24–28. https://doi.org/10.1016/j.gaitpost.2007.09.006
- Simon, J. C., Kruger, K., Krzak, J. J., Altiok, H., & Harris, G. F. (2021). Joint contact force model for patients with knee height asymmetry [Virtual poster presentation]. Transactions of the 66th Annual Meeting of the Orthopaedic Research Society. February 13-16, 2021.
- Suri, A., Rosso, A. L., VanSwearingen, J., Coffman, L. M., Redfern, M. S., Brach, J. S., & Sejdić, E. (2021). Mobility of older adults: Gait quality measures are associated with life-space assessment scores. The Journals of Gerontology. Series A, Biological Sciences and Medical Sciences, 76(10), e299–e306. https://doi.org/10.1093/gerona/glab151
- Toney, M. E., & Chang, Y.-H. (2013). Humans robustly adhere to dynamic walking principles by harnessing motor abundance to control forces. Experimental Brain Research, 231(4), 433–443. https://doi.org/10.1007/s00221-013-3708-9
- Toro, B., Nester, C., & Farren, P. (2003). A review of observational gait assessment in clinical practice. Physiotherapy Theory and Practice, 19(3), 137–149. https://doi.org/10.1080/09593980307964
- Van de Warrenburg, B. P., Bakker, M., Kremer, B. P., Bloem, B. R., & Allum, J. H. (2005). Trunk sway in patients with spinocerebellar ataxia. Movement Disorders, 20(8), 1006–1013. https://doi.org/10.1002/mds.20486
- Wang, Q., Tong, G., & Zhou, S. (2023). A study of dance movement capture and posture recognition method based on vision sensors. HighTech and Innovation Journal, 4(2), 283–293. https://doi.org/10.28991/HIJ-2023-04-02-03
- Watelain, E., Froger, J., Rousseaux, M., Lensel, G., Barbier, F., Lepoutre, F.-X., & Thevenon, A. (2005). Variability of video-based clinical gait analysis in hemiplegia as performed by practitioners in diverse specialties. Journal of Rehabilitation Medicine, 37(5), 317–324. https://doi.org/10.1080/16501970510035610
- White, R., Agouris, I., & Fletcher, E. (2005). Harmonic analysis of force platform data in normal and cerebral palsy gait. Clinical Biomechanics, 20(5), 508–516. https://doi.org/10.1016/j.clinbiomech.2005.01.001
- Wong, C. K., Chen, C. C., Blackwell, W. M., Rahal, R. T., & Benoy, S. A. (2015). Balance ability measured with the Berg balance scale: A determinant of fall history in community-dwelling adults with leg amputation. Journal of Rehabilitation Medicine, 47(1), 80–86. https://doi.org/10.2340/16501977-1882
- Wonsetler, E. C., & Bowden, M. G. (2017). A systematic review of mechanisms of gait speed change post-stroke. Part 1: Spatiotemporal parameters and asymmetry ratios. Topics in Stroke Rehabilitation, 24(6), 435–446. https://doi.org/10.1080/10749357.2017.1285746
- Zmitrewicz, R. J., Neptune, R. R., Walden, J. G., Rogers, W. E., & Bosker, G. W. (2006). The effect of foot and ankle prosthetic components on braking and propulsive impulses during transtibial amputee gait. Archives of Physical Medicine and Rehabilitation, 87(10), 1334–1339. https://doi.org/10.1016/j.apmr.2006.06.013