Figures & data
Table 1. List of abbreviations related to modeling and data privacy protection techniques.
Table 2. Data security vs. privacy: definitions, risks, and technical solutions.
Figure 2. An illustration of (A) central differential privacy (CDP) and (B) local differential privacy (LDP). In CDP, each farm shares its own input data (e.g., soil quality, concentration of indicator microorganisms) to the central server. The data are stored at the central server and the model performance should be indistinguishable if the data from any single farm is modified. In LDP each individual farm does not share the original data, but instead share the privatized data that reveal little information about the farm.
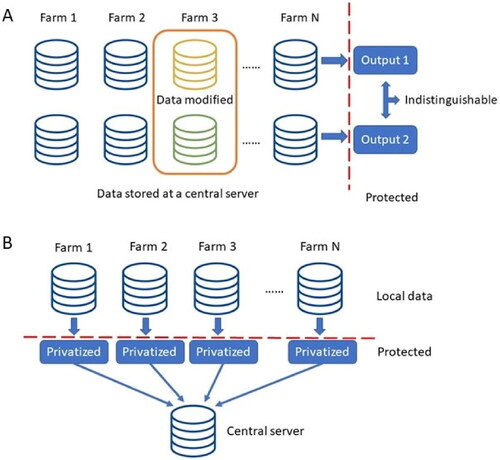
Figure 3. An illustration of the privatized federated Learning framework. Each client maintains its own local data. The clients use the local data to perform local updates which are then sent to the central server in a privatized/encrypted manner. The central server aggregates the local updates from the clients to obtain a new global model which is shared with the clients for the next iteration.

Figure 4. An illustration of various data modification strategies for pathogen data, location data, and land use data collected on multiple farms, depending on the data categories and privacy sensitivity. In this example, the presence of pathogen is flipped with a certain probability to grant the data provider plausible deniability. The GPS locational data can be privatized by adding with a random noise (using local or central differential privacy approaches). The land use data is public, and thus needs no privatization.

Table 3. Examples of food safety data available for various compartments of a simplified food system, including possible data uses and privacy concerns.