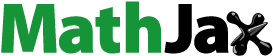
Abstract
Advancements in technology and the compulsion to use environment-friendly materials have been challenging tasks for researchers for the past two decades. Researchers have been focusing on the utilization of plant fibers to produce good quality fiber-reinforced polymer/polyester composites for automobile, structural, and building applications. Researchers have been looking for high-quality and cost-effective drilling processes. The primary goal of this study is to identify optimal drilling conditions for CCFRP composite laminates, affecting thrust force and torque. This is achieved by manipulating drilling process variables using Taguchi’s Design of Experiments (TDOE), Analysis of variance (ANOVA), Response Surface Methodology (RSM), Desirability Function Analysis (DFA) and Artificial Neural Network (ANN). From the results, it was observed that the spindle speed of 2000 rpm, feed of 15 mm/min, point angle of 90°, fiber length of 6 mm, fiber volume of 30%, and fiber diameter of 7 microns gave the optimum results for obtaining minimum thrust force and torque. Further RSM revealed that an increase in fiber vol % and a decrease in spindle speed resulted in an increase in thrust force and torque. From DFA optimization results, the minimum thrust force of 24.0042 N and minimum torque of 0.8001 N-m was obtained. Finally, the experimental values of thrust force and torque were compared with the corresponding values predicted by the MLP-ANN model. The average error percentage for thrust force and torque was 1.75% and 6.56% respectively.
Reviewing Editor:
1. Introduction
Today due to environmental concerns, natural fiber-reinforced polymer composite materials are in increasing demand in manufacturing sector owing to their outstanding characteristics such as being renewable and biodegradable. Natural fiber-reinforced polymer composite materials have been widely used in defence, transportation and automotive applications. Result from the research work in the field of drilling of composite materials has reported that the process input parameters will affect “the quality of the drilled holes. Machining of FRPs causes fiber pullout, particle fracture, excessive tool wear, delamination, debonding at the fiber and matrix interface and severe environmental and health hazards.” Hence, it is very important to select the “reinforcement type, fiber orientation, tool type and material and machining parameters,” while machining these materials (Sapuan et al., Citation2016). There are various techniques for drilling holes in composite materials, but the traditional drilling method is most commonly adopted (Arola et al., Citation2002; Pramanik et al. Citation2006). The study carried out by, (Singh & Bhatnagar, Citation2006; Valarmathi et al., Citation2013) suggested that, “high spindle speed with low feed rate minimizes the thrust force in drilling wood based composites.” Study carried out to determine the effect of cutting parameters on thrust force and torque during drilling has identified that, “feed of 150 mm/min and cutting speeds above 600 rpm” are ideal for drilling composite laminates. (Franke, Citation2011) generated a mathematical model and suggested that cutting edge had maximum influence on the quality of a drilled hole. Thrust force analysis while drilling of carbon fiber laminates along with delamination analysis has been carried out by Khashaba et al. (Citation2021). They suggested that use of a special step drill resulted in minimum thrust force and delamination. Study carried out by, (Murthy et al., Citation2010 & Mohan et al., Citation2005) suggested that, “drill geometry had maximum effect on quality of the drilled hole in comparison with other parameters.” (Jayabal et al. Citation2011) has analysed the mechanical characteristics along with machinability characteristics of glass-coir polyester composites using methodology of factorial design. They suggested that feed rate had maximum effect on output process parameters. (Hasan et al., Citation2021) suggested that while machining of fibre composites there is an adverse effect on reinforcement and matrix. (Singh et al., Citation2020) suggested that during drilling of sisal-glass fiber reinforced polymer composites delamination, crack development, fibre breakage, fibre pull-out and matrix fibre debonding are the route cause for poor machining quality. (Chegdani & El-Mansori, Citation2018) carried out drillability study on flax fibre composites. They suggested that coated drill bit produced highest surface damage and resulted in poor surface roughness. Study carried out by, (Rezghi Maleki et al. Citation2019) suggested that HSS tool produced lower tool wear and improved surface roughness value during machining of natural fibres compared to solid carbide tool. (Debnath et al., Citation2017) carried out research on drilling of needle fibre composites under different drill tool geometry. They suggested that parabolic drill bit had considerable effect on thrust force value compared to four-facet and step drill bit. (Arputhabalan et al., Citation2019) carried out experiments on four different types of natural fiber composites having matrix material as vinyl ester and poly vinyl chloride. They suggested that vinyl ester gave minimum thrust force and lesser delamination compared to poly vinyl chloride. (Díaz-Álvarez et al., Citation2018) suggested that while drilling of cotton and jute fibre composites exhibited improved surface quality compared to flax composites. (Kumar et al. Citation2017) suggested that at lower cutting forces continuous and discontinuous chips were obtained while drilling of coir fibre composites. (Gokul & Rajasekaran, Citation2018) suggested that as sugarcane fibre weight percentage decreases the thrust force value reduced. Lotfi et al. reported that as the thrust force value increases the delamination also increases while drilling flax fiber reinforced bio-composites. There are extensive studies conducted on hybrid optimization technique to control machining performance of graphene/carbon/polymer nano-composites (Kumar, Abhishek, et al., Citation2021). The authors (Nasir et al., Citation2019) suggested that reduction in feed rate results in decrease in delamination while drilling flax fiber composites. Carbon nano-tube/Glass fiber modified hybrid composites are milled and the milling performance optimization is conducted with an integrated module. Further delamination assessment is also conducted (Kumar, Abhishek, et al., Citation2021; Kumar, Kumar, et al., Citation2021). The study carried out by, (Rajmohan & Palanikumar, Citation2012) adopted desirability function for optimizing drilling process parameters and concluded that spindle speed and feed rate had maximum effect on burr and surface roughness while drilling hybrid composites. (Shetty et al., Citation2019) suggested that, “selection of spindle speed (1500 rpm), feed rate (10 mm/min1) and point angle (90°)” has resulted in the best combination to get better values of surface roughness and minimized delamination. Researchers have successfully developed a multiple criterion optimization during end milling of Graphene Oxide doped CFRP hybrid composites (Kumar & Kumar et al., Citation2020). Artificial Neural Networks (ANNs) have emerged as powerful tools for predictive modeling and decision-making in various domains. They are a subset of machine learning algorithms inspired by the structure and functioning of the human brain. ANNs consist of interconnected nodes, known as artificial neurons or perceptions, which process and transmit information. Each perception takes inputs, applies weights to them, and passes them through an activation function to produce an output (Hegde et al., Citation2023). By training on large datasets, ANNs can learn complex relationships and make accurate predictions. In the context of manufacturing and machining processes, ANNs have been extensively used to predict output results based on input parameters. By training on historical data and utilizing the learned patterns, ANNs can provide valuable insights into optimizing manufacturing and machining operations. In the field of machining polymer composites reinforced with natural fibers, several literature gaps persist. One notable gap is the limited focus on optimizing machining parameters specifically tailored for natural fiber-reinforced composites. While extensive research exists for conventional composites, the unique properties of natural fibers demand a specialized approach. Additionally, there is a lack of in-depth studies concerning the machinability of these composites under different environmental conditions, which is crucial for real-world applications where varying temperatures and humidity levels can impact the material behavior. Furthermore, there is a need for comprehensive investigations into the development of novel cutting tools and techniques that can minimize tool wear and improve the surface finish of natural fiber-reinforced polymer composites during machining processes. Bridging these gaps in the literature is essential for advancing the understanding of machining natural fiber-reinforced composites, enabling the efficient and sustainable utilization of these materials in various industries. Hence, this paper presents a study carried out on thrust force and torque generated, while drilling coconut coir fiber reinforced polymer composite laminates based on statistical and soft computing approach.
2. Methodology
The materials used for fabricating composite specimen are coconut coir and polymer. The coconut coir fibers () with different fiber diameter, thickness and volume percentage have been used in this experimentation ( and ). The resin used for the preparation of matrix is “Bisphenol-A based epoxy resin LY-556 and the hardener used is HY-951” (). The resin content of the composites is maintained at around 50% by weight. Compression moulding technique is used to fabricate the CCFRP composite laminates. Initially, the fibers are dried to remove moisture content in a climatic chamber at 60 °C for more than 24 h before composite processing.
Figure 1. Workpiece specimen (a) Image of coconut coir fiber (b) Image of CCFRP composite laminates (c) Microstructure of CCFRP composite laminates.

Table 1. Mechanical properties coir fiber (Hasan et al., Citation2021).
Table 2. Properties of epoxy resin and hardener (Hasan et al., Citation2021).
The drilling experiments on CCFRP composite laminates of 10 mm thickness under different spindle speeds, feed rates, point angle, fiber diameter, fiber length and fiber volume% are carried out by using AMS Vertical Machining Centre (Model: SPARK, BANGALORE) using uncoated solid carbide (K20) drills of 10 mm diameter with three different point angles of 90°, 104° and 1180. shows the drilling test parameters and levels used in the experimentation. The thrust force and the torque generated while drilling CCFRP composite laminates are measured by using a 9257 BA KISTLER Dynamometer. The signals of thrust force and torque are transmitted to KISTLER 5070 charge amplifier and recorded on a personal computer subsequently.
Table 3. Drilling test parameters and levels.
2.1. Taguchi’s design of experiments (TDOE)
To yield valid and objective conclusions an efficient procedure known as TDOE has been widely used in metal cutting operations. The Taguchi L27 orthogonal array is obtained using popular software known as MINITAB 15. Taguchi L27 orthogonal array is one of the application in design of experiments that has been widely used in manufacturing, metal cutting and engineering applications. There has been many research conducted on metal cutting operations for obtaining optimal cutting parameters (Karthik et al. Citation2022; Nian et al., Citation1999; Shetty et al., Citation2009, Citation2018, Citation2020). The S/N ratio smaller the better characteristics (EquationEquation 1[1]
[1] ) has been used as the quality characteristic in this experimentation. For smaller the better characteristics, the result obtained will be better when we consider thrust force and torque.
Smaller is the best characteristic
[1]
[1]
Where, n is the number of observations and y is the observed data.
2.2. Response surface Methodology (RSM)
Cutting industries face difficulty in analyzing “thrust force and torque” during drilling. Thrust force and torque provide most effective way of understanding the machining characteristics for any metal or alloys. RSM helps in predicting thrust force and torque generated in advance as a function of drilling conditions. RSM is an optimising tool used to create a model, analyse the problem and to determine optimum cutting conditions by combining mathematical and statistical techniques (Hegde et al., Citation2022; Shetty et al., Citation2019). The relationship between, “thrust force, torque and drilling parameters such as spindle speed, feed rate, point angle, fiber diameter, fiber length and fiber volume%” has been expressed as shown in EquationEquations 2[2]
[2] and Equation3
[3]
[3] .
[2]
[2]
[3]
[3]
2.3. Desirabilty Function Analysis (DFA)
Desirability function is a “simultaneous optimization technique” to analyze the multi response problems (Anarghya et al., Citation2018; Derringer & Ronald, Citation1980; Mishra & Basu, Citation2020). In this research, the existing values are initially transformed into a scale free value called desirability by the objective function. Further, “optimum level of parameters is evaluated by composite desirability to satisfy selected process output parameters” using MINITAB 15 software.
2.4. Artificial Neural Network (ANN)
In this study, we have utilized an MPM (Multi-Layer Perception) Artificial Neural Network to predict the results of the experimentation. The dataset consists of various process parameters, including spindle speed, feed rate, point angle, fiber length, fiber volume, fiber diameter, and corresponding measurements of thrust force and torque. The dataset is split into training and testing sets using a test size of 20% and a random state of 42. MPM ANN model () is constructed with two hidden layers, each containing 100 neurons, employing the rectified linear unit (ReLU) activation function and the Adam solver. Further, performance of the model is evaluated using metrics such as mean squared error (MSE) and mean absolute error (MAE) on the testing set. Finally, the trained model is utilized to make predictions on new data by providing a sample input of process parameters.
Steps involved in applying MPM (Multi-Layer Perception) Artificial Neural Network along with the corresponding code are provided:
Step 1: Importing the necessary libraries
′′′python
Import pandas as pd
From sklearn.model_selection import train_test_split
From sklearn.neural_network import MLPRegressor
From sklearn.metrics import mean_squared_error, mean_absolute_error
′′′
Step 2: Loading and pre-processing the dataset
′′′python
Dataset = pd.read_csv(‘dataset.csv’)
# Replace ‘your_dataset.csv’ with the actual filename
X = dataset.drop([‘Trial’, ‘Thrust force(N)’, ‘Torque(N-m)’], axis = 1) # Features
Y = dataset[[‘Thrust force(N)’, ‘Torque(N-m)’]] # Targets
′′′
Step 3: Splitting the dataset into training and testing sets
′′′python
X_train, X_test, y_train, y_test = train_test_split(X, y, test_size = 0.2, random_state = 42)
′′′
Step 4: Creating and training the MPM ANN model
′′′python
Model = MLPRegressor(hidden_layer_sizes=(100, 100), activation=’relu’, solver=’adam’, random_state = 42)
Model.fit(X_train, y_train)
′′′
Step 5: Evaluating the model
′′′python
Y_pred = model.predict(X_test)
Mse = mean_squared_error(y_test, y_pred)
Mae = mean_absolute_error(y_test, y_pred)
Print(“Mean Squared Error:”, mse)
Print(“Mean Absolute Error:”, mae)
′′′
Step 6: Making predictions using the trained model
′′′python
New_data = pd.DataFrame({‘Spindle speed(rpm)’: [2000],
‘Feed rate(mm/min)’: [25], ‘Point angle(degree)’: [90],
‘Fiber length(mm)’: [4], ‘Fiber volume(%)’: [40],
‘Fiber diameter(microns)’: [7]})
Prediction = model.predict(new_data)
Print(“Thrust force prediction:”, prediction[0][0])
Print(“Torque prediction:”, prediction[0][1])
′′′
3. Results and discussions
Analyzing the thrust force and torque generated while drilling composites has proved to be the most efficient method of comprehending the machining characteristics. Thereforethe main focus in this section has been on minimising thrust force and torque during drilling of CCFRP composite laminates while using the proper selection of drilling input variables like spindle speed, feed rate, point angle, fibre diameter, fibre length, and fibre volume% using TDOE, RSM, and DFA. MLP-ANN is applied to predict the thrust force and torque and the results are validated using experimental results.
3.1. Thrust force and torque
Thrust force and torque generated while drilling CCFRP composite laminates is strongly related to the process parameters such as, “spindle speed, feed rate, point angle, fiber diameter, fiber length and fiber volume%.” Taguchi’s main effect plot for Thrust force helps in analyzing the influence of process parameters while drilling composite laminates. The main effect plots for “thrust force and torque over spindle speed, feed rate, point angle, fiber diameter, fiber length and fiber volume%” while drilling CCFRP composite laminates are respectively shown in and . shows that the most important process variables affecting the thrust force and torque while drilling CCFRP composite laminates are spindle speed, feed, and fibre volume percentage. Point angle, fiber length and fiber diameter are the least contributing process parameters for thrust force and torque while drilling CCFRP composite laminates. From the main effect plots, it can be concluded that the spindle speed of 2000 rpm, feed of 15 mm/min, point angle of 90°, fiber length of 6 mm, fiber volume of 30% and fiber diameter of 7microns are the optimum process parameters in order to generate better thrust force and torque while drilling CCFRP composite laminates ().
Figure 3. Main Effect Plot for Thrust force (N) against (a) Spindle Speed (RPM), (b) Feed Rate (mm/min), (c) Point Angle (Degrees), (d) Fiber Length (mm), (e) Fiber Volume (%), (f) Fiber Diameter (microns).

Figure 4. Main Effect Plot for Torque (N-m) against (a) Spindle Speed (RPM), (b) Feed Rate (mm/min), (c) Point Angle (Degrees), (d) Fiber Length (mm), (e) Fiber Volume (%), (f) Fiber Diameter (microns).

Table 4. Experimental values of Thrust Force (N) and Torque (N-m).
depict the photographic and SEM images of drilled holes followed by chips formed under different spindle speeds. Highest delamination is observed with 2000 rpm spindle speed. Further, fiber pullout and breakage is maximum with high spindle speed. Long fibrous chips are formed with 1000 rpm spindle speed due to less heat generated during drilling of coconut coir reinforced polymer composite laminates.
Figure 5. Images of drilled hole under different spindle speeds (a) 1000 rpm (b) 1500 rpm (c) 2000 rpm.

Figure 6. SEM images of drilled hole under different spindle speeds. (a) 1000 rpm (b) 1500rpm (c) 2000rpm.

Figure 7. Images of chips formed under different spindle speed (a) 1000 rpm (b) 1500 rpm (c) 2000 rpm.

and , respectively, exhibit the ANOVA results for the thrust force and torque during drilling CCFRP composite laminates. The results of the ANOVA show that the most important variables influencing the thrust force and torque generated while drilling CCFRP composite laminates are spindle speed, feed rate, and fibre volume percentage, whereas point angle, fibre length, and fibre diameter have no statistical or physical significance. Further ANOVA results have indicated spindle speed (75.37% & 95.88%), feed rate (8.99% & 1.62%) and fiber volume% (8.94% & 1.65%) contributed significantly to thrust force and torque while drilling CCFRP composite laminates.
Table 5. Analysis of variance for SN ratios for Thrust force (N).
Table 6. Analysis of variance for SN ratios for Torque (N-m).
Where, (A)- Spindle speed(rpm); (B)- Feed rate(mm/min); (C)- Point angle (degree); (D)- Fiber length(mm); (E)- Fiber volume(%); (F)- Fiber diameter (microns)
Response surface approach can be used to create a second order mathematical model for metal cutting as it is a frequently used technique for identifying the best process output variables with the fewest number of trial runs.
From the analysis ( and ) carried out for the thrust force and torque, it was observed that F calculated value (4.19 and 11.23) was “greater than the F-table value (3.02)” and hence the developed model was adequate. From the second order model generated, the contour and surface plot for each of the response surfaces for different drilling conditions is plotted (). Response contours (a) and surface plot (b) help in predicting the thrust force and torque at any zone of the drilling domain. depict the thrust force and torque signals generated under different fiber volume percentages. From and it was observed that at a fiber volume of 50% the thrust force and torque are 31.23 N and 2.12 N-m. As the fiber volume was reduced to 30% the thrust force and torque gradually reduced to 22.12 N and 0.76 N-m. This may be attributed to the fact that faster penetration and lower fiber pull out decreased the thrust force and torque signal values.
Figure 8. Thrust force (a) contour plot and (b) surface plot in spindle speed- fiber volume% plane at constant feed rate, point angle, fiber length and fiber diameter.

Figure 9. Torque (a) contour plot and (b) surface plot in spindle speed- fiber volume% plane at constant feed rate, point angle, fiber length and fiber diameter.

Figure 10. Thrust force signals for (a) Fiber volume of 50% (b) Fiber volume of 40% and (c) Fiber volume of 30% CCFRP composite.

Figure 11. Torque signals for (a) Fiber volume of 50% (b) Fiber volume of 40% and (c) Fiber volume of 30% CCFRP composite.

Table 7. Analysis of variance for Thrust force (N).
Table 8. Analysis of variance for Torque (N-m).
Desirability Function Approach is an ideal technique adopted for determining the best combination of processing parameters while drilling CCFRP composite laminates. Here the goal is to minimize the thrust force and torque values. RSM optimization result for the thrust force and torque are shown in and . The best combination for minimum thrust force of 24.0042 N is obtained when spindle speed of 2000 rpm, feed of 21 mm/min, point angle of 118°, fiber length of 6 mm, fiber volume of 30% and fiber diameter of 10microns are used. Similarly, the parameters for minimum torque of 0.8001 N-m are spindle speed of 1494 rpm, feed of 17.45 mm/min, point angle of 117°, fiber length of 6 mm, fiber volume of 31.35% and fiber diameter of 6 microns.
3.2. Validation of MLP-ANN results using TDOE results
The results of the MLP-ANN prediction for thrust force and torque are presented in , and . The experimental values of thrust force and torque were compared with the corresponding values predicted by the MLP-ANN model. The predicted values closely approximated the experimental values, indicating a good performance of the MLP-ANN in analyzing the relationships between the process parameters and the output variables.
Table 9. Validation of MLP-ANN prediction results using experimental data.
The average error percentage for thrust force and torque was 1.75% and 6.56% respectively. These low error percentages suggest that the MLP-ANN model has effectively learnt and generalized the patterns in the dataset. Overall, the MLP-ANN has demonstrated its ability to accurately predict the thrust force and torque based on the given process parameters, providing valuable insights for optimizing the machining process.
4. Conclusion
The study’s conclusions highlight the critical influence of drilling parameters on CCFRP composite laminates. Optimal spindle speeds and lower feed rates reduce thrust force and torque, ensuring smoother drilling by minimizing resistance. The choice of point angle directly impacts cutting efficiency, while fiber length, volume, and diameter affect material density and obstruction encountered by the drill bit. Understanding these influences is crucial for efficient drilling processes, enabling industries like aerospace and automotive manufacturing to minimize damage, reduce tool wear, and enhance overall drilling efficiency in the fabrication of CCFRP composite components.
Through Taguchi’s Design of Experiments (TDOE), optimal drilling conditions for CCFRP composite laminates were identified: spindle speed of 2000 rpm, feed rate of 15 mm/min, 90° point angle, 6mm fiber length, 30% fiber volume, and 7-micron fiber diameter, resulting in minimized thrust force and torque.
Analysis of Variance (ANOVA) revealed significant contributions to thrust force and torque: spindle speed (75.37% & 95.88%), feed rate (8.99% & 1.62%), and fiber volume% (8.94% & 1.65%). These factors played pivotal roles in the drilling process.
Response Surface Methodology (RSM) indicated the developed model’s effectiveness, with F calculated values (4.19 and 11.23) surpassing the F-table value (3.02). Drilling efficiency improved with increased fiber volume% and decreased spindle speed, as shown by response contours and surface plots.
Desirability Function Analysis (DFA) optimization pinpointed the ideal parameters: minimum thrust force (24.0042 N) achieved at 2000 rpm spindle speed, 21 mm/min feed rate, 118o point angle, 6mm fiber length, 30% fiber volume, and 10-micron fiber diameter. Additionally, minimum torque (0.8001 N-m) was attained at 1494 rpm spindle speed, 17.45 mm/min feed rate, 117° point angle, 6mm fiber length, 31.35% fiber volume, and 6-micron fiber diameter.
MLP-ANN has successfully predicted the values of thrust force and torque with an average error percentage of 1.75% and 6.56% respectively.
5. Limitations of the research
Despite the comprehensive insights gained, this study has certain limitations. Primarily, the research is confined to a specific set of drilling parameters and may not encompass all possible combinations. Variations in material composition and tool characteristics might yield different results, requiring further exploration. Additionally, the study does not delve into the long-term effects of the drilling process on the structural integrity of CCFRP composite laminates. Moreover, external factors such as environmental conditions and tool wear, which could influence drilling outcomes, were not extensively considered. These limitations highlight the need for more extensive and diverse research to account for various factors affecting drilling processes in composite materials.
6. Future scope of the research
Advanced studies could focus on integrating artificial intelligence and machine learning algorithms to create predictive models, enabling real-time adaptation of drilling parameters based on ongoing feedback. Furthermore, investigating the impact of unconventional drilling techniques, such as laser-assisted or ultrasonic drilling, on CCFRP composite laminates could open new horizons for manufacturing processes. Collaborations with industries and interdisciplinary research involving material scientists, engineers, and environmental experts can lead to the development of eco-friendly drilling methods, ensuring sustainability in composite material processing. Additionally, expanding the scope to include a broader range of composite materials, along with in-depth analyses of post-drilling structural integrity, would be invaluable for both academic research and industrial applications. These future endeavours hold the potential to revolutionize the field of composite material machining and its industrial implementations.
Disclosure statement
No potential conflict of interest was reported by the author(s).
Additional information
Notes on contributors
Sawan Shetty
Dr. Sawan Shetty Assistant Professor from Department of Mechanical and Industrial Engineering , Manipal Institute of Technology Manipal, Dr. Shetty is a an expert in Electrospinning, Synthesis and application of nanomaterials. His major research is focused on Functional nanofibers and composite materials.
Raviraj Shetty
Dr. Raviraj Shetty is a senior Professor from Department of Mechanical and Industrial Engineering, Manipal Institute of Technology Manipal. His area of expertise are Cryogenic Lubrication, Difficult to Machine Materials, Advanced Composite Materials, Tribology in Metal Cutting, Statistical Tool for Metal Cutting, Conventional and Non-conventional Machining of Composite Materials; Soft Computing, Natural Fiber Reinforced Polymers etc. He has more than 1000 citations from above 100 publications from various reputed International Journals.
Rajesh Nayak
Dr. Rajesh Nayak is a senior Associate Professor from Department of Mechanical and Industrial Engineering , Manipal Institute of Technology Manipal. His area of expertise include Manufacturing Engineering, Composite Materials, Machining of Materials, Natural Fiber Composites, Cryogenic Machining of Elastomers.
Adithya Hegde
Adithya Hegde Research Scholar from Department of Mechanical and Industrial Engineering, Manipal Institute of Technology Manipal. His area of interest and experience include and not limited to Advanced Manufacturing Methods, Refractory Ceramic/Metal composites, Natural Fiber Reinforced Polymer Composites, Sustainable Manufacturing, Artificial Neural Networks, Lubrication Systems & Cryogenics, Elastomer Machining, Statistical Design of Experiments etc. In a short research career of 3 years at MIT, Manipal he has published 14 Peer reviewed International Journals with 50+ citations.
Uday Kumar Shetty S. V.
S. V. Uday Kumar Shetty Professor is a senior Assistant Professor from Department of Mechanical and Industrial Engineering, Manipal Institute of Technology Manipal. His area of expertise include Industrial Engineering; Engineering Management, Facility Planning and Design; Work Systems Engineering.
Sudheer M.
Dr. Sudheer M Professor, Dept of Mechanical Engineering, St Joseph Engineering College, Mangaluru. His area of expertise are Polymer Composites, Metal Matrix Composites, Finite Element Techniques.
References
- Arola, D., Sultan, M. B., & Ramulu, M. (2002). Finite element modeling of edge trimming fiber reinforced plastics. Journal of Manufacturing Science and Engineering, 124(1), 32–41., https://doi.org/10.1115/1.1413775
- Anarghya, A., Harshith, D. N., Rao, N., Nayak, N. S., Gurumurthy, B. M., Abhishek, V. N., & Patil, I. G. S. (2018). Thrust and torque force analysis in the drilling of aramid fibre-reinforced composite laminates using RSM and MLPNN-GA. Heliyon, 4(7), e00703. https://doi.org/10.1016/j.heliyon.2018.e00703
- Arputhabalan, J., S, P., K, P., S, V., & K, V. (2019). Assay of machining attributes in drilling of natural hybrid fiber reinforced polymer composite. Materials Today Proceedings, 16, 1097–1105. https://doi.org/10.1177/0731684420958103
- Chegdani, F., & El-Mansori, M. (2018). Friction scale effect in drilling natural fiber composites. Tribology International, 119, 622–630. https://hal.archives-ouvertes.fr/hal-02151230. doi: https://doi.org/10.1016/j.triboint.2017.12.006
- Debnath, K., Singh, I., & Dvivedi, A. (2017). On the analysis of force during secondary processing of natural fiber‐reinforced composite laminates. Polymer Composites, 38(1), 164–174. https://doi.org/10.1002/pc.23572
- Derringer, G., & Ronald, S. (1980). Simultaneous optimization of several response variables. Journal of Quality Technology, 12(4), 214–219. https://doi.org/10.1080/00224065.1980.11980968
- Díaz-Álvarez, A., Rubio-López, Á., Santiuste, C., & Miguélez, M. H. (2018). Experimental analysis of drilling induced damage in biocomposites. Textile Research Journal, 88(22), 2544–2558., https://doi.org/10.1177/0040517517725118
- Hasan, K. M. F., Horváth, P. G., Bak, M., & Alpár, T. (2021). A state-of-the-art review on coir fiber-reinforced biocomposites. RSC Advances, 11(18), 10548–10571. https://doi.org/10.1039/D1RA00231G
- Franke, V. (2011). Drilling of long fiber reinforced thermoplastics—influence of the cutting edge on the machining results. CIRP Annals, 60(1), 65–68. https://doi.org/10.1016/j.cirp.2011.03.078
- Gokul, K., & Rajasekaran, T. (2018). Cutting force analysis on drilling parameters of sugarcane fibre reinforced polymer composite. IOP Conference Series: Materials Science and Engineering, 402, 012183. https://doi.org/10.1088/1757-899X/402/1/012183
- Hasan, K. M. F., Horváth, P. G., Kóczán, Z., & Alpár, T. (2021). Thermo-mechanical properties of pretreated coir fiber and fibrous chips reinforced multilayered composites. Scientific Reports, 11(1), 3618. https://doi.org/10.1038/s41598-021-83140-0
- Hegde, A., Shetty, R., Chiniwar, D., Naik, N., & Nayak, M. (2022). Optimization and prediction of mechanical characteristics on vacuum sintered Ti-6Al-4V-SiCp composites using Taguchi’s design of experiments, response surface methodology and random forest regression. Journal of Composites Science, 6(11), 339. https://doi.org/10.3390/jcs6110323
- Hegde, A., Shetty, R., Naik, N., Murthy, B. R. N., Nayak, M., Kumar, M., & Shanubhogue, D. (2023). Optimization and prediction of thermal conductivity and electrical conductivity of vacuum sintered Ti-6Al-4V-SiC(15 Wt.%) using soft computing techniques. Journal of Composites Science, 7(3), 123.,; https://doi.org/10.3390/jcs7030123
- Jayabal, S., Natarajan, U., & Sekar, U. (2011). Natarajan and Sekar. The International Journal of Advanced Manufacturing Technology, 55(1-4), 263–273. https://doi.org/10.1177/0892705715575094
- Karthick, S. R., Londe, N. V., Shetty, R., Nayak, R., & Hedge, A. (2022). Optimization and prediction of hardness, wear and surface roughness on age hardened stellite 6 alloys. Manufacturing Review, 9(2), 10. https://doi.org/10.1051/mfreview/2022008
- Khashaba, U., Mohamed Abd-Elwahed, S., Mohamed Eltaher, A., Najjar, I., Melaibari, A., & Ahmed, I. K. (2021). Thermo-mechanical and delamination properties in drilling GFRP composites by various drill angles. Polymers, 13(11), 1884. https://doi.org/10.3390/polym13111884
- Kumar, J., & Kumar, V. R. (2020). Experimental investigations and multiple criteria optimization during milling of Graphene Oxide (GO) doped epoxy/CFRP composites using TOPSIS-AHP hybrid module. FME Transactions, 48(3), 628–635. https://doi.org/10.5937/fme2003628K
- Kumar, J., Abhishek, K., Xu, J., & Verma, R. K., (2021). Experimental investigation on machine-induced damages during the milling test of graphene/carbon incorporated thermoset polymer nanocomposites. Journal of Composites Science, 6(3), 77. 10.3390/jcs6030077
- Kumar, J., & Kumar, V. R. (2021). Delamination assessment during machining of laminated polymer nanocomposite. International Journal of Modern Manufacturing Technologies, 13(2), 109–115. 10.54684/ijmmt.2021.13.2.109
- Kumar, K., Kumar, J., Singh, V. K., & Verma, R. K. (2021). An integrated module for machinability evaluation and correlated response optimization during milling of carbon nanotube/glass fiber modified polymer composites. Multiscale and Multidisciplinary Modeling, Experiments and Design, 4(4), 303–318., 101007/s41939-021-00099-1
- Kumar, J., Verma, R. K., Mondal, A. K., & Singh, V. K. (2021). A hybrid optimization technique to control the machining performance of graphene/carbon/polymer (epoxy) nanocomposites. Polymers and Polymer Composites, 29(9_suppl), S1168–S1180. https://doi.org/10.1177/09673911211046789
- Kumar, D. R., Varma, P. S. S. K., & Rao, B. N. (2017). Optimum drilling parameters of coir fiber-reinforced polyester composites. American Journal of Mechanical and Industrial Engineering, 2, 92. https://doi.org/10.11648/j.ajmie.20170202.15
- Mohan, N. S., Ramachandra, A., & Kulkarni, S. M. (2005). Machining of fiber-reinforced thermoplastics: Influence of feed and drill size on thrust force and torque during drilling. Journal of Reinforced Plastics and Composites, 24(12), 1247–1257. https://doi.org/10.1177/0731684405049865
- Mishra, L., & Basu, G. (2020). Coconut fibre: Its structure, properties and applications, handbook of natural fibres. India: Woodhead Publishing Series in Textiles. https://doi.org/10.1016/B978-0-12-818398-4.00010-4
- Murthy, B. R. N., Rodrigues, R. L. L., Sharma, Y. N., & Anjaiah, D. (2010). IEEE Proceedings of 2nd International Conference on Mechanical and Electrical Technology (ICMET) (pp. 87–90). IEE Proceedings.
- Nasir, A. I., Azmi, T., Lih, C., & Majid, M. S. A. (2019). Critical thrust force and critical feed rate in drilling flax fibre composites: A comparative study of various thrust force models. Composites Part B Engineering, 165, 222–232. https://doi.org/10.1177/0731684420958103
- Nian, C. Y., Yang, W. H., & Tarng, Y. S. (1999). Optimization of turning operations with multiple performance characteristics. Journal of Materials Processing Technology, 95(1–3), 90–96. https://doi.org/10.1007/s00170-003-1672-4
- Phadnis, V., Makhdum, F., Roy, R., & Silberschmidt, V. V. (2013). Drilling in carbon/epoxy composites: Experimental investigations and finite element implementation. Composites Part A: Applied Science and Manufacturing, 47, 41–51. https://doi.org/10.1016/j.compositesa.2012.11.020
- Pramanik, A. l., Zhang, L. C., & Arsecularatne, J. A. (2006). Prediction of cutting forces in machining of metal matrix composites. International Journal of Machine Tools and Manufacture, 46(14), 1795–1803. https://doi.org/10.1016/j.ijmachtools.2005.11.012
- Rajmohan, T., & Palanikumar, K. (2012). Optimization of machining parameters for surface roughness and burr height in drilling hybrid composites. Materials and Manufacturing Processes, 27(3), 320–328. https://doi.org/10.1007/978-3-030-04290-5_8
- Rezghi Maleki, H., Hamedi, M., Kubouchi, M., & Arao, Y. (2019). Experimental investigation on drilling of natural flax fiber-reinforced composites. Materials and Manufacturing Processes, 34(3), 283–292. https://doi.org/10.1080/10426914.2018.1532584
- Sapuan, S. M., Tamrin, K. F., Nukman, Y., El-Shekeil, Y. A., Hussin, M. S. A., & Aziz, S. N. A. (2016). Natural Fiber-Reinforced Composites: Types, Development, Manufacturing Process, and Measurement. Comprehensive materials finishing, 203. https://doi.org/10.1016/B978-0-12-803581-8.09183-9
- Shetty, R., Pai, R., Barboza, A. B. V., & Gandhi, V. P. (2018). Processing, mechanical charaterization and its tribological study of discontinously reinforced Caryota Urens Fibre Polyester composites. ARPN Journal of Engineering and Applied Sciences, 13, 3920–3928.
- Shetty, R., Barboza, A. B. V., & Nayak, R. (2019). Mechanical and machinability study on discontinuously reinforced sisal fibre polyester composite laminates. Materials Research Express, 6(10), 105370. https://doi.org/10.1088/2053-1591/ab366
- Shetty, R., Pai, R., Rao, S., Nayak, R., & Braz, J. (2009). Society of Mechanical Sciences and Engineering, 31(1), 12–20. https://doi.org/10.1590/S1678-58782009000100003
- Shetty, R., Kumar, S., Mallagi, R., & Keni, L. (2020). L 16 orthogonal array-based three-dimensional finite element modeling for cutting force and chip formation analysis during dry machining of Ti–6Al–4V. Journal of Advanced Manufacturing Systems, 20(01), 123–134. https://doi.org/10.1142/S0219686721500074
- Singh, I., & Bhatnagar, N. (2006). Drilling of uni-directional glass fiber reinforced plastic (UD-GFRP) composite laminates. The International Journal of Advanced Manufacturing Technology, 27(9-10), 870–876. https://doi.org/10.1007/s00170-004-2280-7
- Singh, Y., Singh, J., Sharma, S., Lam, T.-D., & Nguyen, D.-N. (2020). Fabrication and characterization of coir/carbon-fiber reinforced epoxy based hybrid composite for helmet shells and sports-good applications: influence of fiber surface modifications on the mechanical, thermal and morphological properties. J. Materials Research Technology, 9(6), 15593–15603. https://doi.org/10.1016/j.jmrt.2020.11.023
- Valarmathi, T. N., Palanikumar, K., & Latha, B. (2013). Measurement and analysis of thrust force in drilling of particle board (PB) composite panels. Measurement, 46(3), 1220–1230. https://doi.org/10.1016/j.measurement.2012.11.024