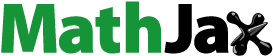
ABSTRACT
Objective
Previous studies have demonstrated that making a target larger is sufficient to compensate for the inaccuracy of gaze-based target selection. However, few studies systemically examined the individual contribution of the target’s motor and visual space size in gaze selection. We investigated how the motor and visual space size affect gaze-based target selection.
Method
Experiment 1 was used to examine the effect of the invisible expanded motor space in a 2D target selection task under hand-based and gaze-based control modes. Experiment 2 was used to examine the impact of the invisible expanded motor space on gaze-based target selection performance with different visual-space sizes.
Results
As the motor-space size increased, participants selected targets more efficiently and experienced less frustration and temporal demand; these effects were more pronounced in the gaze-based control mode. The selection was faster in the group with a larger visual-space size. Moreover, there were significant interactions between visual-space size and motor-space size on the throughput of gaze-based selection, which refers to the efficiency of input method that containing the accuracy and speed. These interactions also significantly affected endpoint distance variation and effort demand.
Conclusions
The motor-space size, even invisible, is the determining factor in facilitating the gaze selection performance. Additionally, the size of the visual space has a feedforward effect on selection speed and modulates the effect of motor space. These findings provide insights into the contribution of these two spaces and a new perspective to optimize the gaze-based interface.
Key Points
What is already known about this topic:
A common way to enhance gaze positioning accuracy is to enlarge the region where the gaze resides in the motor and visual space. However, this method also brings visual interference problems.
The invisible motor-only expansion could facilitate the one-dimensional (1D) gaze-based target selection performance and 2D mouse pointing time.
Visual feedforward and feedback contribute to the perception of target size, resulting in improving the efficiency of hand-controlled pointing.
What this topic adds:
The motor-space size, even invisible, is the determining factor in facilitating the performance of 2D gaze-based target selection, and it affects more in gaze-controlled mode than in hand-controlled mode.
The design in the visual space of a target could provide the user with feedforward and feedback information that helps improve the efficiency and user experience of 2D gaze-based target selection.
Visual-space size could also modulate the effects of motor-space size on the stability and throughput of 2D gaze-based selection.
Introduction
Eye or gaze movement could reveal the interest or intent of the operator during interactions (Borji et al., Citation2015; Kleinke, Citation1986; Rantala et al., Citation2020). Previous research has demonstrated that gaze, as an input channel, could naturally and quickly move a cursor to a target on a two-dimensional (2D) display (Aoki et al., Citation2008; Hayhoe & Ballard, Citation2005; Rayner, Citation1998; Squire et al., Citation2013). However, due to the involuntary eye jitter, users encounter troubles when positioning the gaze-controlled cursor correctly on small targets (Canare et al., Citation2015; Feng Cheng-Zhi & Hui, Citation2003; Hyrskykari et al., Citation2012; Sibert et al., Citation2001; Stellmach & Dachselt, Citation2013; Vertegaal, Citation2008). This is one of the most critical problems in gaze-based interaction.
Increasing the target size to improve gaze positioning accuracy
Researchers have shown that increasing the size of targets can help address the inaccuracy of gaze positioning (R. Bates & Istance, Citation2002; M. Kumar et al., Citation2007; Miyoshi & Murata, Citation2001). The most common way to achieve this is to expand the location, simultaneously in both motor and visual space, where the gaze resides, as these two spaces cannot be physically separated in a gaze-based interface. There are two ways to activate the expansion: using a designated gaze behaviour (Choi et al., Citation2020; Hansen et al., Citation2008; C. Kumar et al., Citation2017; Morimoto et al., Citation2018; Špakov & Miniotas, Citation2005) or using hand-controlled behaviour (R. Bates & Istance, Citation2002; M. Kumar et al., Citation2007). For instance, in Morimoto et al. (Citation2018), the new eye typing interface activated the magnification of a key at the onset of fixation. However, this approach may inadvertently introduce visual distractions. As one types a word or sentence, selecting different keys on the keyboard gives rise to many visual stimuli tied to the enlargement and reduction of each key. These stimuli invariably influence the stability of the user’s gaze, with the impact being notably accentuated in instances of calibration deviation. In M. Kumar et al. (Citation2007), the EyePoint system magnified the area around the gaze when a hotkey was pressed. Such approaches that require additional hand-controlled devices can be time-consuming due to the two-step design.
In contrast, increasing the target size only in motor space is more friendly to gaze-based interaction. Here, the motor space of a target refers to the effective area that a user could interact with (such as fire a click event) when selecting or manipulating the target on the interface (Cockburn & Brock, Citation2006). As shown in , Miniotas et al. (Citation2004) invisibly expanded the target in motor space and kept the visual space unchanged. The results convinced the motor-only expansion could facilitate the one-dimensional (1D) gaze-based target selection in speed and accuracy. However, the effect of the invisible expanded motor space on 2D gaze-based target selection is still unclear, as is the role of visual space. Both spaces have been shown to play an essential role in conventional interfaces individually.
Figure 1. Schematic diagram of motor and visual space of the target. The dotted rectangle represents the invisible expanded motor space. The solid line rectangle represents the non-expanded visual space. Note. Adapted from ”eye gaze interaction with expanding targets”, by miniotas, D., Špakov, O., and MacKenzie, I. S., 2004, in CHI’04 extended abstracts on human factors in computing systems, p. 1256, (https://doi.Org/10.1145/985921.986037). Reprinted with permission.

The impact of motor and visual space on the conventional interface
Studies of mouse pointing have provided evidence for the crucial role of motor space in 2D target selection performance (Usuba et al., Citation2018, Citation2020). For example, Usuba et al. (Citation2018) investigated the effects of the difference between the motor and visual space sizes on the performance of mouse pointing. They highlighted the motor target width before each trial, then hid it after a fixed interval. This simulates a condition where users interact with familiar applications and remember the motor width of these applications. Results showed that the movement time depended on the motor width.
The impact of visual space expansion on traditional 2D target selection performance has been a subject of considerable debate. Gutwin (Citation2002), for instance, identified a detrimental effect, known as the “Gutwin effect”, associated with using a fisheye lens. Conversely, McGuffin and Balakrishnan (Citation2005) underscored the positive implications of visual space expansion, demonstrating that displaying additional visual details with desired targets could enhance performance. Further supporting this perspective, Cockburn and Brock (Citation2006) discovered that visual expansion for small targets with unaltered motor space produces performance gains comparable to motor expansion. These diverse findings underscore the complexity of this research area and highlight the need for further investigation.
The effect of visual feedforward and feedback on target size perception
Previous research demonstrated the critical role of the visual feedforward mechanism in selection performance when target expansion was applied to both the motor and visual spaces (Guillon et al., Citation2014, Citation2015; Su et al., Citation2014). Feedforward is a concept that refers to the use of information that informs the user of available commands and anticipates the results of the action before it is performed (Delamare et al., Citation2016; Djajadiningrat et al., Citation2002; Vermeulen et al., Citation2013). Specifically, the target’s shape or size provides the user with visual feedforward information that could be used to anticipate and plan upcoming movements, which helps improve pointing efficiency. For example, Guillon et al. (Citation2015) proposed that the visible motor space cell served as the visual feedforward information to help users take full advantage of the effective (motor) space expansion, resulting in faster and less error-prone distant pointing. However, when the cell border was invisible, Guillon et al. (Citation2015) found that participants perceived the target size based on the target’s shape in visual space to optimize their pointing. More importantly, Seidler et al. (Citation2004) found that larger targets relied more on feedforward control in hand-controlled joystick movements, increasing speed and accuracy.
Visual feedback is a crucial component in user interface design, significantly influencing pointing performance and interaction efficiency, particularly concerning target size and expansion. McGuffin and Balakrishnan (Citation2005) discovered that users could utilize visual sensory feedback during subsequent movements, enhancing user performance in button selection with a puck by capitalizing on target expansion. A subsequent study by Zhai et al. (Citation2003) revealed that performance improvement persisted even when users were uncertain about the occurrence of target expansion, suggesting that users were adjusting their actions in response to visual feedback rather than merely planning their movements in anticipation of target expansion.
Research questions and solutions
However, to our knowledge, few studies have systemically examined the contribution of the motor and visual spaces in gaze-based target selection. Unlike hand-controlled interaction, gaze in an eye-controlled interface has two roles: perception and interaction. So, it could not be a supportive role as it does during hand-controlled interaction (Bieg et al., Citation2010; Souto et al., Citation2021). All these indicated that the visual information from the target screen space may have a unique effect on the gaze interaction. Therefore, what is the role of the invisible expanded motor space in gaze-based interaction compared to conventional interaction? Does visual space influence the effect of motor space, given that the visual channel has a dual role during the interaction? Answering these questions may help optimize the gaze-based interface design and facilitate interaction performance.
This study aimed to investigate how the target’s motor and visual space size affect gaze-based target selection. We performed two experiments. In experiment 1, we examined the effect of the invisible expanded motor space in a 2D target selection task under hand-based and gaze-based control modes. In experiment 2, we investigated the contribution of the invisible expanded motor space to gaze-based target selection performance with different visual-space sizes.
We developed a visual-space-based highlighting feedback technique for the present study. This design could help participants perceive and take advantage of the hidden motor-space expansion without overloading the visual channel. As shown in , the dotted line around the target circle is the boundary of motor space, which was invisible to the participant. Once the gaze (or hand) controlled cursor crosses the border, the target’s visual space changes colour from yellow to red to inform participants that they have captured the target. Su et al. (Citation2014) employed a similar feedback design. As shown in ,they highlighted the captured target instead of displaying the fan-shaped activation area (dotted line) to reduce the visual distraction in a mouse-controlled target selection task. The results showed that such target-based highlighting yielded the best performance.
Figure 2. Schematic diagrams of (a) visual-space-based highlighting feedback. The dotted line indicates the boundary of motor space, which is not visible to the participants in the experiment. The target visual space would change color (from yellow to red) when the cursor moves into the motor space. (b) target-based highlighting. The dark orange-filled circle indicated the captured target and the fan-shaped activation area (dotted line) was not visible to the participants.
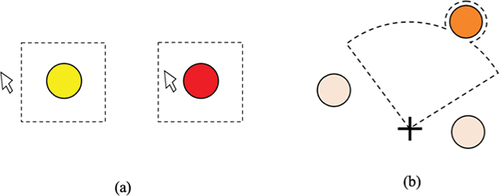
Experiment 1
Experiment 1 was used to investigate the effects of the invisible expansion of motor space on the target selection performance under the gaze-based and hand-controlled modes.
Method
Participants
Eighty college participants (40 males, 40 females) aged 20.0 years old (SD = 1.8 years) were recruited for this experiment. All participants had normal or corrected to normal vision, were right-handed based on self-report, and paid for participation. All experimental methods and procedures in this study conformed to ethical guidelines and were approved by the Institutional Review Board of Zhejiang Sci-Tech University. Informed consent was obtained from each participant.
These participants were randomly assigned to four groups: hand control without motor-space expansion (10 males, 10 females: age M = 20, SD = 1.3 years), hand control with motor-space expansion (10 males, 10 females: age M = 19.3, SD = 1.3 years), gaze-based control without motor-space expansion (10 males, 10 females: age M = 20.9, SD = 2.5 years), and gaze-based control with motor-space expansion (10 males, 10 females: age M = 19.7, SD = 1.6 years).
Apparatus and program
The SMI -iView XTM RED eye-tracker system was used in the current study to track the eye movements with a sampling rate of 120 Hz (accuracy: 0.5°). The eye-tracking system was contact-free and placed at the bottom of a monitor (1440 × 900 pixels, visual angle: 37.7° * 24.5°). The participants were seated 60 cm from the computer’s monitor, with their chins on a bracket, to prevent eye-tracking inaccuracies caused by head movement. All participants utilized a Dell MS111 optical, wired mouse for cursor movement in the hand control mode and for confirming selections across all experimental conditions. The maximum resolution of the mouse is 1000 dpi, and the refresh rate is 3000 frames per second. The pointer velocity and acceleration of the mouse were in its default settings in the Windows 7 Ultimate operating system. Fluorescent lamps provided low-intensity lighting in the laboratory.
The experimental program was compiled using Visual C# 2015. As shown in , the start target is a green-filled circle (RGB = (0, 255, 0)) placed in the centre of the visual display. Around it, there are six goal targets, represented by blue-filled circles ((RGB = (0,0,255)) marked with a number. Targets 1, 2, 3, and 4 are placed in the four directions, with a distance of 290 pixels from the centre of the start target. Targets 5 and 6 are placed farther separately on each side of the horizontal direction, with a distance of 470 pixels from the centre of the start target. The diameter of the target is 50 pixels (visual angle: 1.35°*1.35°) in the practice stage and 20 pixels (visual angle: 0.54°*0.54°) in the experimental stage. The remaining filled circles are interference targets with the same size and colour as the goal targets. The dotted square with a side length of 90 pixels (visual angle: 2.43° * 2.43°) around the target indicates the expanded motor space of the target in the expansion condition. It was invisible to the participants. For the convenience of design, we evenly divided the adjacent free space between the targets.
Figure 3. A schematic illustration of the program interface in experiment 1. In the center of the visual display is the start target filled with green (RGB = (0, 255, 0)). Six blue-filled circles ((RGB = (0,0,255)) marked with a number are the goal targets. 39 targets 1, 2, 3, and 4 are located 290 pixels away from the center of the start target, while targets 5 and 6 are located 470 pixels away. The diameter of the target is 20 pixels (visual angle: 0.54°*0.54°). The remaining filled circles were interference targets with the same size and color as the goal targets. The dotted square with a side length of 90 pixels (visual angle: 2.43° * 2.43°) around the target indicates the expanded motor space of the target in the expansion condition. (note. The dotted square and numbers in the circle were not visible in the experiment).

Design and procedure
A 2 (control mode: hand control vs. gaze-based control) *2 (motor-space expansion: yes vs. no) between-subject design was adopted.
Before the experiment, an instruction containing a diagram for one trial was shown to the participants. They were asked to use their gaze or hand-controlled mouse to move the on-screen cursor to the randomly presented green target accurately and quickly, ending with a left mouse click to confirm the selection. Then, the experimenter conducted a five-point calibration that covers the edges of the experimental space. The calibration ended when the X- and Y-axis errors were smaller than 0.5°. After calibration, participants were asked to complete 18 practice trials (three times for each goal target selection). The formal experiment section was not initiated until the practice trials’ accuracy was ≥ 90%. There were 120 trials for each condition (20 for each target). After finishing all the trials, participants were required to complete the NASA-TLX (Hart & Staveland, Citation1988) survey to measure the subjective rating of task load.
As shown in , the participants must select the start target to initiate each trial and start the time recording. One of the goal targets would change from an initial blue to green, informing the participants to move the cursor to the target quickly. Once the cursor entered the motor space of the target, the target visual space changed to red (RGB = (255,0,0)), indicating the target was captured. Then, the participants could click the left mouse to finish this trial, and the program stopped recording time simultaneously. When the gaze-controlled or mouse-controlled cursor ended outside the target’s motor space, it was marked as an incorrect trial. The program recorded the selection accuracy and time for every trial. The results were provided to participants in the practice session but not the experiment session. The target’s motor and visual space size were identical and unchanged in the no expansion condition, when participants should move the cursor into the visual(motor) space to capture the target.
Figure 4. Illustration of one trial in gaze-based control mode with the target’s motor space expanded. a) participants had to select the start target to initiate one trial and start the time recording. One of the goal targets would change from an initial blue to green. b) participants could move the gaze-controlled cursor into the target motor space (invisible dotted square). Once the cursor entered the motor space, the target visual space changed to red (RGB = (255,0,0)). c) participants could click the left mouse to finish this trial, and the program stopped recording time simultaneously.

Dependent variables
We analysed the selection accuracy, speed, throughput, selection endpoint distance to the target centre, standard deviation of the endpoint distance, and NASA-TLX results as the dependent variables (DVs).
Throughput is an important index to quantify human performance when using a specific input method. It combines speed and accuracy. As a single measure, it directly impacts the usability and accessibility of the system and is often used to compare different input methods (MacKenzie, Citation2018; Zhang & MacKenzie, Citation2007). Since throughput should be calculated based on a sequence of trials, we first filtered out the targets with an accuracy lower than 10% to avoid situations where there is only one correct trial, then calculated the throughput of each target for every subject according to Eq. (1).
Ae represents the distance between the start target centre and endpoint click position (as shown in ), and MT represents the target selection time that elapsed from the mouse click in the start target to the endpoint click. Then, speed measures the value that divides Ae by MT. The endpoint distance to the target centre (dx, as shown in ) is used as an expected behaviour that reflects the overshoot or undershoot of each trial in the direction of motion (MacKenzie, Citation2018). A positive value indicates overshoot, a selection that ends on the far side of the goal target centre, while a negative value indicates undershoot, a selection that ends on the near side. SDx represents the standard deviation of the endpoint distance, which corresponds to the effective target width (MacKenzie, Citation2018).
Figure 5. The geometry of selecting a horizontal right target. ”s” represents the center of the start target; ”g” represents the center of the goal target; ”c” represents the endpoint where participants make a left-mouse click. ”Ae” represents the distance between the start target center and endpoint click position; ”dx” represents the endpoint distance to the target center along the axis of the pointing direction.

We used the full version of NASA-TLX (Hart & Staveland, Citation1988), including six dimensions: mental demand, physical demand, temporal demand, effort, performance, and frustration. We reported the results on the total workload as well as each dimension. Higher scores indicated increased workload.
Data analysis
We constructed linear mixed-effects models (LME4: D. Bates et al., Citation2015) for five DVs to investigate the influence of the control mode and motor-space expansion in determining the target selection performance. LME4 allows for variation in effects based on random factors (variation among different participants and targets) and the condition when unequal observations are entered into different cells within the analysis.
Control mode (i.e., hand or gaze-based control), motor-space expansion (i.e., yes or no), and their interaction entered into the model as the fixed factors. Participants and targets entered into the model as random factors. None of the models included the slope term because the fixed factors were between subjects, and including the slope would have caused the model to fail to converge.
Results
shows the average results of six DVs with or without expansion under the hand- or gaze-based control modes.
Table 1. Results of six DVs under four conditions (M ± SD) in experiment 1.
Selection accuracy
As a dependent variable, we determined whether each target was selected accurately. Since this was a binary variable (i.e., “selected accurately” vs. “not selected accurately”), we used a binomial model to analyse the data. We found significant main effects of control mode (χ2(1) = 73.18, p < 0.001) and motor-space expansion (χ2(1) = 83.90, p < 0.001). We also found an interaction effect between the control mode and motor-space expansion (χ2(1) = 3.84, p = 0.0499). A simple effect analysis showed that the increase in the selection accuracy under the gaze-based mode caused by the expansion (β = 0.447, SE = 0.059, z = 7.58, p < 0.001) was greater than that in hand-controlled mode trials (β = 0.041, SE = 0.011, z = 3.67, p < 0.001), as shown in .
Selection speed
We found significant main effects of control mode (χ2(1) = 70.34, p < 0.001) and motor-space expansion (χ2(1) = 64.93, p < 0.001). We also found an interaction effect between the control mode and motor-space expansion (χ2(1) = 35.53, p < 0.001). A simple effect analysis showed that the increase in the speed under gaze-controlled mode caused by the expansion (β = 169.8, SE = 12.5, t = 13.56, p < 0.001) was greater than that in hand-controlled mode trials (β = 51.7, SE = 12.4, t = 4.16, p < 0.001), as shown in .
Selection throughput
We found significant main effects of control mode (χ2(1) = 90.70, p < 0.001) and motor-space expansion (χ2(1) = 6.91, p = 0.009). We also found an interaction effect between the control mode and expansion effect (χ2(1) = 79.98, p < 0.001). A simple effect analysis showed that the expansion significantly increased the throughput in gaze-controlled mode trials (β = 1.34, SE = 0.12, t = 11.4, p < 0.001) but decreased the throughput in hand-controlled mode trials (β = −0.60, SE = 0.12, t = −5.16, p < 0.001), as shown in .
Endpoint distance to the target center
We found significant main effects of control mode (χ2(1) = 12.64, p < 0.001) and motor-space expansion (χ2(1) = 21.04, p < 0.001). We also found an interaction effect between the control mode and motor-space expansion (χ2(1) = 9.07, p = 0.003). A simple effect analysis showed that the expansion significantly increased the endpoint distance in gaze-controlled mode trials (β = −5.85, SE = 1.01, t = −5.81, p < 0.001). However, no significant difference was found in hand-controlled mode trials (β = −1.50, SE = 0.98, t = −1.54, p = 0.128), as shown in .
Endpoint distance variation
We only found significant main effects of control mode (χ2(1) = 4.48, p = 0.034) and motor-space expansion (χ2(1) = 107.06, p < 0.001). The endpoint distances varied more widely in the gaze-controlled group (M = 8.8, SD = 4.0) than in the hand-controlled mode (M = 7.8, SD = 4.5), as well as in the expansion group (M = 11.9, SD = 3.1) than in the non-expansion group (M = 4.7, SD = 0.7), as shown in .
NASA-TLX
A 2 (control mode: hand- vs. gaze-based) *2 (motor-space expansion: yes vs. no) two-way analysis of variance (ANOVA) was conducted. The results showed that the main effects of control mode and expansion were significant (F control (1, 76) = 26.65, p < 0.001, ηp2 = 0.26, F expansion (1, 76) = 11.78, p < 0.001, ηp2 = 0.13). No significant interaction effect was found (p = 0.086).
shows the NASA-TLX profiles for each condition in experiment 1. We found significant interaction effects on the dimensions of frustration and temporal demand. The expanded motor-space significantly lowered the estimations of frustration (F (1, 37) = 24.51, p < 0.001, ηp2 = 0.40) and temporal demand (F (1, 38) = 18.86, p < 0.001, ηp2 = 0.33) in the gaze-controlled mode, and the effect was larger than that in the hand-controlled mode (F frustration (1, 38) = 5.52, p = 0.024, ηp2 = 0.13, F temporal demand (1, 38) = 0.69, p = 0.411). Furthermore, the estimations of physical demand and effort were higher in the gaze-controlled mode than in the hand-controlled mode (F physical demand (1, 76) = 13.2, p < 0.001, ηp2 = 0.15, F effort (1, 76) = 15.18, p < 0.001, ηp2 = 0.17).
Figure 8. Mental workload profiles in gaze or hand-controlled conditions with motor space expanded or non-expanded (experiment 1). Bars with solid black borders indicate that the corresponding dimension had a significant interaction effect. Bars with dotted borders indicate that the corresponding dimension had a significant main effect. ***, p < 0.001; **, p < 0.01; *, p < 0.05; ns, not significant.

Results summary
These findings indicate that the gaze-controlled group benefited more from invisible expansion of motor-space than in the hand-controlled group, as evidenced by their increased accuracy, speed, throughput, endpoint distance, and reduced mental workload (frustration and temporal demand). The endpoint distances were more widely varied in the gaze-controlled and expansion group. Surprisingly, hand-controlled selection throughput declined significantly in the expansion group compared to those without expansion.
Experiment 2
Experiment 2 was used to examine the effect of target motor-space size and visual-space size on the gaze-based target selection performance.
Method
Participants
180 college participants (83 males, 97 females) aged 20.4 years old (SD = 1.8 years) were recruited for this experiment. All participants had normal or corrected to normal vision, were right-handed based on self-report, and paid for participation. All experimental methods and procedures in this study conformed to ethical guidelines and were approved by the Institutional Review Board of Zhejiang Sci-Tech University. Informed consent was obtained from each participant.
Participants were randomly assigned to nine groups, each with a unique combination of visual and motor space size (in pixels): 10/30 (9 males, 11 females: age M = 20.7, SD = 2.5 years), 10/60 (9 males, 11 females: age M = 20.2, SD = 1.9 years), 10/90 (8 males, 12 females: age M = 20.9, SD = 1.9 years), 20/30 (9 males, 11 females: age M = 20.2, SD = 1.9 years), 20/60 (9 males, 11 females: age M = 20.3, SD = 1.9 years), 20/90 (10 males, 10 females: age M = 20.3, SD = 1.3 years), 30/30 (9 males, 11 females: M = 20.2, SD = 1.6 years), 30/60 (12 males, 8 females: age M = 20.6, SD = 1.6 years), and 30/90 (8 males, 12 females: age M = 20.9, SD = 1.8 years).
Apparatus and program
The apparatus was identical to that used in Experiment 1.
The program interface was identical to Experiment 1 except for the following changes. In Experiment 2, we added four interference targets to each goal target, one in each direction, as shown in . This gave participants a more realistic sense of the available space around the target, which was important because we were manipulating the motor-space size.
Figure 9. Each goal target (labeled with numbers) has four disturbance targets. ‘MS’ is an abbreviation of motor space with three levels: 30, 60, and 90 pixels; ‘VS’ is an abbreviation of visual space (screen target size) with three levels: 10, 20, and 30 pixels. Other display parameters were identical to that in experiment 1.

Design and procedure
A 3 (visual-space size: 10 vs. 20 vs. 30 pixels) ×3 (motor-space size: 30 vs. 60 vs. 90 pixels) between-subject design was adopted. The task and procedure were identical to those used in Experiment 1. In Experiment 2, all the participants were instructed to use their gaze to control the on-screen cursor moving to the goal target and finished the task with a hand-controlled mouse click.
Dependent variables and data analysis
The dependent variables in Experiment 2 were calculated and analysed identically to those in Experiment 1.
Results
shows the average results of six DVs with three motor-space sizes under three visual-space sizes.
Table 2. Results of six DVs under nine conditions (M±SD) in experiment 2.
Selection accuracy
We found a significant main effect of motor-space size (χ2(2) = 211.38, p < 0.001); specifically, the target selection accuracy was higher with a motor size of 90 pixels (M = 0.97, SD = 0.03) versus 60 pixels (M = 0.88, SD = 0.09). The accuracy in these two conditions was higher than that under the 30-pixel condition (M = 0.62, SD = 0.18). No significant effect of visual-space size and interaction effect was found, as shown in .
Selection speed
We only found significant main effects of motor-space size (χ2(2) = 224.6, p < 0.001) and visual-space size (χ2(2) = 13.919, p < 0.001). The pointing speed was faster with a motor size of 90 pixels (M = 308.5, SD = 41.9) versus 60 pixels (M = 278.2, SD = 35.6), and these two levels were both faster than that with 30 pixels (M = 161.5, SD = 44.2). The pointing speed with visual sizes of 30 pixels (M = 259.4, SD = 76.2) and 20 pixels (M = 255.1, SD = 78.9) was faster than that with 10 pixels (M = 233.8, SD = 69.4). No significant interaction effect was found, as shown in .
Selection throughput
We found a significant main effect of motor-space size (χ2(2) = 183.35, p < 0.001). We also found an interaction effect between the motor-space size and visual-space size (χ2(4) = 17.72, p = 0.001). As shown in , a simple effect analysis revealed that the interaction was primarily caused by a significant increase in throughput with a motor-space size of 90 pixels compared to 60 pixels when the visual-space size was 30 pixels (β = 0.59, SE = 0.13, t = 4.58, p < 0.001). However, when the visual space was 10 pixels (p = 0.167) or 20 pixels (p = 0.716), there were no significant differences between these two sizes of motor space (90 pixels vs. 60 pixels).
Endpoint distance to the target center
We only found a significant main effect of motor-space size (χ2(2) = 17.91, p < 0.001); As shown in , the distance was much further away to the target centre with a motor-space size of 90 pixels (M = −5.5, SD = 8.8) than with 60 pixels (M = −1.5, SD = 5.4) and 30 pixels (M = −1.1, SD = 2.7).
Endpoint distance variation
We found a significant main effect of motor-space size (χ2(2) = 164.25, p < 0.001) and visual-space size (χ2(2) = 6.25, p = 0.04). We also found an interaction effect between them (χ2(4) = 19.79, p < 0.001). A simple effect analysis showed that the interaction was mainly caused by the decrease in the endpoint variation in the group of a 90-pixel motor space when the visual-space size was 30 pixels. As shown in , no significant difference between the motor space of 90 and 60 pixels was evident when the visual-space size was 30 pixels (p = 0.957). However, the endpoint variation in the group of a 90-pixel motor space was larger than that of 60 pixels when the visual-space size was 10 pixels (β = 1.44, SE = 0.46, t = 3.16, p = 0.005) and 20 pixels (β = 2.90, SE = 0.46, t = 6.35, p < 0.001).
NASA-TLX
The ANOVA results on NASA-TLX revealed that the main effect of motor-space size was significant (F (2, 171) = 25.80, p < 0.001, ηp2 = 0.23), and post hoc test (Bonferroni) results showed that the participants reported a lower task load with motor sizes of 90 pixels (M = 8.5, SD = 3.0) and 60 pixels (M = 8.9, SD = 3.2) compared to 30 pixels (M = 12.3, SD = 3.1). No significant main effect of visual-space size (p = 0.907) or interaction effect (p = 0.960) was found.
shows NASA-TLX profiles for nine conditions. We found an interaction effect between motor-space size and visual-space size on effort dimension (F (4, 171) = 3.05, p = 0.019, ηp2 = 0.07). A simple effect analysis showed that the interaction was mainly caused by a significant increase in the estimation with a motor-space size of 30 pixels compared to that with 60 pixels (p < 0.001) and 90 pixels (p < 0.001) when the visual-space size was 30 pixels. Besides, the estimations of temporal demand and frustration were higher with a motor-space size of 30 pixels compared to that with 60 pixels (p temporal = 0.003; p frustration < 0.001) and 90 pixels (ps < 0.001). Interestingly, the visual-space size significantly affected the mental demand evaluation (p = 0.048). The participants felt a lower mental demand with a visual-space size of 30 pixels than that with 10 pixels (p(non-adj.) = 0.044) and 20 pixels (p(non-adj.) = 0.024).
Figure 12. Mental workload profiles with three motor-space sizes under three visual space sizes (experiment 2). Bars with solid black borders indicate that the corresponding dimension had a significant interaction effect. Bars with dotted borders indicate that the corresponding dimension had a significant main effect. ***, p < 0.001; **, p < 0.01; *, p < 0.05; ns, not significant.

Results summary
In summary, these findings revealed that as the motor-space size increased, participants selected the target more accurately and quickly, ended much farther away from the target centre, and felt a lower task load (temporal demand and frustration). In addition, the selection was faster in the group with a larger visual-space size, and the participants felt a lower mental demand. More importantly, we found a significant interaction between visual-space size and motor-space size on throughput, endpoint distance variation, and effort demand.
General discussion
The present study examined the effects of the invisible expansion of motor space on target selection performance under the gaze-based and hand-controlled modes (Experiment 1) and how the motor-space size affected the gaze-based target selection with different visual-space sizes (Experiment 2). The results showed that participants could select targets more efficiently and experienced less task load in the invisible expanded motor space group; these effects were more pronounced in the gaze-based control mode compared to the hand-controlled mode. Moreover, we found that the motor space, even invisible, was the main contributor to the performance gains observed in the gaze-based target selection, and the visual-space size concurrently had a unique effect during the process.
The effects of invisible motor-space expansion
Gaze-based selection benefited more from the expansion
We initially found a positive effect of invisible motor-space expansion on target selection performance (accuracy and speed) for gaze and hand-controlled modes (Experiment 1). This finding is consistent with Miniotas et al. (Citation2004) study, which verified the benefits of invisible motor-space magnification in 1D gaze-based target selection. Additionally, our findings revealed a more noticeable enhancement in accuracy and speed when participants utilized the gaze-controlled mode compared to the hand-controlled mode. This aligns with previous studies (Agustin et al., Citation2009; Chun-Yan et al., Citation2020; Miyoshi & Murata, Citation2001), which found that target size, given identical motor and visual space, had a greater impact on gaze-based target selection than hand-controlled mode. Our NASA-TLX rating results also supported the performance results. The expansion significantly reduced participants’ frustration and temporal demand, particularly in gaze-controlled mode, where the decrease was more pronounced than in hand-controlled mode.
Our findings suggest that using an invisible expanded motor space significantly enhances the performance and reduces the subjective workload in gaze-controlled 2D target selection. This indicates that the expanded motor space facilitates a transition in gaze-controlled interaction, shifting from a more challenging and effortful mode to a more efficient and effortless form of motor control. This transition potentially signifies an improvement in executive control, a fundamental aspect of our capacity to produce voluntary behaviour (Munoz & Everling, Citation2004). Supporting this notion, Souto et al. (Citation2021) discovered that a gaze typing-by-pointing task could enhance executive control, as evidenced by shorter antisaccade latencies compared to a group using mouse control.Surprisingly, the invisible motor-space expansion negatively affects throughput under the hand-controlled mode. This phenomenon could potentially be associated with the theory of sensorimotor skill acquisition. According to this theory, two forms of control, conscious and automatic, work in parallel, each dominating at different stages of skill acquisition (Haith & Krakauer, Citation2018; Hardwick et al., Citation2019). As widely recognized, the mouse-controlled selection is a highly proficient operation, with the fast motor control system requiring less workload. Our results in performance and subjective workload rating corroborate this. However, the invisible expansion of the motor space seems to demand additional attention, as suggested by the more considerable variance in selection offset. This increased attentional demand could potentially hinder the performance of hand-controlled selection.
The perception of the invisible expanded motor space
We found that participants made selections significantly further away from the target (undershoot) in the expansion condition with the gaze-controlled mode (Experiment 1). The undershoot magnitude increased as the motor space became more extensive (Experiment 2). Our findings suggest that participants may favour a speed-accuracy trade-off strategy in gaze-controlled mode, which can manifest as an undershoot (Peternel et al., Citation2017). This implies they might utilize visual feedback information more in gaze compared to hand mode. In our experiment, participants were instructed that if the gaze or mouse-controlled cursor lands within the target’s motor space, they could use feedback from the visual space to confirm the selection. However, an invisible expanded motor space increases the feedback uncertainty in the visual space. Elliott et al. (Citation2014) suggested that uncertain visual feedback may lead participants to adopt a more conservative approach in their movement planning, resulting in greater landing undershooting.
In addition, the results from Experiment 1 and Experiment 2 indicated that the endpoint distance varies more in the group with larger invisible motor space. MacKenzie (Citation2018) noted that wide variability in endpoint distance corresponds to a larger effective target width. Our results provide evidence that participants were able to adapt to the enlarged motor space. This adaptation can be understood in the context of implicit visuomotor learning, a process through which individuals learn and adapt motor skills without conscious awareness or explicit instructions. It involves acquiring and refining motor movements through repeated practice and exposure to sensory feedback (Reichenthal et al., Citation2016). Despite the invisible expansion of the target’s motor space in our study, participants were able to perceive feedback from the visual space. This perceptible visual feedback may drive a learning process, influencing the participants’ adaptation to the expanded motor space.
The contribution of motor space and visual space
The results in Experiment 2 supported the idea that motor space played a major role in gaze selection performance, even though it was invisible. They demonstrated that only as the motor-space size increased participants selected the target more accurately and quickly, accompanied by a lower task load (effort, temporal demand, and frustration). These results confirmed the effect of motor space in Experiment 1 and are partially consistent with previous hand-controlled studies (Usuba et al., Citation2018, Citation2020), which found that the movement time depends on the target clickable width.
Besides, we also found a positive effect of visual-space size on target selection speed. This aligns with the findings of Cockburn and Brock (Citation2006), who demonstrated the facilitation effect of visual-only expansion for small targets on acquisition time. However, our results differ from those of Usuba et al. (Citation2020), who observed that the visual width slightly affected mouse movement time. It is unclear why the findings differ so greatly, but there are a few notable differences between them. Firstly, Usuba et al. delivered feedback on the clickable area (equal to the motor space in our study). In contrast, we delivered feedback in the target’s visual space. Secondly, Usuba et al. used a hand-controlled mode task that includes eye-mouse coordination during operation. Qvarfordt (Citation2017) observed that mouse and eye movements were less synchronized when selecting a large target than when selecting a small target. These lead to less attention given to the target visual space in hand-controlled mode, which is different in gaze-controlled mode. A previous study confirmed that the participants focused more on a target when they were required to use their gaze to point to it than when they were asked to tap it (Epelboim et al., Citation1995). Therefore, we inferred that the gaze-controlled mode and the visual-space-based feedback design led to extra attention on the target visual space, thus resulting in the facilitative effect of visual space found in our study. Furthermore, our results, to some extent, support the observations of Seidler et al. (Citation2004) that larger targets are more dependent on feedforward control, even when the participants could only get the sensory information from visual space. However, this needs further validation in future studies.
Importantly, we observed a significant interaction between visual-space size and motor-space size on selection throughput. When the target has a large visual-space size (30 pixels), selection throughput was significantly increased in the group with a 90-pixel motor-space size compared to that with a 60-pixel space size. However, no significant differences were found between them (90 vs. 60 pixels) in the group with 10-pixel or 20-pixel visual-space size. The results reflected that a large visual space size not only helps speed up the target selection but also modulates the effect of motor space to unleash the throughput of the technique. This aligns with the view put forward by Guillon et al. (Citation2015), who noted that if the participants have no information on target expanded shapes, they rely on the screen-target shapes.
Correspondingly, we found a significant decrease in the dispersion of the endpoints in the group of 30/90 pixels compared to the group of 20/90 pixels. The results suggest that when the motor space is sufficiently large, an expanded visual space can enhance the stability of gaze-based selection. This phenomenon could be attributed to the interplay between feedback and feedforward processes, integral to optimal movement control (Desmurget & Grafton, Citation2000). The presence of a large motor space that facilitates visual feedback, coupled with a large visual space that promotes the activation of feedforward control, could account for this observation. These insights provide a theoretical foundation for optimizing gaze-based interfaces through the integration of visual and motor space, particularly in scenarios (e.g., gaze-controlled games) where the visual space of a target does not need to be as large as the target’s motor space.
Limitations and future research directions
This study has several limitations. First, this study primarily investigated the effects of visual and motor space size on gaze-based interaction. Future research could explore the optimal combination of these two spaces, which would have direct implications for gaze-based interface design. Second, this study was a preliminary investigation of how participants perceive motor and visual space during gaze-based selection. Follow-up research using eye movement behaviour could provide more detailed insights into this process. Third, the current study was limited to healthy young adults. Future research should investigate more diverse samples and tasks to generalize the findings to broader populations and applications.
Conclusion
In this study, we explored the contribution of motor and visual space to gaze-based target selection performance. We found that both spaces impacted the target selection. Results confirmed that the (invisible) motor-space size is the determining factor in facilitating the performance, particularly beneficial to the gaze-based selection accuracy. Concurrently, the visual-space size had a unique effect during the process. Increasing the target size in visual space could speed up the gaze-based target selection and lower the mental demand of participants. More importantly, visual-space size could also modulate the effects of motor-space size on the stability and throughput of gaze-based selection.
These findings provide a better understanding of the role of motor and visual space in gaze-based target selection and provide a new perspective for optimizing gaze-based interfaces. It suggests that the design should consider the unique effect of motor and visual space. We could improve the gaze interaction accuracy by invisibly expanding the motor space. Meanwhile, the design in the visual space of a target could provide the user with feedforward and feedback information that helps improve the efficiency and user experience of gaze selection.
Disclosure statement
No potential conflict of interest was reported by the author(s).
Data availability statement
The data that support the findings of this study are available upon reasonable request: a written request can be submitted to investigator, Qi-jun Wang ([email protected]).
Additional information
Funding
References
- Agustin, J. S., Mateo, J. C., Hansen, J. P., & Villanueva, A. (2009). Evaluation of the potential of gaze input for game interaction. PsychNology Journal, 7(2), 213–17.
- Aoki, H., Hansen, J. P., & Itoh, K. (2008). Learning to interact with a computer by gaze. Behaviour & Information Technology, 27(4), 339–344. https://doi.org/10.1080/01449290701760641
- Bates, R., & Istance, H. (2002, July). Zooming interfaces! Enhancing the performance of eye controlled pointing devices. Proceedings of the Fifth International ACM Conference on Assistive Technologies (pp. 119–126). Association for Computing Machinery. https://doi.org/10.1145/638249.638272
- Bates, D., Mächler, M., Bolker, B., & Walker, S. (2015). Fitting linear mixed-effects models using lme4. Journal of Statistical Software, 67(1), 1–48. https://doi.org/10.18637/jss.v067.i01
- Bieg, H. J., Chuang, L. L., Fleming, R. W., Reiterer, H., & Bülthoff, H. H. (2010, March). Eye and pointer coordination in search and selection tasks. Proceedings of the 2010 Symposium on Eye-Tracking Research & Applications (pp. 89–92). Association for Computing Machinery. https://doi.org/10.1145/1743666.1743688
- Borji, A., Lennartz, A., & Pomplun, M. (2015). What do eyes reveal about the mind?: Algorithmic inference of search targets from fixations. Neurocomputing, 149, 788–799. https://doi.org/10.1016/j.neucom.2014.07.055
- Canare, D., Chaparro, B., & He, J. (2015, August). A comparison of gaze-based and gesture-based input for a point-and-click task. International Conference on Universal Access in Human-computer Interaction (pp. 15–24). Springer, Cham. https://doi.org/10.1007/978-3-319-20681-3_2
- Choi, M., Sakamoto, D., & Ono, T. (2020, June). Bubble gaze cursor+ bubble gaze lens: Applying area cursor technique to eye-gaze interface. In ACM Symposium on Eye Tracking Research and Applications (pp. 1–10). Association for Computing Machinery. https://doi.org/10.1145/3379155.3391322
- Chun-Yan, K., Zhi-Hao, L., Shao-Yao, Z., Hong-Ting, L., Chun-Hui, W., & Tian, Y. (2020). Influence of target size and distance to target on performance of multiple pointing techniques. Space Medicine & Medical Engineering, 33(2), 112–119. https://doi.org/10.16289/j.cnki.1002-0837.2020.02.004
- Cockburn, A., & Brock, P. (2006). Human on-line response to visual and motor target expansion. Proceedings of the Graphics Interface 2006, Quebec City, Canada (pp. 81–87). Canadian Information Processing Society.
- Delamare, W., Janssoone, T., Coutrix, C., & Nigay, L. (2016, June). Designing 3D gesture guidance: Visual feedback and feedforward design options. Proceedings of the International Working Conference on Advanced Visual Interfaces (pp. 152–159). Association for Computing Machinery. https://doi.org/10.1145/2909132.2909260
- Desmurget, M., & Grafton, S. (2000). Forward modeling allows feedback control for fast reaching movements. Trends in Cognitive Sciences, 4(11), 423–431. https://doi.org/10.1016/S1364-6613(00)01537-0
- Djajadiningrat, T., Overbeeke, K., & Wensveen, S. (2002, June). But how, Donald, tell us how? On the creation of meaning in interaction design through feedforward and inherent feedback. Proceedings of the 4th Conference on Designing Interactive Systems: Processes, Practices, Methods, and Techniques (pp. 285–291). Association for Computing Machinery. https://doi.org/10.1145/778712.778752
- Elliott, D., Dutoy, C., Andrew, M., Burkitt, J. J., Grierson, L. E., Lyons, J. L., & Bennett, S. J.(2014). The influence of visual feedback and prior knowledge about feedback on vertical aiming strategies. Journal of Motor Behavior, 46(6), 433–443. https://doi.org/10.1080/00222895.2014.933767
- Epelboim, J., Steinman, R. M., Kowler, E., Edwards, M., Pizlo, Z., Erkelens, C. J., & Collewijn, H. (1995). The function of visual search and memory in sequential looking tasks. Vision Research, 35(23–24), 3401–3422. https://doi.org/10.1016/0042-6989(95)00080-X
- Feng Cheng-Zhi, S. M.-W., & Hui, S. (2003). Spatial and temporal characteristic of eye movement in human-computer interface design. Space Medicine & Medical Engineering, 16(4), 304–306. https://doi.org/10.1023/A:1022080713267
- Guillon, M., Leitner, F., & Nigay, L. (2014, May). Static voronoi-based target expansion technique for distant pointing. Proceedings of the 2014 International Working Conference on Advanced Visual Interfaces (pp. 41–48). Association for Computing Machinery. https://doi.org/10.1145/2598153.2598178
- Guillon, M., Leitner, F., & Nigay, L. (2015). Investigating Visual Feedforward for Target Expansion Techniques. In Proceedings of the 33rd Annual ACM Conference on Human Factors in Computing Systems - CHI ’15 (pp. 2777–2786). Association for Computing Machinery. https://doi.org/10.1145/2702123.2702375
- Gutwin, C. (2002, April). Improving focus targeting in interactive fisheye views. In Proceedings of the SIGCHI Conference on Human Factors in Computing Systems (pp. 267–274). Association for Computing Machinery. https://doi.org/10.1145/503376.503424
- Haith, A. M., & Krakauer, J. W. (2018). The multiple effects of practice: Skill, habit and reduced cognitive load. Current Opinion in Behavioral Sciences, 20, 196–201. https://doi.org/10.1016/j.cobeha.2018.01.015
- Hansen, D. W., Skovsgaard, H. H., Hansen, J. P., & Møllenbach, E. (2008, March). Noise tolerant selection by gaze-controlled pan and zoom in 3D. Proceedings of the 2008 Symposium on Eye Tracking Research & Applications (pp. 205–212). Association for Computing Machinery. https://doi.org/10.1145/1344471.1344521
- Hardwick, R. M., Forrence, A. D., Krakauer, J. W., & Haith, A. M. (2019). Time-dependent competition between goal-directed and habitual response preparation. Nature Human Behaviour, 3(12), 1252–1262. https://doi.org/10.1038/s41562-019-0725-0
- Hart, S. G., & Staveland, L. E. (1988). Development of NASA-TLX (Task Load Index): Results of empirical and theoretical research. In Advances in psychology (Vol. 52, pp. 139–183). North-Holland. https://doi.org/10.1016/S0166-4115(08)62386-9
- Hayhoe, M., & Ballard, D. (2005). Eye movements in natural behavior. Trends in Cognitive Sciences, 9(4), 188–194. https://doi.org/10.1016/j.tics.2005.02.009
- Hyrskykari, A., Istance, H., & Vickers, S. (2012, March). Gaze gestures or dwell-based interaction? Proceedings of the Symposium on Eye Tracking Research and Applications (pp. 229–232). Association for Computing Machinery. https://doi.org/10.1145/2168556.2168602
- Kleinke, C. L. (1986). Gaze and eye contact: A research review. Psychological Bulletin, 100(1), 78. https://doi.org/10.1037/0033-2909.100.1.78
- Kumar, C., Menges, R., Müller, D., & Staab, S. (2017, April). Chromium based framework to include gaze interaction in web browser. Proceedings of the 26th International Conference on World Wide Web Companion (pp. 219–223). International World Wide Web Conferences Steering Committee. https://doi.org/10.1145/3041021.3054730
- Kumar, M., Paepcke, A., & Winograd, T. (2007, April). Eyepoint: Practical pointing and selection using gaze and keyboard. Proceedings of the SIGCHI Conference on Human factors in Computing Systems (pp. 421–430). Association for Computing Machinery. https://doi.org/10.1145/1240624.1240692
- MacKenzie, I. S. (2018). Fitts’ law. In K. L. Norman & J. Kirakowski (Eds.), Handbook of human-computer interaction (pp. 349–370). Wiley. https://doi.org/10.1002/9781118976005.ch17
- McGuffin, M. J., & Balakrishnan, R. (2005). Fitts’ law and expanding targets: Experimental studies and designs for user interfaces. ACM Transactions on Computer-Human Interaction (TOCHI), 12(4), 388–422. https://doi.org/10.1145/1121112.1121115
- Miniotas, D., Špakov, O., & MacKenzie, I. S. (2004, April). Eye gaze interaction with expanding targets. CHI’04 Extended Abstracts on Human Factors in Computing Systems, 1255–1258. https://doi.org/10.1145/985921.986037
- Miyoshi, T., & Murata, A. (2001, October). Usability of input device using eye tracker on button size, distance between targets and direction of movement. In 2001 IEEE International Conference on Systems, Man and Cybernetics. e-Systems and e-Man for Cybernetics in Cyberspace (Cat. No. 01CH37236) (Vol. 1, pp. 227–232). IEEE. https://doi.org/10.1109/ICSMC.2001.969816
- Morimoto, C. H., Leyva, J. A. T., & Diaz-Tula, A. (2018). Context switching eye typing using dynamic expanding targets. Proceedings of the Workshop on Communication by Gaze Interaction - COGAIN ’18. Association for Computing Machinery. https://doi.org/10.1145/3206343.3206347
- Munoz, D. P., & Everling, S. (2004). Look away: The anti-saccade task and the voluntary control of eye movement. Nature Reviews Neuroscience, 5(3), 218–228. https://doi.org/10.1038/nrn1345
- Peternel, L., Sigaud, O., & Babič, J. (2017). Unifying speed-accuracy trade-off and cost-benefit trade-off in human reaching movements. Frontiers in Human Neuroscience, 11, 615. https://doi.org/10.3389/fnhum.2017.00615
- Qvarfordt, P. (2017). Gaze-informed multimodal interaction. The handbook of multimodal-multisensor interfaces: Foundations, user modeling, and common modality combinations-volume 1 (pp. 365–402). https://doi.org/10.1145/3015783.3015794
- Rantala, J., Majaranta, P., Kangas, J., Isokoski, P., Akkil, D., Špakov, O., & Raisamo, R. (2020). Gaze interaction with vibrotactile feedback: Review and design guidelines. Human–Computer Interaction, 35(1), 1–39. https://doi.org/10.1080/07370024.2017.1306444
- Rayner, K. (1998). Eye movements in reading and information processing: 20 years of research. Psychological Bulletin, 124(3), 372–422. https://doi.org/10.1037/0033-2909.124.3.372
- Reichenthal, M., Avraham, G., Karniel, A., & Shmuelof, L. (2016). Target size matters: Target errors contribute to the generalization of implicit visuomotor learning. Journal of Neurophysiology, 116(2), 411–424. https://doi.org/10.1152/jn.00830.2015
- Seidler, R. D., Noll, D. C., & Thiers, G. (2004). Feedforward and feedback processes in motor control. Neuroimage: Reports, 22(4), 1775–1783. https://doi.org/10.1016/j.neuroimage.2004.05.003
- Sibert, L. E., Templeman, J. N., & Jacob, R. J. (2001). Evaluation and analysis of eye gaze interaction (No. NRL/FR/5513–01-9990). Naval Research Lab
- Souto, D., Marsh, O., Hutchinson, C., Judge, S., & Paterson, K. B. (2021). Cognitive plasticity induced by gaze-control technology: Gaze-typing improves performance in the antisaccade task. Computers in Human Behavior, 122, 106831. https://doi.org/10.1016/j.chb.2021.106831
- Špakov, O., & Miniotas, D. (2005, October). Gaze-based selection of standard-size menu items. Proceedings of the 7th International Conference on Multimodal Interfaces (pp. 124–128). Association for Computing Machinery. https://doi.org/10.1145/1088463.1088486
- Squire, P., Mead, P., Smith, M., Coons, R., & Popola, A. (2013). Quick-eye: Examination of human performance characteristics using Eye tracking and manual-based control systems for monitoring multiple displays. Naval Surface Warfare Center.
- Stellmach, S., & Dachselt, R. (2013, April). Still looking: Investigating seamless gaze-supported selection, positioning, and manipulation of distant targets. Proceedings of the Sigchi Conference on Human Factors in Computing Systems (pp. 285–294). Association for Computing Machinery. https://doi.org/10.1145/2470654.2470695
- Su, X., Au, O. K. C., & Lau, R. W. (2014, April). The implicit fan cursor: A velocity dependent area cursor. Proceedings of the SIGCHI Conference on Human Factors in Computing Systems (pp. 753–762). Association for Computing Machinery. https://doi.org/10.1145/2556288.2557095
- Usuba, H., Yamanaka, S., & Miyashita, H. (2018). Pointing to targets with difference between motor and visual widths. Proceedings of the 30th Australian Conference on Computer-Human Interaction (pp.374–383). Association for Computing Machinery. https://doi.org/10.1145/3292147.3292150
- Usuba, H., Yamanaka, S., & Miyashita, H. (2020, December). A model for pointing at targets with different clickable and visual widths and with distractors. 32nd Australian Conference on Human-Computer Interaction (pp. 1–10). Association for Computing Machinery. https://doi.org/10.1145/3441000.3441019
- Vermeulen, J., Luyten, K., van den Hoven, E., & Coninx, K. (2013, April). Crossing the bridge over Norman’s gulf of execution: Revealing feedforward’s true identity. Proceedings of the SIGCHI Conference on Human Factors in Computing Systems (pp. 1931–1940). Association for Computing Machinery. https://doi.org/10.1145/2470654.2466255
- Vertegaal, R. (2008, October). A Fitts law comparison of eye tracking and manual input in the selection of visual targets. Proceedings of the 10th International Conference on Multimodal Interfaces (pp. 241–248). Association for Computing Machinery. https://doi.org/10.1145/1452392.1452443
- Zhai, S., Conversy, S., Beaudouin-Lafon, M., & Guiard, Y. (2003, April). Human on-line response to target expansion. Proceedings of the SIGCHI Conference on Human Factors in Computing Systems (pp. 177–184). Association for Computing Machinery. https://doi.org/10.1145/642611.642644
- Zhang, X., & MacKenzie, I. S. (2007). Evaluating eye tracking with ISO 9241-part 9. In Human-Computer Interaction. HCI Intelligent Multimodal Interaction Environments: 12th International Conference, HCI International 2007, Beijing, China, July 22-27, 2007, Proceedings, Part III 12 (pp. 779–788). Springer.