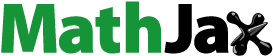
Abstract
Consumer interactions on social media have been analysed in the literature as sources of information about their ability to generate engagement and knowledge about how to interact with brands. The present study contributes to this line of research, focusing on empirically determining those elements that affect the interaction between a fast fashion brand and its users, providing central elements of brand strategies on Instagram. This study examines the engagement generated by Primark posts on Instagram based on three coding categories that analyse the content of the message, the communication strategy and the formal and product category aspects present in the posts. This study therefore identifies the variables with the greatest potential to generate engagement with this brand’s posts on Instagram, which in turn predicts previous unexplored outcomes such as brand image and consumer loyalty. This study also provides valuable academic insights and guides fast fashion brands in their social media strategies.
REVIEWING EDITOR:
JEL CLASSIFICATIONS:
1. Introduction
Consumers are increasingly using social networking sites (SNSs) to obtain brand information and interact with other consumers (Gaber et al., Citation2021; Gutierrez et al., Citation2023). Through them, users can contribute ideas, evaluations or suggestions, thereby producing content.
These interactions can influence consumer intention (Bhattacharya, Citation2023) and help build and manage brand identity. They also help shape debate on social media (Jamil et al., Citation2021). Since these consumer interactions are subject to less control and can spread quickly, they also pose a number of challenges that must be continually monitored (Xue et al., Citation2023).
There is therefore an academic and managerial need to better understand consumer interactions on the Internet. To generate knowledge on the subject, this study attempts to identify those variables that generate a greater degree of participation and interaction that users have with a fashion brand in the fast fashion segment, a sector that has revolutionised the world of fashion and that has changed production, distribution and consumption habits, encouraging a rapid turnover of garments and trends (Stringer, Citation2023). Moreover, fast fashion brands are emerging as the most effective in online communication.
To do this, we analysed the likes and comments and identified the communication strategy of the brand Primark in its social interaction with followers of its official Instagram account. This company is one of the world’s most popular fashion retailers and its genuine communication with customers has helped it achieve global reach (Ramesh, Citation2022). As Kraaijenbrink (Citation2019) points out, both its market share and operating profit margin have increased over the years.
The use and popularity of Instagram has grown rapidly, especially among young people (Romero Saletti et al., Citation2022). This social network allows users to share images and videos publicly or privately, and other users can view, comment and click the ‘Like’ icon. It also provides the option to apply certain photographic filters to the images they want to share (Y. J. Lee, Citation2023). Its growth rate is very high in terms of registered users thanks to the rise of smartphones. In 2021, there were 1.21 billion monthly active users, representing more than 28 per cent of the world’s Internet users (Statista, Citation2023) and this number is increasing daily.
Since its launch, Instagram has been strongly connected to fashion (Tang & Raheem, Citation2023), one of the industries that represents an important part of the world economy (McKinsey & Company, Citation2019), which makes Instagram particularly interesting for fashion brands, as they have significantly more followers and interactions than brands linked to other sectors (Ibrahim & Aljarah, Citation2023). According to the literature reviewed, most brands use Instagram for marketing purposes.
A growing number of studies focusing on Instagram are currently emerging, although most of these highlight the content posted (eg Jin & Ryu, Citation2019; Jinyoung Yoo et al., Citation2023; Kim et al., Citation2023), the reasons for using Instagram (eg Milanesi et al., Citation2022), consumer interaction behaviours (Bonilla et al., Citation2019), and the characteristics of brand-generated content.
In the context of the communication strategy, the content of Primark’s posts and its interaction on Instagram are analysed, and whether these interactions point to specific strategies and trends.
Therefore, the main aim of this study is to analyse the levels of engagement of users with the content posted by Primark on Instagram, the social network where the brand has a greater online presence. To this end, this study focuses on the following question: What information does engagement between the Primark brand and consumers provide?
The structure of the study is as follows: Section 2 establishes the theoretical foundations of the research: theoretical framework, empirical model and hypothesis development. The methodology is set out in section 3 and the results are analysed in section 4, while the discussion and managerial implications are presented in section 5. Finally, the limitations and future lines of research are presented in section 7.
2. Theoretical framework, empirical model and hypothesis development
The importance of multimedia content is relevant for fashion brands on Instagram (Jones & Lee, Citation2022). The image constitutes the main element in the content of the post and therefore decisively affects the engagement of fashion brands with consumers. Several investigations address this subject, including those by Hu et al. (Citation2014), who define five main categories (selfie, body snap, marketing, product only and not fashion) and seven subcategories (phase, logo, brand logo, smile, outdoors, people and items) or those of Na and Kim (Citation2019), who establish seven categories (lookbook and product photos, collection photos, videos and photos from advertisements and magazine spreads, brand editing, sensitivity images not directly related to the brand, photos provided by sponsors to celebrities and events using the Instagram account). Çukul (Citation2015) establishes nine categories to analyse the contents of posts: product, promotion, advertising, social responsibility (CSR), special days, workplace/workers, consumer-provided content and public relations (). Similarly, D. Lee et al. (Citation2018) found that direct information content, such as mentions of prices or offers, is associated with lower levels of engagement when included in messages in isolation, but with higher levels of engagement when provided in combination with attributes related to brand personality, while for Hu et al. (Citation2014) images of fashion brands that show only the product are less effective in generating likes and comments. However, for Lindell (Citation2019), photos that show faces attract more likes.
Table 1. Definition of the Çukul (Citation2015) model variables.
Engagement, or the way in which a follower or consumer relates and interacts with a fashion brand, is one of the non-economic benefits of brands’ presence on Instagram. Behavioural engagement refers to an individual’s active, observable participation in actions, behaviours, or specific activities that indicate a deep, ongoing connection to a brand, organisation, or entity. In essence, behavioural engagement involves tangible, measurable actions that reflect an active sustained bond with the entity. Various authors have conceptualised engagement in different ways depending on its dimension (Pansari & Kumar, Citation2017). While some have described it as a multidimensional construct composed of three dimensions – cognitive, affective and behavioural – (Ma et al., Citation2022), others have described it as being unidimensional or two-dimensional (Mir, Citation2023). Some authors have also included the social dimension in the previous three when referring to engagement in the social context (Cao et al., Citation2021). Professionals, on the other hand, consider behavioural engagement differently. They define it as activities that facilitate ‘repeated interactions that strengthen the emotional, psychological or physical investment that a customer has with a brand’ (Ting et al., Citation2021). presents the dimensions used by various authors that describe consumer engagement and helps identify the common characteristic of the most significant differences in the way these dimensions are presented.
Table 2. The dimensions of engagement.
Instagram analytics tools measure engagement levels based on different types of follower interaction (likes, shares, comments) (Jones & Lee, Citation2022).
These interactions are explained by the speed, interactivity and content of these posts. Therefore, content analysis has been extended to fashion retail brands, where both brands and consumers are highly engaged and show high probabilities of social interaction (Molina-Prados et al., Citation2022).
Recent studies have proposed different approaches to analyse the factors that influence engagement in fashion brands’ Instagram posts. For example, Jones and Lee (Citation2022) identify four groups of factors in the Instagram posts of fashion brands that influence the engagement of followers: characteristics, media types, digital influencers, and messaging strategies used in the posts, while Ha et al. (Citation2017) examine how images in posts are ‘framed’ and its relationship to user perception and brand marketing on Instagram.
The research framework follows these findings to firstly analyse social interaction at the content levels of the posts; secondly, the communication strategy used through the publication of these posts and, thirdly, the characteristics of the offer, as well as other formal aspects of the post. Therefore, the content of the posts allows us to formulate the following hypothesis:
Hypothesis 1 (H1): The content of the posts affects CE
Hypothesis 2 (H2): Brand communication strategies affect CE.
Hypothesis 3 (H3): The post format and the product categories present in the posts generate CE.
H3a) postulates that the number of likes in Primark’s posts is positively related to the communication strategy, while H3b) establishes that the number of comments is positively associated with the company’s interaction on Instagram. These hypotheses seek to deepen our understanding of how different forms of engagement can be linked to specific aspects of Primark’s online presence on Instagram. Together, these variables and hypotheses are designed to provide a comprehensive understanding of the factors that contribute to engagement, differentiating between likes and comments in interactions with the audience. Therefore, engagement in the form of likes allows us to formulate the following hypothesis:
Hypothesis 3a (H3a): Users’ intention to interact with an Instagram account has a positive effect on the number of likes.
Hypothesis 3b (H3b): Users’ intention to interact with an Instagram account has a positive effect on the number of comments.
3. Methodology
3.1. Method
The design of this study is causal, non-experimental and cross-sectional. The study used data from Primark’s official Instagram account. Swedberg recommends the use of a case study when undertaking exploratory work. Similarly, studies related to the fashion sector mainly use content analysis. Therefore, in order to achieve the objectives described above, this study used a quantitative method and, specifically, content analysis, which consists of tracking and drawing conclusions from qualitatively collected data (Serafini & Reid, Citation2023).
This study used data collected from Primark’s official Instagram profile. To evaluate the behavioural engagement (Schreiner et al., Citation2021) of Primark followers, reactions to each post published by Primark on Instagram during 2017, a key period in which Instagram launched carousel posts that allowed multiple photos or videos in a single post (Postbuilder, Citation2023), were recorded. The analysis covered a full year to obtain data across all seasons of the year and to avoid bias from specific seasons or commercial campaigns. Systematic randomised sampling was carried out among the posts published by Primark each day, and the full analysis was carried out with the post chosen for each day. The aim of this sampling was to analyse a single post each day but without following a fixed rule about which post of the day was chosen to ensure that patterns of timing or order of publication within a given day did not influence the observed values.
3.2. Analysis
In the content categorisation process, three different systems were incorporated, highlighting their relevance as key variables in the comprehensive definition of the evaluation. The first, proposed by Çukul (Citation2015), and the second, outlined by Goor (Citation2012), stand as contrasting models, each contributing a unique and significant perspective to the classification. Added to this set of established approaches is a third categorisation, meticulously developed internally by the authors, with a special emphasis on the analysis of the Primark story. This third system stands as an essential variable by faithfully adhering to the fundamental values of integrity, mutual exclusion, objectivity, fidelity and relevance, according to the guidelines proposed by Krippendorff (Citation2004).
Each post was scrupulously evaluated, considering its relevance with respect to the 31 predefined categories and thus highlighting the importance of these variables in the comprehensive analysis. In addition, a precise count of the reactions generated by each post, whether in the form of likes or comments, was carried out, highlighting the relevance of these metrics as significant indicators of the interaction with and reception of the content. It should be noted that in numerically quantifying the comments, objectivity was preserved by not performing a detailed sentiment analysis, given the linguistic diversity of the account analysed and the possible disconnect with the specific themes of the posts.
Prior to the analysis of the data, three coders from outside the research team were trained. The reliability of the coded data was checked and an inter-coder reliability analysis was subsequently carried out, measuring Scott’s Pi coefficient and Cohen’s Kappa coefficient, which relate the coded data to the values that would be obtained randomly. The inter-coder reliability analysis was performed on 50 analysis units, obtaining values in all cases above 0.80 and in most cases with a value of 1, which, however, was expected in all the descriptive categories in which the subjective evaluation did not appear.
For the treatment and analysis of the data, R version 3.5.1 software was used. The statistical decisions were made taking 0.05 as the level of significance. Three levels were used for the statistical decisions: the most demanding with a value of p < .001 (designated ***p), an intermediate value of p < .05 (represented by **p) and a weaker but relevant third value of p < .1 (denoted *p). Thus, a higher level of statistical significance, where p < .001, would reveal that the presence of the analysed variable has a strong and significant relationship with the generation of comments and likes. Frequency tables with the absolute and relative frequencies for the qualitative variables were obtained, as well as tables with the summary statistics –N, median and quartiles– for the quantitative variables. Similarly, bivariate tests were performed for each variable, using the Khi-square test for the qualitative variables and the non-parametric Mann-Witney test for the quantitative variables. Bivariate tests were also carried out between the response variables (comments and likes) and each explanatory variable using the non-parametric Mann-Witney or Kruskal-Wallis test, obtaining the summary statistics N, median and quartiles. Regarding modelling, for each response variable a multiple linear regression model was fitted. The variables were also transformed by logarithms. Thus, the observed variable for the comments was:
and the observed variable for the likes was:
As initial explanatory variables, all those with a p value of less than .1 were included in the bivariate analysis, while the final model was obtained by eliminating all non-significant variables.
4. Results
In order to obtain the maximum information from the coded data, we carried out a bivariate analysis of the impact of each variable of the three proposed models on comments and likes, and a multivariate analysis that helped us strengthen the solidity of the conclusions.
Regarding the variables of the Çukul (Citation2015) model shown in , no variable exceeds the strictest level of significance for likes. In other words, the presence or absence of none of the variables in this first model has a high positive or negative impact that shows a statistically significant difference.
This result, while revealing very little, can largely be attributed to the fact that some of the variables proposed by the model involve a number of observations with very asymmetric results regarding their presence or absence, most notably the case of some variables with an extremely low incidence, such that differences in the medians of likes, although perceptible in some cases, do not show statistical significance. The existence of few observations of some of the Çukul (Citation2015) model variables can be explained by the obviousness of their presence, as is the case, for example, of the variable Product.
On the other hand, regarding the explanatory factors of the comments, on analysing the p-values, some variables show statistically significant results at the highest level: in the positive sense, the variables Product and Promotion, and in the negative sense with an intermediate level of significance the variable Consumer-provided content. In the latter case the divergence between the results obtained, with a negative impact for both comments and likes, is striking (although in this case it should be noted that this variable did not achieve statistical significance at the established levels). This finding is contrary to what was expected, since by generating greater identification and proximity with the user, the variable Consumer-provided content would a priori be expected to generate more likes.
It is worth noting the absence of observations for one of the variables, namely Social responsibility, which a priori could be seen as a candidate for having a greater impact on comments and likes, an absence that should be evaluated if these observations correspond to a deliberate strategy by Primark.
shows the results of the bivariate analysis for the Goor (Citation2012) model, which in terms of statistical significance are slightly higher than those of the Çukul (Citation2015) model.
Table 3. Results of the bivariate analysis: the Çukul (Citation2015) model.
For the comments, two variables show high statistical significance but in opposite directions: in the positive sense Sales response, which when present generates a greater level of interaction, and Symbolism, in negative, which when present produces fewer comments. At the intermediate level of significance, the variable Relationship shows a positive impact; however, it is worth noting the opposite negative impact of this same variable on the generation of likes, although not statistically significant. And along the same line, beyond statistical stringency, Emotions yielded an unexpected result: its presence generated fewer comments than its absence.
Regarding the number of likes in the Goor (Citation2012) model, only one variable, Symbolism, achieves the highest established level of statistical significance. Again, it is the absence of this variable that is associated with a greater number on average of likes. A second variable, Sales response, achieves a level of statistical significance of *p, which in this case, is in the positive sense.
With respect to the statistical results of our own model (), the number of variables showing significant results at the statistical level is greater than the two previous models.
Table 4. Results of the bivariate analysis: the Goor (Citation2012) model.
Regarding the comments, the variables that explain a difference in the median of responses, with the highest level of statistical significance, are of two types. First, those whose presence increases the number of comments, namely Product only, Studio and For women, and, secondly, those whose absence increases the number of comments: People, People’s faces and Location. With an intermediate level of statistical significance, the variable Username mentioned generates fewer comments when absent. This result is striking because it is different to what was expected, since we might think that its presence would lead to greater empathy through identification with the user mentioned. This variable also has less impact on the likes, although without a notable level of significance.
The variables that generate a greater number of likes, with a high level of statistical significance, are Product only, Studio, Video and For women, and at an intermediate level of significance the variable Series of images. Statistically significant negative variables include People, People’s faces, Location and For kids. As discussed in the previous paragraph, those variables with different results to those expected are striking, such as People and People’s faces, which, far from generating greater empathy, result in less engagement.
It should be noted that certain variables have contrasting impacts on comments and likes, such as the presence of Video, which impacts positively and significantly (**p) on likes and negatively on comments, although in this case without statistical significance.
In the multivariate modelling, the relationship between the comments ( and ) and likes ( and ) and the set of variables considered significant in the previous bivariate test are analysed. In general terms, the multivariate modelling confirms the analyses and implications mentioned in the previous paragraphs.
Table 5. Results of the bivariate analysis: own model.
Table 6. Results of the multivariate analysis for comments.
Table 7. Results of the multivariate analysis considering the three models for comments.
Table 8. Results of the multivariate analysis: Goor (Citation2012) model and our own model for likes.
Regarding comments (), it should be noted that of the Çukul (Citation2015) model variables, only Promotion achieves statistical significance and does so at the maximum level defined and in a positive sense. Two of the Goor (Citation2012) model variables, Relationship and Sales response, have a positive impact on comments, the former with an intermediate level of statistical significance and the latter with a high level. And again, as in the bivariate analysis, our own model yields a greater number of significant variables, with the variables People, Product only and For women having a positive impact, while the presence of the variable People’s faces has a negative impact. Similarly, our model yields a significantly higher result for R2.
In a second version of the multivariate modelling, simultaneously considering the three models and eliminating the variables that do not reach the minimum level of statistical significance required in the analysis, the explanatory power increases ().
Regarding likes, in the multivariate analysis considering each model independently (), no variable from the Çukul (Citation2015) model exceeds the established filters, while in the case of the Goor (Citation2012) model, only Sales response, with a very low R2 value and a positive impact, meets the conditions of significance at the established statistical level. The results improve for our own model, with three variables exceeding the imposed filters of statistical significance: with a positive impact, For women, at a level of significance of ***p, and Other products at the intermediate level of significance of **p; and, with a negative impact, People’s faces, as occurred for comments.
In the simultaneous consideration of the three models for likes (), the same variables that appeared when considering each model independently reappear, confirming the strength of statistical significance at the maximum level established: People’s faces, whose presence it is confirmed has a negative impact on the interaction with followers, while the variables For women and Other products have a positive impact at a high level of statistical significance of ***p. Sales response also has a positive impact, though with a lower level of significance of *p.
5. Discussion and managerial implications
In order to rigorously determine the variables that result in behavioural engagement, this study used three models that group the variables into categories and analysed their impact on the response variables. This study provides an analysis of the impact on engagement on Instagram with an unusually high number of variables. The results contribute to the previous literature and, at the same time, clarify aspects in this emerging field of research. This research also provides relevant information for marketing managers, since determining which factors drive user engagement with the brand has been the subject of interest in recent years for both academics and marketing specialists. Compared to other social networks, Instagram turns out to be the most effective in generating user engagement (Stein et al., Citation2021).
The results reveal that, in line with the contributions of Jones and Lee (Citation2022), posts attract different levels of engagement and in different senses (positive/negative) depending on the presence or not of variables such as Product, People, People’s faces, Sales response or For women, in the case of comments, and in the case of the likes, Sales response, People’s faces, For women and Other products. This information suggests different, wide-ranging managerial implications. For example, although previous research has shown consumers’ rejection of advertisements, promotions increase participation on Instagram. Thus, we observe that, in terms of communication strategies, the factor that generates greater interaction, both in the form of comments and likes, is the presence of the variable Sales response in the communication strategy. Thus, those posts intended to generate an immediate sale achieve greater interaction, revealing this to be the communication strategy to emphasise if the objective is to generate more comments. Thus, according to the results, we suggest to digital marketing managers that to encourage more productive, positive engagement in terms of comments, it is important to consider posts that pursue immediate sales and to avoid consumer-generated content, which, contrary to expectations, does not seem to generate empathy or adhesion, an observation that is in line with the study by Bonilla Quijada et al. (Citation2022).
The result of the negative impact on both comments and likes of the variable People’s faces is striking, a finding that casts doubt on a very common resource as an element in Instagram images and that is often justified in terms of the humanisation of posts regarding approaching users but which seems not to generate the expected responses in terms of engagement. This result is in contrast to the study by Lindell (Citation2019), which confirms the opposite, and that of Bakhshi et al. (Citation2014), which concludes that photos with faces are more likely to receive a like. Nevertheless, the variable People has a significant positive impact on comments. In this regard, several studies have attempted to determine the impact on self-esteem of images with the presence of People (Cohen et al., Citation2017; Tiggemann & Zinoviev, Citation2019). Of these, it is worth mentioning in relation to our study that of Tiggemann et al. (Citation2018), who found that the number of likes does not affect body dissatisfaction. The finding of the positive impact on both comments and likes of the variable For women is also notable, clearly indicating a bias in favour of posts that present products for women. Similarly, the positive impact of the presence of the variable Product only on comments is striking.
However, digital communication managers must give prominence to the product that generates greater interest among users by giving it a dominant position, especially those aimed at women. The presence of the personal element is positive as long as it does not focus on the faces of people if the aim is to generate greater engagement.
Therefore, this study will help fast fashion brand managers and decision makers to implement marketing decisions after evaluating the engagement pursued by the brand and to understand the impact of its activity on social media in order to improve the brand’s presence and image with the aim of contributing to the company’s financial results. Managing Instagram well is an important opportunity for those organisations that wish to incorporate this marketing tool into their strategy, since it can contribute very effectively to strengthening ties with their users. Followers’ attention can be attracted through content, as this study shows, by prioritising those posts that show the product if the aim is to achieve greater engagement in the form of comments. Through a communication strategy that seeks an immediate sales response, greater engagement is also achieved in terms of both comments and likes by avoiding the presence of people’s faces and encouraging the presentation of products for women.
6. Conclusions
The primary purpose of this study was to explore the relationships between the content, visual elements, communication strategies, post formats and product categories in Instagram posts and their impact on engagement. Focusing on the context of fast fashion, this study aims to make a significant contribution to the understanding of the phenomenon of engagement on Instagram. In today’s era, where social media marketing is of central importance to fashion companies, effective message management on these platforms becomes crucial to ensure connection with users.
Through this research, we have not only identified but also quantified the elements of posts that influence the engagement of brands with users. The correct management of content, product categories, visual elements and post formats emerges as a determining factor in ensuring a successful social media presence. The results obtained contribute to the understanding of the aspects of Primark’s Instagram posts that generate meaningful engagement with users, differentiating those elements that have a positive or negative impact on the responses through comments and likes.
It is important to note that some variables that, a priori, would be expected to show differences in the generation of engagement do not always meet this expectation. This finding raises the question of whether companies are reformulating their digital communication strategies, prioritising elements that generate greater engagement. Moreover, the identification of variables that exhibit asymmetric responses in comments and likes opens up the possibility of evaluating whether these differential impacts can be used to reformulate digital communication strategies.
However, it is surprising that none of the variables studied in any of the categorisation models used in this study generated more comments but fewer likes, although the opposite was quite often the case.
The results obtained suggest that the measurement of engagement, as applied to market research, is of great commercial importance. However, to date, they point to an under-exploitation of the digital potential for engagement in the sector, with companies mainly managing their social media to disseminate information. Furthermore, as Schultz (Citation2016) highlights, using a social network as a communication channel requires the integration of business knowledge supported by a customer relationship management system. This highlights the need for a more comprehensive strategic approach to social media management to optimise engagement potential in the fast fashion sector.
7. Limitations of the research
Despite the important contributions of this study regarding the understanding of those variables that generate greater engagement, this analysis has limitations that present possibilities for fruitful future research. An important limitation of the present study is that the analysis of the comments was based on quantitative and not qualitative aspects, which would provide valuable information in the analysis of engagement. Another limitation is that it does not address how user engagement translates into brand sales.
8. Future lines of research
In order to generalise and confirm the results obtained in this case study, following Conde and Casais (Citation2023), this study must be replicated with a larger sample of Instagram accounts of mass market organisations. Other interesting aspects would include extending the study over time by aggregating the data for more years; determining behavioural engagement by investigating other social networks; extending the study to organisations in other sectors; broadening this study by analysing behavioural engagement in the luxury sector; reviewing the variables used in this study; and, incorporating qualitative and not just quantitative of the content of the comments. Another point for improvement would be to answer the question of how engagement can have an impact on improving the brand’s financial results, the most ambitious of which would be to study how the interactions that reflect engagement translate into effective purchasing decisions.
Disclosure statement
No potential conflict of interest was reported by the author(s).
Data availability statement
The data from which this work has been developed is available.
Additional information
Notes on contributors
María Bonilla-Quijada
María Bonilla-Quijada, PhD is Professor of Economics at the Abat Oliba CEU University, and collaborating teacher at de University of Barcelona. Focus your research on business through social networks ([email protected]).
Josep Lluís Del Olmo-Arriaga
Josep Lluís Del Olmo-Arriaga, PhD is professor of Marketing at the Abat Oliba CEU University. His research is focuses in fashion.
David Adreu Domingo
David Adreu Domingo, PhD is professor of Communication at the Abat Oliba CEU University. His research is focuses in social media.
Joan Ripoll-i-Alcon
Joan Ripoll-i-Alcon, PhD is professor of Econmics at the Abat Oliba CEU University. His research is focuses in business.
References
- Armstrong, K., Rutter, C., Helberger, E., & Tormey, A. (2018). Drivers, constraints, and consequences of consumer-brand engagement in the luxury fashion sector. In Digital marketing strategies for fashion and luxury brands (pp. 1–14). IGI Global.
- Bakhshi, S., Shamma, D. A., & Gilbert, E. (2014). Facesengage us: Photos with faces attract more likes and comments on Instagram. In Proceedings of the SIGCHI Conference on Human Factors in Computing Systems (pp. 965–974).
- Bhattacharya, A. (2023). Parasocial interaction in social media influencer-based marketing: An SEM approach. Journal of Internet Commerce, 22(2), 272–14. https://doi.org/10.1080/15332861.2022.2049112
- Bonilla, M. D. R., del Olmo Arriaga, J. L., & Andreu, D. (2019). The interaction of Instagram followers in the fast fashion sector: The case of Hennes and Mauritz (H&M). Journal of Global Fashion Marketing, 10(4), 342–357. https://doi.org/10.1080/20932685.2019.1649168
- Bonilla Quijada, M. D. R., Perea Muñoz, E., Corrons, A., & Olmo-Arriaga, J. L. (2022). Engaging students through social media. Findings for the top five universities in the world. Journal of Marketing for Higher Education, 32(2), 197–214. https://doi.org/10.1080/08841241.2020.1841069
- Brodie, R. J., Hollebeek, L. D., Jurić, B., & Ilić, A. (2011). Customer engagement: Conceptual domain, fundamental propositions, and implications for research. Journal of Service Research, 14(3), 252–271. https://doi.org/10.1177/1094670511411703
- Brodie, R. J., Ilic, A., Juric, B., & Hollebeek, L. (2013). Consumer engagement in a virtual brand community: An exploratory analysis. Journal of Business Research, 66(1), 105–114. https://doi.org/10.1016/j.jbusres.2011.07.029
- Buhalis, D., & Mamalakis, E. (2015). Social media return on investment and performance evaluation in the hotel industry context. In Information and communication technologies in tourism 2015 (pp. 241–253). Springer.
- Cao, D., Meadows, M., Wong, D., & Xia, S. (2021). Understanding consumers’ social media engagement behaviour: An examination of the moderation effect of social media context. Journal of Business Research, 122, 835–846. https://doi.org/10.1016/j.jbusres.2020.06.025
- Chugh, R., Patel, S. B., Patel, N., & Ruhi, U. (2019). Likes, comments and shares on social media: Exploring user engagement with a state tourism Facebook page. International Journal of Web Based Communities, 15(2), 1. https://doi.org/10.1504/IJWBC.2019.10020618
- Cohen, R., Newton-John, T., & Slater, A. (2017). The relationship between Facebook and Instagram appearance-focused activities and body image concerns in young women. Body Image, 23, 183–187. https://doi.org/10.1016/j.bodyim.2017.10.002
- Conde, R., & Casais, B. (2023). Micro, macro and mega-influencers on Instagram: The power of persuasion via the parasocial relationship. Journal of Business Research, 158, 113708. https://doi.org/10.1016/j.jbusres.2023.113708
- Çukul, D. (2015). Fashion marketing in social media: Using Instagram for fashion branding (no. 2304324). In Proceedings of the Proceedings of the 1st Business & Management Conference (pp. 116–129). International Institute of Social and Economic Sciences.
- Cvijikj, I. P., & Michahelles, F. (2013). Online engagement factors on Facebook brand pages. Social Network Analysis and Mining, 3(4), 843–861. https://doi.org/10.1007/s13278-013-0098-8
- Gaber, H. R., Elsamadicy, A., & Wright, L. T. (2021). Do Instagram fan pages develop consumer–brand relationships? Evidence from the telecommunications industry. Cogent Business & Management, 8(1), 1884344. https://doi.org/10.1080/23311975.2021.1884344
- Goor, M. (2012). Instagram: A content analysis into marketing on Instagram [Master’s thesis]. University of Amsterdam.
- Gutierrez, A., Punjaisri, K., Desai, B., Syed Alwi, S. F., O’Leary, S., Chaiyasoonthorn, W., & Chaveesuk, S. (2023). Retailers, don’t ignore me on social media! The importance of consumer-brand interactions in raising purchase intention-Privacy the Achilles heel. Journal of Retailing and Consumer Services, 72, 103272. https://doi.org/10.1016/j.jretconser.2023.103272
- Ha, Y. I., Kwon, S., M., & Joo, J. (2017). Cha “Fashion conversation data on Instagram” [Paper presnetation]. Eleventh International AAAI Conference on Web and Social Media.
- Hoffman, D. L., & Fodor, M. (2010). Can you measure the ROI of your social media marketing? MIT Sloan Management Review, 52(1), 41–49.
- Hollebeek, L. D. (2011). Demystifying customer brand engagement: Exploring the loyalty nexus. Journal of Marketing Management, 27(7-8), 785–807. https://doi.org/10.1080/0267257X.2010.500132
- Hollebeek, L. D., Glynn, M. S., & Brodie, R. J. (2014). Consumer brand engagement in social media: Conceptualization, scale development and validation. Journal of Interactive Marketing, 28(2), 149–165. https://doi.org/10.1016/j.intmar.2013.12.002
- Hu, Y., Manikonda, L., & Kambhampati, S. (2014). What we Instagram: A first analysis of Instagram photo content and user types. Proceedings of the International AAAI Conference on Web and Social Media, 8(1), 595–598. https://doi.org/10.1609/icwsm.v8i1.14578
- Ibrahim, B., & Aljarah, A. (2023). The era of Instagram expansion: Matching social media marketing activities and brand loyalty through customer relationship quality. Journal of Marketing Communications, 29(1), 1–25. https://doi.org/10.1080/13527266.2021.1984279
- Jaakonmäki, R., Müller, O., & Vom Brocke, J. (2017). The impact of content, context, and creator on user engagement in social media marketing. In Proceedings of the 50th Hawaii International Conference on System Sciences (Vol. 50, pp. 1152–1160). IEEE Computer Society Press. https://doi.org/10.24251/HICSS.2017.136
- Jamil, K., Dunnan, L., Gul, R. F., Shehzad, M. U., Gillani, S. H. M., & Awan, F. H. (2021). Role of social media marketing activities in influencing customer intentions: A perspective of a new emerging era. Frontiers in Psychology, 12, 808525. https://doi.org/10.3389/fpsyg.2021.808525
- Jin, S. V., & Ryu, E. (2019). Instagram fashionistas, luxury visual image strategies and vanity. Journal of Product & Brand Management, 29(3), 355–368. https://doi.org/10.1108/JPBM-08-2018-1987
- Jinyoung Yoo, J., Choi, S., & Song, H. (2023). Effect of brand prominence on fashion advertising images on Instagram: A computational analysis. International Journal of Advertising, 42(2), 384–407. https://doi.org/10.1080/02650487.2022.2073133
- Jones, A. R., & Lee, S. E. (2022). Factors influencing engagement in fashion brands’ Instagram posts. Fashion Practice, 14(1), 99–123. https://doi.org/10.1080/17569370.2021.1938820
- Kim, K., Chung, T. L. D., & Fiore, A. M. (2023). The role of interactivity from Instagram advertisements in shaping young female fashion consumers’ perceived value and behavioral intentions. Journal of Retailing and Consumer Services, 70, 103159. https://doi.org/10.1016/j.jretconser.2022.103159
- Kim, Y. H., Kim, D. J., & Wachter, K. (2013). A study of mobile user engagement (MoEN): Engagement motivations, perceived value, satisfaction, and continued engagement intention. Decision Support Systems, 56, 361–370. https://doi.org/10.1016/j.dss.2013.07.002
- Kraaijenbrink, J. (2019). Why is Primark so successful? The power of alignment. Forbes. https://www.forbes.com/sites/jeroenkraaijenbrink/2019/01/22/why-isprimark-so-successful-the-power-of-alignment/?sh=24416d15769d
- Krippendorff, K. (2004). Reliability in content analysis. Human Communication Research, 30(3), 411–433. https://doi.org/10.1111/j.1468-2958.2004.tb00738.x
- Lee, D., Hosanagar, K., & Nair, H. S. (2018). Advertising content and consumer engagement on social media: Evidence from Facebook. Management Science, 64(11), 5105–5131. https://doi.org/10.1287/mnsc.2017.2902
- Lee, Y. J. (2023). Language learning affordances of Instagram and TikTok. Innovation in Language Learning and Teaching, 17(2), 408–423. https://doi.org/10.1080/17501229.2022.2051517
- Lindell, A. K. (2019). Left cheek poses garner more likes: The effect of pose orientation on Instagram engagement. Laterality, 24(5), 600–613. https://doi.org/10.1080/1357650X.2018.1556278
- Liu, L., Liu, R., Lee, M., & Chen, J. (2019). When will consumers be ready? A psychological perspective on consumer engagement in social media brand communities. Internet Research, 29(4), 704–724. https://doi.org/10.1108/IntR-05-2017-0177
- Ma, L., Ou, W., & Lee, C. S. (2022). Investigating consumers’ cognitive, emotional, and behavioral engagement in social media brand pages: A natural language processing approach. Electronic Commerce Research and Applications, 54, 101179. https://doi.org/10.1016/j.elerap.2022.101179
- Malthouse, E. C., Haenlein, M., Skiera, B., Wege, E., & Zhang, M. (2013). Managing customer relationships in the social media era: Introducing the social CRM house. Journal of Interactive Marketing, 27(4), 270–280. https://doi.org/10.1016/j.intmar.2013.09.008
- McKinsey & Company. (2019). The state of fashion 2019. https://www.mckinsey.com/industries/retail/our-insights/the-state-of-fashion-2019-a-year-of-awakening
- Milanesi, M., Kyrdoda, Y., & Runfola, A. (2022). How do you depict sustainability? An analysis of images posted on Instagram by sustainable fashion companies. Journal of Global Fashion Marketing, 13(2), 101–115. https://doi.org/10.1080/20932685.2021.1998789
- Molina-Prados, A., Muñoz-Leiva, F., & Prados-Peña, M. B. (2022). The role of customer brand engagement in the use of Instagram as a “shop window” for fashion-industry social commerce. Journal of Fashion Marketing and Management: An International Journal, 26(3), 495–515. https://doi.org/10.1108/JFMM-12-2020-0275
- Mir, I. A. (2023). Self-escapism motivated online shopping engagement: A determinant of users’ online shopping cart use and buying behavior. Journal of Internet Commerce, 22(1), 40–73. https://doi.org/10.1080/15332861.2021.2021582
- Na, Y., & Kim, J. (2019). Sensitivity and response to keywords of users according to the types of fashion publications Instagram: Focused on Korean fashion brands. International Journal of Clothing Science and Technology, 32(1), 23–36. https://doi.org/10.1108/IJCST-03-2018-0032
- O’Brien, H. L. (2010). The influence of hedonic and utilitarian motivations on user engagement: The case of online shopping experiences. Interacting with Computers, 22(5), 344–352. https://doi.org/10.1016/j.intcom.2010.04.001
- Pansari, A., & Kumar, V. (2017). Customer engagement: The construct, antecedents, and consequences. Journal of the Academy of Marketing Science, 45(3), 294–311. https://doi.org/10.1007/s11747-016-0485-6
- Peters, K., Chen, Y., Kaplan, A. M., Ognibeni, B., & Pauwels, K. (2013). Social media metrics – A framework and guidelines for managing social media. Journal of Interactive Marketing, 27(4), 281–298. https://doi.org/10.1016/j.intmar.2013.09.007
- PostBuilder. (2023). Instagram’s history: Full evolution of Instagram timeline (2023). https://postbuilderapp.com/blog/instagram-history-and-updated-timeline
- Ramesh, D. N. (2022). Importance of brand strategy in stakeholder communication and satisfaction (A study on fashion retail company’s stakeholder management)[Doctoral dissertation]. Dublin, National College of Ireland.
- Romero Saletti, S. M., Van den Broucke, S., & Van Beggelaer, W. (2022). Understanding motives, usage patterns and effects of Instagram use in youths: A qualitative study. Emerging Adulthood, 10(6), 1376–1394. https://doi.org/10.1177/21676968221114251
- Sashi, C. M. (2012). Customer engagement, buyer–seller relationships, and social media. Management Decision, 50(2), 253–272. https://doi.org/10.1108/00251741211203551
- Schreiner, M., Fischer, T., & Riedl, R. (2021). Impact of content characteristics and emotion on behavioral engagement in social media: Literature review and research agenda. Electronic Commerce Research, 21(2), 329–345. https://doi.org/10.1007/s10660-019-09353-8
- Schultz, C. D. (2016). Insights from consumer interactions on a social networking site: Findings from six apparel retail brands. Electronic Markets, 26(3), 203–217. https://doi.org/10.1007/s12525-015-0209-7
- Serafini, F., & Reid, S. F. (2023). Multimodal content analysis: Expanding analytical approaches to content analysis. Visual Communication, 22(4), 623–649. https://doi.org/10.1177/1470357219864133
- Statista. (2023). Number of Instagram users worldwide from 2020 to 2025. https://www.statista.com/statistics/183585/instagram-number-of-global-users/
- Stein, J. P., Krause, E., & Ohler, P. (2021). Every (Insta) Gram counts? Applying cultivation theory to explore the effects of Instagram on young users’ body image. Psychology of Popular Media, 10(1), 87–97. https://doi.org/10.1037/ppm0000268
- Stringer, T. (2023). Ethical consumption in a fast fashion world [Doctoral dissertation]. Queensland University of Technology.
- Syrdal, H. A., & Briggs, E. (2018). Engagement with social media content: A qualitative exploration. Journal of Marketing Theory and Practice, 26(1-2), 4–22. https://doi.org/10.1080/10696679.2017.1389243
- Tang, R. Y. S., & Raheem, M. (2023). The impact of Instagram marketing on sale in the fashion industry. Journal of Applied Technology and Innovation, 7(2), 29.
- Tiggemann, M., Hayden, S., Brown, Z., & Veldhuis, J. (2018). The effect of Instagram “likes” on women’s social comparison and body insatisfaction. Body Image, 26, 90–97. https://doi.org/10.1016/j.bodyim.2018.07.002
- Tiggemann, M., & Zinoviev, K. (2019). The effect of #enhancement-free Instagram images and hashtags on women’s body image. Body Image, 31, 131–138. https://doi.org/10.1016/j.bodyim.2019.09.004
- Ting, D. H., Abbasi, A. Z., & Ahmed, S. (2021). Examining the mediating role of social interactivity between customer engagement and brand loyalty. Asia Pacific Journal of Marketing and Logistics, 33(5), 1139–1158. https://doi.org/10.1108/APJML-10-2019-0576
- Valentini, S., Montaguti, E., & Neslin, S. A. (2018). Decision process evolution in customer channel choice. Journal of Marketing, 75(6), 72–86. https://doi.org/10.1509/jm.09.0362
- Van Doorn, J., Lemon, K. N., Mittal, V., Nass, S., Pick, D., Pirner, P., & Verhoef, P. C. (2010). Customer engagement behavior: Theoretical foundations and research directions. Journal of Service Research, 13(3), 253–266. https://doi.org/10.1177/1094670510375599
- Van Tonder, E., & Petzer, D. J. (2018). The interrelationships between relationship marketing constructs and customer engagement dimensions. The Service Industries Journal, 38(13–14), 948–973. https://doi.org/10.1080/02642069.2018.1425398
- Vivek, S. D., Beatty, S. E., Dalela, V., & Morgan, R. M. (2014). A generalized multidimensional scale for measuring consumer engagement. Journal of Marketing Theory and Practice, 22(4), 401–420. https://doi.org/10.2753/MTP1069-6679220404
- Xue, Z., Li, Q., & Zeng, X. (2023). Social media user behavior analysis applied to the fashion and apparel industry in the big data era. Journal of Retailing and Consumer Services, 72, 103299. https://doi.org/10.1016/j.jretconser.2023.103299