Abstract
There are numerous studies comparing young and old adults in terms of muscle coordination in standard tasks (e.g., walking, reaching) and small variations of them. These tasks might hide differences: individuals would converge to similar behavior as they practice these throughout life. Also, we are unaware of studies that considered the muscle recruitment nested dynamics. For this reason, our study evaluated how young and old women coordinate and control the movement system while performing an unusual redundant motor control task through the network physiology approach. We acquired electromyographic signals from nine leg muscles of the dominant and non-dominant limbs during maximum voluntary isometric contractions (knee extension and flexion) and co-contraction bouts. Our results showed that young participants presented higher peak torque output, with similar EMG variability, compared to older participants. Considering firing rate frequencies, old and young women demonstrated different traits for network clustering and efficiency for the task. Age seems to affect muscle coordination at higher frequencies, even with a similar number of muscle synergies, indicating that younger women might have more integrated synergies than older women. The findings also point to differential muscle coordination adaptability.
1. Introduction
The human movement system possesses many degrees of freedom distributed in several interacting levels of analysis (Ivanov, Citation2021). Understanding how the system is organized/coordinated toward a goal represents a formidable problem of research (Bernstein, Citation1967). The field of Network Physiology aims to understand the issue through a systems approach, investigating the emergence of organisms’ functions and behavior by describing the integration of physiological systems (Bartsch et al., Citation2015). In our specific case, it implies the usage of network measures (e.g., Bullmore & Sporns, Citation2009; Rubinov & Sporns, Citation2010) to understand the control and coordination of the musculoskeletal system (Boonstra et al., Citation2015; Rizzo et al., Citation2020). This approach allows one to observe how the musculoskeletal system coordination emerges through integration of descending neural drives and many feedback loops during a certain task (Boonstra et al., Citation2015; Kerkman et al., Citation2018). These analyses seem suited to advance understanding of the processes and mechanisms of the movement system by going beyond the simple consideration of what the elements that are coordinated (associated) with each other, but also qualifying the coordination itself in terms of, for instance, its integration, clustering, and centrality (see, for instance, Boonstra & Breakspear, Citation2012; Kerkman et al., Citation2018). Network Analysis captures higher-order characteristics of coordination patterns which might be the correct level to understand principles of change in coordination avoiding specificalities given task or other situational constraints. In the present study, we focused on the differences that emerge in muscle coordination patterns in aging through network analyses.
Previous studies have explored the relationship between age and various task demands, highlighting how age is associated with structural changes (Edström et al., Citation2007; Seidler et al., Citation2010) and disparities in motor outcomes (Lipsitz & Goldberger, Citation1992; Vaillancourt & Newell, Citation2003). Indeed, many studies have investigated muscle coordination changes as individuals age (e.g., Da Silva Costa et al., Citation2020, Citation2023; Sawers et al., Citation2017). These studies point to “less adaptable” behavior provided a lower number of muscle synergies and differential activation. Generally, the coordination is analyzed through the extraction of covariance patterns from the muscles’ myoelectric activation amplitude over time (a “time-based” synergy extraction, see, for instance, d‘Avella et al., Citation2003).
It is important to note, however, that analyses based on muscle activation only might oversimplify the multi time scale nature of muscle control (i.e., the frequency domain, Farmer, Citation1998). There is a rich literature that has demonstrated—and argued in favor of—the possibility of systems communication (neural-muscle paths) through different frequency bands (e.g., Farina et al., Citation2013; Hug et al., Citation2021; Stegeman et al., Citation2010). Independent of whether the argument holds (see Feldman, Citation2015 for a counter argument on muscle control), one cannot dismiss possibilities a priori; one might as well consider this multi time scales emergent coordination—something that network analyses seem suited to consider. To the best of our knowledge, comparisons of young and old adults have not yet considered network analyses to understand coordination dynamics in aging.
Previous studies have used these tools to characterize muscle synergies in healthy individuals in postural control or pinching (Boonstra et al., Citation2015; Stins et al., Citation2011). These tasks, nonetheless, might be limited in generalization. These tasks are well practiced in life; individuals might demonstrate similar outcomes given convergence in behavior over the life span. Such emphasis on “tasks under convergence” might limit observable differences between individuals. In new tasks, we expect unique behaviors as individuals differ in their intrinsic dynamics and their approach to task demands (Kostrubiec et al., Citation2012; Pacheco et al., Citation2019). These differences might be predictive of adaptation and learning capacities (Kostrubiec et al., Citation2012; Pacheco & Newell, Citation2018).
In the present study, we chose an “unusual” task: the co-contraction (derived from training studies, see Maeo et al., Citation2014). The task is to perform voluntary simultaneous contraction of agonist and antagonist muscles around a given joint, producing zero net torque. This is an interesting task as it is redundant in, at least, two important levels of analysis. First, considering a given total force that both extensors and flexors produce against each other, many extensor and flexor muscles can covary to result in these opposing forces, resulting in a zero-net torque. However, the maximum total force that extensors and flexors produce might also vary depending on the capacity of the individual in doing this—which also results in the zero-net torque. Note that there is no augmented information on the latter to increase muscle activation—which alleviates even further this task demand. Such a task allows all potential differences between age groups to be observed. Indeed, previous studies with this paradigm showed large variability between trained male individuals in muscle coordination patterns and overall EMG activation (Silva et al., Citation2023).
Thus, in this study, we described how muscle coordination and control strategies during this redundant task vary across different age groups considering their multi time scale nature. For this, we employ network analyses. Briefly, a network is a map of a given system’s components and their interactions represented as nodes and edges, respectively. In this way, many types of systems with different types of interactions can be studied (see Barabási, Citation2015 for an introduction): in our case, from time-based muscle synergies to frequency-based muscle synergies. From the resultant “mapping” (network) one can extract characteristics of the mapping that summarize overall network structure (as has been applied in neural networks, Rubinov & Sporns, Citation2010).
As far as we are aware, network analyses have never been applied to time-based synergies. Also, when considering the frequency-based synergies, studies have assumed similar frequency (spectral) profiles between participants (see Boonstra et al., Citation2015; Kerkman et al., Citation2018)—which we have no a priori reason to assume. Thus, there is a third possibility for network analyses considering individualized spectral profiles. Beyond the underlying assumptions of these possibilities, the main differences between time-based, matched, and unmatched frequency-based analyses rely on the comparisons that can be made afterwards. First, time-based analyses do not require long time series for precise estimation as frequency-based analyses, which allow one to explore change between trials of the task. Second, unmatched frequency-based analyses do not allow direct comparison of the network measures extracted for each “frequency-band coordination” observed as the frequency bands between participants might differ. This analysis, thus, implies averaging the measures before comparison. In an attempt to explore such possibilities (and compare them), we employed all three of them exploring their potentialities to uncover age differences in muscle coordination and control.
In our study, we described how motor coordination and control strategies during this redundant task vary across different age groups. We elucidate (a) how the muscle activation patterns differ between two age groups, (b) what are the recruitment synergies presented during the task, and (c) differences (if there is any) between the analyses employed. Despite the exploratory nature of the present study, we expect that, given to specific adaptations that occur with aging from motor unit recruitment to structural changes in muscle capacity and general coordination tendencies, we will corroborate previous findings demonstrating differential recruitment strategies between age groups (i.e., differential frequency activation of the synergies, number of synergies demonstrated). These strategies might only be comprehended through the higher-order aspects that network analysis is able to capture.
2. Materials and Methods
2.1. Participants
Ten active female young adults and ten active female older adults participated in this study (minimum of six months practicing systematic physical activity at least three times a week) ( shows the descriptive measures of all participants). Participants reported no orthopedic injuries on the lower limbs in the previous six months or any health problem that could affect performance. The School of Physical Education and Sports at Ribeirão Preto (University of São Paulo) ethics research committee approved the study (protocol 3.898.742).
Table 1. Age, mass and height per participant.
2.2. Procedures
Participants performed a ten-minute warm-up on a stationary exercise bike, at moderate intensity (70–80 RPM). Then, they performed the following procedures with each limb (the order of the limbs was randomly chosen) in a single session. We placed wireless electromyography (EMG) sensors (Trigno Lab Wireless; Delsys, 2000 Hz) on the rectus femoris, vastus lateralis and vastus medialis, sartorius, biceps femoris long and short heads, semitendinosus, semimembranosus, and tensor fascia latae, following Hermens et al. (Citation2000) and Perotto (Citation2011) recommendations.
2.2.1. Maximal Voluntary Isometric Contraction (MVIC)
For the MVIC test, the participants were positioned on an isokinetic dynamometer (System 4Pro™, Biodex) according to the manufacturer’s recommendations. They performed up to three submaximal isometric knee extensions and flexion for familiarization and rested for 60 s. Next, they performed three maximum isometric knee extensions and flexions lasting 5 s each, with 60 s of rest between them. The MVIC was performed in an alternating way.
2.2.2. Co-Contraction Sets
Participants performed the co-contraction task seated on an isokinetic dynamometer (System 4Pro™, Biodex). Participants were instructed to co-contract their thigh muscles as strong as possible, keeping the knee angle fixed at 60° (not strapped). For familiarization, the participant performed up to five co-contractions in a row followed by 30 s of rest. Participants performed 20 repetitions. The knee angle was visually controlled, and participants were instructed to use the device arm as a position reference. Each co-contraction bout lasted four-seconds, followed by four-seconds of relaxation (Maeo et al., Citation2013). The co-contractions bouts and rests were dictated by a metronome app (Intervals Pro, FourthFrame Technologies, Columbus, OH).
2.3. Data Analysis
We inspected the data to remove small artifacts from the EMG time series (less than 192 ms). Repetitions with artifacts lasting longer than 192 ms were removed from analysis (0.11% of the data was removed in this process). We used a Matlab algorithm (https://osf.io/a2pyb/) to extract the EMG activity from each repetition and filter the data with a zero-phase forward and reverse digital IIT filtering (filtfilt in Matlab) with a second order bandpass Butterworth filter (10–500 Hz).
2.3.1. Peak Torque
From MVIC, we registered the peak torque recorded by the dynamometer considering all three attempts for each extension/flexion and legs.
2.3.2. EMG Overall Activation (EMG%) and Between-Muscle EMG Variability (EMGvar)
We calculated the root mean squared (RMS) value for each MVIC trial and noted the maximum value for each muscle (maximum RMS). Then, we calculated the RMS for each co-contraction bout normalizing it in terms of the maximum RMS per muscle. To understand how changes occurred over bouts, we used the average RMS for each block of 6 bouts (eliminating bouts 7 and 14). Also, we calculated the average and standard deviation for all muscles to report an overall EMG activity (EMG%) and the variability between muscles (EMGvar).
2.3.3. Synergy and Network Measures
We performed three different muscle coordination (synergy) analyses. The first two were based on the frequency domain through coherence measurement between muscle pairs. The third was based on the time domain through covariance measurement between muscle pairs. The initial two synergy analyses are based on the idea of a common neural drive for a set of muscles (Boonstra et al., Citation2015; Farmer, Citation1998). That is, “common input” to different muscles would be captured through similar frequency profiles. Indeed, if older adults have differential frequency recruitment of motor units or fiber types, different synergy organizations would be observed. The third synergy analysis is based on the idea that the central nervous system controls the overall activation of a set of muscles (see Bizzi & Cheung, Citation2013; Cheung et al., Citation2005; d‘Avella & Bizzi, Citation2005). Differential capacity to control independent muscle groups given age would provide distinct synergy profiles.
The overall analysis procedure was similar for the three methods: we concatenated the data of interest, filtered it to avoid high frequency oscillations created by concatenation, and performed the non-negative matrix factorization (nnmf code in Matlab). The factorization used the multiplicative update algorithm with 100 random starting replicates. To determine the number of synergies, we iteratively extracted different numbers of synergies (from 1 to 10) and noted the variance accounted for (VAF) the actual input data using the extracted synergies. We also estimated the standard error of VAF by bootstrap: we sampled with replacement the input data and the reconstructed data from the synergies and calculated the VAF for 250 iterations. We set a VAF higher than 90% (considering the bootstrapped confidence interval) as the criteria to select the required number of synergies.
The Frequency Matched Synergies (see Boonstra et al., Citation2015) analysis assumes common spectral profiles between participants. For this, we concatenated the data of all bouts of a given person and leg to have a single long time series–sufficient for our coherence analyses. We filtered the data with a fourth order high-pass Butterworth filter (50 Hz) and then used the Hilbert transform envelope to rectify the data (Myers et al., Citation2003). For all 36 possible muscle pairs, we computed the magnitude-squared coherence with a one second window and 750 ms overlap for all frequencies between 0 and 250Hz. We chose up to 250 Hz due to the broad power spectrum demonstrated by different individuals.
To not be constrained by the assumption of similar spectral profiles between individuals, we also performed an individual-based extraction of muscle synergies. Then, we performed analyses using Unmatched Frequency Synergies. For this, we concatenated all bouts of one leg of a person and performed the magnitude-squared coherence analyses for each muscle pair. However, for the non-negative matrix factorization, we used the coherence of each individual (per leg) to extract person (and leg) specific synergies.
Finally, researchers have also investigated time-varying synergies (see d‘Avella & Bizzi, Citation2005). Following Valk et al. (Citation2019) steps, we first filtered the data of each bout with a low-pass Butterworth filter (4th order) at 10 Hz to get the EMG envelope. This EMG was normalized by getting the maximum value per muscle of the EMG envelope from MVIC trials. Then, we concatenated blocks of six bouts (ignoring bouts 7 and 14) per leg and performed the non-negative matrix factorization following the same procedure as described in the previous two synergy extraction methods.
For all methods described, after the selection of the synergies, we calculated three network measures to infer the structure of the synergies (Rubinov & Sporns, Citation2010). First, we calculated the global efficiency: a measure of network integration (i.e., how all muscles are directly connected to each other). One can consider it as the inverse of how long it would take to pass information from one node to another. Second, we calculated the clustering coefficient: a measure of network segregation (i.e., how many nodes—muscles—have the same neighbors—coherence with other muscles—as their neighbors). This measure informs whether muscles group (are coherent) with a given set of muscles but not others. Third, we calculated betweenness centrality: a measure of whether hubs exist within the network (i.e., if one muscle is highly coherent with others while others are not coherent with each other). The absolute value of these measures cannot be considered on its own (0.5 is not necessarily a small or large value). It really depends on the expected value for a given type of network. Here, we consider them for comparison between young and old women in our specific task. All these measures were computed using the Brain Connectivity Toolbox https://sites.google.com/site/bctnet).
For the Time-Based Synergies, we also noted the number of synergies per leg and two other measures of synergy structure (see Da Silva Costa et al., Citation2020). The first is the number of muscles actively participating in the synergy and the second is the synergy participation over time. The former was calculated by counting the number of muscles that, after normalization by the maximum load within the synergy, contribute more than 0.3 in loading. The latter was calculated as the sum of the contribution of the synergy over time. Both measures were calculated for each synergy for a given person, leg, and block and then averaged.
2.4. Statistical Analysis
We used robust inferential analyses (Wilcox, Citation2012). These analyses deal with deviations from normality by using central tendency and dispersion measures that are unaffected by these deviations. Provided the small sample and lack of power to verify the distribution profile, these analyses were preferred.
For EMG%, EMGvar, and peak torque, we performed a three-way robust trimmed analysis of variance (ANOVA) (bwwtrim code in R) with groups as the between factor and legs and extension/flexion as the two within participants factors. For network measures (betweenness-centrality, clustering coefficient, and global efficiency) in the matched frequency synergies, we performed a three-way robust trimmed ANOVA with groups as the between factor and legs and synergies as the two within participant factors. For the number of synergies in the unmatched frequency synergies, we performed a between by within rank-based ANOVA with groups as the between factor and legs as the within participant factor. For network measures in the unmatched frequency synergies, we performed a two-way robust trimmed ANOVA (bwtrim code in R) with groups as the between factor and legs as the within participant factor. For number of synergies, number of muscles contributing for a synergy, contributions of each synergy over time, and network measures in the time-based synergies, we performed three-way robust trimmed ANOVAs with groups as the between factor and legs and blocks as the two within participant factors. For all trimmed analyses, the algorithm trimmed 20% of data.
The effect sizes were provided in terms of ξ–values above 0.15, 0.35, and 0.50 are considered small, medium, and large effects, respectively (Wilcox, Citation2012). There are, currently, no specific codes for effect size estimation of three-way robust analyses. Provided the lack of three-way interactions in this paper, to estimate the effect size for two-way and one-way effects, we pooled the data for the factors being compared.
Provided the exploratory nature of the analyses, for all results of the ANOVAs, we corrected for the multiplicity of ANOVA by controlling for the false discovery rate (Cramer et al., Citation2016). For this, we used the p.adjust function in R using the Benjamini Hochberg correction.
3. Results
Two participants (one for each group) were excluded from the analyses due to faulty EMG data. We report only significant values here, but all statistical analyses are presented in the supplementary file.
3.1. EMG Overall Activation and Peak Torque
Young women showed higher peak torque than old women (main effect age: Q = 48.86; p <.001, ξ = 0.45) ( and ). The extension peak torque was higher than the flexion peak torque for both groups (main effect extension/flexion: Q = 305.50; p<.001, ξ = 0.95) with greater effect for young women (interaction age x extension/flexion: Q = 11.35; p=.002, ξ = 0.73; post hoc: p’s<.001).
Figure 1. Normalized muscle activation per group (average: bar height; 95% confidence interval: error bars) and per leg. Each blue bar represents individual average muscle activation over the 20 bouts of co-contraction. (RF: rectus femoris; VL: vastus lateralis; VM: vastus medialis; SAR: sartorius; ST: semitendinosus; SM: semimembranosus; BFL: biceps femoris long head; BFS: biceps femoris short head; TFL: tensor fascia latae).

Table 2. Descriptive measures (mean and standard deviation) of the peak torque (N.m) test and EMG (mean and standard deviation) per individual and leg.
Considering the EMG%, we found no significant results for any main or interaction effects. For EMGvar, the variability for young was lower in the right leg than for old women (interaction age × legs: Q = 7.27; p=.050; ξ = 0.46; post hoc: p<.001).
3.2. Frequency Matched Synergies
From visual inspection of , we observe that old women showed lower coherence than young women for frequencies higher than 5 Hz. A large peak was observed for all pairs in frequencies lower than 5 Hz.
Figure 2. Magnitude squared coherences from 0 to 90 Hz for each muscle pair considering young (lower left) and old (upper right) women groups. The central line represents the average coherence between individuals and the shaded area represents the 95% confidence interval. (RF: rectus femoris; VL: vastus lateralis; VM: vastus medialis; SAR: sartorius; ST: semitendinosus; SM: semimembranosus; BFL: biceps femoris long head; BFS: biceps femoris short head; TFL: tensor fascia latae).
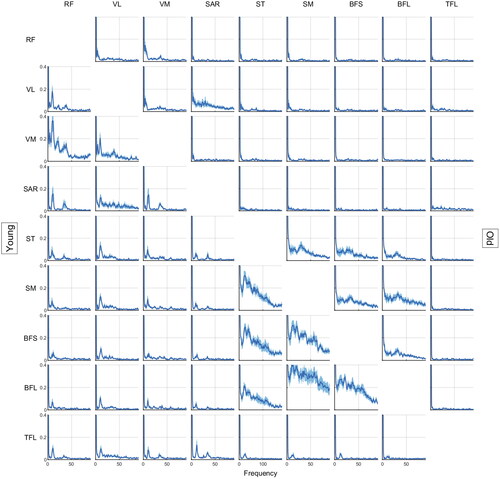
From , it is seen that the first synergy largely represents the large coherence of low frequencies (from 0.1 to 4Hz) with a highly interactive network. The second synergy is more selective of few muscles and represents a frequency band from 0.1 to 40Hz. The third synergy is more broadband (with an increase around 14 Hz and a long tail that goes up to 250 Hz) encompassing fewer muscles. shows the network measures per group, leg, and synergy.
Figure 3. Exemplary network profiles (top row) for the extracted coherence profiles (bottom row) from the matched-frequency synergies analysis (see text). The thickness of the edges represents the magnitude of the relation (coherence) between nodes (muscles). The colors of nodes represent whether they are flexors (red), extensors (blue), or unrelated (purple). Edges below a given threshold are not shown (for illustration purposes).
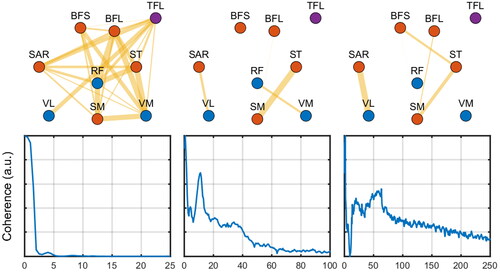
Figure 4. Network measures (from matched-frequency synergies, see text) per group, leg, and synergies. The dots represent each individual.
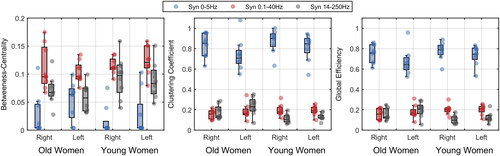
presents the distribution of network measures per group, leg, and synergies. For betweenness centrality, we found that the synergies differed one from each other (first synergy: 0.029 ± 0.036; second synergy: 0.091 ± 0.028; third synergy: 0.082 ± 0.029; Q = 11.19; p<.001; ξ = 0.79; post hoc: p’s<.001).
For cluster coefficient, there was a main effect of synergies (Q = 155.26; p<.001; ξ = 0.84) and an interaction effect between age and synergies (Q = 6.11; p=.008; ξ = 0.35). The synergies differed one from each other for young women (first synergy: 0.848 ± 0.066; second synergy: 0.211 ± 0.054; third synergy: 0.126 ± 0.043; p’s<.001), while the second (0.1–40Hz) and third synergies (14–250Hz) were similar for old women (first synergy: 0.801 ± 0.138; second synergy: 0.228 ± 0.100; third synergy: 0.202 ± 0.066). Additionally, old women showed higher cluster coefficient in the third synergy (14–250Hz) than young women (p<.001).
For global efficiency, there was a main effect of synergies (Q = 226.46; p<.001; ξ = 0.84) and an interaction effect between age and synergies (Q = 7.43; p<.001; ξ = 0.36). The synergies differed one from each other for young women (first synergy: 0.777 ± 0.057; second synergy: 0.211 ± 0.050; third synergy: 0.119 ± 0.048; p’s<.001), while the second and third synergies were similar for old women (first synergy: 0.723 ± 0.112; second synergy: 0.213 ± 0.090; third synergy: 0.175 ± 0.061). Additionally, old women showed higher global efficiency in the third synergy than young women (p=.002).
3.3. Unmatched Synergies
When individuality was allowed, similar spectral profiles emerged. For the participants shown in the figures, we observed a large coherence for slow frequencies (below 5 Hz) and peaks around 35–40Hz (). The broadband synergies were less common.
Figure 5. Exemplary network profiles (odd rows) for the extracted coherence profiles (even rows) from the unmatched-frequency synergies analysis (see text). The thickness of the edges represents the magnitude of the relation (coherence) between nodes (muscles). The colors of nodes represent whether they are flexors (red), extensors (blue), or unrelated (purple). Edges below a given threshold are not shown (for illustration purposes).

There was a large variability between participants in both network measurements and number of synergies (). The mode between participants and leg, however, was still three synergies. The number of synergies was not dependent on age groups or legs (main effect age: F = 0.61; p=.675; main effect of leg: F = 0.01; p=.980; interaction age x leg: F = 3.02; p=.247).
Figure 6. Number of synergies extracted and the network measures (from unmatched-frequency synergies, see text) per group and leg averaged over synergies. The dots represent each individual.
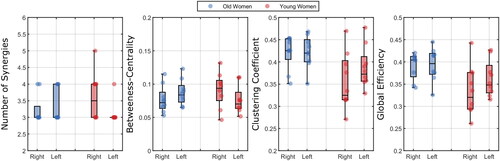
Considering the network measures, we found no significant effects.
3.4. Time-Based Synergies
shows two exemplary participants on the extracted muscle synergies. The most prominent synergy could vary largely between individuals ().
Figure 7. Exemplary network profiles (left column), muscle loadings (center column) of the most prominent synergy, and these synergies’ contributions over time (right column) considering one young and one old woman. For the network profiles, the thickness of the edges represents the magnitude of the relation (absolute correlation) between nodes (muscles). The colors of nodes represent whether they are flexors (red), extensors (blue), or unrelated (purple). Edges below a given threshold are not shown (for illustration purposes). The contributions over time were plotted as a function of time and trials (see legend).
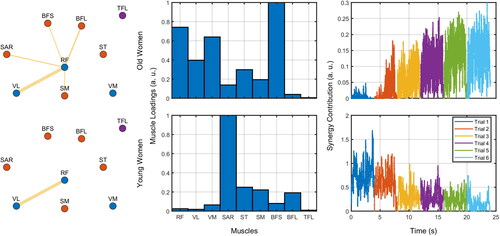
Figure 8. Number of synergies, participating muscles and synergy contribution per group, leg, and blocks. The dots represent each individual.
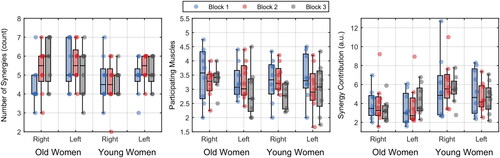
The number of synergies did not differ between groups, legs, or blocks. For the number of muscles contributing to a synergy, we did not find any significant effect. For the contribution of each synergy over time, we found that young women had larger synergy contribution than old women (5.31 ± 2.14 vs. 3.76 ± 1.62; Q = 7.85; p=.036, ξ = 0.55).
Considering the network measures, we found that for betweenness-centrality, there were no significant effects. For the clustering coefficient, we found a significant effect of blocks (Q = 5.53; p=.029; ξ = 0.53) provided a decrease in value from the first (0.46 ± 0.14) to the second (0.33 ± 0.11) and third blocks (0.34 ± 0.12) (p’s<.001). For global efficiency, we found similar results as the clustering coefficient. There was a significant effect of blocks (Q = 5.53; p=.018; ξ = 0.54) provided a decrease in value from the first (0.40 ± 0.11) to the second (0.29 ± 0.09) and third blocks (0.30 ± 0.10) (p’s<.001).
4. Discussion
The present study explored the motor control strategies adopted by young and older women in a redundant co-contraction task. We found that young and old women demonstrated similar number of synergies, differed in the high-frequency related synergies, and in overall muscle activation. Notably, the differences in high frequencies were demonstrated through the network measures—which highlights the utility of the method. The differences, however, vanished when averaging the network measures (unmatched frequencies) or not considering the multi time scales of muscle control (time-based synergies).
As expected, older women demonstrated lower peak torque than younger ones (as in Fiogbé et al., Citation2021). Aging is accompanied by changes in the neuromusculoskeletal system (Clark & Manini, Citation2008), leading to a decrease in muscle strength (Brooks & Faulkner, Citation1994). Young and healthy individuals demonstrate a great ability to adapt force output, quickly and accurately, in response to a stimulus (Vaillancourt & Newell, Citation2003). Older people, nonetheless, have less ability to generate appropriate force outcomes; observation related to loss of synchronization of motor unit firing, variability in motor unit discharge rate, reduced size of neurons, reduced number of synapses, and lower levels of neurotransmitters (Enoka et al., Citation2003): a process of loss of complexity in the neuromuscular system (Lipsitz & Goldberger, Citation1992; Pincus, Citation1994). This might explain our results on variability of EMG activation between muscles, where old women showed larger variability than young ones (see also Kang & Dingwell, Citation2009). It is important to highlight that the mentioned factors/mechanisms possibly related to torque loss and/or variability may vary depending on the level of physical activity. Our study considered only active individuals.
A striking result is the commonality between young and old women in most comparisons. Old women showed similar “structural” properties in most synergies: the same number of synergies when these were free to vary between participants (unmatched frequency-based and time-based synergies) and contributing muscles per synergy (time-based synergies). Additionally, when block to block analyses were performed (for the time-based synergies), old and young women changed similarly over time. These results contrast many studies that showed that coordination, in general (Ranganathan et al., Citation2019; Shinohara et al., Citation2003) and between muscles (Casamento-Moran et al., Citation2017; Da Silva Costa et al., Citation2020), differ between these age groups. In these studies, old adults showed less compensation between components to achieve a task requirement (Shinohara et al., Citation2004), were not able to explore new coordination modes (Ranganathan et al., Citation2019), and employed fewer synergies (Da Silva Costa et al., Citation2020; Ranganathan et al., Citation2019).
A potential explanation for this difference is the fact that the co-contraction task largely differs in terms of its requirements from the tasks studied before. Most of these earlier tasks require precision on a given outcome which is achieved through modification of the intrinsic tendencies of the system. In Da Silva Costa et al. (Citation2020), participants had to walk over a narrow surface–modifying the usual walking dynamics–where young adults managed to make it better by increasing the number of employed synergies. This has been observed in a few studies comparing young and old adults: older adults are less adaptive to new task demands (Sosnoff & Newell, Citation2006, Citation2008; Vaillancourt & Newell, Citation2003). The co-contraction paradigm, nevertheless, despite its uncommon nature for strength training, involves a common (and even simplistic) control strategy: increase apparent stiffness around joints by increasing co-activation of antagonist muscles. Indeed, old adults seem to rely more on such a strategy than young adults (Piche et al., Citation2022; Seidler et al., Citation2002).
The second relevant result was that young and old women differed in terms of the magnitude of recruitment of synergies. This result could be interpreted as, despite general coordination between age groups being similar, old women would not be able to “parameterize” these to a high magnitude of activation (modify its “gain”). In line with the discussion of the general similarities between young and old women above, it is possible to state that old adults are able to employ the same coordination for co-contraction but fail to adapt to the new task requirements (Vaillancourt & Newell, Citation2003).
Maximum co-contraction was not, to our knowledge, studied comparing young and old adults. However, the lack of adaptability demonstrated in our study (low EMG amplitude) is also found in other paradigms. For instance, Pijnappels et al. (Citation2006) found that, when anticipating a possible trip while walking, young adults, but not older ones, increased co-contraction. Alizadehsaravi et al. (Citation2020) found that, when varying the surface compliance in postural control, young adults increased the co-contraction of leg muscles while old adults showed higher co-contraction magnitudes overall (see also Iwamoto et al., Citation2017). Thus, despite previous studies claiming that a higher level of co-contraction is a common feature of old adults’ control (reaching: Seidler et al., Citation2002; walking: Piche et al., Citation2022); we believe that this depends on the task constraints (Vaillancourt & Newell, Citation2003). This seems to be an issue of lack of adaptability to decrease or increase the levels of co-contraction rather than a larger general higher co-contraction response.
From all network measures extracted from matched and unmatched frequency between participants, we saw that similar coherence profiles can be found. Using the standard coherence profiles of the matched frequencies, we found synergies at 0.1–4Hz, 0.1–40Hz, and 14–250Hz. The unmatched-frequencies showed more specific-band profiles–most profiles showing lower frequencies than 50 Hz peaks. These profiles are way broader than previous studies (see, for instance, Boonstra et al., Citation2015; Farmer et al., Citation2007; Kerkman et al., Citation2018)–but these also considered limited spectra (up to 60 Hz) and different tasks (postural control and reaching).
Considering the coherence outcome directly (), old women demonstrated lower coherence at higher frequencies. Despite the lack of inferential statistics on coherence in the present paper, one could claim that this is contrary to Obata et al. (Citation2014) findings: in quiet standing, old adults showed larger coherence at 8 and 12 Hz compared to younger ones (see Walker et al., Citation2018). Note that shows decreased coherence magnitude in frequencies higher than 12 Hz for older women. A potential reason for such changes comes from the fact that aging leads to a reduction in type II muscle fibers–those with higher firing rates (Nilwik et al., Citation2013). The loss of these fibers is not homogeneous in different muscles; mainly because muscles have different quantities of these fibers to start with. Indeed, Watanabe et al. (Citation2016) showed lower firing rates for the vastus lateralis in different force requirements for older adults. Considering such a structural change, old individuals would show larger variability in the remaining fast firing rate fibers leading to lower coherence in high firing rates (higher frequencies). Thus, this would represent a structural constraint for older adults when trying to achieve maximum contraction.
When considering what was demonstrated in terms of network measures, it was in high frequency synergies that old women differed. Specifically, they differed in terms of the clustering coefficient. It means that there are more groupings of muscles that are separated in the network than considering young women. In other words, the emergent synergy is more integrated in younger women than in older women. The fact that global efficiency also differed for higher frequencies likewise points in this direction.
The present results must be cautiously considered. First, this is an exploratory study and just indicates a direction for further research. We attempted to study emergent coordination muscle networks without a priori assumption on the best methodology to do so. We explored three potential paths (frequency-matched, unmatched-frequencies, and time-based synergies) considering three common network measurements. Despite this, we found commonalities that can be further considered. Second, we have limited power in our analyses given the small sample size. Additionally, we decided to be conservative in our inferences by addressing multiple comparisons in our analyses. Thus, many differences between age groups must be readdressed since they were borderline in many cases.
Supplemental Material
Download MS Word (29.3 KB)DISCLOSURE STATEMENT
No potential conflict of interest was reported by the author(s).
DATA AVAILABILITY STATEMENT
The data and codes are available at the Open Science Foundation website associated with the project (doi: 10.17605/OSF.IO/3YVSN)
Additional information
Funding
References
- Alizadehsaravi, L., Bruijn, S. M., Maas, H., & Van Dieën, J. H. (2020). Modulation of soleus muscle H-reflexes and ankle muscle co-contraction with surface compliance during unipedal balancing in young and older adults. Experimental Brain Research, 238(6), 1371–1383. https://doi.org/10.1007/s00221-020-05784-0
- Barabási, A. L. (2015). Network science. Cambridge University Press.
- Bartsch, R. P., Liu, K. K. L., Bashan, A., & Ivanov, P. C. (2015). Network physiology: How organ systems dynamically interact. PLOS ONE, 10(11), e0142143. https://doi.org/10.1371/journal.pone.0142143
- Bernstein, N. A. (1967). The co-ordination and regulation of movements. Pergamon.
- Bizzi, E., & Cheung, V. C. K. (2013). The neural origin of muscle synergies. Frontiers in Computational Neuroscience, 7, 51. https://doi.org/10.3389/fncom.2013.00051
- Boonstra, T. W., & Breakspear, M. (2012). Neural mechanisms of intermuscular coherence: Implications for the rectification of surface electromyography. Journal of Neurophysiology, 107(3), 796–807. https://doi.org/10.1152/jn.00066.2011
- Boonstra, T. W., Danna-Dos-Santos, A., Xie, H.-B., Roerdink, M., Stins, J. F., & Breakspear, M. (2015). Muscle networks: Connectivity analysis of EMG activity during postural control. Scientific Reports, 5(1), 17830. https://doi.org/10.1038/srep17830
- Brooks, S. V., & Faulkner, J. A. (1994). Skeletal muscle weakness in old age: Underlying mechanisms. Medicine and Science in Sports and Exercise, 26(4), 432–439. https://doi.org/10.1249/00005768-199404000-00006
- Bullmore, E., & Sporns, O. (2009). Complex brain networks: Graph theoretical analysis of structural and functional systems. Nature Reviews-Neuroscience, 10(3), 186–198. https://doi.org/10.1038/nrn2575
- Casamento-Moran, A., Chen, Y.-T., Lodha, N., Yacoubi, B., & Christou, E. A. (2017). Motor plan differs for young and older adults during similar movements. Journal of Neurophysiology, 117(4), 1483–1488. https://doi.org/10.1152/jn.00640.2016
- Cheung, V. C. K., d‘Avella, A., Tresch, M. C., & Bizzi, E. (2005). Central and sensory contributions to the activation and organization of muscle synergies during natural motor behaviors. The Journal of Neuroscience, 25(27), 6419–6434. https://doi.org/10.1523/JNEUROSCI.4904-04.2005
- Clark, B. C., & Manini, T. M. (2008). Sarcopenia != dynapenia. The Journals of Gerontology. Series A, Biological Sciences and Medical Sciences, 63(8), 829–834. https://doi.org/10.1093/gerona/63.8.829
- Cramer, A. O. J., Van Ravenzwaaij, D., Matzke, D., Steingroever, H., Wetzels, R., Grasman, R. P. P. P., Waldorp, L. J., & Wagenmakers, E.-J. (2016). Hidden multiplicity in exploratory multiway ANOVA: Prevalence and remedies. Psychonomic Bulletin & Review, 23(2), 640–647. https://doi.org/10.3758/s13423-015-0913-5
- d‘Avella, A., & Bizzi, E. (2005). Shared and specific muscle synergies in natural motor behaviors. Proceedings of the National Academy of Sciences of the United States of America, 102(8), 3076–3081. https://doi.org/10.1073/pnas.0500199102
- d‘Avella, A., Saltiel, P., & Bizzi, E. (2003). Combinations of muscle synergies in the construction of a natural motor behavior. Nature Neuroscience, 6(3), 300–308. https://doi.org/10.1038/nn1010
- Da Silva Costa, A. A., Hortobágyi, T., Den Otter, R., Sawers, A., & Moraes, R. (2023). Age, cognitive task, and arm position differently affect muscle synergy recruitment but have similar effects on walking balance. Neuroscience, 527, 11–21. https://doi.org/10.1016/j.neuroscience.2023.07.010
- Da Silva Costa, A. A., Moraes, R., Hortobágyi, T., & Sawers, A. (2020). Older adults reduce the complexity and efficiency of neuromuscular control to preserve walking balance. Experimental Gerontology, 140, 111050. https://doi.org/10.1016/j.exger.2020.111050
- Edström, E., Altun, M., Bergman, E., Johnson, H., Kullberg, S., Ramírez-León, V., & Ulfhake, B. (2007). Factors contributing to neuromuscular impairment and sarcopenia during aging. Physiology & Behavior, 92(1–2), 129–135. https://doi.org/10.1016/j.physbeh.2007.05.040
- Enoka, R. M., Christou, E. A., Hunter, S. K., Kornatz, K. W., Semmler, J. G., Taylor, A. M., & Tracy, B. L. (2003). Mechanisms that contribute to differences in motor performance between young and old adults. Journal of Electromyography and Kinesiology, 13(1), 1–12. https://doi.org/10.1016/S1050-6411(02)00084-6
- Farina, D., Negro, F., & Jiang, N. (2013). Identification of common synaptic inputs to motor neurons from the rectified electromyogram. The Journal of Physiology, 591(10), 2403–2418. https://doi.org/10.1113/jphysiol.2012.246082
- Farmer, S. F. (1998). Rhythmicity, synchronization and binding in human and primate motor systems. The Journal of Physiology, 509(Pt 1), 3–14. https://doi.org/10.1111/j.1469-7793.1998.003bo.x
- Farmer, S. F., Gibbs, J., Halliday, D. M., Harrison, L. M., James, L. M., Mayston, M. J., & Stephens, J. A. (2007). Changes in EMG coherence between long and short thumb abductor muscles during human development: Development of human EMG coherence. The Journal of Physiology, 579(Pt 2), 389–402. https://doi.org/10.1113/jphysiol.2006.123174
- Feldman, A. G. (2015). Referent control of action and perception: Challenging conventional theories in behavioral neuroscience. Springer.
- Fiogbé, E., Vassimon-Barroso, V., Catai, A. M., De Melo, R. C., Quitério, R. J., Porta, A., & Takahashi, A. C. D. M. (2021). Complexity of knee extensor torque: Effect of aging and contraction intensity. Journal of Strength and Conditioning Research, 35(4), 1050–1057. https://doi.org/10.1519/JSC.0000000000002888
- Hermens, H. J., Freriks, B., Disselhorst-Klug, C., & Rau, G. (2000). Development of recommendations for SEMG sensors and sensor placement procedures. Journal of Electromyography and Kinesiology, 10(5), 361–374. https://doi.org/10.1016/S1050-6411(00)00027-4
- Hug, F., Vecchio, A. D., Avrillon, S., Farina, D., & Tucker, K. (2021). Muscles from the same muscle group do not necessarily share common drive: Evidence from the human triceps surae. Journal of Applied Physiology, 130(2), 342–354. https://doi.org/10.1152/japplphysiol.00635.2020
- Ivanov, P. C. (2021). The new field of network physiology: Building the human physiolome. Frontiers in Network Physiology, 1, 711778. https://doi.org/10.3389/fnetp.2021.711778
- Iwamoto, Y., Takahashi, M., & Shinkoda, K. (2017). Differences of muscle co-contraction of the ankle joint between young and elderly adults during dynamic postural control at different speeds. Journal of Physiological Anthropology, 36(1), 32. https://doi.org/10.1186/s40101-017-0149-3
- Kang, H. G., & Dingwell, J. B. (2009). Dynamics and stability of muscle activations during walking in healthy young and older adults. Journal of Biomechanics, 42(14), 2231–2237. https://doi.org/10.1016/j.jbiomech.2009.06.038
- Kerkman, J. N., Daffertshofer, A., Gollo, L. L., Breakspear, M., & Boonstra, T. W. (2018). Network structure of the human musculoskeletal system shapes neural interactions on multiple time scales. Science Advances, 4(6), eaat0497. https://doi.org/10.1126/sciadv.aat0497
- Kostrubiec, V., Zanone, P.-G., Fuchs, A., & Kelso, J. A. S. (2012). Beyond the blank slate: Routes to learning new coordination patterns depend on the intrinsic dynamics of the learner—experimental evidence and theoretical model. Frontiers in Human Neuroscience, 6, 222. https://doi.org/10.3389/fnhum.2012.00222
- Lipsitz, L. A., & Goldberger, A. L. (1992). Loss of “complexity” and aging. Potential applications of fractals and chaos theory to senescence. JAMA, 267(13), 1806–1809. https://doi.org/10.1001/jama.1992.03480130122036
- Maeo, S., Yoshitake, Y., Takai, Y., Fukunaga, T., & Kanehisa, H. (2013). Effect of short-term maximal voluntary co-contraction training on neuromuscular function. International Journal of Sports Medicine, 35(2), 125–134. https://doi.org/10.1055/s-0033-1349137
- Maeo, S., Yoshitake, Y., Takai, Y., Fukunaga, T., & Kanehisa, H. (2014). Neuromuscular adaptations following 12-week maximal voluntary co-contraction training. European Journal of Applied Physiology, 114(4), 663–673. https://doi.org/10.1007/s00421-013-2801-x
- Myers, L. J., Lowery, M., O'Malley, M., Vaughan, C. L., Heneghan, C., St Clair Gibson, A., Harley, Y. X. R., & Sreenivasan, R. (2003). Rectification and non-linear pre-processing of EMG signals for cortico-muscular analysis. Journal of Neuroscience Methods, 124(2), 157–165. https://doi.org/10.1016/S0165-0270(03)00004-9
- Nilwik, R., Snijders, T., Leenders, M., Groen, B. B. L., Van Kranenburg, J., Verdijk, L. B., & Van Loon, L. J. C. (2013). The decline in skeletal muscle mass with aging is mainly attributed to a reduction in type II muscle fiber size. Experimental Gerontology, 48(5), 492–498. https://doi.org/10.1016/j.exger.2013.02.012
- Obata, H., Abe, M. O., Masani, K., & Nakazawa, K. (2014). Modulation between bilateral legs and within unilateral muscle synergists of postural muscle activity changes with development and aging. Experimental Brain Research, 232(1), 1–11. https://doi.org/10.1007/s00221-013-3702-2
- Pacheco, M. M., Lafe, C. W., & Newell, K. M. (2019). Search strategies in the perceptual-motor workspace and the acquisition of coordination, control, and skill. Frontiers in Psychology, 10(AUG), 1874. https://doi.org/10.3389/fpsyg.2019.01874
- Pacheco, M. M., & Newell, K. M. (2018). Transfer of a learned coordination function: Specific, individual and generalizable. Human Movement Science, 59, 66–80. https://doi.org/10.1016/j.humov.2018.03.019
- Perotto, A. O. (2011). Anatomical guide for the electromyographer. Chales C Thomas.
- Piche, E., Chorin, F., Zory, R., Duarte Freitas, P., Guerin, O., & Gerus, P. (2022). Metabolic cost and co-contraction during walking at different speeds in young and old adults. Gait & Posture, 91, 111–116. https://doi.org/10.1016/j.gaitpost.2021.10.014
- Pijnappels, M., Bobbert, M. F., & Van Dieën, J. H. (2006). EMG modulation in anticipation of a possible trip during walking in young and older adults. Journal of Electromyography and Kinesiology, 16(2), 137–143. https://doi.org/10.1016/j.jelekin.2005.06.011
- Pincus, S. M. (1994). Greater signal regularity may indicate increased system isolation. Mathematical Biosciences, 122(2), 161–181. https://doi.org/10.1016/0025-5564(94)90056-6
- Ranganathan, R., Lee, M.-H., Padmanabhan, M. R., Aspelund, S., Kagerer, F. A., & Mukherjee, R. (2019). Age-dependent differences in learning to control a robot arm using a body-machine interface. Scientific Reports, 9(1), 1960. https://doi.org/10.1038/s41598-018-38092-3
- Rizzo, R., Zhang, X., Wang, J. W. J. L., Lombardi, F., & Ivanov, P. C. (2020). Network physiology of cortico–muscular interactions. Frontiers in Physiology, 11, 558070. https://doi.org/10.3389/fphys.2020.558070
- Rubinov, M., & Sporns, O. (2010). Complex network measures of brain connectivity: Uses and interpretations. NeuroImage, 52(3), 1059–1069. https://doi.org/10.1016/j.neuroimage.2009.10.003
- Sawers, A., Pai, Y. C. C., Bhatt, T., & Ting, L. H. (2017). Neuromuscular responses differ between slip-induced falls and recoveries in older adults. Journal of Neurophysiology, 117(2), 509–522. https://doi.org/10.1152/jn.00699.2016
- Seidler, R. D., Alberts, J. L., & Stelmach, G. E. (2002). Changes in multi-joint performance with age. Motor Control, 6(1), 19–31. https://doi.org/10.1123/mcj.6.1.19
- Seidler, R. D., Bernard, J. A., Burutolu, T. B., Fling, B. W., Gordon, M. T., Gwin, J. T., Kwak, Y., & Lipps, D. B. (2010). Motor control and aging: Links to age-related brain structural, functional, and biochemical effects. Neuroscience and Biobehavioral Reviews, 34(5), 721–733. https://doi.org/10.1016/j.neubiorev.2009.10.005
- Shinohara, M., Li, S., Kang, N., Zatsiorsky, V. M., & Latash, M. L. (2003). Effects of age and gender on finger coordination in MVC and submaximal force-matching tasks. Journal of Applied Physiology, 94(1), 259–270. https://doi.org/10.1152/japplphysiol.00643.2002
- Shinohara, M., Scholz, J. P., Zatsiorsky, V. M., & Latash, M. L. (2004). Finger interaction during accurate multi-finger force production tasks in young and elderly persons. Experimental Brain Research, 156(3), 282–292. https://doi.org/10.1007/s00221-003-1786-9
- Silva, N. R. S., Pacheco, M. M., Fujita, R. A., Villalba, M. M., & Gomes, M. M. (2023). Individual control strategies in training: Myoelectric activity and recruitment strategies in the co-contraction training. Brazilian Journal of Motor Behavior, 17(5), 228–237. https://doi.org/10.20338/bjmb.v17i5.386
- Sosnoff, J. J., & Newell, K. M. (2006). The generalization of perceptual-motor intra-individual variability in young and old adults. The Journals of Gerontology-Series B, Psychological Sciences and Social Sciences, 61(5), P304–P310. https://doi.org/10.1093/geronb/61.5.P304
- Sosnoff, J. J., & Newell, K. M. (2008). Age-related loss of adaptability to fast time scales in motor variability. The Journals of Gerontology-Series B, Psychological Sciences and Social Sciences, 63(6), P344–P352. https://doi.org/10.1093/geronb/63.6.P344
- Stegeman, D. F., van de Ven, W. J. M., van Elswijk, G. A., Oostenveld, R., & Kleine, B. U. (2010). The α-motoneuron pool as transmitter of rhythmicities in cortical motor drive. Clinical Neurophysiology, 121(10), 1633–1642. https://doi.org/10.1016/j.clinph.2010.03.052
- Stins, J. F., Roerdink, M., & Beek, P. J. (2011). To freeze or not to freeze? Affective and cognitive perturbations have markedly different effects on postural control. Human Movement Science, 30(2), 190–202. https://doi.org/10.1016/j.humov.2010.05.013
- Vaillancourt, D. E., & Newell, K. M. (2003). Aging and the time and frequency structure of force output variability. Journal of Applied Physiology, 94(3), 903–912. https://doi.org/10.1152/japplphysiol.00166.2002
- Valk, T. A., Mouton, L. J., Otten, E., & Bongers, R. M. (2019). Fixed muscle synergies and their potential to improve the intuitive control of myoelectric assistive technology for upper extremities. Journal of NeuroEngineering and Rehabilitation, 16, 6. https://doi.org/10.1186/s12984-018-0469-5
- Walker, S., Avela, J., Wikgren, J., Meeusen, R., Piitulainen, H., Baker, S. N., & Parviainen, T. M. (2018). Aging and strength training influence knee extensor intermuscular coherence during low- and high-force isometric contractions. Frontiers in Physiology, 9, 1933. https://doi.org/10.3389/fphys.2018.01933
- Watanabe, K., Holobar, A., Kouzaki, M., Ogawa, M., Akima, H., & Moritani, T. (2016). Age-related changes in motor unit firing pattern of vastus lateralis muscle during low-moderate contraction. AGE, 38(3), 48. https://doi.org/10.1007/s11357-016-9915-0
- Wilcox, R. (2012). Introduction to robust estimation and hypothesis testing. Elsevier.