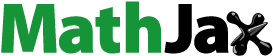
Abstract
In recent times, there has been a growing focus on multi-micro-grids (MMGs) system, owing to its well-suited structures for efficiently accommodating large-scale integration of distributed energy resources (DER). This attention is driven by the system’s cost-effectiveness, enhanced efficiency, stability, and reliability performance, achieved through the collaborative exchange of power flow among individual micro-grids (MGs) and the main grid. Fundamental strategies for attaining optimal energy flow and sharing involve the optimal sizing of MGs and the implementation of an Energy Management System (EMS). These strategies play a crucial role in addressing uncertainties associated with intermittent generation, load fluctuations and energy market dynamics. This paper offers a review of grid-connected MMG topologies, EMS structures, coordination methods and current optimization approaches designed to meet EMS objectives. To address the inherent volatilities, the paper introduces various uncertainty quantification techniques along with current challenges. Additionally, it suggests future directions, emphasizing intelligent and predictive modeling to handle uncertainties, as well as recommending the incorporation of energy storage systems (ESSs) to align with emerging trends.
REVIEWING EDITOR:
1. Introduction
In recent years, energy crisis, power loss and environmental pollutions due to the static fossil fuel based generations led to the increasing penetration of distributed energy resources (DERs) based microgrid (MG) (Babatunde et al., Citation2020). MG can be defined as a self-sufficient low-voltage power distribution system comprised of different distributed generation (DG), energy storage system (ESS) such as battery energy storage system (BESS), flywheels, pumped hydro, fuel cell (FC), energy capacitors, etc., controllable loads which can be operated in the grid-connected or islanded mode (Zhou et al., Citation2021). The recent advancements on power electronics, smart measurement and communications in MGs promote networked operation in multi-microgrid (MMG). MMG systems refer to the interconnection of adjacent microgrids for seamless operation in terms of energy management with the main grid or between microgrids (Zou et al., Citation2019). The MMG has numerous benefits such as the reduction of investment and operating costs of DER, reduction of transmission and distribution losses, customer satisfaction, environmental benefits, etc. while it’s connected to the grid. For the stable and economic operations of grid-connected MMGs, a MMGs energy management system (MMGEMS) is mandatory to plan, manage and coordinate DERs, dynamic load demand, sate of charge of the BESS and the energy trading among MGs and the main grid for maintaining stochastic nature of generations of DERs and load demands balances (Muqeet et al., Citation2022; Castellanos et al., Citation2023).
The MMGEMS manages the intermittency of renewable energy sources (RES) and dynamic load demand, based on formulated objective functions, in order to optimize the overall grid connected operation of the system in terms of levelized cost of energy, reliability and availability, emission cost, lifespan of DERs, and other objectives by fulfilling related system constraints such as grid losses, active and reactive power generation limits, BESS capacity, grid and MMG energy exchanges through feed-in-tariff (FIT) agreement, and many others (Ouramdane et al., Citation2021). Before the energy management strategy is done in these sophisticated MMG systems, optimal sizing of each DER component should be evaluated based on the criteria such as the energy balance between generation and load demand, availability, periodic generation limits, economical aspects, etc. Hence, the execs energy will be stored in the BESS and FC energization and the rest will be curtailed using the optimization technique to be cost-effective in the planning aspects of DER (Copp et al., Citation2022; Babatunde et al., Citation2019).
BESS is vital for large-scale RES based grid integrated systems for peak shaving and cost minimization of the MGs by storing the excess energy during off-peak periods. In addition to this BESS can help the system by overcoming the intermittency and support the main grid. Scheduled MMG networks are claimed in improving energy utilization efficiency, minimizing operational cost of an MMG network and maximizing reliability of the system by considering operation constraints and emissions through optimal energy management system (EMS) (Zhong et al., Citation2022; Bazmohammadi et al., Citation2020; Roy et al., Citation2020).
In MMGs there are two common scheduling optimization structures; centralized and distributed/ decentralized EMS or controllers. Based on (Qiu & You, Citation2020) centralized controllers in MMG gathers detail information from energy generation, consumption, and storage of each microgrid and uses this information to make real-time decisions based on the optimization algorithm on how to allocate energy resources across the different microgrids. It can also forecast energy demand and supply for each microgrid and schedule the operation of different energy assets to optimize overall energy efficiency (Alam & Arefifar, Citation2019). This method of controlling has an advantage of improved reliability and stability, increased efficiency, reduced energy costs, and better utilization of renewable energy resources. Centralized EMS can also allow operators to monitor and manage the entire system from a centralized location, enabling them to quickly respond to changes in energy demand and supply (Yang et al., Citation2019). Therefore, in this method coordination can be maintained easily, but it needs complex communication and higher computational complexity and stakeholders may not willing to provide their information due to the privacy issues. A single point of failure may impact the entire system. Due to these drawbacks, centralized EMS controllers are not common in MMG systems (Naderi et al., Citation2023; Nawaz et al., Citation2022).
In a decentralized system, each microgrid is responsible for managing its own energy resources, with limited coordination and control from a central entity. The microgrids can communicate with each other and share information about their energy resources, but they operate independently and make decisions based on local conditions (Nawaz et al., Citation2022; Guo et al., Citation2021; Asimakopoulou et al., Citation2013; Mao et al., Citation2017; Mansour-Saatloo et al., Citation2021). Distributed energy management optimization method is critical for networked MMGs to increase resiliency, increase flexibility and enhanced security (Zhou et al., Citation2019; Sheikhahmadi et al., Citation2020). However, it may not be suitable for all situations and requires careful consideration of the trade-offs involved due to the limitations related to limited coordination and local optimization issues. These EMS policies are discussed in section 5 in detail. The optimization techniques used in distributed EMS are also described well in the sections below for comparison and showing directions for future trends.
2. Previous reviews
In this focus area to contribute to the body of knowledge with respect to energy management optimization for MMG systems, various technical reviews and surveys have been conducted in the last few years. Yimy et al., presented a review on EMS of RES based MGs mainly on the comparative analysis of different optimization approaches with the formulation methods of objective functions, constraints, solutions, and simulation tools used in both the interconnected and isolated MGs (García Vera et al., Citation2019). EMS optimizations has been surveyed based on capability and efficiency to solve the mono-objective and multi-objective problems. To mitigate the intermittency of RES, the review recommends the energy storage technologies (Li et al., Citation2019; Li et al., Citation2021).
Okhuegbe et al. (Okhuegbe et al., Citation2022) reviewed the optimization algorithms used in optimal sizing of DERs which is highly complex when considering multiple DERs and also considering various objectives such as levelized cost of energy (LCOE), emission, reliability and related constraints etc.
Ref (Nawaz et al., Citation2022) reviews MMG different contemporary architecture, the prevalent EMS schemes adopted in MMG system, their topologies and functionalities and also overview the different techniques of demand-side management techniques. This review also incorporated coordination schemes in energy transaction and resilience operations in MMGs with suggested directions for future research trends. Due to the variability and uncertainties of RES impose notable seamless operation issues, MMG with coordinated control, provide ancillary services, market participation and communication (Villanueva-Rosario et al., Citation2022). This review also indicates the uncertainties due to the intermittency of RES and coordinated service management among microgrids in MMG. Review about MG clustering claimed that the current smart distribution issues such as power sharing, coordination reliability and stability challenges can be overcome through efficient scheduling of MMG (Ediriweera & Lidula, Citation2022; Kulasekera et al., Citation2011; Zubidi et al., Citation2023). The authors also indicate the methods of MG protection and stability enhancements under different operating conditions subjected to flexible RES and non-dispatchable distributed generators needs more consideration and more research needs to be carried out. Bandeiras et al. (Bandeiras et al., Citation2020) review the different EMS and control schemes of MG clusters to secure the seamless operation and cost-effectiveness of the system and proposed the protection schemes in MGs and data privacy and cybersecurity are indicated as future works. The paper also compared different energy market designs applied in MMG to optimize and facilitate the energy trade among MGs and the main grid. But in this case uncertainties of load demand, RES generations and BESS operations are not considered. Authors in (Spiegel et al., Citation2020; Aghdam et al., Citation2018; Ahmadi & Rezaei, Citation2020; Alhasnawi & Jasim, Citation2020; Du & Li, Citation2020; Moghimi et al., Citation2018; Arefifar et al., Citation2016) reviews and assess the practicality of current approaches in MMG operation, control and protection.
Although the aforementioned reviews have covered the vast range of modern-day issues on the optimal sizing of DER and EMS approaches of MMGs, a state-of-the-art survey that combines the optimal sizing of DERs and other components to reduce curtailment burden of the system, suitable approaches and clear future trends in MMG scheduling with uncertainties, and coordination are yet to be reported. Furthermore, the MMG scheduling and effectiveness of approaches is under research for more impacted research that can be implemented in the real system scenarios. This study then bridges the aforementioned knowledge gap by presenting a review on different topologies, optimization structures and approaches surrounding EMS of MMG with challenges and prospects for future implementation.
3. MMG current trend and topology
In this paper, we present an up-to-date review of the optimal sizing and energy management strategies of grid-connected multi-microgrids to foster development of MMG scheduling and practicality of smart microgrids at large-scale integration to the transmission and distribution systems. This paper mainly deals with the optimal sizing of DERs and MMG scheduling optimization structures and approaches in the development of smart microgrid. The remaining of this paper is organized as follows. The overview of MMG components and topology presented in this Section. In Section 4 problem formulation of optimal DER components and EMS for MMGs are discussed. The EMS optimization structures used in MMGs are presented in Section 5. Section 6 provides review of optimization approaches implemented in the area of grid-connected MMGs with different perspectives. In Section 7, challenges and future directions are presented. Finally, conclusion is drawn in Section 8.
3.1. Systematic review
A systematic review is a rigorous assessment of all recent and high quality published existing literature to address the pre-formulated research questions. This review uses explicit methods to systematically identify, select, evaluate and synthesize all relevant original scientific studies and gray literature pertinent to the specific research problem (Page et al., Citation2021). A good systematic review requires a comprehensive literature survey protocol with sound literature searching strategy using eligible criteria for inclusion and exclusion of contender studies (Page et al., Citation2021). Then based on (Page et al., Citation2021) eligible studies and literature are then filtered and screened for relevance and quality.
After the comprehensive literature search, 436 potentially relevant papers were accessed and retrieved from online databases namely Google Scholar, IEEE, MDPI, Scopus and other documents outside of aforementioned directories. Duplicates and unrelated works led to the exclusion of 123 at first stage. In the second stage, the abstracts of the remaining 313 papers were read and evaluated based on inclusion and exclusion criteria. As a result, 104 of the 313 papers were excluded after reading the abstracts and methodology focuses that did not meet the inclusion criteria. Full-text evaluation was carried out for the remaining 209 records, and 79 more are excluded based on the criteria of limited relevance poor quality and unreliability of data. Finally, 130 were selected for the systematic literature survey based on relevance and methodology used. show the searching and filtering of collected data.
Figure 1. Literature survey methodology procedure based on (Page et al., Citation2021).

3.2. MMG components and topology
MMG are consisting of DER such as PV, wind turbine, fuel cells, diesel generator sets, energy storage systems (ESS), different converters, local and central controllers and distribution network operators (DNO), controlled loads, etc. the individual MGs can exchange power and information through the lines. The DNO controls the power flow and energy market such as buying and selling energy to the grid and among MGs, and alleviating the likely system congestion for energy trade in the whole interconnected system (Saha et al., Citation2023; Zhao et al., Citation2022). The four common types of MMG topologies are illustrated and discussed in below.
Radial Topology: In this architecture, the individual MGs are directly connected to the main bus and the energy exchange is executed through the main grid connection as shown in (a). Each MG can respond to the decision of its own EMS with the DNO but the energy exchange is mainly relied on the dedicated power lines. Example, if one of the MGs is in power deficiency, it can purchase power from the main grid through the power exchange bus to satisfy its demand (Nikmehr & Ravadanegh, Citation2015). On the other hand, an MG can sell its surplus power for those in need to the main grid exchange through the bus (Zou et al., Citation2019).
Daisy-Chain Structure: In this topology, each MGs are connected to the main grid and to two adjacent MGs for power and information exchange (Zou et al., Citation2019; Du et al., Citation2018). As shown in (b), each microgrid can exchanges information and power with the main grid, two adjucent MGs and the DNO which enables collaboration among microgrids. In this structure, MGs can trade with nonboring MG based on peer-to-peer (P2P) communication to secure the user’s privacy (Jafari et al., Citation2020; Wang et al., Citation2018; Xie et al., Citation2018; Backhaus et al., Citation2016). It’s more reliable than radial structure as compared to radial interconnections due to the direct energy exchange of adjacent MGs (Saha et al., Citation2023; Hussain et al., Citation2018).
Interconnected MMGS with distribution feeders: in this configuration, the main grid is connected to end point MGs and each MGs are connected between its adjacent MGs (Saha et al., Citation2023) as shown in (a). The EMS optimization is a bit complex in this structure as the energy transferred from both neighboring MG and the main grid (Tian et al., Citation2016). The DNO operations in these MMGs are strongly coupled, and their energy schedules are more connected. And these MMG architectures are more reliable than the radial configuration as each MGs has more possibilities for power exchange during disturbances and calamities (Yang et al., Citation2019).
Mesh Topology: the interconnected MMGs are connected in electricity and information which enables them to enhance the overall efficiency and reduced operating cost (Zou et al., Citation2019; Saha et al., Citation2023). On contrary, this topology is more complex than the aforementioned structures in terms of energy collaboration and coordination as shown in (b). Each MG is not only influenced by its local controller but also other MG demand and supply scheduling (Ouammi et al., Citation2015). It’s suitable for more enhanced interaction and coordination operations, but the EMS optimization algorithm will definitely become more complicated and challenging.
4. Problem formulation
4.1. Optimal sizing of DERs
Economic aspects are prioritized in MG planning in order to address long-term operations. Optimal siting and sizing of DERs aims to cost-effectiveness of the overall system and technical feasibility while deploying the DER components which conventionally known as economic load dispatch as it aims to minimization of the total cost. According to research works in (Zhao et al., Citation2014; Chen et al., Citation2012; Cao et al., Citation2020), the MG planning problems include the following problems such as DER mix selection and siting to minimize the net present cost of the investment and power loss minimization in the deployment, and scheduling to minimize the operating cost (Gamarra & Guerrero, Citation2015). Integrating large-scale RERs to the grid requires a BESS to overcome the volatility of the RESs and peak shaving in order to stabilize the network by storing energy during off-peak periods and supplying in peak hour times. The objective problems may vary as the DERs type and the need of the consumer. For instance, most of the Multi objective (MO) problems in optimal sizing of MGs are concerned in minimization of loss of power supply probability (LPSP), loss of power supply (LPS), deficiency of power supply probability (DPSP), cost of energy (COE), and maximizing renewable factor (RF). These objective problems can be formulated according to the DER mix and grid power transfer and tariff, and the network structures.
A. Costs: The operating costs include the costs of generating electricity from the WTs and PV panels, the costs of the battery charging (discharging), the costs of the diesel generator, and the costs of the electricity exchange second between the HMS and macrogrid. The costs can be expressed as follows (Waqar et al., Citation2019).
(1)
(1)
B. Reliability: The LPSP is defined for a situation in which the load is so large that the amount of electricity produced and purchased is insufficient to satisfy the demand. This situation is shown in EquationEq. 2(2)
(2) (Waqar et al., Citation2019).
(2)
(2)
C. Renewable Factor: The RF is defined as the percentage of power generated from renewable sources compared to the power generated from non-renewable sources such as diesel generator sets, microturbines, etc, and is expressed as Equationequation 3(3)
(3) (Bazmohammadi et al., Citation2019).
(3)
(3)
D. Power balance: When the microgrid is connected to the macrogrid, increasing the self-producing and selling ability within the HMS in each time period is beneficial for the effective operation of the system. We achieve this by controlling the minimum variance of the difference between the load and the power generated by the WTs and the PV panels. The function is shown in Equationequation 4(4)
(4) (Hossain et al., Citation2019):
(4)
(4)
E. Constraints: Each distributed power supply should have a certain initial range according to the actual situation as shown in EquationEquations (5–9) (Hossain et al., Citation2019).
(5)
(5)
(6)
(6)
(7)
(7)
(8)
(8)
(9)
(9)
In planning of MG, DERs are sized, sited and configured using SO and MO problem solving approaches as summarized in .
Table 1. SO and MO problems in MG optimal sizing.
4.2. EMS objectives in MMGs
In formulation of energy management problem for MMGs, the sizing of DERs in each MG is important to customize the objective function. Hence, it can be single objective (SO) or MO problem with other implicit scheduling methods as shown in Equationequation 10(10)
(10) .
(10)
(10)
Operating Cost: the objective function of a specific problem in (Elkazaz et al., Citation2020) shows the minimization of the operating cost of the grid-connected MG as show in Equationequation 1
(1)
(1) .
Pollution Emission: The power and the emission depend on the kind of element, example MT, DG, and FC can be examined in Equationequation 11
(11)
(11) (Mahmoud et al., Citation2022).
(11)
(11)
EquationEquation 11(11)
(11) can be expanded as Equationequation 12
(12)
(12) .
(12)
(12)
Where is the emission cost of the component,
is the emission level,
is the emission factor.
Constraints: the EMS of MGs can have equality and inequality constraints such as power balance, energy trading variables and DER convenient generation limits as shown EquationEquations (13)–(18) (Elkazaz et al., Citation2020).
(13)
(13)
(14)
(14)
(15)
(15)
(16)
(16)
(17)
(17)
(18)
(18)
Some of SO and MO based EMS problems implemented on MG environment are summarized in .
Table 2. SO and MO problems in MGEMS.
5. EMS structures in MMGs
EMS in MMG is responsible to manage the generation and load demand and dispatching DERs and control other MG components. In grid connected mode, EMS of MMG works to maximize the benefits of each MG by enhancing the utilization of ancillary services. While in islanded operation, the EMS aims to improve the resilience, reliability and security during disturbances. There are different EMS strategies implemented in MMG functionality from many sources of literature. deals with the components and parameters to be considered in the MG energy management structures.
5.1. Centralized EMS
In centralized MMG controller, detailed global information is collected from each individual MG and DNO, then after analyzing the data the decision is made through optimization algorithms as shown in (a) (Nawaz et al., Citation2022; Sun et al., Citation2020). Since the centralized EMS is a single owner, it’s only useful for standalone MGs (Moghimi et al., Citation2018). Nevertheless, this strategy has a drawback of high computational burden and a single point of failure lead to no coordination at all (Bustos et al., Citation2023).
5.2. Decentralized EMS
In decentralized EMS approach, every individual MGs schedules its own activities through local controllers to maximize techno-economic benefits as an autonomous entity (Mao et al., Citation2017; Afrakhte & Bayat, Citation2020) as shown in (b). Each individual MG can exchange/ trade power directly from the main grid and has no communication to the neighboring MG which reduces the computational complexity burden (Harmouch et al., Citation2018). In this topology, each MG privacy is secured but there is no means to know whether the neighboring MG has surplus/ deficit power (Zhou & Erol-Kantarci, Citation2020). The structure has an advantage of islanded mode operation and it’s suitable for grid-connected MMG different owners of MG. Multi-Agent Soft Actor-Critical Algorithm (MASAC) is a framework for multi-agent centralized training and distributed execution, designed to deal with environmental uncertainty and find the best trading strategy for MMG scheduling, to facilitate energy transactions between different entities and to improve the operational economics of the MMG system (Gao et al., Citation2023). The federated multi-agent deep reinforcement learning (F-MADRL) is claimed for the EMS of MMG with a decentralized structure to control and maintain the dispatchability of each DGs in the MG. The proposed F-MADRL is effective for privacy protection and security by adopting self-training of agents thereby the cost is minimized and enhanced self-energy sufficiency (Li et al., Citation2023).
5.3. Hybrid EMS
The shortcoming of centralized and decentralized EMS can be mitigated using hybrid EMS by considering their strength (Gong et al., Citation2022). As (a) shows each MG can schedule its local activities using local controller to optimize the local resources only, but the surplus/ deficit energy information exchange through central EMS. Many research works have been proposed (Liu et al., Citation2017; Karimi et al., Citation2021; Babanezhaad Shirdar & Ghafouri, Citation2022) hybrid EMS to facilitate the MMG scheduling. However, due to the parallel operation of the local and central EMS, the operation cost is only depend on load demand situations. When a single MG gets disconnected from the grid it can operate autonomous but the performance will be reduced from that of the shared efficiency.
5.4. Distributed EMS
In distributed EMSs, each MG has local EMS and can communicate with other MGs through communication lines as shown in (b). In this method, time complexity burden is less than the centralized EMS due the distributed and less information transfer (Nawaz et al., Citation2023; Ceja-Espinosa et al., Citation2022). Each MG can dispatch the resources locally and communicate with neighboring MGs with limited information exchange. Hence the EMS computation of one MG will affect the other which is the current challenge in MMG scheduling (Köbrich et al., Citation2022). As recent works showed, multi-agent, game theory, and IOT based control schemes are implementing and recommended for optimal scheduling of grid-connected MMGs (Mahmoud et al., Citation2021; Alseyat et al., Citation2021). These EMS structures are highly recommended for large scale MMG networks due to lower time computational complexity.
5.5. Nested EMS
The nested EMS employs a hierarchical structure with a several levels as shown in . Each bottom MG has its own local controller to optimize local resources and responsible to its EMS status to the next upper-level MG controller (Gong et al., Citation2022). In nested structure, the privacy of each MG and consumers are secured because of the multiple layered privacy structure. The EMS of a top level can get the information of surplus/ deficit energy of MGs of all lower levels through the layered information interaction (Lin et al., Citation2018). The topmost MG considers the total amount of surplus/deficit energy as the energy resources/loads. Therefore, topmost EMS level schedules the whole MMG system, and also calculates the energy trading and exchanges with the main DNO by considering several security constraints (Mehraban & Eslami, Citation2023; Hao et al., Citation2020; Ghaemi et al., Citation2019). The upper-level EMS optimizes the local resources using this information which able to reduce the operation cost of the system. The challenge in this structure is optimal operation of MMGs due to the complex layered information sequences by considering uncertainties. If a single MG is disconnected from the network and goes to an islanded mode, still its resiliency can be improved via sub-grouping (Islam et al., Citation2021).
6. Optimization approaches used in MMG EMS
Many researches have been used various solution algorithms for solving energy management problems to achieve efficient and optimal operation of MMGs with different scenarios. These strategies are used to schedule DERs, and loads, minimize system operational costs, losses and outages, controls RES intermittency and volatility, and maintaining RES penetration factors, forecast generation, load demand, energy storage system status, energy prices, etc as shown in . The following subsections are going to describe these strategies and solution algorithms after an extensive critical review of recent research works.
6.1. Classical approach based EMS
Classical optimization approaches are generally mathematical techniques used to solve both linear and non-linear problems with small space and action EMS in MG planning and operation. Seyed et. al (Mirbarati et al., Citation2022) proposed MILP approach to minimize the daily energy production cost. As penetration of RES increase, the energy cost is minimized linearly. All variable parameters such as energy price, wind and solar input data, and demand are predicted well with the help of Long-Short Term Memory (LSTM) neural networks to handle the uncertainties in RES. MILP has successfully reduced the operating cost of clustered MGs with the presence of storage battery (Babanezhaad Shirdar & Ghafouri, Citation2022). Fuzzy logic system is used to predict the load demand and RES generation for effective scheduling. Hamed and Alizera (Babanezhaad Shirdar & Ghafouri, Citation2022) present MILP based EMS to minimize the daily operating cost using a multi-layer system. As activating multiple layers in the execution of EMS, operating cost of the entire system will be reduced. DER based MMG has been presented in (Ahmadi & Rezaei, Citation2020) in which MILP algorithm was responsible for bi-level EMS aimed in information and power exchange from the grid, and energy scheduling after isolation of MGs by considering uncertainties and demand response programs.
6.2. Metaheuristic approach based EMS
Nondominated sorting genetic algorithm II (NSGA-II) based dynamic networking model has developed which successfully deduced the daily operating cost of networked MGs with graph theory (Ge et al., Citation2021). And the work also improved the reliability of the network in terms of both convergence and runtime in different scenarios. Ref. (Kampelis et al., Citation2018) presents ANN based short-term load demand forecasting and MOGA optimization for solving alternative day-ahead demand response solutions. This approach also examines the reduction of energy price and load shifting possibilities. But the article has not considered the RES and other volatile sources which really challenges the EMS uncertainties handling (Zhang et al., Citation2023). Haddadian and Noroozian (Haddadian & Noroozian, Citation2019) proposed network of MGs which is determined using technical indices such as voltage profile, supply-adequacy, efficiency and reliability by considering demand response (DR) energy transaction costs. NSGA-II approach was proposed to the MO indices MMGs problems, and capital cost has been minimized by accounting detailed DR program (Dey et al., Citation2023; Dey et al., Citation2023). Planning and operating MMG needs careful consideration and handling of uncertainties in order to be cost-effective and realistic (Mohamed et al., Citation2021; Ali et al., Citation2022).
In (Javidsharifi et al., Citation2022) the variable RES and load parameters are forecasted and analyzed using deterministic solver without uncertainty and compared with uncertainty of parameters using UT-MJAYA approach where the maximum profit has been reduced and the environmental pollutions has also increased when uncertainties are considered using stochastic optimization. Oppositional Gradient-based Grey Wolf Optimizer (OGGWO) (Rajagopalan et al., Citation2022) is proposed in grid-connected MMG to minimize operating cost and environmental pollution. This approach has compared with other metaheuristic methods like PSO, GWO, CSA, and GGWO and it’s claimed that the OGGWO is capable of eliminating premature convergence. A Snake optimizer has been proposed to solve the EM problem in cooperated MMG by considering DR program (Alamir et al., Citation2022; Wynn et al., Citation2022). The algorithm has been compared with conventional PSO and it has better performance especially when it considers the DR uncertainty. PSO is proposed for optimal EMS of MMG in effective power scheduling and energy trading and the outcomes are compared with GA results (Pisei et al., Citation2017). And it’s clearly seen that the PSO has better performance over GA for minimizing the operating cost of the system over a 24-hour basis.
6.3. Artificial intelligence based EMS
In this section soft-computing and machine learning related AI techniques applied in EMS of MMG are reviewed. Yan et al. (Du & Li, Citation2020) proposed deep neural network (DNN) and model-free reinforcement learning techniques based EMS to maximize the MMG/ DNO profit through energy trading and decrease the demand side peak-to-average ration through peak shaving storage system. Here the privacy of individual MG is protected with the help of DNN without direct access of MG’s information. DNN and Monte Carlo are compared as DNN is powerful in extracting data and Monte Carlo has the ability of strong data exploration problems but has high time complex burden. Hence, it is recommended to apply hybrid of two for better performance. Deep convolutional neural network (CNN) has proposed for estimating daily operating cost of various interconnected MGs (Samuel et al., Citation2020). It has effectively reduced the operating cost and compared with MPC, greedy policy method and ADP which was superior in cost reduction and relatively has low computational burden. Microgrids Energy Trading Bayesian Game (METBG) is proposed to coordinate MGs and maximize profit including the stochastic nature of plug in EVs (Yu et al., Citation2020). The effectiveness of the method is verified in terms of buyers and sellers profit, the utilities of buyers, and the net energy usage of MG is has been derived through game equilibrium (Keshta et al., Citation2019). Authors in (Wu et al., Citation2022) proposed multi-stakeholder benefit optimization method based on Stackelberg game theory (SGT) to model local energy transaction to maximize the profit of MMG system to improve the autonomy of MGs (Gao et al., Citation2023; Guo & Gong, Citation2023). But the proposed approach does not consider the uncertainties in RES, load demand and demand side management.
6.4. Stochastic and Robust control based EMS
A multi-microgrid scenario involves several microgrids that are connected or running close by. These microgrids’ operation coordination becomes more difficult as a result of growing uncertainties, such as fluctuating renewable energy production and load requirements. Robust optimization offers a paradigm for systematically managing uncertainties and variances (Zhou et al., Citation2021; Yang & Su, Citation2021; Tan et al., Citation2022). A Robust Data Predictive Control framework for Energy Management System (RDPC-EMS)is proposed to overcome the uncertainty of retail electricity price transaction and minimization of operating cost of MMG (Brahmia et al., Citation2021). Distributed Model Predictive Control (DMPC) optimizes electricity forecasting and energy scheduling between DNO and cooperative MGs in the first layer to minimize the daily operating cost based on the forecasted energy price. In the second layer, by having the data from first layer, supply-demand balance through DER prediction (Karimi et al., Citation2021). A two stage adaptive robust optimization is developed in (Tan et al., Citation2022; Zhang et al., Citation2018) based on the collaborative operation of MMG with PV worst uncertainties and the model was effective not only in operating cost reduction but also it has maintained frequent energy interactions among MGs.
But the papers have a limitation of not considering dynamic tariff structure of electricity for more effective scheduling of MMGs. Instead of seeking individual benefits, a contradictory multi-objective goal programing has proposed to maximize the collective benefits of MMGs in trading and dispatching DERs in the entire system (Choobineh & Mohagheghi, Citation2019). Chance-constrained programming (CCP) has been proposed for the optimization of degradation cost of ESSs (Aghdam et al., Citation2020). The outcome of this study claims that the decreasing confidence level in CCP decreases the system operational costs and enhance the lifespan of batteries, but it threatens the system stability of the network during other disturbances and uncertainties. A receding horizon data-driven chance constrained energy flexibility trading framework has developed for MMGs flexible energy trading with the help of Nash Equilibrium (NE) data extraction method (Bagheri et al., Citation2021).
6.5. Other methods
Author in (Hu et al., Citation2018) present the MPC to reduce the computation complexity burden in MMGEMS based on predictive algorithm and compared with conventional PSO. It claims that the convergence spend is faster than PSO using decomposition and coordination approaches to achieve the power balance of DERs. But the study didn’t consider the stochastic nature of generations, load demand and energy price adopted in networked MGs. Due to the low-inertia and high intermittency of resource in clustered MGs, the frequency security should be considered with EMS problems (Mazidi et al., Citation2020). A hierarchical EMS is proposed for islanded MMGs to minimize the total operating cost with levels of controls and uncertainties to maintain frequency simultaneously (Moghimi et al., Citation2018). Convex optimization based multi-objective optimization EMS problem is considered in MMG scheduling of sharing the power from groups of MGs based on priority approach to reduce the electricity cost (Sandgani & Sirouspour, Citation2018).
This review reveals the performance and comparison of different types of optimization algorithms from classical ones to the complex huge data driven and efficient AI and machine learning methodologies on the study of MMGEMS. A critical analysis of MMG energy management approaches/ algorithms are summarized in . However, many more works are still required on the large-scale RES based MMGs integration, efficient scheduling of DERs, a day-ahead generation/ load management, alleviating environmental pollutions, uncertainty management, demand side management, power quality, reliability and protection, customer privacy issues, etc (Zia et al., Citation2018).
Table 3. EMS optimization algorithms in MMG reported in the recent literatures.
7. Challenges and future directions
In recent research endeavors, the planning of MMGs is generally straightforward, involving considerations such as optimizing the placement of RES, configuring generation mixes, accounting for load and generation fluctuations, and addressing market dynamics. Looking ahead, the planning of MMG networks is expected to evolve to encompass fully controllable loads and integrated systems for combined heat, cold, and power. This distinctive feature of the network presents a challenge for retailers due to its unique power flow characteristics. Presently, there is a growing demand for efficient planning and EMS controllers to enable the smarter delivery and management of energy within MMGs. However, achieving solutions for various challenges in MG operation is hindered by numerous uncertainties. The involvement of demand-side management and electric vehicle participation significantly impacts ancillary services in energy trading and peak shaving applications.
When authors consider the GHG emissions in EMS problem, they only consider the emissions sources in the MG system but not the grid that may come from different static generations. ESS state of charge, degradation costs, and all generation/ load, market dynamics and cyber security issues are attracting researchers for further pace of enhancement. During dispatching and trade-off in interconnected MMG, still security and privacy issues are sounding consumers and individual MGs. The most common challenge is adopting suitable optimization approaches for effective uncertainty modeling. This paper reviews that most of the recent works have used AI and machine learning approaches for efficient data-driven extraction of uncertainty and tried to secure the privacy of the individual MG data in the case of centralized EMS structure. In general, stochastic and robust optimization approaches are suitable for uncertainties modeling but it fails as the penetration of RES increase in the MMG network.
This review summarizes the future trends related to MMG planning, operation, scheduling and control required to be considered for further research in the following aspects:
MMGs can support the grid and interact each other in the energy market to act as a ‘price maker’ hence the energy trade will be affected by their capacities. Hence, long term planning of high penetration of RES and participation of MMG will further reduce the cost of energy by considering the dynamic electricity market and other generation and load uncertainties.
Smart energy coordination, trading and scheduling has to be implemented in means of effective communication and security intelligence for participating different MGs by alleviating privacy issues using deep data analysis AI tools.
Current researches are using stochastic and robust optimization techniques to model and handle uncertainties to show the how uncertain issues hider the operation, control and scheduling of MMG. But future researches have to focus on AI based uncertainty management to identify where the uncertainties are originated, model these uncertainties using machine learning and AI methodologies for the seamless operation of next generation MMGs.
Since the MMGEMS problems are multi-objective, efficient metaheuristic optimization approaches are required to be adopted in distributed and nested structures for better coordination, improved energy sharing capability with grid connection, networked, and islanded operations. Furthermore, online optimal scheduling strategy should be applied for reducing the computational complexity of algorithms to achieve fast convergence performance among controllers.
Besides RES, load and electricity price uncertainty consideration, ESS and demand side management techniques like DR program has to be considered in MMG scheduling by predicting their variable states using efficient AI or soft computing methods in order to maximize the benefits of the system through this peak shaving and filling activity.
Current researches are focusing on the scheduling, trading and coordination of MMGs. On the other hand, power quality issues due to the intermittency of DERs and application of various power electronics-based converter in MGs impacted the power quality of the system negatively. Reliability and protection analysis are not studied yet.
8. Conclusion
This review has outlined the optimal planning and Energy Management System (EMS) of Multi-Microgrid (MMG) networks, which play a crucial role in enhancing efficiency, efficacy, and reliability within modern distribution systems. The paper introduces typical MMG topologies, their EMS structures, and approaches, encompassing formulations for optimal sizing and EMS with associated constraints.
Through an extensive and critical literature review, various problem formulations, solutions, existing approaches, algorithms, opportunities, and prospects are explored, highlighting the utilization of numerous efficient methods to date. Research indicates that different EMS strategies and uncertainties significantly impact the techno-economic analysis of MMGs, and these effects can be alleviated through diverse optimization approaches.
The paper concludes by presenting various models and solving algorithms for addressing MMG EMS, both with and without uncertainties. Approaches such as MILP, DNN, MOPSO, MPC, NSGA-II, JAYA, GWO, stochastic and robust optimizations, and other intelligent techniques are discussed in the context of different EMS problem formulations. The document summarizes the key contributions and limitations of EMS approaches across different MMGEMS architectures and outlines the challenges and future directions of MMGEMS. It concludes with a discussion on open challenges and potential paths for future research.
Dess_SLR.tex
Download Latex File (56.9 KB)Disclosure statement
No potential conflict of interest was reported by the author(s).
Data availability statement
The data of this study can be obtained by contacting the corresponding author.
Additional information
Funding
Notes on contributors
Dessalegn Bitew Aeggegn
Dessalegn Bitew Aeggegn obtained his B.Sc. from Debre Markos University in Electrical and computer Engineering in 2015, and gained his M.Sc. from Bahir Dar University in 2019 in Power system Engineering. He embraced the role of a lecturer at Debre Markos University teaching various electrical power engineering courses. He has authored and co-authored more than ten research articles. Currently he is pursuing his Ph.D. in Electrical Engineering at Pan African university institute for basic Sciences, Technology and Innovation. His research interests include Microgrid, renewable energy resources, power quality and artificial intelligent based control.
George Nyauma Nyakoe
George Nyauma Nyakoe graduated in 1993 with a B.Sc. degree in Electrical and Electronic Engineering from the University of Nairobi. He received the M.Sc. and Ph.D. degrees in Electrical Engineering from Tottori University, Japan, in 2000 and 2003, respectively. He is currently a Professor at Jomo Kenyatta University of Agriculture and Technology (JKUAT) and an adjunct professor at the Pan African University Institute for Basic Science, Technology and Innovation (PAUSTI), Kenya. His current research interests include control of power electronic systems, renewable energy integration and artificial intelligence applications.
Cyrus Wekesa
Cyrus Wekesa received the B.S. Cyrus Wabuge Wekesa received the B.Sc. and M.Sc. degrees from the University of Nairobi, Nairobi, Kenya, and the Ph.D. degree from Tokushima University, Tokushima, Japan, all in electrical engineering. Currently, he is an Associate Professor with the School of Engineering, University of Eldoret. Previously, he has taught at the Jomo Kenya University of Agriculture and Technology and the University of Nairobi. He is a pioneer Researcher in renewable energy sources for electrification in Kenya and has published papers. He has successfully supervised more than 25 M.Sc./Ph.D. students. His research interests include electrical power systems, distributed energy resources, demand side management, demand response, and energy management.
References
- Afrakhte, H., & Bayat, P. (2020). A self-evolving type-2 fuzzy energy management strategy for multi-microgrid systems. Computers & Electrical Engineering, 85, 106702. https://doi.org/10.1016/j.compeleceng.2020.106702
- Aghdam, F. H., Ghaemi, S., & Kalantari, N. T. (2018). Evaluation of loss minimization on the energy management of multi-microgrid based smart distribution network in the presence of emission constraints and clean productions. Journal of Cleaner Production, 196, 185–201. https://doi.org/10.1016/j.jclepro.2018.06.023
- Aghdam, F. H., Kalantari, N. T., & Mohammadi-Ivatloo, B. (2020). A chance-constrained energy management in multi-microgrid systems considering degradation cost of energy storage elements. Journal of Energy Storage, 29, 101416. https://doi.org/10.1016/j.est.2020.101416
- Ahmadi, S. E., & Rezaei, N. (2020). A new isolated renewable based multi microgrid optimal energy management system considering uncertainty and demand response. International Journal of Electrical Power & Energy Systems, 118, 105760. https://doi.org/10.1016/j.ijepes.2019.105760
- Ahmed, D., Ebeed, M., Ali, A., Alghamdi, A. S., & Kamel, S. (2021). Multi-objective energy management of a micro-grid considering stochastic nature of load and renewable energy resources. Electronics, 10(4), 403. https://doi.org/10.3390/electronics10040403
- Alam, M. S., & Arefifar, S. A. (2019). Energy management in power distribution systems: Review, classification, limitations and challenges. IEEE Access. 7, 92979–93001. https://doi.org/10.1109/ACCESS.2019.2927303
- Alamir, N., Kamel, S., Megahed, T. F., Hori, M., & Abdelkader, S. M. (2022). Energy management of multi-microgrid considering demand response using snake optimizer [Paper presentation]. 2022 23rd International Middle East Power Systems Conference (MEPCON) (pp. 1–6). IEEE. https://doi.org/10.1109/MEPCON55441.2022.10021750
- Alhasnawi, B. N., & Jasim, B. H. (2020). A novel hierarchical energy management system based on optimization for multi-microgrid. International Journal on Electrical Engineering & Informatics, 12(3), 586–606. https://doi.org/10.15676/ijeei.2020.12.3.10
- Ali, L., Muyeen, S., Bizhani, H., & Simoes, M. G. (2022). Economic planning and comparative analysis of market-driven multi-microgrid system for peer-to-peer energy trading. IEEE Transactions on Industry Applications, 58(3), 4025–4036. https://doi.org/10.1109/TIA.2022.3152140
- Ali, S. A., Hussain, A., Haider, W., Rehman, H. U., & Kazmi, S. A. A. (2023). Optimal energy management system of isolated multi-microgrids with local energy transactive market with indigenous PV-, wind-, and biomass-based resources. Energies, 16(4), 1667. https://doi.org/10.3390/en16041667
- Ali, S., Kazmi, S. A. A., Malik, M. M., Bhatti, A. H. U., Haseeb, M., Kazmi, S. M. R., & Shin, D. R. (2022). Energy management in high rer multi-microgrid system via energy trading and storage optimization. IEEE Access. 10, 6541–6554. https://doi.org/10.1109/ACCESS.2021.3132505
- Alseyat, A., Ullah, M. H., & Park, J.-D. (2021). A plug-and-play-capable multi-agent network for distributed consensus-based operation in dc power systems [Paper presentation]. 2021 IEEE Energy Conversion Congress and Exposition (ECCE) (pp. 681–687). IEEE.https://doi.org/10.1109/ECCE47101.2021.9595141
- Al-Shamma’a, A. A., Hussein Farh, H. M., Noman, A. M., Al-Shaalan, A. M., & Alkuhayli, A. (2022). Optimal sizing of a hybrid renewable photovoltaic-wind system-based microgrid using harris hawk optimizer. International Journal of Photoenergy, 2022, 1–13. https://doi.org/10.1155/2022/4825411
- Amoussou, I., Tanyi, E., Ali, A., Agajie, T. F., Khan, B., Ballester, J. B., & Nsanyuy, W. B. (2023). Optimal modeling and feasibility analysis of grid-interfaced solar pv/wind/pumped hydro energy storage based hybrid system. Sustainability, 15(2), 1222. https://doi.org/10.3390/su15021222
- Arefifar, S. A., Ordonez, M., & Mohamed, Y. A.-R I. (2016). Energy management in multi-microgrid systems—development and assessment. IEEE Transactions on Power Systems, 32(2), 1–1. https://doi.org/10.1109/TPWRS.2016.2568858
- Asimakopoulou, G. E., Dimeas, A. L., & Hatziargyriou, N. D. (2013). Leader-follower strategies for energy management of multi-microgrids. IEEE Transactions on Smart Grid, 4(4), 1909–1916. https://doi.org/10.1109/TSG.2013.2256941
- Babanezhaad Shirdar, H., & Ghafouri, A. (2022). Design of hybrid multilayer systems to improve energy management system in multi-microgrid systems in the presence of wind and solar power. Wind Engineering, 46(1), 34–51. https://doi.org/10.1177/0309524X211000379
- Babanezhaad Shirdar, H., & Ghafouri, A. (2022). Optimization of multi-microgrid system operation cost with different energy management tools in the presence of energy storage system. Electric Power Components and Systems, 50(16–17), 951–971. https://doi.org/10.1080/15325008.2022.2143938
- Babatunde, O. M., Munda, J. L., & Hamam, Y. (2019). A comprehensive state-of-the-art survey on power generation expansion planning with intermittent renewable energy source and energy storage. International Journal of Energy Research, 43(12), 6078–6107. https://doi.org/10.1002/er.4388
- Babatunde, O. M., Munda, J. L., & Hamam, Y. (2020). A comprehensive state-of-the-art survey on hybrid renewable energy system operations and planning. IEEE Access. 8, 75313–75346. https://doi.org/10.1109/ACCESS.2020.2988397
- Backhaus, S. N., Dobriansky, L., Glover, S., Liu, C.-C., Looney, P., Mashayekh, S., Pratt, A., Schneider, K., Stadler, M., Starke, M., et al. (2016). Networked microgrids scoping study. Los Alamos National Lab.(LANL), Los Alamos, NM (United States), Tech. Rep.
- Bagheri, Z., Doostizadeh, M., & Aminifar, F. (2021). A receding horizon data-driven chance-constrained approach for energy flexibility trading in multi-microgrid distribution network. IET Renewable Power Generation, 15(13), 2860–2877. https://doi.org/10.1049/rpg2.12215
- Bandeiras, F., Pinheiro, E., Gomes, M., Coelho, P., & Fernandes, J. (2020). Review of the cooperation and operation of microgrid clusters. Renewable and Sustainable Energy Reviews, 133, 110311. https://doi.org/10.1016/j.rser.2020.110311
- Battula, A. R., Vuddanti, S., & Salkuti, S. R. (2023). A day ahead demand schedule strategy for optimal operation of microgrid with uncertainty. Smart Cities, 6(1), 491–509. https://doi.org/10.3390/smartcities6010023
- Bazmohammadi, N., Anvari-Moghaddam, A., Tahsiri, A., Madary, A., Vasquez, J. C., & Guerrero, J. M. (2020). Stochastic predictive energy management of multi-microgrid systems. Applied Sciences, 10(14), 4833. https://doi.org/10.3390/app10144833
- Bazmohammadi, N., Tahsiri, A., Anvari-Moghaddam, A., & Guerrero, J. M. (2019). A hierarchical energy management strategy for interconnected microgrids considering uncertainty. International Journal of Electrical Power & Energy Systems, 109, 597–608. https://doi.org/10.1016/j.ijepes.2019.02.033
- Brahmia, I., Wang, J., Xu, H., Wang, H., & Turci, L. D. O. (2021). Robust data predictive control framework for smart multi-microgrid energy dispatch considering electricity market uncertainty. IEEE Access. 9, 32390–32404. https://doi.org/10.1109/ACCESS.2021.3060315
- Bukar, A. L., Tan, C. W., & Lau, K. Y. (2019). Optimal sizing of an autonomous photovoltaic/wind/battery/diesel generator microgrid using grasshopper optimization algorithm. Solar Energy, 188, 685–696. https://doi.org/10.1016/j.solener.2019.06.050
- Bustos, R., Marín, L. G., Navas-Fonseca, A., Reyes-Chamorro, L., & Sáez, D. (2023). Hierarchical energy management system for multi-microgrid coordination with demand-side management. Applied Energy, 342, 121145. https://doi.org/10.1016/j.apenergy.2023.121145
- Cao, B., Dong, W., Lv, Z., Gu, Y., Singh, S., & Kumar, P. (2020). Hybrid microgrid many-objective sizing optimization with fuzzy decision. IEEE Transactions on Fuzzy Systems, 28(11), 2702–2710. https://doi.org/10.1109/TFUZZ.2020.3026140
- Castellanos, J., Correa-Flórez, C. A., Garcés, A., Ordóñez-Plata, G., Uribe, C. A., & Patino, D. (2023). An energy management system model with power quality constraints for unbalanced multi-microgrids interacting in a local energy market. Applied Energy, 343, 121149. https://doi.org/10.1016/j.apenergy.2023.121149
- Ceja-Espinosa, C., Pirnia, M., & Cañizares, C. A. (2022). A privacy-preserving energy management system for cooperative multi-microgrid networks. arXiv preprint arXiv:2207.04359.
- Chen, S. X., Gooi, H. B., & Wang, M. (2012). Sizing of energy storage for microgrids. IEEE Transactions on Smart Grid, 3(1), 142–151. https://doi.org/10.1109/TSG.2011.2160745
- Choobineh, M., & Mohagheghi, S. (2019). Robust optimal energy pricing and dispatch for a multi-microgrid industrial park operating based on just-in-time strategy. IEEE Transactions on Industry Applications, 55(4), 3321–3330. https://doi.org/10.1109/TIA.2019.2903182
- Copp, D. A., Nguyen, T. A., Byrne, R. H., & Chalamala, B. R. (2022). Optimal sizing of distributed energy resources for planning 100% renewable electric power systems. Energy, 239, 122436. https://doi.org/10.1016/j.energy.2021.122436
- Dey, B., Basak, S., & Bhattacharyya, B. (2023). Demand-side-management-based bi-level intelligent optimal approach for cost-centric energy management of a microgrid system. Arabian Journal for Science and Engineering, 48(5), 6819–6830. https://doi.org/10.1007/s13369-022-07546-2
- Dey, B., Misra, S., & Marquez, F. P. G. (2023). Microgrid system energy management with demand response program for clean and economical operation. Applied Energy, 334, 120717. https://doi.org/10.1016/j.apenergy.2023.120717
- Du, Y., & Li, F. (2020). Intelligent multi-microgrid energy management based on deep neural network and model-free reinforcement learning. IEEE Transactions on Smart Grid, 11(2), 1066–1076. https://doi.org/10.1109/TSG.2019.2930299
- Du, Y., Wang, Z., Liu, G., Chen, X., Yuan, H., Wei, Y., & Li, F. (2018). A cooperative game approach for coordinating multi-microgrid operation within distribution systems. Applied Energy, 222, 383–395. https://doi.org/10.1016/j.apenergy.2018.03.086
- Ediriweera, W. S., & Lidula, N. (2022). Design and protection of microgrid clusters: A comprehensive review. AIMS Energy, 10(3), 375–411. https://doi.org/10.3934/energy.2022020
- El-Hendawi, M., Gabbar, H. A., El-Saady, G., & Ibrahim, E.-N A. (2018). Control and ems of a grid-connected microgrid with economical analysis. Energies, 11(1), 129. https://doi.org/10.3390/en11010129
- Elkazaz, M., Sumner, M., & Thomas, D. (2020). Energy management system for hybrid pv-wind-battery microgrid using convex programming, model predictive and rolling horizon predictive control with experimental validation. International Journal of Electrical Power & Energy Systems, 115, 105483. https://doi.org/10.1016/j.ijepes.2019.105483
- Gamarra, C., & Guerrero, J. M. (2015). Computational optimization techniques applied to microgrids planning: A review. Renewable and Sustainable Energy Reviews, 48, 413–424. https://doi.org/10.1016/j.rser.2015.04.025
- Gamil, M. M., Senjyu, T., Takahashi, H., Hemeida, A. M., Krishna, N., & Lotfy, M. E. (2021). Optimal multi-objective sizing of a residential microgrid in Egypt with different tou demand response percentages. Sustainable Cities and Society, 75, 103293. https://doi.org/10.1016/j.scs.2021.103293
- Gao, J., Li, Y., Wang, B., & Wu, H. (2023). Multi-microgrid collaborative optimization scheduling using an improved multi-agent soft actor-critic algorithm. Energies, 16(7), 3248. https://doi.org/10.3390/en16073248
- García Vera, Y. E., Dufo-López, R., & Bernal-Agustín, J. L. (2019). Energy management in microgrids with renewable energy sources: A literature review. Applied Sciences, 9(18), 3854. https://doi.org/10.3390/app9183854
- Ge, L., Song, Z., Xu, X., Bai, X., & Yan, J. (2021). Dynamic networking of islanded regional multi-microgrid networks based on graph theory and multi-objective evolutionary optimization. International Transactions on Electrical Energy Systems, 31(1), e12687. https://doi.org/10.1002/2050-7038.12687
- Ghaemi, S., Salehi, J., & Hamzeh Aghdam, F. (2019). Risk aversion energy management in the networked microgrids with presence of renewable generation using decentralised optimisation approach. IET Renewable Power Generation, 13(7), 1050–1061. https://doi.org/10.1049/iet-rpg.2018.5573
- Ghiasi, M. (2019). Detailed study, multi-objective optimization, and design of an AC-DC smart microgrid with hybrid renewable energy resources. Energy, 169, 496–507. https://doi.org/10.1016/j.energy.2018.12.083
- Gong, C., Zeng, S., Li, X., Chen, P., Ding, Y., Liu, C., & Jiang, L. (2022). A brief view on energy management of multi-microgrid systems: Framework, communication technologies, and dispatching strategies [Paper presentation]. 2022 7th Asia Conference on Power and Electrical Engineering (ACPEE) (pp. 677–683). IEEE. https://doi.org/10.1109/ACPEE53904.2022.9783998
- Guo, C., Wang, X., Zheng, Y., & Zhang, F. (2021). Optimal energy management of multi-microgrids connected to distribution system based on deep reinforcement learning. International Journal of Electrical Power & Energy Systems, 131, 107048. https://doi.org/10.1016/j.ijepes.2021.107048
- Guo, G., & Gong, Y. (2023). Multi-microgrid energy management strategy based on multi-agent deep reinforcement learning with prioritized experience replay. Applied Sciences, 13(5), 2865. https://doi.org/10.3390/app13052865
- Haddadian, H., & Noroozian, R. (2019). Multi-microgrid-based operation of active distribution networks considering demand response programs. IEEE Transactions on Sustainable Energy, 10(4), 1804–1812. https://doi.org/10.1109/TSTE.2018.2873206
- Hao, R., Ai, Q., Liu, Y., Xiong, W., Wang, L., Yu, Z., Zhang, Z., & Zhang, Y. (2020). Distributed interactive energy management for networked microgrids based on nested architecture [Paper presentation]. 2020 IEEE Power & Energy Society General Meeting (PESGM) (pp. 1–5). IEEE. https://doi.org/10.1109/PESGM41954.2020.9281756
- Harmouch, F. Z., Krami, N., & Hmina, N. (2018). A multiagent based decentralized energy management system for power exchange minimization in microgrid cluster. Sustainable Cities and Society, 40, 416–427. https://doi.org/10.1016/j.scs.2018.04.001
- Haseeb, M., Kazmi, S. A. A., Malik, M. M., Ali, S., Bukhari, S. B. A., & Shin, D. R. (2020). Multi objective based framework for energy management of smart micro-grid. IEEE Access. 8, 220302–220319. https://doi.org/10.1109/ACCESS.2020.3041473
- Hossain, M. A., Pota, H. R., Squartini, S., & Abdou, A. F. (2019). Modified pso algorithm for real-time energy management in grid-connected microgrids. Renewable Energy. 136, 746–757. https://doi.org/10.1016/j.renene.2019.01.005
- Hu, K-y., Li, W-j., Wang, L-d., Cao, S-h., Zhu, F-m., & Shou, Z-x (2018). Energy management for multi-microgrid system based on model predictive control. Frontiers of Information Technology & Electronic Engineering, 19(11), 1340–1351. https://doi.org/10.1631/FITEE.1601826
- Hussain, A., Bui, V.-H., & Kim, H.-M. (2018). A resilient and privacy-preserving energy management strategy for networked microgrids. IEEE Transactions on Smart Grid, 9(3), 2127–2139. https://doi.org/10.1109/TSG.2016.2607422
- Islam, M. M., Nagrial, M., Rizk, J., & Hellany, A. (2021). General aspects, islanding detection, and energy management in microgrids: A review. Sustainability, 13(16), 9301. https://doi.org/10.3390/su13169301
- Jafari, A., Ganjehlou, H. G., Khalili, T., & Bidram, A. (2020). A fair electricity market strategy for energy management and reliability enhancement of islanded multi-microgrids. Applied Energy, 270, 115170. https://doi.org/10.1016/j.apenergy.2020.115170
- Jain, R., & Mahajan, V. (2022). Energy management of multi-microgrids in joint energy and ancillary service market considering uncertainties of renewables. Journal of Renewable Energy and Environment, 10(2), 95–107. https://doi.org/10.30501/jree.2022.329343.1334
- Javidsharifi, M., Pourroshanfekr Arabani, H., Kerekes, T., Sera, D., & Guerrero, J. M. (2022). Stochastic optimal strategy for power management in interconnected multi-microgrid systems. Electronics, 11(9), 1424. https://doi.org/10.3390/electronics11091424
- Kampelis, N., Tsekeri, E., Kolokotsa, D., Kalaitzakis, K., Isidori, D., & Cristalli, C. (2018). Development of demand response energy management optimization at building and district levels using genetic algorithm and artificial neural network modelling power predictions. Energies, 11(11), 3012. https://doi.org/10.3390/en11113012
- Karimi, H., Bahmani, R., Jadid, S., & Makui, A. (2021). Dynamic transactive energy in multi-microgrid systems considering independence performance index: A multi-objective optimization framework. International Journal of Electrical Power & Energy Systems, 126, 106563. https://doi.org/10.1016/j.ijepes.2020.106563
- Karimi, H., Gharehpetian, G., Ahmadiahangar, R., & Rosin, A. (2023). Optimal energy management of grid-connected multi-microgrid systems considering demand-side flexibility: A two-stage multi-objective approach. Electric Power Systems Research, 214, 108902. https://doi.org/10.1016/j.epsr.2022.108902
- Karimi, H., Jadid, S., & Makui, A. (2021). Stochastic energy scheduling of multi-microgrid systems considering independence performance index and energy storage systems. Journal of Energy Storage, 33, 102083. https://doi.org/10.1016/j.est.2020.102083
- Keshta, H., Ali, A., Saied, E., & Bendary, F. (2019). Real-time operation of multi-micro-grids using a multi-agent system. Energy, 174, 576–590. https://doi.org/10.1016/j.energy.2019.02.145
- Köbrich, D., Marín, L. G., Muñoz‐Carpintero, D., Ahumada, C., Sáez, D., Sumner, M., & Jiménez‐Estévez, G. (2022). A robust distributed energy management system for the coordinated operation of rural multi-microgrids. International Journal of Energy Research, 46(14), 19775–19795. https://doi.org/10.1002/er.8502
- Kulasekera, A., Gopura, R., Hemapala, K., & Perera, N. (2011). A review on multi-agent systems in microgrid applications. ISGT2011-India (pp. 173–177).
- Kumar, A., Sharma, S., & Verma, A. (2020). Optimal sizing and multi-energy management strategy for pv-biofuel-based off-grid systems. IET Smart Grid. 3(1), 83–97. https://doi.org/10.1049/iet-stg.2019.0178
- Kumar, M., & Tyagi, B. (2020). An optimal multivariable constrained nonlinear (mvcnl) stochastic microgrid planning and operation problem with renewable penetration. IEEE Systems Journal, 14(3), 4143–4154. https://doi.org/10.1109/JSYST.2019.2963729
- Li, B., Roche, R., & Miraoui, A. (2017). Microgrid sizing with combined evolutionary algorithm and milp unit commitment. Applied Energy, 188, 547–562. https://doi.org/10.1016/j.apenergy.2016.12.038
- Li, C., Jia, X., Zhou, Y., & Li, X. (2020). A microgrids energy management model based on multi-agent system using adaptive weight and chaotic search particle swarm optimization considering demand response. Journal of Cleaner Production, 262, 121247. https://doi.org/10.1016/j.jclepro.2020.121247
- Li, Y., Han, M., Yang, Z., & Li, G. (2021). Coordinating flexible demand response and renewable uncertainties for scheduling of community integrated energy systems with an electric vehicle charging station: A bi-level approach. IEEE Transactions on Sustainable Energy, 12(4), 2321–2331. https://doi.org/10.1109/TSTE.2021.3090463
- Li, Y., He, S., Li, Y., Shi, Y., & Zeng, Z. (2023). Federated multiagent deep reinforcement learning approach via physics-informed reward for multimicrogrid energy management. IEEE Transactions on Neural Networks and Learning Systems, 1–13. https://doi.org/10.1109/TNNLS.2022.3232630
- Li, Y., Yang, Z., Li, G., Zhao, D., & Tian, W. (2019). Optimal scheduling of an isolated microgrid with battery storage considering load and renewable generation uncertainties. IEEE Transactions on Industrial Electronics, 66(2), 1565–1575. https://doi.org/10.1109/TIE.2018.2840498
- Lin, Y., Bie, Z., & Qiu, A. (2018). A review of key strategies in realizing power system resilience. Global Energy Interconnection, 1(1), 70–78.
- Liu, Y., Gooi, H. B., & Xin, H. (2017). Distributed energy management for the multi-microgrid system based on admm. 2017 IEEE Power & Energy Society General Meeting (pp. 1–5). IEEE.
- Liu, Z., Gao, J., Yu, H., & Wang, X. (2020). Operation mechanism and strategies for transactive electricity market with multi-microgrid in grid-connected mode. IEEE Access. 8, 79594–79603. https://doi.org/10.1109/ACCESS.2020.2990297
- Luo, S., & Guo, X. (2023). Multi-objective optimization of multi-microgrid power dispatch under uncertainties using interval optimization. Journal of Industrial and Management Optimization, 19(2), 823–851. https://doi.org/10.3934/jimo.2021208
- Mahmoud, F. S., Abdelhamid, A. M., Al Sumaiti, A., El-Sayed, A.-H M., & Diab, A. A. Z. (2022). Sizing and design of a pv-wind-fuel cell storage system integrated into a grid considering the uncertainty of load demand using the marine predators algorithm. Mathematics, 10(19), 3708. https://doi.org/10.3390/math10193708
- Mahmoud, I., Omran, W. A., & Hasanien, H. (2021). Day-ahead scheduling for grid connected multi-microgrids via hierarchical energy management strategy [Paper presentation]. 2021 22nd International Middle East Power Systems Conference (MEPCON) (pp. 58–64). IEEE. https://doi.org/10.1109/MEPCON50283.2021.9686224
- Mansour-Saatloo, A., Pezhmani, Y., Mirzaei, M. A., Mohammadi-Ivatloo, B., Zare, K., Marzband, M., & Anvari-Moghaddam, A. (2021). Robust decentralized optimization of multi-microgrids integrated with power-to-x technologies. Applied Energy, 304, 117635. https://doi.org/10.1016/j.apenergy.2021.117635
- Mao, M., Wang, Y., Chang, L., & Du, Y. (2017). Operation optimization for multi-microgrids based on centralized-decentralized hybrid hierarchical energy management [Paper presentation]. 2017 IEEE Energy Conversion Congress and Exposition (ECCE) (pp. 4813–4820). IEEE. https://doi.org/10.1109/ECCE.2017.8096818
- Mazidi, M., Rezaei, N., Ardakani, F. J., Mohiti, M., & Guerrero, J. M. (2020). A hierarchical energy management system for islanded multi-microgrid clusters considering frequency security constraints. International Journal of Electrical Power & Energy Systems, 121, 106134. https://doi.org/10.1016/j.ijepes.2020.106134
- Mehraban, S. A., & Eslami, R. (2023). Multi-microgrids energy management in power transmission mode considering different uncertainties. Electric Power Systems Research, 216, 109071. https://doi.org/10.1016/j.epsr.2022.109071
- Mirbarati, S. H., Heidari, N., Nikoofard, A., Danish, M. S. S., & Khosravy, M. (2022). Techno-economic-environmental energy management of a micro-grid: A mixed-integer linear programming approach. Sustainability, 14(22), 15036. https://doi.org/10.3390/su142215036
- Moghimi, M., Jamborsalamati, P., Hossain, J., Stegen, S., & Lu, J. (2018). A hybrid communication platform for multi-microgrid energy management system optimization [Paper presentation]. 2018 IEEE 27th International Symposium on Industrial Electronics (ISIE) (pp. 1215–1220). IEEE. https://doi.org/10.1109/ISIE.2018.8433861
- Mohamed, M. A., Hajjiah, A., Alnowibet, K. A., Alrasheedi, A. F., Awwad, E. M., & Muyeen, S. (2021). A secured advanced management architecture in peer-to-peer energy trading for multi-microgrid in the stochastic environment. IEEE Access. 9, 92083–92100. https://doi.org/10.1109/ACCESS.2021.3092834
- Muqeet, H. A., Javed, H., Akhter, M. N., Shahzad, M., Munir, H. M., Nadeem, M. U., Bukhari, S. S. H., & Huba, M. (2022). Sustainable solutions for advanced energy management system of campus microgrids: Model opportunities and future challenges. Sensors, 22(6), 2345. https://doi.org/10.3390/s22062345
- Naderi, M., Khayat, Y., Shafiee, Q., Blaabjerg, F., & Bevrani, H. (2023). Dynamic modeling, stability analysis and control of interconnected microgrids: A review. Applied Energy, 334, 120647. https://doi.org/10.1016/j.apenergy.2023.120647
- Nawaz, A., Wu, J., Ye, J., Dong, Y., & Long, C. (2023). Distributed mpc-based energy scheduling for islanded multi-microgrid considering battery degradation and cyclic life deterioration. Applied Energy, 329, 120168. https://doi.org/10.1016/j.apenergy.2022.120168
- Nawaz, A., Zhou, M., Wu, J., & Long, C. (2022). A comprehensive review on energy management, demand response, and coordination schemes utilization in multi-microgrids network. Applied Energy, 323, 119596. https://doi.org/10.1016/j.apenergy.2022.119596
- Nikmehr, N., & Ravadanegh, S. N. (2015). Optimal power dispatch of multi-microgrids at future smart distribution grids. IEEE Transactions on Smart Grid, 6(4), 1648–1657. https://doi.org/10.1109/TSG.2015.2396992
- Okhuegbe, S., Mwaniki, C., & Akorede, M. (2022). Optimal sizing of hybrid energy systems in a microgrid: A review. In Proceedings of the Sustainable Research and Innovation Conference (pp. 88–100).
- Ouammi, A., Dagdougui, H., Dessaint, L., & Sacile, R. (2015). Coordinated model predictive-based power flows control in a cooperative network of smart microgrids. IEEE Transactions on Smart Grid, 6(5), 2233–2244. https://doi.org/10.1109/TSG.2015.2396294
- Ouramdane, O., Elbouchikhi, E., Amirat, Y., & Sedgh Gooya, E. (2021). Optimal sizing and energy management of microgrids with vehicle-to-grid technology: a critical review and future trends. Energies, 14(14), 4166. https://doi.org/10.3390/en14144166
- Page, M. J., McKenzie, J. E., Bossuyt, P. M., Boutron, I., Hoffmann, T. C., Mulrow, C. D., Shamseer, L., Tetzlaff, J. M., Akl, E. A., Brennan, S. E., Chou, R., Glanville, J., Grimshaw, J. M., Hróbjartsson, A., Lalu, M. M., Li, T., Loder, E. W., Mayo-Wilson, E., McDonald, S., … Moher, D. (2021). The prisma 2020 statement: An updated guideline for reporting systematic reviews. International Journal of Surgery (London, England), 88, 105906. https://doi.org/10.1016/j.ijsu.2021.105906
- Pisei, S., Choi, J.-Y., Lee, W.-P., & Won, D.-J. (2017). Optimal power scheduling in multi-microgrid system using particle swarm optimization. Journal of Electrical Engineering and Technology, 12(4), 1329–1339.
- Qiu, H., & You, F. (2020). Decentralized-distributed robust electric power scheduling for multi-microgrid systems. Applied Energy, 269, 115146. https://doi.org/10.1016/j.apenergy.2020.115146
- Rajagopalan, A., Nagarajan, K., Montoya, O. D., Dhanasekaran, S., Kareem, I. A., Perumal, A. S., Lakshmaiya, N., & Paramasivam, P. (2022). Multi-objective optimal scheduling of a microgrid using oppositional gradient-based grey wolf optimizer. Energies, 15(23), 9024. https://doi.org/10.3390/en15239024
- Ramli, M. A., Bouchekara, H., & Alghamdi, A. S. (2018). Optimal sizing of pv/wind/diesel hybrid microgrid system using multi-objective self-adaptive differential evolution algorithm. Renewable Energy. 121, 400–411. https://doi.org/10.1016/j.renene.2018.01.058
- Roy, A., Auger, F., Olivier, J.-C., Schaeffer, E., & Auvity, B. (2020). Design, sizing, and energy management of microgrids in harbor areas: a review. Energies, 13(20), 5314. https://doi.org/10.3390/en13205314
- Saha, D., Bazmohammadi, N., Vasquez, J. C., & Guerrero, J. M. (2023). Multiple microgrids: A review of architectures and operation and control strategies. Energies, 16(2), 600. https://doi.org/10.3390/en16020600
- Samuel, O., Javaid, N., Khalid, A., Khan, W. Z., Aalsalem, M. Y., Afzal, M. K., & Kim, B.-S. (2020). Towards real-time energy management of multi-microgrid using a deep convolution neural network and cooperative game approach. IEEE Access. 8, 161377–161395. https://doi.org/10.1109/ACCESS.2020.3021613
- Sandgani, M. R., & Sirouspour, S. (2018). Priority-based microgrid energy management in a network environment. IEEE Transactions on Sustainable Energy, 9(2), 980–990. https://doi.org/10.1109/TSTE.2017.2769558
- Seyednouri, S. R., Safari, A., Farrokhifar, M., Ravadanegh, S. N., Quteishat, A., & Younis, M. (2023). Day-ahead scheduling of multi-energy microgrids based on a stochastic multi-objective optimization model. Energies, 16(4), 1802. https://doi.org/10.3390/en16041802
- Sheikhahmadi, P., Bahramara, S., Shahrokhi, S., Chicco, G., Mazza, A., & Catalão, J. P. (2020). Modeling local energy market for energy management of multi-microgrids [Paper presentation].2020 55th International Universities Power Engineering Conference (UPEC) (pp. 1–6). IEEE. https://doi.org/10.1109/UPEC49904.2020.9209891
- Spiegel, M. H., Veith, E. M., & Strasser, T. I. (2020). The spectrum of proactive, resilient multi-microgrid scheduling: A systematic literature review. Energies, 13(17), 4543. https://doi.org/10.3390/en13174543
- Sun, Y., Cai, Z., Zhang, Z., Guo, C., Ma, G., & Han, Y. (2020). Coordinated energy scheduling of a distributed multi-microgrid system based on multi-agent decisions. Energies, 13(16), 4077. https://doi.org/10.3390/en13164077
- Tan, B., & Chen, H. (2020). Multi-objective energy management of multiple microgrids under random electric vehicle charging. Energy, 208, 118360. https://doi.org/10.1016/j.energy.2020.118360
- Tan, B., Chen, H., Zheng, X., & Huang, J. (2022). Two-stage robust optimization dispatch for multiple microgrids with electric vehicle loads based on a novel data-driven uncertainty set. International Journal of Electrical Power & Energy Systems, 134, 107359. https://doi.org/10.1016/j.ijepes.2021.107359
- Tian, P., Xiao, X., Wang, K., & Ding, R. (2016). A hierarchical energy management system based on hierarchical optimization for microgrid community economic operation. IEEE Transactions on Smart Grid, 7(5), 2230–2241. https://doi.org/10.1109/TSG.2015.2470551
- Vaka, S. S. K. R., & Matam, S. K. (2021). Optimal sizing and management of battery energy storage systems in microgrids for operating cost minimization. Electric Power Components and Systems, 49(16–17), 1319–1332. https://doi.org/10.1080/15325008.2022.2061641
- Villanueva-Rosario, J. A., Santos-García, F., Aybar-Mejía, M. E., Mendoza-Araya, P., & Molina-García, A. (2022). Coordinated ancillary services, market participation and communication of multi-microgrids: A review. Applied Energy, 308, 118332. https://doi.org/10.1016/j.apenergy.2021.118332
- Wang, X., Wang, C., Xu, T., Guo, L., Li, P., Yu, L., & Meng, H. (2018). Optimal voltage regulation for distribution networks with multi-microgrids. Applied Energy, 210, 1027–1036. https://doi.org/10.1016/j.apenergy.2017.08.113
- Waqar, A., Nawaz, M. S., Aamir, M., Alam, I., Ali, S. U., & Ahmad, J. (2019). Multi-objective analysis of der sizing in microgrids using probabilistic modeling [Paper presentation]. 2019 International Conference on Electrical, Communication, and Computer Engineering (ICECCE) (pp. 1–6). IEEE. https://doi.org/10.1109/ICECCE47252.2019.8940702
- Wu, D., Ma, X., Huang, S., Fu, T., & Balducci, P. (2020). Stochastic optimal sizing of distributed energy resources for a cost-effective and resilient microgrid. Energy, 198, 117284. https://doi.org/10.1016/j.energy.2020.117284
- Wu, Q., Xie, Z., Li, Q., Ren, H., & Yang, Y. (2022). Economic optimization method of multi-stakeholder in a multi-microgrid system based on stackelberg game theory. Energy Reports, 8, 345–351. https://doi.org/10.1016/j.egyr.2021.11.148
- Wynn, S. L. L., Pinthurat, W., & Marungsri, B. (2022). Multi-objective optimization for peak shaving with demand response under renewable generation uncertainty. Energies, 15(23), 8989. https://doi.org/10.3390/en15238989
- Xie, H., Teng, X., Xu, Y., & Wang, Y. (2019). Optimal energy storage sizing for networked microgrids considering reliability and resilience. IEEE Access. 7, 86336–86348. https://doi.org/10.1109/ACCESS.2019.2922994
- Xie, M., Ji, X., Hu, X., Cheng, P., Du, Y., & Liu, M. (2018). Autonomous optimized economic dispatch of active distribution system with multi-microgrids. Energy, 153, 479–489. https://doi.org/10.1016/j.energy.2018.04.021
- Yang, J., & Su, C. (2021). Robust optimization of microgrid based on renewable distributed power generation and load demand uncertainty. Energy, 223, 120043. https://doi.org/10.1016/j.energy.2021.120043
- Yang, X., He, H., Zhang, Y., Chen, Y., & Weng, G. (2019). Interactive energy management for enhancing power balances in multi-microgrids. IEEE Transactions on Smart Grid, 10(6), 6055–6069. https://doi.org/10.1109/TSG.2019.2896182
- Yu, Y., Li, G., & Li, Z. (2020). A game theoretical pricing mechanism for multi-microgrid energy trading considering electric vehicles uncertainty. IEEE Access. 8, 156519–156529. https://doi.org/10.1109/ACCESS.2020.3019815
- Zeinal-Kheiri, S., Shotorbani, A. M., & Mohammadi-Ivatloo, B. (2020). Real-time energy management of grid-connected microgrid with flexible and delay-tolerant loads. Journal of Modern Power Systems and Clean Energy, 8(6), 1196–1207. https://doi.org/10.35833/MPCE.2018.000615
- Zhang, B., Li, Q., Wang, L., & Feng, W. (2018). Robust optimization for energy transactions in multi-microgrids under uncertainty. Applied Energy, 217, 346–360. https://doi.org/10.1016/j.apenergy.2018.02.121
- Zhang, Y., Lv, Y., & Zhou, Y. (2023). Research on economic optimal dispatching of microgrid based on an improved bacteria foraging optimization. Biomimetics, 8(2), 150. https://doi.org/10.3390/biomimetics8020150
- Zhao, B., Zhang, X., Li, P., Wang, K., Xue, M., & Wang, C. (2014). Optimal sizing, operating strategy and operational experience of a stand-alone microgrid on Dongfushan Island. Applied Energy, 113, 1656–1666. https://doi.org/10.1016/j.apenergy.2013.09.015
- Zhao, J., Li, F., Mukherjee, S., & Sticht, C. (2022). Deep reinforcement learning-based model-free on-line dynamic multi-microgrid formation to enhance resilience. IEEE Transactions on Smart Grid, 13(4), 2557–2567. https://doi.org/10.1109/TSG.2022.3160387
- Zhong, X., Zhong, W., Liu, Y., Yang, C., & Xie, S. (2022). Optimal energy management for multi-energy multi-microgrid networks considering carbon emission limitations. Energy, 246, 123428. https://doi.org/10.1016/j.energy.2022.123428
- Zhou, B., Zou, J., Chung, C. Y., Wang, H., Liu, N., Voropai, N., & Xu, D. (2021). Multi-microgrid energy management systems: Architecture, communication, and scheduling strategies. Journal of Modern Power Systems and Clean Energy, 9(3), 463–476. https://doi.org/10.35833/MPCE.2019.000237
- Zhou, H., & Erol-Kantarci, M. (2020). Decentralized microgrid energy management: A multi-agent correlated q-learning approach [Paper presentation]. 2020 IEEE International Conference on Communications, Control, and Computing Technologies for Smart Grids (SmartGridComm) (pp. 1–7). IEEE. https://doi.org/10.1109/SmartGridComm47815.2020.9302992
- Zhou, X., Ai, Q., & Yousif, M. (2019). Two kinds of decentralized robust economic dispatch framework combined distribution network and multi-microgrids. Applied Energy, 253, 113588. https://doi.org/10.1016/j.apenergy.2019.113588
- Zhu, W., Guo, J., Zhao, G., & Zeng, B. (2020). Optimal sizing of an island hybrid microgrid based on improved multi-objective grey wolf optimizer. Processes, 8(12), 1581. https://doi.org/10.3390/pr8121581
- Zia, M. F., Elbouchikhi, E., & Benbouzid, M. (2018). Microgrids energy management systems: A critical review on methods, solutions, and prospects. Applied Energy, 222, 1033–1055. https://doi.org/10.1016/j.apenergy.2018.04.103
- Zou, H., Mao, S., Wang, Y., Zhang, F., Chen, X., & Cheng, L. (2019). A survey of energy management in interconnected multi-microgrids. IEEE Access. 7, 72158–72169. https://doi.org/10.1109/ACCESS.2019.2920008
- Zubidi, A. N., Ismail, B., Al Hamrounni, I. M. A., Rahman, N. H. A., & Mohd Rozlan, M. H. H. (2023). The impact of integrating multi-microgrid system with facts devices for voltage profile enhancement and real power loss reduction in power system: A review. Pertanika Journal of Science & Technology, 31(2), 633–654. https://doi.org/10.47836/pjst.31.2.01.