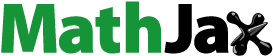
ABSTRACT
This study examined the associations of long-term exposure to ambient fine particulate matter (PM2.5) compositions/ozone with methylation of peripheral brain-derived neurotrophic factor (BDNF) promoters. A total of 101 participants were recruited from a cohort in Shijiazhuang, Hebei province, China. They underwent baseline and follow-up surveys in 2011 and 2015. DNA methylation levels were detected by bisulfite-PCR amplification and pyrosequencing. Participants‘ three-year average levels of PM2.5 compositions and ozone were estimated. Bayesian kernel machine regression (BKMR) models were used to examine the joint effects of pollutants on methylation levels. Exposure to PM2.5 compositions and ozone mixtures at the 75th percentile was associated with increased methylation levels at CpG2 of BDNF promoter (203%, 95% CI: 89, 316) than the lowest level of exposure, and sulfate dominated the effect in the BKMR models.Our findings provide clues to the epigenetic mechanisms for the associations of PM2.5 compositions and ozone with BDNF.
Introduction
Brain-derived neurotrophic factor (BDNF), as one neurotrophins, plays an essential role in synaptic plasticity, survival and maintenance of various types of neurons, and long-term hippocampal enhancement. A reduction of BDNF in the brain can impede synaptic plasticity, leading to an increased risk of cognitive decline, depression and Alzheimer’s disease (AD) (Franzmeier et al. Citation2021). DNA methylation is an important epigenetic mechanism regulating gene, and DNA methylation of BDNF promoter decrease the mRNA or protein levels in the hippocampus or frontal cortex of the brain (Fransquet et al. Citation2020). Further, BDNF promoter methylation can serve as an epigenetic biomarker to predict diseases, such as depression, dementia and cognitive impairment (Fransquet et al. Citation2018; Poon et al. Citation2021). In fact, some studies have investigated the association between air pollution exposure and BDNF levels. It has been shown that increased level of exposure to air pollutants, such as particulate matter and ozone was associated with decreased BDNF expression and levels, and the main mechanisms underlying the associations were related to inflammation and oxidative stress (Zhou et al. Citation2020; Cassilhas et al. Citation2021). However, limited studies considered the epigenetic modifications for the associations.
Health effects of ambient particulate matter ≤2.5 μm in diameter (PM2.5) and ozone (O3) are currently of great global concern. As major compositions of PM2.5, sulfate (SO42-), nitrate (NO3−), ammonium (NH4+), organic matter (OM) and black carbon (BC) can all play important roles in the health effects of PM2.5 (Huang et al. Citation2019). Previous studies have indicated that PM2.5 and O3 co-exposure could increase risks of health outcomes (Siddika et al. Citation2019). Furthermore, PM2.5 and O3 can mutually influence each other’s concentration in photochemical reactions. Studying their interaction effects can provide valuable information for devising effective strategies for the joint control of these two pollutants (Deng et al. Citation2022). Some studies have examined the individual health effect of O3 and PM2.5 compositions, but research on their interactive effects is limited (Lin et al. Citation2019). Moreover, previous studies have reported that the secretion of BDNF was inhibited, upon exposure to PM2.5 compositions and O3 (Rose et al. Citation2020; Haghani et al. Citation2021; Song et al. Citation2022). However, few studies have focused on individual and joint effects of PM2.5 compositions and O3, and their interactive effects on DNA methylation of BDNF promoter (Zhou et al. Citation2020).
This study aims to examine the associations of long-term exposure to PM2.5 compositions and O3 with DNA methylation of BDNF promoter and their interactive effects among people in Shijiazhuang, Hebei Province, China. This study can contribute to the development of strategies aimed at preventing nervous system diseases by effectively managing exposure to PM2.5 compositions and O3 during the initial stages of these conditions.
Material and methods
Study population
We randomly selected participants as a panel from the Mild cognitive impairment and Alzheimer’s disease Study in Hebei Province (MASHB), China. Details of the MASHB study are provided in the Section “Study population” of the supporting information. In brief, the MASHB study used a four-stage sampling process to select 3,240 participants aged ≥60 years from 36 communities of 4 cities in Hebei Province, China. Among the participants, 265 individuals were excluded, who declined participation, were unable to reached, or passed away. Additionally, 549 with nervous system diseases, poor hearing and vision, dementia, or psychiatric disorders were also excluded. Finally, the MASHB study included 2,426 participants (Xu et al. Citation2014). In order to facilitate sample collection and save sample collection cost, we randomly selected 101 participants from the 810 participants in Shijiazhuang City, who were part of the MASHB study. The sample selection process and participants’ residential addresses are presented in Figure S1, S2 in the supporting information. All 101 participants completed a baseline survey via a face-to-face interview with well-trained investigators in 2011. We collected information including sex, age, educational attainment, occupation, smoking status, physical activity (the frequency, duration, and intensity of physical activity per week), and histories of diabetes, respiratory diseases, and cardiovascular diseases. In addition, whole blood samples were collected from all participants in both 2011 and 2015 and stored at −20°C for further analysis. All participants provided written informed consent. The protocol of this study was approved by the Ethics Committee of the First Hospital of Hebei Medical University (2011001).
Exposure measurements
The data on participants’ exposures to PM2.5 compositions during the three years before 2011 or 2015 were obtained from Tracking Air Pollution (TAP, http://tapdata.org.cn) in China. Details about TAP have been reported (Geng et al. Citation2017). In brief, TAP project has developed a machine learning model to predict daily PM2.5 concentrations at a spatial resolution of 10 km across China. The data sources include ground PM2.5 measurements, satellite-derived aerosol optical depth (AOD) retrievals, meteorological reanalysis data, land use information, etc. Then, the TAP project developed PM2.5 composition data set based on the above 10-km PM2.5 grided dataset. Original conversion factors between PM2.5 concentration and PM2.5 compositions were simulated using an atmospheric chemical transport model. Combined with ground measurement data from the air monitoring stations, revised conversion factors between PM2.5 concentration with PM2.5 compositions were obtained based on a machine learning algorithm, and then near real-time concentration data on major chemical compositions [sulfate (SO42-), nitrate (NO3−), ammonium (NH4+), organic matter (OM) and black carbon (BC)] was obtained. The chemical compositions estimated by TAP had good consistency with ground measurements, with correlation coefficient in the range of 0.65 to 0.75 for five compositions. Finally, we used participants’ geolocation information of residential addresses (longitude and latitude coordinates) to estimate daily levels of PM2.5 and five compositions based on above approaches. We aggregated these estimates into three-year average levels of exposure to PM2.5 compositions and used them in the final analyses (Chen et al. Citation2019).
The three-year average concentrations of ozone (O3) prior to date of whole blood collection were estimated using a satellite-based random forests model (Chen et al. Citation2021). In brief, the random forest model was developed by linking ground-monitored O3 from the air monitoring stations with the data of satellite-observed O3 column amount, meteorological variables, land use, vegetation, etc. The model was used to estimate surface O3 across China at a spatial resolution of 0.0625°. Further, we tested the predictive ability of the model using 10-fold cross-validation (CV). The results reported that the CV R2 value for estimated O3 was 84%, indicating good predictive ability. Finally, we used participants’ geolocation information of residential addresses (longitude and latitude coordinates) to estimate daily max 8-h average O3 levels using above models, and we aggregated these estimates into three-year averages (Chen et al. Citation2019).
BDNF promoter methylation detection
Details of the BDNF promoter methylation detection are provided in Section “BDNF promoter methylation detection” of the supporting information. In brief, we firstly extracted genomic DNA from whole-blood samples using a genomic DNA extraction kit. Then, we chose seven BDNF promoter CpG sites (CpG1 to 3 of promoter I and CpG4 to 7 sites of promoter IV) according to previous studies (Figure S3) (Ikegame et al. Citation2013). Finally, we conducted Bisulfite-PCR amplification and pyrosequencing to detect DNA methylation of above CpG sites using MethPrimer software and PSQ 96 MA instrument. The amplification primers are present in Table S1.
Statistical analyses
Single pollutant analyses
Linear mixed-effect models were used to examine the association of each air pollutant with BDNF methylation. We included participants’ ID number in the models as a random-effect variables. We adjusted a range of covariates including sex, age, educational attainment, occupation, smoking, physical activity [metabolic equivalent (MET)-min/week], respiratory disease, cardiovascular disease, and diabetes (Alemany et al. Citation2021). Given the skewed distribution of CpG methylation levels, the methylation levels were log-transformed. In addition, to examine the potential nonlinear associations, a natural cubic spline with 3 degrees of freedom (df) was used for each PM2.5 composition and O3 (Li et al. Citation2016).
Using linear mixed-effect models, we calculated the percent methylation changes and 95% confidence intervals (CI) associated with an interquartile range (IQR) increase in each composition/O3. We used the formula [100 × (expβ −1)] (where β represents coefficient from the models) to calculate percent changes (Li et al. Citation2019). Based on nonlinear mixed-effect models, we plotted the exposure-response curves for associations of PM2.5 compositions/O3 levels with methylation levels. We set the lowest points of above curves as references (zero percent change) when testing for increased methylation levels associated with PM2.5 compositions and O3, and vice versa (Li et al. Citation2016).
Joint effect analyses
Bayesian kernel machine regression (BKMR) models were used to examine associations of the mixtures of all measured chemical compositions and O3 with BDNF promoter methylation levels (Wang et al. Citation2020), which considers potential nonlinearities among the correlated pollutants. Details of the BKMR models have been previously reported as follows (Bobb et al. Citation2018):
where Y is the methylation level; Z is a vector of PM2.5 compositions and O3 assessed in this study; X is a range of the same covariates as those of the single pollutant analyses. The function h () is the Gaussian kernel function. Due to the high correlation between PM2.5 compositions (Figure S4), we used a hierarchical variable selection to fit the models, and scaled the exposure variables to facilitate efficient Markov Chain Monte Carlo (MCMC) sampling. We ran BKMR models with 10,000 iterations for methylation level at each CpG site (Peralta et al. Citation2021).
Based on BKMR models, we calculated the group posterior inclusion probability (groupPIP) and the conditional posterior inclusion probability (condPIP) for each pollutant through hierarchical variable selection. Then, we examined overall effects of air pollutant mixtures on methylation levels when the mixtures were fixed at specific percentiles (0th to 75th percentile, with 5% increments) compared to their lowest levels. Further, to examine the individual effect of each pollutant among mixtures, we compared the effect of each pollutant from 25th percentile to 75th percentile on BDNF methylation levels, when the other pollutants were fixed at 25th, 50th, and 75th percentiles.
Interaction and stratified analyses
To investigate the interactive effects of PM2.5 compositions and O3, associations between PM2.5 compositions and BDNF promoter methylation at different O3 exposure levels (O3 ≤75th percentile, O3 >75th percentile) were examined (Lin et al. Citation2019). Considering the possible modifications by demographic factors, we conducted analyses stratified by sex (male, female) and age (<75 years, ≥ 75 years).
Sensitivity analyses
To evaluate the robustness of the main results, we conducted several sensitivity analyses: (1) Since some covariates, such as occupation, smoking, and cardiovascular disease, were potential risk factors for BDNF expression (Pius-Sadowska and Machaliński Citation2017; Pivac et al. Citation2022), we developed models adjusted these covariables (model1) and models without these covariables adjusted (model2). (2) Considering the associations of respiratory diseases/diabetes with serum BDNF levels or BDNF DNA methylation (Liu et al. Citation2016; Karim et al. Citation2021), we excluded participants with respiratory diseases (n = 13, 12.9%) or diabetes (n = 12, 11.9%) in the analyses.
The statistical significance was set at a two tailed P < 0.05, except for interaction terms (two tailed P < 0.1). Statistical analyses were performed by R (version 4.1.2).
Results
Population characteristics
The baseline characteristics of 101 participants are summarized in . About 63.4% participants were female and all participants’ mean age was 69.2 ± 5.4 years. The majority of them never smoked (75.2%). In terms of disease histories, most participants had cardiovascular disease (71.3%), and a minority of them had respiratory disease (12.9%) and diabetes (11.9%).
Table 1. Baseline characteristics of 101 participants in this study.
Exposure and outcome assessment
Participants’ median levels of exposure to SO42-, NO3−, NH4+, OM, BC and O3 during the three years before the baseline survey (in 2011) were 15.59 μg/m3, 15.05 μg/m3, 10.23 μg/m3, 24.29 μg/m3, 4.92 μg/m3 and 97.20 μg/m3, respectively, and the levels of five compositions and O3 were significantly higher in the follow-up period (). Additionally, there were significant differences in the DNA methylation levels at CpG2, CpG4, and CpG5 between the baseline and follow-up periods, which may be due to air pollution as well as other underlying factors.
Table 2. Summary statistics of PM2.5 compositions, O3 and BDNF promoter methylation levels in this panel study.
Single pollutant analyses
Exposure to high levels of PM2.5 compositions were significantly associated with increased CpG2 methylation levels, and decreased CpG4 methylation levels (, Table S2). For instance, each interquartile range (IQR) increases of SO42- and NO3− were associated with increases of 63% (95%CI: 31, 95) and 48% (95%CI: 5, 90) in CpG2 methylation levels, respectively. While, each IQR increases of SO42-, NO3−, NH4+ and OM were associated with decreases of −125% (95%CI: −194, −55), −181% (95%CI: −270, −93), −153% (95%CI: −232, −74) and −103% (95%CI: −171, −36) in CpG4 methylation levels, respectively. O3 was not significantly associated with the methylation levels of any CpG sites. The non-linear exposure-response curves for associations of PM2.5 compositions/O3 with methylation levels were positive for CpG2, while negative for CpG4 and CpG5, and these showed consistent increases/decreases with no clear thresholds for the significant associations. The slopes of the curves seemed to flatten when the pollutant levels were above the 75th percentiles (Figure S5).
Table 3. The percent changes of BDNF promoter methylation levels associated with each interquartile range increase of PM2.5 compositions and O3..
Joint effect analyses
The groupPIPs and conPIPs are presented in Table S3. A threshold of 0.5 PIP value is used to determine the importance of a pollutant (Yu et al. Citation2021). The groupPIP and conPIP of SO42- (groupPIP: 0.995; conPIP: 0.977) were both higher than 0.5 for CpG2 methylation levels. However, both groupPIPs and conPIPs of PM2.5 compositions above 0.5 were not observed for CpG4 and CpG5 methylation levels.
The joint effects of five PM2.5 compositions and O3 on CpG2, CpG4 and CpG5 methylation levels are shown in . For instance, exposure to a high level of air pollutant mixtures (75th percentile) was associated with increased methylation levels at CpG2 [202.50% (95% CI: 89.14, 315.87)] and decreased methylation levels at CpG4 [−281.87% (95% CI −499.87, −63.88)] compared to their lowest levels (Table S4).
Figure 1. The joint and individual effects of PM2.5 compositions and O3 on BDNF promoter methylation using BKMR models.

Individual effect of each pollutant among mixtures are shown in . Fixing all other individual air pollutants at their 25th, 50th, and 75th percentiles in the BKMR models, SO42- was positively associated with methylation levels of CpG2. Further, the results indicated that the associations of each pollutant among mixtures with methylation levels were consistent with those when all other individual pollutants were fixed at different percentiles.
Interactive effects between PM2.5 compositions and O3
The associations between PM2.5 compositions and BDNF promoter methylation (CpG2) were stronger at high O3 exposure levels (O3 >75th percentile) (). For example, each IQR increase of SO42- was associated with a 30% (95%CI: 0, 59) increase of CpG2 methylation levels at high O3 exposure levels, and that was 2% (95%CI: −13, 52) at low O3 exposure levels (P-interaction = 0.088). Similar results were found for OM.
Figure 2. The percent changes of BDNF promoter methylation levels (CpG2) associated with each interquartile range increase of PM2.5 compositions (μg/m3) in different O3 exposure levels.
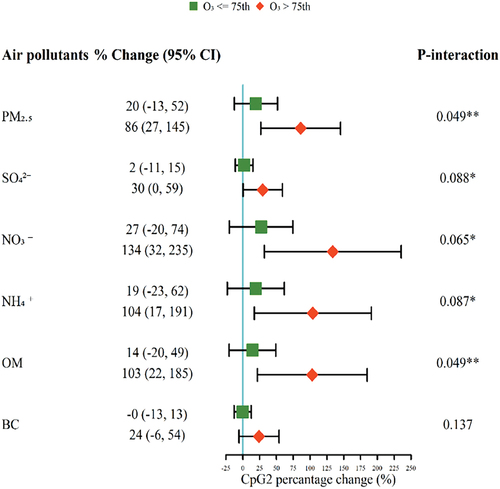
Stratified and sensitivity analyses
No significant modification effects were observed when the data were stratified by sex and age (Table S5, S6). For instance, each IQR increase of SO42- was related to a 54% (95%CI: 20, 88) increase of CpG2 methylation levels in females, and that was 86% (95%CI: 2, 171) in males (P-interaction = 0.5690). Consistency was observed between different models in the sensitivity analyses. For instance, a 63% (95%CI: 31, 95) change in CpG2 methylation level was associated with an IQR increase of SO42- in the model1, and that was 65% (95%CI: 32, 97) in the model2 (Table S8). Moreover, the most results did not substantial changed after excluding participants with respiratory diseases and diabetes (Table S7).
Discussion
This study examined the individual and joint effects of PM2.5 compositions and O3 on DNA methylation of BDNF promoter. High levels of SO42- and NO3− were associated with increased methylation level of CpG2 in promotor I and decreased methylation level of CpG4 in promotor IV. We also observed a positive correlation between air pollutant mixtures and CpG2 methylation levels, with SO42- dominating the effects of the mixtures. In addition, we observed synergistic effects of exposure to PM2.5 compositions and O3 on the BDNF promoter methylation, particularly at CpG2.
Evidence for the associations of PM2.5 compositions with BDNF promoter methylation is limited. However, some studies have indicated that exposure to particulate matter, including SO42-, NO3−, NH4+, OM or BC, could reduce the secretion of BDNF. For example, two animal experiments from China and USA reported that PM, containing more than 60% SO42-, NO3−, NH4+, BC, induced a general down-regulation of BDNF in mice (Liu et al. Citation2018; Haghani et al. Citation2021). Moreover, a panel study among 34 retirees in China reported that PM2.5 (including SO42- and NO3−) exposure reduced BDNF levels (Song et al. Citation2022). Further, many studies have shown that decreased BDNF expression in the brain regions have been linked to methylation of the BDNF gene (Polli et al. Citation2020). Therefore, the positive associations of the SO42- and NO3− with methylation at CpG2 in this study could be supported by the previous studies. However, to our knowledge, no previous studies reported negative associations between PM2.5 compositions and methylation of BDNF promoters or BDNF secretion. Discrepancies in the ranges of PM2.5 concentrations and sample sizes may contribute to the negative associations of SO42-, NO3−, NH4+ and OM with methylation levels at CpG4 and CpG5 in our study.
No significant associations between O3 and BDNF promoter methylation were observed in our study. However, an animal experiment from Canada reported that exposure to O3 (0.8 ppm for 4 h) reduced BDNF expression in the hippocampus of rats (Rose et al. Citation2020), while another experiment from Italy found that exposure to O3 (0.3 or 0.6 ppm from 30 days before breeding pairs until 17th gestational day) increased BDNF levels in the striatum of mice (Santucci et al. Citation2006). In brief, it is suggested that high levels of exposure to O3 inhibited BDNF expression, while low levels of exposure to O3 promoted BDNF expression. Compared to the above studies, the nonsignificant O3-methylation associations in this study could be due to discrepancies in ranges of O3 concentration and study designs.
To our knowledge, no evidence for joint effects of PM2.5 compositions and O3 on BDNF promoter methylation has been reported. The joint effect analyses in this study indicated that joint exposures to five compositions (SO42-, NO3−, NH4+, OM and BC) and O3 had positive associations with BDNF promoter methylation, especially at CpG2 site, and SO42- played an essential role in the association. Further, this study observed that PM2.5 compositions (e.g. SO42- and OM) and O3 had synergistic effects on BDNF promoter methylation, and the underlying mechanism was unclear. According to previous studies, it may be that O3 exposure can increase the permeability of the lung epithelium barrier, facilitating the direct absorption of particles into the circulatory system (Siddika et al. Citation2019).
Although the mechanisms for associations of BNDF methylation with exposure to PM2.5 compositions were unclear, there could be several possible mechanisms. For instance, upon exposure to SO42- in PM2.5, hypermethylation on the BNDF promoter could be induced by the activation of DNA methyltransferases (Zhou et al. Citation2020). Besides, upon exposure to the composition, S-adenosyl methionine can be activated and supply methyl groups to CpG of the promoter (Rider and Carlsten Citation2019). The silencing effect of hypermethylation on the BNDF promoter results in decreased BDNF levels (Polli et al. Citation2020). A reduction of BDNF in the brain can hinder synaptic plasticity and result in nervous system diseases, such as AD (Ng et al. Citation2019).
One implication of this study was that the results of our study provided clues to the epigenetic mechanisms underlying associations of PM2.5 with nervous system diseases. Previous studies have shown that DNA methylation of BDNF promoter can serve as an epigenetic biomarker to predict the onset of nervous system diseases (Fransquet et al. Citation2018). Thus, these findings can contribute to the establishment of strategies to prevent nervous system diseases by reducing the levels of exposure to PM2.5 compositions, especially for SO42-. For instance, previous studies have reported that the major sources of SO42- in PM2.5 are and industrial emissions and vehicle emissions, and effective measures (e.g. installation of flue-gas desulfurization and selective catalytic reduction equipment, and control of vehicle population) should be taken to control these emissions (Geng et al. Citation2017). In addition, this study observed that the co-exposure to PM2.5 and O3 could enhance the adverse health effects, and it may help make policies to simultaneously control both particulate matter and O3.
There are several strengths in this study. We have examined associations of long-term exposure to PM2.5 compositions/O3 with BDNF promoter methylation and their interactive effects, which have been seldom reported by prior studies. Moreover, as a panel study, this research considered variations both between individuals and within individuals. In addition, we used NanoDrop for quality control of DNA samples above 5 μg without degradation, and high-accuracy pyrosequencing for reliable analysis. It should be noted that there are also several limitations for this study. First, due to limited funding, we did not detect serum BDNF level or examine the mediation effect of methylation between air pollutants and BDNF. Further work should examine both BDNF and methylation levels to investigate the impact of air pollutants on BDNF, which may be mediated by methylation. Second, this study was conducted in Shijiazhuang City, which may not provide a comprehensive representation of China. Future research should encompass other regions and aggregate the findings for greater representativeness. Third, some covariates were not considered, such as body mass index, which are potentially associated with both air pollution and BDNF and may have impacts on the air pollution-methylation associations. Therefore, future studies should consider additional covariates to enhance the accuracy of examining these associations.
Conclusions
Overall, our findings suggested both individual and joint associations between long-term exposure to PM2.5 compositions (especially SO42-) and BDNF promoter methylation levels. Our study contributes valuable evidence to the interactive effects of PM2.5 and O3 on BDNF promoter methylation, which is relevant to nervous system diseases. These findings can inform the development of prevention strategies that involve the joint control of PM2.5 and O3. Moreover, further research is warranted to explore the associations of other compositions, such as perfluorochemicals and heavy metals, with BDNF promoter methylation across various populations and regions.
Supplemental Material
Download MS Word (1.9 MB)Disclosure statement
No potential conflict of interest was reported by the author(s).
Supplementary data
Supplemental data for this article can be accessed online at https://doi.org/10.1080/09603123.2023.2280157
Additional information
Funding
References
- Alemany S, Crous-Bou M, Vilor-Tejedor N, Milà-Alomà M, Suárez-Calvet M, Salvadó G, Cirach M, Arenaza-Urquijo EM, Sanchez-Benavides G, Grau-Rivera O, et al. 2021. Associations between air pollution and biomarkers of Alzheimer’s disease in cognitively unimpaired individuals. Environ Int. 157:106864. doi: 10.1016/j.envint.2021.106864.
- Bobb JF, Claus Henn B, Valeri L, Coull BA. 2018. Statistical software for analyzing the health effects of multiple concurrent exposures via Bayesian kernel machine regression. Environ Health. 17(1):67. doi: 10.1186/s12940-018-0413-y.
- Cassilhas RC, De Sousa RAL, Caxa L, Viana V, Meeusen R, Goncalves FL, Magalhaes CODE, Tufik S, Peixoto MFD, Monteiro RS, et al. 2021. Indoor aerobic exercise reduces exposure to pollution, improves cognitive function, and enhances BDNF levels in the elderly. Air Qual Atmos Hlth. 15(1):35–45. doi:10.1007/s11869-021-01083-x.
- Chen GB, Chen J, Dong GH, Yang BY, Liu YS, Lu TJ, Yu P, Guo YM, Li SS. 2021. Improving satellite-based estimation of surface ozone across China during 2008-2019 using iterative random forest model and high-resolution grid meteorological data. Sust Cities Soc. 69:9. doi: 10.1016/j.scs.2021.102807.
- Chen G, Xiang H, Mao Z, Huo W, Guo Y, Wang C, Li S. 2019. Is long-term exposure to air pollution associated with poor sleep quality in rural China? Environ Int. 133(Pt B):105205. doi: 10.1016/j.envint.2019.105205.
- Deng C, Tian S, Li Z, Li K. 2022. Spatiotemporal characteristics of PM(2.5) and ozone concentrations in Chinese urban clusters. Chemosphere. 295:133813. doi: 10.1016/j.chemosphere.2022.133813.
- Fransquet PD, Lacaze P, Saffery R, McNeil J, Woods R, Ryan J. 2018. Blood DNA methylation as a potential biomarker of dementia: a systematic review. Alzheimer’s & Dementia: The Journal Of The Alzheimer’s Association. 14(1):81–103. doi: 10.1016/j.jalz.2017.10.002.
- Fransquet PD, Ritchie K, Januar V, Saffery R, Ancelin ML, Ryan J, Mukaetova-Ladinska E. 2020. Is peripheral BDNF promoter methylation a preclinical biomarker of dementia? J Alzheimers Dis. 73(2):645–655. doi: 10.3233/jad-190738.
- Franzmeier N, Ren J, Damm A, Monté-Rubio G, Boada M, Ruiz A, Ramirez A, Jessen F, Düzel E, Rodríguez Gómez O, et al. 2021. The BDNF(Val66Met) SNP modulates the association between beta-amyloid and hippocampal disconnection in Alzheimer’s disease. Mol Psychiatry. 26(2):614–628. doi:10.1038/s41380-019-0404-6.
- Geng G, Zhang Q, Tong D, Li M, Zheng Y, Wang S, He K. 2017. Chemical composition of ambient PM2. 5 over China and relationship to precursor emissions during 2005–2012. Atmos Chem Phys. 17(14):9187–9203. doi: 10.5194/acp-17-9187-2017.
- Haghani A, Feinberg JI, Lewis KC, Ladd-Acosta C, Johnson RG, Jaffe AE, Sioutas C, Finch CE, Campbell DB, Morgan TE, et al. 2021. Cerebral cortex and blood transcriptome changes in mouse neonates prenatally exposed to air pollution particulate matter. J Neurodev Disord. 13(1):30. doi: 10.1186/s11689-021-09380-3.
- Huang X, Zhang B, Wu L, Zhou Y, Li Y, Mao X, Chen Y, Wang J, Luo P, Ma J, et al. 2019. Association of exposure to ambient fine particulate matter constituents with semen quality among men attending a fertility center in China. Environ Sci Technol. 53(10):5957–5965. doi:10.1021/acs.est.8b06942.
- Ikegame T, Bundo M, Sunaga F, Asai T, Nishimura F, Yoshikawa A, Kawamura Y, Hibino H, Tochigi M, Kakiuchi C, et al. 2013. DNA methylation analysis of BDNF gene promoters in peripheral blood cells of schizophrenia patients. Neurosci Res. 77(4):208–214. doi: 10.1016/j.neures.2013.08.004.
- Karim A, Muhammad T, Qaisar R. 2021. Prediction of Sarcopenia using multiple biomarkers of neuromuscular junction degeneration in chronic obstructive pulmonary disease. J Pers Med. 11(9):919. doi: 10.3390/jpm11090919.
- Li W, Dorans KS, Wilker EH, Rice MB, Ljungman PL, Schwartz JD, Coull BA, Koutrakis P, Gold DR, Keaney JF Jr., et al. 2019. Short-term exposure to ambient air pollution and circulating biomarkers of endothelial cell activation: the Framingham heart study. Environ Res. 171:36–43. doi: 10.1016/j.envres.2018.10.027.
- Li S, Guo Y, Williams G. 2016. Acute impact of hourly ambient air pollution on preterm birth. Environ Health Perspect. 124(10):1623–1629. doi: 10.1289/EHP200.
- Lin H, Guo Y, Ruan Z, Yang Y, Chen Y, Zheng Y, Cummings-Vaughn LA, Rigdon SE, Vaughn MG, Sun S, et al. 2019. Ambient PM2.5 and O3 and their combined effects on prevalence of presbyopia among the elderly: a cross-sectional study in six low- and middle-income countries. Sci Total Environ. 655:168–173. doi: 10.1016/j.scitotenv.2018.11.239.
- Liu W, Han X, Zhou X, Zhang S, Cai X, Zhang L, Li Y, Li M, Gong S, Ji L. 2016. Brain derived neurotrophic factor in newly diagnosed diabetes and prediabetes. Mol Cell Endocrinol. 429:106–113. doi: 10.1016/j.mce.2016.04.002.
- Liu X, Qian X, Xing J, Wang J, Sun Y, Wang Q, Li H. 2018. Particulate matter triggers depressive-like response associated with modulation of inflammatory cytokine homeostasis and brain-derived neurotrophic factor signaling pathway in mice. Toxicol Sci. 164(1):278–288. doi: 10.1093/toxsci/kfy086.
- Ng T, Ho C, Tam W, Kua E, Ho R. 2019. Decreased serum Brain-derived neurotrophic factor (BDNF) levels in patients with Alzheimer’s disease (AD): a systematic review and meta-analysis. Int J Mol Sci. 20(2):257. doi: 10.3390/ijms20020257.
- Peralta AA, Schwartz J, Gold DR, Coull B, Koutrakis P. 2021. Associations between PM(2.5) metal components and QT interval length in the normative aging study. Environ Res. 195:110827. doi: 10.1016/j.envres.2021.110827.
- Pius-Sadowska E, Machaliński B. 2017. BDNF - A key player in cardiovascular system. J Mol Cell Cardiol. 110:54–60. doi: 10.1016/j.yjmcc.2017.07.007.
- Pivac N, Nedic Erjavec G, Sagud M, Nikolac Perkovic M, Tudor L, Uzun S, Kovacic Petrovic Z, Konjevod M, Dvojkovic A, Kozumplik O, et al. 2022. The association between BDNF C270T genetic variants and smoking in patients with mental disorders and in healthy controls. Prog Neuropsychopharmacol Biol Psychiatry. 113:110452. doi: 10.1016/j.pnpbp.2021.110452.
- Polli A, Ghosh M, Bakusic J, Ickmans K, Monteyne D, Velkeniers B, Bekaert B, Godderis L, Nijs J. 2020. DNA methylation and brain-derived neurotrophic factor expression account for symptoms and widespread hyperalgesia in patients with chronic fatigue syndrome and comorbid fibromyalgia. Arthritis Rheumatol. 72(11):1936–1944. doi: 10.1002/art.41405.
- Poon CH, Heng BC, Lim LW. 2021. New insights on brain-derived neurotrophic factor epigenetics: from depression to memory extinction. Ann N Y Acad Sci. 1484(1):9–31. doi: 10.1111/nyas.14458.
- Rider CF, Carlsten C. 2019. Air pollution and DNA methylation: effects of exposure in humans. Clin Epigenetics. 11(1):131. doi: 10.1186/s13148-019-0713-2.
- Rose M, Filiatreault A, Guénette J, Williams A, Thomson EM. 2020. Ozone increases plasma kynurenine-tryptophan ratio and impacts hippoca mpal serotonin receptor and neurotrophic factor expression: role of st ress hormones. Environ Res. 185:109483. doi: 10.1016/j.envres.2020.109483.
- Santucci D, Sorace A, Francia N, Aloe L, Alleva E. 2006. Prolonged prenatal exposure to low-level ozone affects aggressive beha viour as well as NGF and BDNF levels in the central nervous system of. Behav Brain Res. 166(1):124–130. doi: 10.1016/j.bbr.2005.07.032.
- Siddika N, Rantala AK, Antikainen H, Balogun H, Amegah AK, Ryti NRI, Kukkonen J, Sofiev M, Jaakkola MS, Jaakkola JJK. 2019. Synergistic effects of prenatal exposure to fine particulate matter (PM2.5) and ozone (O3) on the risk of preterm birt h: a population-based cohort study. Environ Res. 176:108549. doi: 10.1016/j.envres.2019.108549.
- Song J, Qu R, Sun B, Chen R, Kan H, An Z, Jiang J, Li J, Zhang Y, Wu W. 2022. Associations of short-term exposure to fine particulate matter with neural damage biomarkers: a panel study of healthy retired adults. Environ Sci Technol. 56(11):7203–7213. doi: 10.1021/acs.est.1c03754.
- Wang C, Koutrakis P, Gao X, Baccarelli A, Schwartz J. 2020. Associations of annual ambient PM(2.5) components with DNAm PhenoAge acceleration in elderly men: the normative aging study. Environ Pollut. 258:113690. doi: 10.1016/j.envpol.2019.113690.
- Xu S, Xie B, Song M, Yu L, Wang L, An C, Zhu Q, Han K, Zhao X, Zhang R, et al. 2014. High prevalence of mild cognitive impairment in the elderly: a community-based study in four cities of the Hebei province, china. Neuroepidemiology. 42(2):123–130. doi: 10.1159/000357374.
- Yu S, Feng WR, Liang ZM, Zeng XY, Bloom MS, Hu GC, Zhou Y, Ou YQ, Chu C, Li QQ, et al. 2021. Perfluorooctane sulfonate alternatives and metabolic syndrome in adults: new evidence from the isomers of C8 health project in China. Environ Pollut. 283:117078. doi: 10.1016/j.envpol.2021.117078.
- Zhou Y, Zhang M, Liu W, Li Y, Qin Y, Xu Y. 2020. Transgenerational transmission of neurodevelopmental disorders induced by maternal exposure to PM2.5. Chemosphere. 255:126920. doi: 10.1016/j.chemosphere.2020.126920.