Abstract
Oil spills threaten the productivity of marine forage fish that spawn in shallow shoreline habitats. In western North America, this includes Pacific herring (Clupea pallasii), a keystone species for marine food webs. Crude oil-derived polycyclic aromatic hydrocarbons (PAHs) are highly toxic to herring embryos and larvae. Despite our understanding of individual-based toxicity, the impacts of oil spills at the population-scale remain uncertain. We modeled the response of Puget Sound herring stocks to simulated oil spills and found that declining stocks are less likely to withstand the short-term impacts of a small, localized spill. Moreover, conventional stock assessment methods may only detect large population declines in response to high rates of mortality in a single year, or sustained losses across multiple year classes. We discuss the importance of herring life history and environmental variation on the predictive usefulness of conventional population modeling as a basis for estimating injury to highly variable marine forage fish resources.
Introduction
Oil spills and Natural Resource Damage Assessments
Seabed oil development, marine and land-based (i.e., rail) transport, and industrial refining operations often occur in proximity to commercially and ecologically important fish populations, creating the potential for injury to these resources as a consequence of accidental spills. In the United States, a key impetus for characterizing the impacts of oil spills is the Natural Resource Damage Assessment and Restoration (NRDA) process (Burlington Citation2002). The goal of NRDA is to quantify spill-related injuries to natural resources and services so that appropriate restoration can occur. The toxicity of oil to individual fish is well known (Incardona and Scholz Citation2017, Citation2018; Incardona Citation2017). However, the links between individual-based injury and the productivity and demographic characteristics of wild populations and sub-populations (i.e., stocks) remain poorly understood (Heintz et al. Citation2000; Scholz and Incardona Citation2015; Fodrie et al. Citation2014). For example, the 1989 Exxon Valdez oil spill in Prince William Sound, Alaska caused extensive oiling of Pacific herring (Clupea pallasii) spawning habitat. Subsequent field assessments documented developmental abnormalities and larval mortality (Hose et al. Citation1996; McGurk and Brown Citation1996; Norcross et al. Citation1996), which were confirmed by laboratory toxicity tests (Marty et al. Citation1997; Carls et al. Citation1999; Incardona et al. Citation2015). However, the degree to which the spill contributed to the decline of the Prince William Sound herring stock and collapse of the fishery 3–4 years later is unresolved (Peterson et al. Citation2003; Short Citation2003; Thorne and Thomas Citation2008; Incardona et al. Citation2015; Ward et al. Citation2017). Following the Cosco Busan oil spill in 2007, injuries to herring eggs were documented at oiled spawning sites and an estimated 14% to 29% of the San Francisco Bay herring spawn deposition was impacted (Incardona et al. Citation2012a, Citation2012b; Cosco Busan Oil Spill Trustees Citation2012). However, population-level effects from the spill were not determined, despite record low spawning biomass and the closure of the commercial fishery in subsequent years, in part due to high variability in historical spawning records and the importance of other environmental factors in the development and growth of herring (Cosco Busan Oil Spill Trustees Citation2012). Population models that scale individual injuries in oil-exposed fish over space and time to changes in stock abundance and demographics could substantively inform post-spill injury assessments for NRDA and fisheries management decisions. Such models could also provide a framework for predicting the risks that future spills may pose to fisheries resources (Raimondo et al. Citation2006; Ohlberger and Langangen Citation2015; Langangen et al. Citation2017; Carroll et al. Citation2018; White et al. Citation2022; Langangen et al. Citation2023).
In the context of oil spill injury, environmental exposure conditions, cumulative toxic impact, and species life history characteristics are all key determinants of individual fitness and lifetime reproductive success and, by extension, population-level responses (Barron and Ka’aihue Citation2001; Barron et al. Citation2003; Barron Citation2017). Population models developed to inform oil spill injury assessments draw from conceptual models for each of the key determinants and link them to investigate potential population-level effects. Models may also include factors that are independent of a spill impact, such as water temperature, as well as those that may or may not be associated with a spill, such as prey availability or carrying capacity. Moving from conceptual relationships to a quantitative model requires quantifying the empirical data linking environmental exposure conditions, developmental toxicity, and aggregate outcomes at the population-scale. These relationships are informed by existing crude oil exposure-response information and the life history characteristics of the focal species. Demographic data are typically derived from field surveys collected over multiple years and are more available and comprehensive for commercially managed fisheries, such as Pacific herring. Field observations of oil deposition or oil transport and fate models can be utilized to generate exposure scenarios and projected outcomes to inform management, response, and assessment.
Oil toxicity to fish embryos and larvae
Fish embryos and larvae are particularly vulnerable to oil toxicity. This is in part because many marine species spawn in the upper water column or along shorelines, which are habitats where spilled oil is likely to accumulate and persist, increasing the exposure risk for resulting early life stages. Lipid-rich eggs and larvae readily accumulate oil chemicals, and metabolic detoxification pathways in the liver and other tissues do not fully mature until later in development (Incardona and Scholz Citation2017). Additionally, translucent embryonic and larval fish in the photic zone of the water column or intertidal areas are susceptible to photoenhanced toxicity during or after exposure to oil (Barron and Ka’aihue Citation2001; Barron et al. Citation2003; Barron Citation2017). Crude oil-derived polycyclic aromatic hydrocarbons (PAHs) are a driver of developmental toxicity in fish (Incardona et al. Citation2004; Barron et al. Citation2004); exposure during early development causes a range of morphological and bioenergetic effects that reduce the survival of marine fish through either acute larval lethality or latent reductions in fitness and productivity (Heintz et al. Citation2000; Incardona et al. Citation2004, Citation2009, Citation2014, Citation2015, Citation2021; Hicken et al. Citation2011; Edmunds et al. Citation2015; Sørhus et al. Citation2017, Citation2021; Morris et al. Citation2018; Laurel et al. Citation2019).
In the decades since the Exxon Valdez disaster, Pacific herring have been a focal species for studies on oil toxicity (Hose et al. Citation1996; Carls et al. Citation1999; Incardona et al. Citation2009, Citation2012a, Citation2012b, Citation2015). Herring are a commercially, ecologically, and culturally important forage fish species throughout the Pacific coastal waters of North America. They spawn primarily on subtidal aquatic vegetation in nearshore habitats that are especially vulnerable to short-term and lingering impacts of shoreline oiling. Across years, herring stocks form spawning aggregations at specific times and locations. Embryonic development occurs while eggs are adhered to vegetation, and hatched planktonic larvae tend to remain in nearshore waters close to their spawning grounds for 2–3 months thereafter. These early life history characteristics increase the potential for oil exposure across a large portion of a given cohort, lasting over the course of several years in spawning habitats where shoreline oiling persists. Both embryonic and larval herring are susceptible to injury from oil exposure, including photoenhanced toxicity (Barron et al. Citation2003). Embryonic herring are especially sensitive; oil exposure during early development causes a characteristic suite of acute and latent injuries, including PAH-driven developmental cardiotoxicity (Incardona et al. Citation2009; Incardona et al. Citation2021) that leads to persistent latent effects on cardiorespiratory performance (Incardona et al. Citation2015).
Puget Sound herring stocks
In the southern Salish Sea, Puget Sound supports a diverse estuarine and marine ecosystem. Additionally, approximately 4 million people live and work in the Puget Sound basin, a number that continues to increase (U.S. Environmental Protection Agency Citation2021). The oil refining and transport industries in the region rely upon significant infrastructure that includes tankers, port terminals, refineries, pipelines, and oil-by-rail. The expansion of crude oil exports is expected to increase future tanker traffic in the Salish Sea by several-fold (Greene and Aschoff Citation2023).
Puget Sound Pacific herring stocks have been closely monitored for decades, reflecting their importance as a historic commercial fishery, tribal resource and linchpin for food webs that support seabirds, pinnipeds, Chinook salmon, and Southern Resident Killer Whales – the latter two species currently listed as threatened and endangered under the U.S. Endangered Species Act, respectively (Sandell et al. Citation2019). The Washington Department of Fish and Wildlife (WDFW) manages 21 herring stocks in Puget Sound, each delineated by the spawning areas they utilize (). The WDFW describes the conservation status of each stock based upon estimated spawning biomass relative to the long-term (i.e., prior 25 years) mean biomass. Four stocks are considered healthy or moderately healthy, five are depressed/declining, nine are at critically low abundances, and the conservation status of three are unknown (Sandell et al. Citation2019). The stocks are managed by WDFW as independent populations. The degree of connectivity between them is not well understood (Siple and Francis Citation2016). It has been proposed that up to 20% migration could occur between some stocks (Stick et al. Citation2014), but this has not been consistently observed in later biomass estimates from field surveys (Sandell et al. Citation2019) or predicted from other modeling assessments (Landis and Bryant Citation2010; Siple and Francis Citation2016). This suggests that the variation in stock abundance over time may be driven by local embryo and larval survival rates. Consistent biomass to egg production relationships observed across stocks (Stick et al. Citation2014; Sandell et al. Citation2019) reinforces the importance of local survival rates to stock productivity. There is some evidence from otolith stable isotope microchemistry that some stocks remain resident near spawning grounds, while others move to feeding areas where they may mix with other stocks (Gao et al. Citation2001; Burger et al. Citation2017). Given that the four stocks currently considered healthy or moderately healthy are in close proximity to depressed and declining stocks that vary independently (Siple and Francis Citation2016; Sandell et al. Citation2019), it is unlikely that density-dependent migration is a strong driver for most stocks. Their ecological significance and well documented vulnerability to oil toxicity make Pacific herring well-suited for investigating potential stock-level responses to future spills.
Figure 1. Discrete Pacific herring spawning locations in Puget Sound. Circled areas represent site-specific fidelity for distinct stocks (Stick et al. Citation2014). Dotted circles indicate the four central Sound stocks that were the focus of this study. Map courtesy of Blake Feist adapted from Shelton et al. Citation2017.
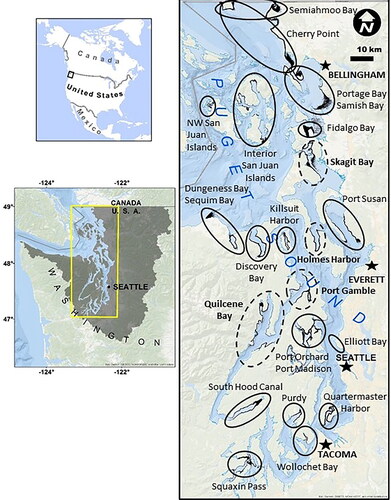
Pacific herring life history includes reproductive maturity starting at age two, annual spawning, and a life span of up to 9–11 years depending on the region. In Puget Sound, life spans do not exceed 6–9 years (Landis and Bryant Citation2010; Stick et al. Citation2014). While it is unclear if individual herring have interannual fidelity to specific spawning sites, schools of spawning herring return to consistent spawning locations year after year. Eggs are deposited on submerged vegetation or hard substrates in nearshore areas. Hatching occurs at approximately two weeks post-fertilization, and currents carry larvae to rearing areas. After metamorphosis and the onset of free feeding, young-of-the-year (YOY) initially rear in sheltered bays and inlets before moving to open water during their first fall. Juveniles form schooling aggregations for 1–2 years until they join the reproductively mature adult schools (Norcross et al. Citation2001; Stick et al. Citation2014). Rates at which herring survive and recruit to the spawning stage are highly variable across years (Stick et al. Citation2014; Siple et al. Citation2017), in part due to highly fluctuating YOY survival in response to environmental conditions (Cooney et al. Citation2001; Siple and Francis Citation2016). Influential determinants of YOY survival include (1) predation on spawned embryos by birds, fish and marine invertebrates (Bishop and Green Citation2001; Norcross and Brown Citation2001); (2) marine currents and the productivity of larval rearing areas (Cooney et al. Citation2001; Norcross and Brown Citation2001; Stick et al. Citation2014); (3) the abundance of planktonic prey during the critical first feeding period (summer to fall) (Cooney et al. Citation2001; Norcross and Brown Citation2001; Stick et al. Citation2014; Siple and Francis Citation2016); and (4) the length of the overwinter starvation period prior to onset of spring plankton blooms (Cooney et al. Citation2001; Norcross and Brown Citation2001; Stick et al. Citation2014; Siple and Francis Citation2016). For example, predation rates on spawn can be highly variable across years, with estimates ranging from 27% to over 95% (Bishop and Green Citation2001; Norcross and Brown Citation2001).
Here we adapted age-structured population models for a subset of Puget Sound herring stocks, using abundance data derived from acoustical surveys of spawning adults. Our analyses included healthy and declining stocks (, ). We assessed how oil spills exposing the spawning areas of one or two stocks from between one to nine spawning seasons might reduce stock productivity resulting from losses from the young-of-the-year (YOY) age class as a consequence of acute and latent mortality resulting from oil exposure. Modeled assessment endpoints included changes to the intrinsic population growth rate (lambda, λ), changes in future abundance (growing populations), and accelerated time to extinction (declining populations). Estimates of demographic uncertainty for all model parameters incorporated both the natural variability of stock dynamics (incorporating individual variability, variation in herring distributions in space and time, patchy food resources, and varying forms and rates of predation in marine ecosystems) and sampling error in the acoustical survey data. Our goal was to explore the usefulness of population models and field-collected abundance data, as means to assess injury to keystone forage fish species in the aftermath of future spill events.
Figure 2. Estimated Puget Sound herring spawning biomass for the four stocks from Quilcene Bay, Port Gamble, Holmes Harbor, and Skagit Bay from 1976 to 2016. Estimates from spawn deposition surveys, acoustic/trawl surveys of schooling spawners, or both (Stick et al. Citation2014, Sandell et al. Citation2019).

Table 1. Stock condition, connection, and 5 year mean spawning biomass reported in 2012 and 2016 for select Puget Sound herring stocks (Stick et al. Citation2014; Sandell et al. Citation2019).
Methods
Stock selection
We developed our population model based upon four herring stocks in the Central/South Puget Sound: Quilcene Bay, Port Gamble, Holmes Harbor and Skagit Bay (). These stocks represent a range of conservation status conditions, spawning biomass estimates, potential for genetic/migratory interaction, and data availability (). While the Quilcene Bay and Holmes Harbor stocks are considered healthy and moderately healthy, respectively, the Port Gamble and Skagit Bay stocks are both depressed. The Quilcene Bay/Port Gamble and Holmes Harbor/Skagit Bay stocks are in close proximity to one another, and there may be genetic interactions via reciprocal migration (Stick et al. Citation2014). Demographic data for age-specific survival and reproduction can only be derived from acoustic field surveys (Stick et al. Citation2014). Therefore, demographic parameters for each stock were calculated from acoustical data collected in years when the trends in the stock data matched the current designated conservation status of the stock (i.e., healthy, moderately healthy, or depressed, ; years for which acoustical data was used are listed in ).
Table 2. Transition values (and standard deviation, std) for each stock’s life history matrix. Additionally, the timespan and number of years (n) of acoustic data used to calculate the transition values are listed (Stick et al. Citation2014). Reproduction includes fecundity, survival between egg-stage and initial recruitment at age two. Age 1 survival is a placeholder to allow annual time steps. Age 2 survival includes survival and recruitment to reproductive maturity at age 3. All other survival values are survival to the next year class.
Model development
An age-structured, stock-specific (based upon acoustical data) population matrix model was developed for each of the four stocks. The model structure was adapted from a previously published Puget Sound herring model (Landis and Bryant Citation2010). Age-specific demographic rates for survival and reproductive contribution were incorporated into matrix models (described below) and assessed using RAMAS Metapop version 5.0 (Applied Biomathematics, Setauket, NY), and MATLAB 2015b (The Mathworks, Natick MA). The projection matrix methods followed Caswell (Citation2001) for deriving the transition matrix. The transitions from each age to the next were incorporated into a 9 × 9 square matrix (A) by assigning each age a number (1 through 9 years) and each transition to age i from age j becomes the element aij of matrix A (i = row, j = column) to represent the proportion of the individuals in each year class in the next age. Reproduction in the model occurred at the end of each model time step (year) following all other survival events. Since the abundance data are based upon observation of schools of reproductive adults, reproduction transition values also include recruitment, surviving to become reproductively mature. The models only tracked females, and used a sex ratio of 1:1 (Stick et al. Citation2014). Some female herring become reproductively mature at age 2. Starting at age 3, all adults are assumed to spawn annually (Stick et al. Citation2014). The reproductive element (a1j) is the number of offspring that hatch and recruit to age 2 per individual in the contributing age, j. The reproductive values for each year class were calculated from an age/fecundity relationship (fecundity per individual of age X =((5419*age X)-2125); Chapman et al. Citation1941) as well as the stock-specific estimate for survival between egg-stage and initial recruitment at age two. Age one fish cannot be directly enumerated in the field with the standard adult surveys conducted by the WDFW, but the estimate for survival to age two encompasses the survival rate of this year class. The age 1 survival estimates were not artificially separated to fit into discrete matrix elements because the associated variability could not be appropriately parsed based on available data. Therefore, transition values for age 1 (S1) were set at 1.0 with a standard deviation of 0.0 to allow annual time steps in the model runs (Landis and Bryant Citation2010). The associative properties of multiplication functioning in the matrix calculations allow this process to result in the same abundance calculations.
Average annual survival rates (with associated standard deviations) for herring ages two and older were calculated from the acoustic data estimating the spawner abundance for each stock available from WDFW (Stick et al. Citation2014). Although the transition matrices allowed stocks to have fish up to age nine, their incidence is very low, reflecting the contracted life history of Puget Sound stocks, which are slowly recovering (Landis and Bryant Citation2010; Stick et al. Citation2014). The available acoustic data are restricted to schools of spawner-stage fish that aggregate near shoreline habitats (Stick et al. Citation2014), and therefore exclude reproductively immature fish. Also, acoustic data across several stocks consistently showed more age three fish in a given year than might be predicted from the proportion of age two fish the previous year. This is likely a consequence of some herring reaching reproductive maturity a year later (age three versus two), a migratory influx of age three fish to a particular stock, or a combination of both. Irrespective, the transition value from age 2 to 3 (S2, representing survival, recruitment to reproductive maturity and net migration), as calculated from observed data, accounts for this even if it yielded a value greater than 1.0 (Landis and Bryant Citation2010).
Density dependence was integrated as a ceiling factor related to the shoreline habitat historically available for spawning for each of the four focal stocks. The production of YOY resulting from fully utilizing all available spawning habitat defined the carrying capacity (). Spawning habitat is not currently limiting, as Puget Sound stocks have been estimated to use only 9–29% of the available nearshore habitat within their respective ranges (Shelton et al. Citation2014, Citation2017). The proportional extent of habitat use for each stock was determined from field survey data, as the basis for estimating the maximum production of age one females if the stock spawned throughout the entire historical distribution of suitable shoreline areas (Shelton et al. Citation2014). Carrying capacity would only come into effect if field surveys documented large increases in these stocks, with full use of available spawning habitats. Neither is the case for the Puget Sound stocks considered here. Therefore, density independent dynamics drive the model with a cap on age 1 abundance at the carrying capacity ().
Table 3. Population model ceiling carrying capacity for age one individuals of each stock and initial abundances for all model runs. Initial abundance value was the sum of all ages according to the stable age distribution.
Although acoustic data were unavailable for the Quilcene Bay stock, the survival rates of this population are considered to be similar to Port Gamble, with the exception of very low reproduction and embryo survival for the Port Gamble stock resulting from historic contamination (Stick et al. Citation2014). Therefore, acoustic data from years when the Port Gamble stock was categorized as healthy (1978–1992) were used to parameterize the age-specific survival rates for the Quilcene Bay model. To reproduce Quilcene Bay’s high growth rate, the reproductive values for Holmes Harbor (1996–2009) – the highest of the stocks with acoustic data available – were applied to herring from Quilcene Bay ().
Spawner biomass data from WDFW (2013–2016) indicate a reduction in Port Gamble, Skagit Bay, and Holmes Harbor forage fish abundance while Quilcene Bay increased by over 170% (Sandell et al. Citation2019). The overall estimated spawner biomass for the four focal stocks over a 30–year period (1976–2016) is shown in . Intrinsic population growth rates for each modeled stock were calculated and compared to the field-collected survey data dating back to 2009, to ensure model calculations and parameters were consistent with observed abundance trends over the past decade.
A prospective analysis of each stock’s transition matrix (A) explored the intrinsic population growth rate as a function of vital demographic rates (Caswell Citation2001; Caswell et al. Citation2004). The population growth rate, or lambda (λ), equals the dominant eigenvalue of matrix A. The stable age distribution, or the proportional distribution of individuals among ages for a population at equilibrium, was provided by the right normalized eigenvector (w) corresponding to the dominant eigenvalue, λ. The influence of each matrix element, aij, on λ was assessed by calculating the sensitivity and elasticity values for matrix A (Caswell Citation2001; Caswell et al. Citation2004). Sensitivity quantifies the magnitude of change in λ resulting from a per unit change in the matrix vital rate value (aij). The sensitivity of matrix element aij equals the rate of change in λ with respect to aij, defined by δλ/δaij. Higher sensitivity values indicate greater influence on λ. The elasticity of matrix element aij is defined as the proportional change in λ relative to the proportional change in aij, and equals (aij/λ) times the sensitivity of aij. Elasticity also identified the vital rates that, when altered, had the most substantial and proportional impact on λ. Additionally, model outputs relative to changes in ceiling carrying capacity (K) for each stock were also evaluated using a sensitivity analysis.
Endpoints
Model endpoints included population growth rates, percent change in population growth rate as a consequence of different oil spill scenarios, percent baseline abundance (increasing or healthy populations), and time to extinction (decreasing or depressed populations). Demographic variability was included in each model parameter as the standard deviation of the mean demographic values calculated from acoustic survey data () and used to calculate uncertainty estimates for each model endpoint. Population growth rates were derived directly from each stock transition matrix as the dominant eigenvalue of matrix A (Caswell et al. Citation2004), and recalculated 10000 times to incorporate variability by selecting each survival and reproductive parameter value independently from the distribution of its mean and standard deviation. The model abundance runs, multiplying the transition matrix A by the age-specific abundance vector for each time step, projected annually for 100 years. To capture the variability in stock abundance estimates, projections were repeated 1000 times with each of the survival and reproductive parameter values selected independently from the distribution of its mean and standard deviation. The variability results from the variation in the observed acoustic abundance data that was used to parameterize the survival and reproductive values. Initial conditions were set such that the stable age distribution yielded numbers of spawning females similar to those recently observed in Puget Sound waters (, Sandell et al. Citation2019).
Oil spill impact scenarios
Precisely modeling dynamic oil and PAH exposure conditions, as well as UV radiation and other co-stressors in a diversity of Puget Sound nearshore habitats for hypothetical oil spills was outside the scope of this study. We investigated scenarios with 10–90% of oiled spawning habitat, equating to 10–90% reductions in YOY survival based on observations from previous spills and lab studies that show impacts inconsistent with long-term survival even with very low and transient exposures. These scenarios represent a realistic range of exposure and individual effects conditions, as observed, for example, in field studies after the Exxon Valdez (Marty et al. Citation1997) and Cosco Busan (Cosco Busan Oil Spill Trustees Citation2012) oil spills and subsequent toxicity studies. Lab studies conducted after the Exxon Valdez spill exposed herring embryos and larvae to effluent from oiled gravel columns, simulating visibly oiled beaches, and showed that very low levels of crude oil cause severe morphological and cardiac defects (Carls et al. Citation1999; Incardona et al. Citation2009). Moreover, herring embryos transiently exposed to PAH concentrations in the parts-per-trillion range showed lasting impacts on cardiorespiratory and swimming performance that are likely to preclude survival through the first year (Incardona et al. Citation2015). Though many of these studies have sought to determine lowest effects levels and mechanisms of action, results from environmentally relevant oil exposures show up to 100% acute mortality in early life stage herring, especially in the presence of UV light (Barron et al. Citation2003). Previous work has characterized the degree of exposure within spawning habitat[s] (Cosco Busan Oil Spill Trustees Citation2012) and range of effects at different exposure levels (Incardona et al. Citation2012a), but population-level effects were not determined. We also investigated scenarios with mutliple years of impacts on herring spawn due to exposure to residual oil in shoreline and nearshore habitats that remains bioavailable or is remobilized by weather, extreme tides, or other factors, as has been observed in multiple spills (Flint et al. Citation2012; Yin et al. Citation2015; Short Citation2017; Nixon and Michel Citation2018).
Spill scenarios focused only on effects from oil exposures to fertilized embryos developing on substrates near visibly oiled shorelines, independent of how the oil reached the shoreline. Scenarios explored the ability of the model to detect population-level impacts resulting from oil-induced toxicity in YOY fish of different mangitudes and durations. As noted above, we assumed that a proportion of exposed herring would not survive the near-term and delayed consequences of oil exposure and toxicity would manifest only within their first year. Therefore, YOY survival (herein S1) was the single demographic parameter altered in the model runs, reflecting combined losses from acute larval mortality and latent effects in the first year. Potential injuries to later life stages, including spawning adults or their reproductive capacity, were not considered for this study, though they could be included in an assessment of spill impacts when appropriate and as additional data become available. The associative properties of multiplication functioning in the matrix calculations would result in the same outcomes if the proportional toxicity were applied to all the reproductive values, but S1 was chosen to simplify the scenarios, e.g., setting one value rather than nine for each run. Changes in population growth rate resulting from single year oil exposures in spawning and rearing habitats were directly calculated from each stock matrix for YOY oil-induced reductions in survival ranging from 10–90%. (Methods are described in the ‘Endpoints’ section.) Reduced growth rates in response to habitat oiling were then compared to the baseline growth rate. To assess changes in stock abundance resulting from spill impact scenarios (), 100-year model projections were run with the simulated spills beginning at year five. (Abundance projection methods are described in the ‘Endpoints’ section.) Projections of abundance resulting from single year spill impacts were conducted for 50% and 90% reductions in survival. We also considered oil toxicity spanning multiple spawning seasons as a consequence of repeated exposures to remnant oil stranded or buried on spawning beaches beyond the year of a spill. To explore multi-year spill impacts, we evaluated scenarios where successive cohorts of YOY herring experienced declining degrees of reduced survival over 2, 5 or 9 years ().
Table 4. Oil spill impact scenarios to assess changes in herring abundance resulting from a localized spill in the spawning area for each Puget Sound herring stock. All scenarios start exposure at model year 5 and extend for a defined duration. Example calculation for 90% mortality: YOY survival = S1*(1–0.9).
Metapopulation scenarios
To assess the potential influence of migration between stocks, we constructed a simple metapopulation model that linked the Quilcene Bay and Port Gamble stocks with a reciprocal emigration rate of 5% annually (i.e., 5% of reproductively mature herring from Quilcene Bay emigrate to Port Gamble and vice versa). To examine this effect, we used the most severe impact scenario, whereby oil exposure was projected to cause a 90% loss of age one herring in the year of the spill, thereafter incrementally decreasing to a 10% annual loss over the course of nine years. These loss rates were applied to the Quilcene Bay and Port Gamble stocks independently and then in tandem to evaluate how the movement of individuals between the two stocks might influence the population-level response(s) to a future disaster.
Results
Sensitivity and elasticity analysis
Recruitment to reproductive maturity, or juvenile survival rates through the first and second year (S1 and S2), had the greatest influence on the population growth rate for each of the modeled central Puget Sound herring stocks, as calculated by matrix sensitivity and elasticity analyses. The relative importance of early survival (egg to age 2) on the intrinsic population growth rate (λ) was expected based upon the compressed population age structure of the stocks (Landis and Bryant Citation2010). Accordingly, environmental and demographic factors directing individual recruitment to the adult spawner stage (age 2 or 3) have an outsized influence on population growth rate and associated variability. This dominance was evident when comparing the summed elasticity values for recruitment (S1 and S2) and total adult survival (S3-S9), (, Quilcene Bay (S1 + S2) = 0.55 vs. (S3-S9) = 0.13). Correspondingly, the reproductive contributions of age 2 (R2) and age 3 (R3) spawners had larger proportional influences on λ than those of older fish ().
Table 5. Sensitivity and elasticity values calculated from the baseline matrix for each stock.
We explored the influence of selecting spawning area as the stock-limiting resource and found that changes in spawning area carrying capacity did not disproportionately influence abundance. Estimates of spawning area defined the ceiling density-dependence, and the sensitivity of healthy, moderately healthy, and vulnerable (declining) herring populations was assessed by calculating the mean abundance at 100 years for the former and mean time extinction for the latter. In each case, the carrying capacity (K) was changed to −50%, −25%, +50%, and +100% relative to the estimated baseline (0%). Modifying the carrying capacity for the healthy Quilcene Bay and moderately healthy Holmes Harbor stocks yielded nearly 1:1 changes in abundance for K values ranging from −50% (52% resulting abundance) to −25% (73% and 77%), +50% (147% and 154%) and +100% (199% and 205%). The median estimated times to extinction for the declining Port Gamble and Skagit Bay stocks were not influenced by density dependence given that population abundances remained well below carrying capacity. Therefore, herring stocks of different sizes were not disproportionally sensitive to modest (one-fold) changes in carrying capacity, and any discrepancies in the estimation of K or future changes due to spawning habitat degradation or restoration will not skew or invalidate our results.
Baseline and oil spill scenario outputs
In the absence of an oil spill, the baseline conditions predict eventual growth to an equilibrium abundance for Quilcene Bay and Holmes Harbor stocks and, conversely, a decline in the Skagit Bay and Port Gamble stocks to the point of eventual extinction (). The potential sizes of the of the Quilcene Bay and Holmes Harbor stocks are constrained by the amount of spawning habitat available, with acoustic survey-derived demographic data revealing highly variable estimated growth rates with one standard deviation corresponding to 23–33% of λ. The mean (standard deviation) equilibrium abundances across all ages for Quilcene Bay and Holmes Harbor stocks were 100.8 M (56.5 M) and 14.6 M (7.1 M) individual herring, respectively. With intrinsic growth rates below 1.0, the Skagit Bay and Port Gamble stocks both declined to extinction at an average (standard deviation) of 66 (13) and 74 (16) years, respectively.
Figure 3. Modeled Puget Sound herring stock abundances for baseline (open circles and gray upward error bars) and nine-year exposure scenario (filled circles and black downward error bars). Shaded area in panel B indicates a post-spill downward abundance deflection exceeding one standard deviation from the unexposed baseline. The mean annual values (solid lines) and associated standard deviations are derived from 100-year model runs repeated 1000 times.

Against this backdrop of widely varying yearly juvenile survival rates and consequent population growth rates, we estimated that the minimum single year loss of YOY herring due to oil toxicity in the range of 60–80% would be required to reduce the intrinsic population growth rate by one standard deviation from baseline depending upon the stock (). Although modest declines in early survival also reduced productivity, no spill impact scenario with an effect lasting for only one year reduced spawner abundances enough to be distinguishable from baseline with the natural variability and sampling error inherent in these stocks as observed in annual field surveys. Since this variability in abundance estimates can mask productivity losses, we examined a range of potential oil spill impacts to identify when population level-effects may be observable in the field.
Table 6. Mean population growth rates (λ), standard deviations (std) calculated directly from each stock matrix. The percent change in growth rate resulting from toxicity to YOY herring (percent mortality on first year survival, S1). Values in italics exceed the percent of one standard deviation of λ.
Local oil spills with decreasing impacts on survival over multiple years resulted in lower spawner abundance or shorter times to extinction, but only exceeded baseline model variability for the longest scenario (). The spill impact scenario with YOY mortality declining from 90% down to 10% over 9 years produced the largest change in abundance examined (). This was the only scenario that produced an average abundance that dipped more than one standard deviation below the modeled baseline at any time point. The mean time to extinction for Skagit Bay and Port Gamble stocks decreased by 11 years with this exposure scenario, still within one standard deviation of the baseline (). Effects on spawner abundance result from direct oil-induced reductions in YOY survival decreasing recruitment over several years. The largest declines in abundance resulted from multiyear scenarios that produced recruitment losses across all spawner age classes. Once oil exposure and related impacts on YOY survival ended, the healthy and moderately healthy stocks began increasing (). The relative abundances highlighted the loss of productivity over time whether or not field sampling may be able to detect these impacts in population demographics.
Figure 4. Mean relative abundances in Puget Sound herring stocks following crude oil toxicity in spawning habitats that persist for 1, 2, 5, and 9 years. The modeled spill occurred at year 5. Standard deviations (not shown) averaged 63%, 55%, 267%, and 230% for Quilcene Bay, Holmes Harbor, Port Gamble and Skagit Bay, respectively.
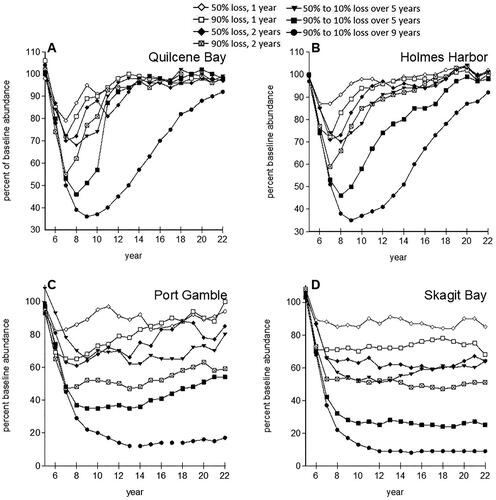
Table 7. Time to extinction for Skagit Bay and Port Gamble stocks for each exposure scenario.
Metapopulation scenario outputs
Detection of oil impacts was further complicated with the addition of dispersal of spawners between stocks which either mitigated or exacerbated impacts depending upon the spill location and the relative size of the stocks. The influence of dispersal between the large Quilcene Bay and small Port Gamble stocks showed that even a 5% exchange of individuals between these stocks prevented the Port Gamble stock from declining to extinction (). A localized spill affecting only Port Gamble produced minimal response in either stock, while a spill at Quilcene Bay or affecting both spawning areas resulted in short term declines in both stocks (). Quilcene Bay’s high spawner abundance provided large numbers of migrants to the Port Gamble Stock and mitigated the spill impacts. This demonstrates that migration from unaffected stocks after a spill event could simultaneously assist in recovery and mask the extent of injury.
Figure 5. Mean Puget Sound herring abundance (solid line) and baseline standard deviation for Quilcene Bay (A) and Port Gamble (B) stocks examining the combinations of 5% dispersal between the stocks for baseline and longest exposure scenario affecting either or both stocks. Standard deviations for each scenario were similar, so only baseline are shown for clarity.

Discussion
Stock resiliency depends on conservation status and growth trajectory
Our Pacific herring models demonstrated that the strongest determinants of resiliency to an oil spill in nearshore spawning and rearing habitats for Puget Sound stocks are the conservation status and the growth or decline trajectory of the stock at the time of the spill. This intuitive result is exemplified by the accelerated extinction risk for declining populations (Skagit Bay or Port Gamble), in contrast to a modest and temporary reduction in modeled spawner abundance for comparatively healthy stocks (Quilcene Bay or Holmes Harbor). Nevertheless, more than two thirds of Puget Sound stocks are currently in decline (Sandell et al. Citation2019), and thus particularly vulnerable to a future regional disaster.
Variable natural processes strongly influence stock response and its detection
Modeled oil spill injury to wild herring stocks sharing the contracted life history characteristic of Puget Sound stocks (lifespan of 6 rather than 9–11 years as is typical of Pacific herring) was often masked by natural variability and sampling variability in the underlying spawner abundance numbers, as estimated from acoustic surveys. Pacific herring populations typically rely on older females (ages 7–11) to produce the majority of the offspring (Hixon et al. Citation2014). In Puget Sound stocks, young spawners occupy this role due to the population’s contracted age structure and this has increased the influence of recruitment on λ (Landis and Bryant Citation2010). With recruitment driven by local and highly variable natural processes, stock abundances vary considerably, limiting the ability to detect impacts, like an oil spill. If the population age structure expands toward its historic range, the influence on productivity of recruitment and early reproduction and the corresponding natural variability would decrease. Reduced variability in stock abundance would make future impacts easier to identify and measure. The natural processes that most influenced abundance dynamics included dispersal (or migration), highly variable predation pressures, and the availability of food resources. Independently, each can result in increased or decreased survival, combining for good years, bad years, or neutral years. In good years with relatively high baseline productivity, improved survival of unexposed embryos will likely offset localized losses in response to a small oil spill. Conversely, overlaying the impact of a spill at a time of unfavorable marine habitat conditions or high background predation pressures may exacerbate spill impacts in space and time. Therefore, the ecological footprint of small and localized oil spills will be more difficult to resolve in years of relatively high zooplankton abundance and low predation on nearshore herring spawn.
Modeling herring stock abundance in scenarios where stranded oil persisted in spawner habitats for several years revealed the importance of exposure duration. Forage fish monitoring data are inherently noisy and resulted in wide standard deviations around baseline abundance estimates. The standard deviation is a representation of natural variability and observation error in the monitoring data used to parameterize the survival and reproductive rates carried through the model and serve as a backdrop for detecting a population-level injury signal attributable to a spill. These signals were only evident in the model results for the longest exposure scenarios, incorporating combined acute embryolarval and delayed young-of-the-year mortality 60% to 80% depending upon the stock (). Thus, while future Puget Sound oil spills are likely to produce significant localized losses of individual forage fish, several years of post-spill monitoring efforts will likely be needed to accurately determine the long-term, population-level consequences for dynamic stocks and the marine food webs they support.
Methods for identifying and quantifying oil spill impacts
Models can be useful for identifying priority data gaps; our approach here was constrained by conventional fisheries methods for tracking forage fish trends in Puget Sound. Although acoustic surveys yield high quality adult distribution and abundance data, they can be expensive and therefore infrequent. Current, lower cost methods project future adult recruits from stock-specific spawner counts and rake surveys of egg biomass. This creates a 2–3 year gap between direct measures of spawn in the nearshore and the subsequent direct measure of herring aggregations in offshore marine waters. While retrospective studies have been carried out to evaluate changes in fish stocks after oil spills (e.g., Deepwater Horizon; Fodrie and Heck Citation2011), they relied on extensive field surveys and concluded that processes unrelated to oil toxicity become more influential over the course of months and years. Parameterizing models based upon infrequent, lower cost field sampling methods could increase model uncertainty and, if used in isolation, limit the ability to distinguish between spill-related effects and natural drivers of the long-term stock response.
As noted above, there are important limitations when retrospectively using post-spill stock assessment data for the purposes of modeling spill-induced natural resource injury, as the two management goals are very different. Alternatively, the modeling framework developed here could be applied to forecast forage fish losses during and immediately after a spill based on direct site-specific measures of PAHs and related compounds in spawning habitats and biological matrices, in combination with laboratory-derived thresholds for near-term (i.e., embryo-larval) and delayed (post-larval) mortality. The adverse outcome pathway for developmental oil toxicity in fish is now extensively documented (Incardona and Scholz Citation2016, Citation2017, Citation2018), at biological scales ranging from the dysregulation of specific ion channels in excitable myocytes to post-larval abnormalities in the structure of the heart and cardiac output, all of which underpin swimming performance and survival in the aerobically-demanding life history of schooling herring. Therefore, the detection of bioavailable PAHs in nearshore spawning habitats, at concentrations sufficient to trigger propagating adverse outcomes, is a likely harbinger of acute and latent reduced survival.
Shoreline Cleanup and Assessment Technique (SCAT) surveys are used to document stranded oil on beaches and other shoreline substrates to inform emergency response clean-up actions (e.g., Michel et al. Citation2013). While the presence of visible oil can serve as proxy evidence of exposure, it is important to note that early developmental toxicity in fish occurs in response to very low concentrations of PAHs. Therefore, acute and delayed injuries (Laurel et al. Citation2019; Gardner et al. Citation2019) are likely in spill zones where SCAT surveys do not report visible oil (Incardona et al. Citation2015). More sensitive oil detection methods, such as chemical analyses of environmental media and passive sampling, will provide valuable information for exposure and injury assessments and reduce the risk of underestimating impacts to natural resources. Similarly, while damage assessment may rely on spawning and demographic data collected for fisheries management, when available, targeted collection of biological data may reduce uncertainty when modeling lost productivity or population-level impacts.
The modeling framework developed here could guide sampling methods to better assess the occurrence of impacts in the field. Given that oil-derived PAHs are known to be highly toxic to herring early life stages (Barron et al. Citation2004; Incardona et al. Citation2004, Citation2009, Citation2015, Citation2021; Hicken et al. Citation2011), the proportional loss of age 1 recruits can be predicted from PAH exposure conditions (particularly levels of tricycylic phenanthrenes) in oiled spawning habitats. Direct measures of recruitment loss could be incorporated into future abundance surveys, to reduce the uncertainty intrinsic to hindcasting based on abundance data for spawning adults (age 2–3 herring) collected several years post-spill. Looking to the future, conventional forage fish monitoring programs can be expected to benefit from a new generation of biomarkers for oil exposure and biological response, the latter phenotypically anchored to adverse outcome pathways for acute and delayed mortality, or other productivity endpoints, such as reduced growth and fecundity. Further research on productivity endpoints across different age groups could inform model development to explore these effects. Over the past decade, efforts to develop new indicators have focused on ontogenetic pathways involving cardiotoxicity (e.g., Edmunds et al. Citation2015; Xu et al. Citation2016, Citation2017), and more recently the dysregulated development of the jaw, eyes, and other structures (Sørhus et al. Citation2017). The future incorporation of these new tools into field surveys can yield information about individual forage fish condition (in relation to a prior sublethal crude oil exposure), beyond merely presence or absence, at the critical life history stages between hatching and recruitment to adult spawning populations.
Migration between stocks could buffer declines
Our results also point to the importance of migration between stocks as a potential buffer against local extinctions. Specifically, our model outputs suggest that declining stocks could be supplemented by low but consistent in-migration (straying) from nearby, healthy stocks. These linkages in space and time should be evident as synchrony in the larger abundance trends across Puget Sound. However, Puget Sound herring runs are managed independently, reflecting an assumption of minimal straying (Bargmann Citation1998; Stick et al. Citation2014; Sandell et al. Citation2019). There is no indication of panmixing (Gao et al. Citation2001; Small et al. Citation2005), but rather evidence that local-scale forces (e.g., local predation and prey availability) drive observed variability across stocks (Siple and Francis Citation2016). Consistent with this, it does not appear the robust Quilcene Bay population is supplementing nearby stocks at risk (Sandell et al. Citation2019). Absent new data indicating a greater degree of interconnectivity among adult spawners, future impact assessments in Puget Sound are likely to be stock-specific, depending on the geographic location, timing, and size of the spill.
Implications of model assumptions
Our model focused on stock injury resulting from acute or latent mortality in YOY herring. Effects of oil exposures on Pacific herring predators and/or prey were not included. Potential oil impacts on predators could lead to decreased herring mortality. Conversely, impacts on prey could reduce food availability and growth potential, leading to increased herring mortality. Other additive or synergistic impacts from an oil spill, such as habitat degradation, fishery closures, or other factors were not considered, though these can also affect fish populations after an oil spill (Fodrie and Heck Citation2011). Additionally, our assumption that demographic rates vary independently may underestimate stock injury if, in fact, all ages of herring experience similar and coordinated influences each year. All survival and reproductive parameters were varied independently because data suggested the Puget Sound stocks’ schooling and migratory behaviors are inconsistent with all the age classes schooling together year around (Gao et al. Citation2001). The influence of density dependence on Puget Sound herring stocks is presently unknown (Stick et al. Citation2014; Sandell et al. Citation2019). Here we have assumed density-independent survival when YOY juveniles are below a stock’s carrying capacity. The relative impacts of a localized oil spill might be lessened by density-dependent compensatory mechanisms, wherein limited prey and other resources are freed up for surviving individuals (Langangen et al. Citation2023). However, given the absence of density relationships for these forage fish stocks, and the corresponding lack of data for parallel oil impacts to zooplankton and other components of Puget Sound food webs (which would offset any compensatory response), we utilized density-independent processes in our models. Inaccurate application of density dependence could underestimate a stock’s response to an oil spill. New information on stock independence (e.g., genetics, migratory pathways) and driving environmental factors (e.g., sea surface temperature) could refine the model and reduce uncertainty about model assumptions and structure.
Conclusions
The health and productivity of wild Pacific herring populations have been a focus for large-scale natural resource injury assessments in the US for decades, from the collapse of the herring fishery in Prince William Sound in the years following the 1989 Exxon Valdez disaster (Hose et al. Citation1996; McGurk and Brown Citation1996; Carls et al. Citation1999; Marty et al. Citation1997; Peterson et al. Citation2003; Barron et al. Citation2004) to the 2007 Cosco Busan spill in San Francisco Bay (Incardona et al. Citation2012a, Citation2012b). Estimating toxicological injury is inherently a process of biological scaling, from molecular initiating events in the cells of individual fish to the dynamics of wild forage fish populations that feed seabirds, marine mammals, and threatened fish predators such as Chinook salmon. As we have shown here, population modeling can provide a useful framework for organizing existing stock assessment and ecotoxicological information for herring, but the approach is currently limited in its ability to determine population-level effects from a spill by the high degree of natural variability and observation error in forage fish aggregations, independent of oil spills. The strength of our model predictions could be improved with better demographic data (particularly between the larval and spawner recruitment stages) and, eventually, specific biomarkers for oil exposure and consequent/subsequent poor health in herring that survive to hatch in oiled spawning habitats. These gaps notwithstanding, population modeling in support of NRDA will be most promising for predicting population-level impacts when relatively large spills converge on the most vulnerable (declining) wild stocks. In other oil spill scenarios, the inability to determine a population-level loss through modeling for field surveys does not necessarily indicate a lack of injury in the resource. For smaller spills and more robust herring populations, we recommend an assessment of lost fish or productivity based on direct contaminant monitoring as it corresponds to specific toxicity thresholds from the expanding adverse outcome pathway for crude oil injury to fish early life stages. The latter approach would also allow consideration of parallel habitat stressors (e.g., temperature, UV radiation, other contaminants) that are known to exacerbate crude oil impacts but were not included here.
Authors’ contributions
Julann A. Spromberg: conceptualization, methodology, software, writing – original draft. Sarah E. Allan: conceptualization, writing – original draft, funding acquisition. Nathaniel L. Scholz: conceptualization, writing – review and editing.
Acknowledgments
The authors thank T. Sandell for acoustic data, W. Landis for early modelling discussions, and T. Baker for manuscript review.
Disclosure statement
The authors declare that they have no known competing financial interests or personal relationships that could have appeared to influence the work reported in this paper.
Additional information
Funding
References
- Bargmann G. 1998. Forage fish management plan: a plan for managing the forage fish resources and fisheries of Washington. Olympia (WA): Washington Department of Fish and Wildlife; p. 77.
- Barron MG. 2017. Photoenhanced toxicity of petroleum to aquatic invertebrates and fish. Arch Environ Contam Toxicol. 73(1):40–46. doi: 10.1007/s00244-016-0360-y.
- Barron MG, Carls MG, Heintz R, Rice SD. 2004. Evaluation of fish early life-stage toxicity models of chronic embryonic exposure to complex polycyclic aromatic hydrocarbon mixtures. Toxicol Sci. 78(1):60–67. doi: 10.1093/toxsci/kfh051.
- Barron MG, Carls MG, Short JW, Rice SD. 2003. Photoenhanced toxicity of aqueous phase and chemically dispersed weathered Alaska North Slope crude oil to Pacific herring eggs and larvae. Enviro Toxic Chem. 22(3):650–660. doi: 10.1002/etc.5620220326.
- Barron MG, Ka’aihue L. 2001. Potential for photoenhanced toxicity of spilled oil in Prince William Sound and Gulf of Alaska waters. Mar Pollut Bull. 43(1–6):86–92. doi: 10.1016/s0025-326x(01)00037-6.
- Bishop MA, Green SP. 2001. Predation on Pacific herring (Clupea pallasi) spawn by birds in Prince William Sound, Alaska. Fish Oceanogr. 10(s1):149–158. doi: 10.1046/j.1054-6006.2001.00038.x.
- Burger M, Sandell T, Fanshier C, Lindquist A, Biondo P, Lowry D. 2017. 2016-2017 Puget Sound Acoustic Midwater Trawl Survey. Olympia, WA: Washington Department of Fish and Wildlife Report No. FPA-29-09; p. 41.
- Burlington LB. 2002. An update on implementation of Natural Resource Damage Assessment and Restoration under OPA. Spill Sci Tech Bull. 7(1–2):23–29. doi: 10.1016/S1353-2561(02)00064-6.
- Carls MG, Rice SD, Hose JE. 1999. Sensitivity of fish embryos to weathered crude oil: part I. Low-level exposure during incubation causes malformations, genetic damage, and mortality in larval pacific herring (Clupea pallasi). Environ Tox Chem. 18(3):481–493.
- Carroll J, Vikebø F, Howell D, Broch OJ, Nepstad R, Augustine S, Skeie GM, Bast R, Juselius J. 2018. Assessing impacts of simulated oil spills on the Northeast Arctic cod fishery. Mar Pollut Bull. 126:63–73. doi: 10.1016/j.marpolbul.2017.10.069.
- Caswell H. 2001. Matrix population models: construction, analysis, and interpretation. California, USA: Sinauer Associates.
- Caswell H, Takada T, Hunter CM. 2004. Sensitivity analysis of equilibrium in density-dependent matrix population models. Ecol Lett. 7(5):380–387. doi: 10.1111/j.1461-0248.2004.00595.x.
- Chapman W, Katz M, Erickson D. 1941. The races of herring in the state of Washington. Olympia (WA): State of Washington Department of Fisheries.
- Cooney RT, Allen JR, Bishop MA, Eslinger DL, Kline T, Norcross BL, McRoy CP, Milton J, Olsen J, Patrick V, et al. 2001. Ecosystem controls of juvenile pink salmon (Onchorynchus gorbuscha) and Pacific herring (Clupea pallasi) populations in Prince William Sound, Alaska. Fish Oceanogr. 10(s1):1–13. doi: 10.1046/j.1054-6006.2001.00039.x.
- Cosco Busan Oil Spill Trustees. 2012. Cosco Busan Oil Spill Final Damage Assessment and Restoration Plan/Environmental Assessment. Prepared by California Department of Fish and Game, California State Lands Commission, National Oceanic and Atmospheric Administration, United States Fish and Wildlife Service, National Park Service, Bureau of Land Management.
- Edmunds RC, Gill JA, Baldwin DH, Linbo TL, French BL, Brown TL, Esbaugh AJ, Mager EM, Stieglitz J, Hoenig R, et al. 2015. Corresponding morphological and molecular indicators of crude oil toxicity to the developing hearts of mahi mahi. Sci Rep. 5(1):17326. doi: 10.1038/srep17326.
- Flint PL, Schamber JL, Trust KA, Miles AK, Henderson JD, Wilson BW. 2012. Chronic hydrocarbon exposure of harlequin ducks in areas affected by the Selendang Ayu oil spill at Unalaska Island, Alaska. Environ Toxicol Chem. 31(12):2828–2831. doi: 10.1002/etc.1997.
- Fodrie FJ, Able KW, Galvez F, Heck KL, Jr., Jensen OP, López-Duarte PC, Martin CW, Turner RE, Whitehead A. 2014. Integrating organismal and population responses of estuarine fishes in Macondo spill research. BioScience. 64(9):778–788. doi: 10.1093/biosci/biu123.
- Fodrie FJ, Heck KL. Jr. 2011. Response of coastal fishes to the Gulf of Mexico oil disaster. PLoS One. 6(7):e21609. doi: 10.1371/journal.pone.0021609.
- Gao YW, Joner SH, Bargmann GG. 2001. Stable isotopic composition of otoliths in identification of spawning stocks of Pacific herring (Clupea pallasi) in Puget Sound. Can J Fish Aquat Sci. 58(11):2113–2120. doi: 10.1139/f01-146.
- Gardner LD, Peck KA, Goetz GW, Linbo TL, Cameron JR, Scholz NL, Block BA, Incardona JP. 2019. Cardiac remodeling in response to embryonic crude oil exposure involves unconventional NKX family members and innate immunity genes. J Exper Bio. 222(21):jeb205567.
- Greene GH, Aschoff J. 2023. Oil spill assessment maps of the central Salish Sea – Marine seafloor & coastal habitats of concern – a tool for oil spill mitigation within the San Juan Archipelago, Washington State, USA. Cont Shelf Res. 253:104880. doi: 10.1016/j.csr.2022.104880.
- Heintz RA, Rice SD, Wertheimer AC, Bradshaw RF, Thrower FP, Joyce JE, Short JW. 2000. Delayed effects on growth and marine survival of pink salmon Oncorhynchus gorbuscha after exposure to crude oil during embryonic development. Mar Ecol Prog Ser. 208:205–216. doi: 10.3354/meps208205.
- Hicken C, Linbo T, Baldwin D, Willis MJ, Myers M, Holland L, Larsen M, Stekoll M, Rice S, Collier T, et al. 2011. Sublethal exposure to crude oil during embryonic development alters cardiac morphology and reduces aerobic capacity in adult fish. Proc Natl Acad Sci USA. 108(17):7086–7090. doi: 10.1073/pnas.1019031108.
- Hixon MA, Johnson DW, Sogard SM. 2014. BOFFFFs: on the importance of conserving old-growth age structure in fishery populations. ICES J Marine Sci. 71(8):2171–2185. doi: 10.1093/icesjms/fst200.
- Hose JE, McGurk MD, Marty GD, Hinton DE, Brown ED, Baker TT. 1996. Sublethal effects of the Exxon Valdez Oil Spill on herring embryos and larvae: morphological, cytogenetic, and histopathological assessments, 1989-1991. Can J Fish Aquat Sci. 53:2355–2365.
- Incardona JP. 2017. Molecular mechanisms of crude oil developmental toxicity in fish. Arch Environ Contam Toxicol. 73(1):19–32. doi: 10.1007/s00244-017-0381-1.
- Incardona JP, Carls MG, Day HL, Sloan CA, Bolton JL, Collier TK, Scholz NL. 2009. Cardiac arrhythmia is the primary response of embryonic Pacific Herring (Clupea pallasi) exposed to crude oil during weathering. Environ Sci Technol. 43(1):201–207. doi: 10.1021/es802270t.
- Incardona JP, Carls MG, Holland L, Linbo TL, Baldwin DH, Myers MS, Peck KA, Tagal M, Rice SD, Scholz NL. 2015. Very low embryonic crude oil exposures cause lasting cardiac defects in salmon and herring. Sci Rep. 5(1):13499. doi: 10.1038/srep13499.
- Incardona JP, Collier TK, Scholz NL. 2004. Defects in cardiac function precede morphological abnormalities in fish embryos exposed to polycyclic aromatic hydrocarbons. Toxicol Appl Pharmacol. 196(2):191–205. doi: 10.1016/j.taap.2003.11.026.
- Incardona JP, Gardner LD, Linbo TL, Brown TL, Esbaugh AJ, Mager EM, Stieglitz JD, French BL, Labenia JS, Laetz CA, et al. 2014. Deepwater horizon crude oil impacts the developing hearts of large predatory pelagic fish. Proc Nat Acad Sci. 111:e 1510–E1518.
- Incardona JP, Linbo TL, French BL, Cameron J, Peck KA, Laetz CA, Hicks MB, Hutchinson G, Allan SE, Boyd DT, et al. 2021. Low-level embryonic crude oil exposure disrupts ventricular ballooning and subsequent trabeculation in Pacific herring. Aquat Toxicol. 235:105810. doi: 10.1016/j.aquatox.2021.105810.
- Incardona JP, Scholz NL. 2016. The influence of heart developmental anatomy on cardiotoxicity-based adverse outcome pathways in fish. Aquat Toxicol. 177:515–525. doi: 10.1016/j.aquatox.2016.06.016.
- Incardona JP, Scholz NL. 2017. Environmental pollution and the fish heart. Fish Phys. 36B:373–433.
- Incardona JP, Scholz NL. 2018. Case study: the 2010 Gulf oil spill and its environmental developmental impacts. In: W. Burggren and B. Dubansky, editors. Development and Environment. Cham Switzerland\: Springer. p. 235–283. https://doi.org/10.1007/978-3-319-75935-7_10
- Incardona JP, Vines CA, Anulacion BF, Baldwin DH, Day HL, French BL, Labenia JS, Linbo TL, Myers MS, Olson OP, et al. 2012a. Unexpectedly high mortality in Pacific herring embryos exposed to the 2007 Cosco Busan oil spill in San Francisco Bay. Proc Nat Acad Sci. 109(2):E51–E58.
- Incardona JP, Vines CA, Linbo TL, Myers MS, Sloan CA, Anulacion BF, Boyd DT, Collier TK, Morgan S, Cherr GN, et al. 2012b. Potent phototoxicity of marine bunker oil to translucent herring embryos after prolonged weathering. PLoS One. 7(2):e30116. doi: 10.1371/journal.pone.0030116.
- Landis WG, Bryant PT. 2010. Using weight of evidence characterization and modeling to investigate the cause of the changes in Pacific herring (Clupea pallasi) population dynamics in Puget Sound and at Cherry Point, Washington. Risk Anal. 30(2):183–202. doi: 10.1111/j.1539-6924.2009.01288.x.
- Langangen Ø, Ohlberger J, Stige LC, Patin R, Buttay L, Stenseth NC, Ono K, Durant JM. 2023. Effects of early life mass mortality events on fish populations. Fish Fisheries. 24(1):176–186. doi: 10.1111/faf.12718.
- Langangen Ø, Olsen E, Stige LC, Ohlberger J, Yaragina NA, Vikebø FB, Bogstad B, Stenseth NC, Hjermann DØ. 2017. The effects of oil spills on marine fish: implications of spatial variation in natural mortality. Mar Pollut Bull. 119(1):102–109. doi: 10.1016/j.marpolbul.2017.03.037.
- Laurel BJ, Copeman LA, Iseri P, Spencer ML, Hutchinson G, Nordtug T, Donald CE, Meier S, Allan SE, Boyd DT, et al. 2019. Embryonic crude oil exposure impairs growth and lipid allocation in a keystone Arctic forage fish. iScience. 19:1101–1113. doi: 10.1016/j.isci.2019.08.051.
- Marty GD, Hose JE, McGurk MD, Brown ED, Hinton DE. 1997. Histopathology and cytogenetic evaluation of Pacific herring larvae exposed to petroleum hydrocarbons in the laboratory or in Prince William Sound, Alaska, after the Exxon Valdez oil spill. Can J Fish Aquat Sci. 54(8):1846–1857. doi: 10.1139/f97-091.
- McGurk MD, Brown ED. 1996. Egg–larval mortality of Pacific herring in Prince William Sound, Alaska, after the Exxon Valdez oil spill. Can J Fishand Aquat Sci. 53(10):2343–2354.
- Michel J, Owens EH, Zengel S, Graham A, Nixon Z, Allard T, Holton W, Reimer PD, Lamarche A, White M, et al. 2013. Extent and degree of shoreline oiling: deepwater horizon oil spill, Gulf of Mexico, USA. PLoS One. 8(6):e65087. doi: 10.1371/journal.pone.0065087.
- Morris JM, Gielazyn M, Krasnec MO, Takeshita R, Forth HP, Labenia JS, Linbo TL, French BL, Gill JA, Baldwin DH, et al. 2018. Crude oil cardiotoxicity to red drum embryos is independent of oil dispersion energy. Chemosphere. 213:205–214. doi: 10.1016/j.chemosphere.2018.09.015.
- Nixon Z, Michel J. 2018. A review of distribution and quantity of lingering subsurface oil from the Exxon Valdez oil spill. Deep-Sea Res Part II. 147:20–26. doi: 10.1016/j.dsr2.2017.07.009.
- Norcross BL, Brown ED. 2001. Estimation of first-year survival of Pacific Herring from a review of recent stage-specific studies. In: F. Funk, J. Blackburn, and D. Hay, editors. Herring: expectations for a new millenium. Fairbanks, AK: University of Alaska Sea Grant, AK-SG-01-04; p. 800.
- Norcross BL, Brown ED, Foy RJ, Frandsen M, Gay SM, Kline TC, Mason DM, Patrick EV, Paul AJ, Stokesbury KDE. 2001. A synthesis of the life history and ecology of juvenile Pacific herring in Prince William Sound, Alaska. Fisheries Oceanogr. 10(s1):42–57. doi: 10.1046/j.1054-6006.2001.00040.x.
- Norcross BL, Hose JE, Frandsen M, Brown ED. 1996. Distribution, abundance, morphological condition, and cytogenetic abnormalities of larval herring in Prince William Sound, Alaska, following the Exxon Valdez oil spill. Can J Fish Aquat Sci. 53(10):2376–2387. doi: 10.1139/f96-212.
- Ohlberger J, Langangen Ø. 2015. Population resilience to catastrophic mortality events during early life stages. Ecol Appl. 25(5):1348–1356. doi: 10.1890/14-1534.1.
- Peterson CH, Rice SD, Short JW, Esler D, Bodkin JL, Ballachey BE, Irons DB. 2003. Long-term ecosystem response to the Exxon Valdez Oil Spill. Science. 302(5653):2082–2086. doi: 10.1126/science.1084282.
- Raimondo S, McKenney Jr CL Jr, Barron MG. 2006. Application of perterbation simulations in population risk assessment for different life history strategies and elasticity patterns. Human Ecol Risk Assmt. 12(5):983–999. doi: 10.1080/10807030600826904.
- Sandell T, Lindquist A, Dionne P, Lowry D. 2019. 2016 Washington State Herring Status Report. Olympia, WA, Washington Department of Fish and Wildlife Report No FPT 19-07; p. 87.
- Scholz NL, Incardona JP. 2015. Scaling PAH toxicity to fish early life stages. Environ Toxicol Chem. 34(3):459–461. doi: 10.1002/etc.2830.
- Shelton AO, Francis TB, Feist B, Williams GD, Lindquist A, Levin PS. 2017. Forty years of seagrass population stability and resilience in an urbanizing estuary. Ecology. 105(2):458–470. doi: 10.1111/1365-2745.12682.
- Shelton AO, Francis TB, Williams GD, Feist B, Stick K, Levin PS. 2014. Habitat limitation and spatial variation in Pacific herring egg survival. Mar Ecol Prog Ser. 514:231–245. doi: 10.3354/meps10941.
- Short J. 2003. Long-term effects of crude oil on developing fish: lessons from the Exxon Valdez oil spill. Energy Sources. 25(6):509–517. doi: 10.1080/00908310390195589.
- Short J. 2017. Advances in understanding the fate and effects of oil from accidental spills in the United States beginning with the Exxon Valdez. Arch Environ Contam Toxicol. 73(1):5–11. doi: 10.1007/s00244-016-0359-4.
- Siple MC, Francis TB. 2016. Population diversity in Pacific herring of the Puget Sound, USA. Oecologia. 180(1):111–125. doi: 10.1007/s00442-015-3439-7.
- Siple MC, Shelton AO, Francis TB, Lowry D, Lindquist AP, Essington TE. 2017. Contributions of adult mortality to declines of Puget Sound Pacific herring. ICES J of Mar Sci. 75(1):319–329. doi: 10.1093/icesjms/fsx094
- Small MP, Loxterman JL, Frye AE, Von Bargen JF, Bowman C, Young SF. 2005. Temporal and spatial genetic structure among some Pacific Herring populations in Puget Sound and the southern Strait of Georgia. Trans Am Fish Soc. 134(5):1329–1341. doi: 10.1577/T05-050.1.
- Sørhus E, Donald CE, da Silva D, Thorsen A, Karlsen Ø, Meier S. 2021. Untangling mechanisms of crude oil toxicity: linking gene expression, morphology and PAHs at two developmental stages in a cold-water fish. Sci Total Environ. 757:143896. doi: 10.1016/j.scitotenv.2020.143896.
- Sørhus E, Incardona JP, Furmanek T, Goetz GW, Scholz NL, Meier S, Edvardsen RB, Jentoft S. 2017. Novel adverse outcome pathways revealed by chemical genetics in a developing marine fish. Elife. 6:e20707. doi: 10.7554/eLife.20707.
- Stick KC, Lindquist A, Lowry D. 2014. 2012 Washington State Herring Stock Status Report. Olympia, WA, Washington Department of Fish and Wildlife Report No. FPA-14-09; p. 107.
- Thorne RE, Thomas GL. 2008. Herring and the “Exxon Valdez” oil spill: an investigation into historical data conflicts. ICES J Mar Sci. 65(1):44–50. doi: 10.1093/icesjms/fsm176.
- U.S. Environmental Protection Agency. 2021. EPA Geographic Funding at Work on Puget Sound Recovery. EPA-910-K-21-001. p. 48.
- Ward EJ, Adkison M, Couture J, Dressel SC, Litzow MA, Moffitt S, Hoem Neher T, Trochta J, Brenner R. 2017. Evaluating signals of oil spill impacts, climate, and species interactions in Pacific herring and Pacific salmon populations in Prince William Sound and Copper River, Alaska. PLoS One. 12(3):e0172898. doi: 10.1371/journal.pone.0172898.
- White JW, Barceló C, Hastings A, Botsford LW. 2022. Pulse disturbances in age structured populations: life history predicts initial impact and recovery time. J Anim Ecol. 91(12):2370–2383. doi: 10.1111/1365-2656.13828.
- Xu EG, Khursigara AJ, Magnuson J, Hazard ES, Hardiman G, Esbaugh AJ, Roberts AP, Schlenk D. 2017. Larval red drum (Sciaenops ocellatus) sublethal exposure to weathered Deepwater Horizon crude oil: developmental and transcriptomic consequences. Environ Sci Technol. 51(17):10162–10172. doi: 10.1021/acs.est.7b02037.
- Xu EG, Mager EM, Grosell M, Pasparakis C, Schlenker LS, Stieglitz JD, Benetti D, Hazard ES, Courtney SM, Diamante G, et al. 2016. Time- and oil-dependent transcriptomic and physiological responses to deepwater horizon oil in mahi-mahi (Coryphaena hippurus) embryos and larvae. Environ Sci Technol. 50(14):7842–7851. doi: 10.1021/acs.est.6b02205.
- Yin F, John GF, Hayworth JS, Clement TP. 2015. Long-term monitoring data to describe the fate of polycyclic aromatic hydrocarbons in Deepwater Horizon oil submerged off Alabama’s beaches. Sci Total Environ. 508:46–56. doi: 10.1016/j.scitotenv.2014.10.105.