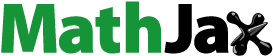
ABSTRACT
Purpose
Reading disability (RD) is frequently associated with deficits in auditory processing (i.e., processing speech and non-linguistic sounds). Several hypotheses exist regarding the link between RD and auditory processing, but none fully account for the range/variety of auditory impairments reported in the literature. These impairments have been primarily summarized by qualitative reviews and meta-analytic evidence for most auditory processing impairments is lacking.
Method
We conducted a PRISMA-compliant meta-analysis quantifying the degree to which individuals with RD are impaired on four categories of auditory processing abilities: frequency discrimination, intensity discrimination, duration discrimination, and gap detection. This methodology was accepted and executed as a Registered Report.
Results
Auditory processing impairments of medium to large effect size were present in RD vs. typical groups for all categories: frequency (g = 0.79), duration (g = 0.80), and intensity discrimination (g = 0.60), as well as gap detection (g = 0.80). No differences were found across task designs (i.e., testing methods).
Conclusion
This meta-analysis documents a large, multiple-domain non-linguistic, auditory processing impairment in RD. Contrary to previous studies, we found a significant deficit in intensity discrimination. The impairments described here must be accounted for by future causal hypotheses in RD and suggest that auditory processing impairments are broader than previously thought.
Introduction
Difficulty in learning to read can have many possible causes, and any given individual may struggle with one or many of the skills necessary to read accurately, fluently, and with comprehension. This multifactorial view of reading ability posits that multiple underlying skills, including phonological awareness (PA), letter knowledge, and rapid automatized naming (RAN) ability, predict reading outcomes, and that weaknesses in one skill may be able to be compensated with facility in another (Compton, Citation2020; O’Brien & Yeatman, Citation2020). These skills are mostly linguistic; however, children with reading disabilityFootnote1 (RD) also struggle with skills that are not purely linguistic, such as auditory processing (Hämäläinen et al., Citation2013; Rosen, Citation2003). Auditory processing skills are often tested with sound stimuli that are difficult to discriminate or detect; for example, in a frequency discrimination task, an individual might be asked to indicate whether two similar sounds were the same or different frequencies (i.e., pitch). Other types of auditory processing skills include stream segregation (Helenius et al., Citation1999), beat detection (Goswami et al., Citation2002), speech-in-noise (Nittrouer et al., Citation2018), and rapid temporal order judgment tasks (Tallal, Citation1980). Any given individual with RD may perform worse (or better) than a typical peer on any constellation of auditory processing tasks, as different peripheral and central mechanisms are responsible for encoding each sound feature.
There is considerable overlap between children who struggle with auditory processing tasks (who may be diagnosed with auditory processing disorder, APD)Footnote2 and children who have impairment in reading or language (Sharma et al., Citation2009). However, many children with RD have typical auditory processing abilities (Heath & Hogben, Citation2004; Ramus et al., Citation2003; Tallal, Citation1980), further reinforcing the notion that RD is a heterogeneous diagnostic category and leaving unanswered the question of how auditory processing and RD may be related.
Some previous studies suggested that auditory processing is directly causal to RD. Tallal (Citation1980) hypothesized that children with RD cannot “consistently process” rapidly changing sound features, such as formant transitions. This rapid auditory temporal processing deficit model for reading difficulties presented by Tallal (Citation1980, Citation1998) spurred a number of studies looking at short vs. long inter-stimulus intervals (ISIs), rapid formant transitions, and fast vs. slow amplitude or frequency modulation rates in individuals with RD (Marshall et al., Citation2001; Ramus et al., Citation2003; Wright & Conlon, Citation2009). Key to this hypothesis is that these small acoustic changes could result in cascading difficulties with perceiving the difference between phonemes and thus, the meaning of words. For example, vowel duration differences create a phoneme distinction in some languages (e.g., Finnish), so if a child has trouble consistently discriminating the duration of sounds, then they may have trouble with phonemic awareness for sounds or words that differ in duration. Similar patterns might be observed for speakers of Mandarin, for which frequency (tone/pitch) is phonemic, and so on.
The results of these studies about processing rapidly changing sounds were mixed, with some finding marked accuracy differences between RD and control children at fast intervals (Reed, Citation1989), but many others found impairment among poorer readers regardless of whether the stimuli were rapidly changing or short in duration (Amitay, Ben‐Yehudah, et al., Citation2002; Marshall et al., Citation2001; Mody et al., Citation1997; Ramus et al., Citation2003). Further, the rapid auditory temporal processing hypothesis fails to explain a few key findings in the literature. First, the deficit in auditory processing in RD is not specific to rapidly changing stimuli, nor is it present in all rapid auditory tasks (Marshall et al., Citation2001; Protopapas, Citation2014; Rosen, Citation2003). For example, Marshall et al. (Citation2001) observed no interaction between typical and RD group performance on the auditory task used by Tallal (Citation1980) and ISI, indicating that RD children’s deficits were not specific to rapid stimuli. Second, this hypothesis is challenged by weak clinical intervention results from a popular computerized program for training rapid auditory processing, Fast ForWord. The training showed no meta-analytic effect on remediating reading difficulties in randomized controlled trials (Strong et al., Citation2011). Finally, several early results that reported large effect sizes supporting the hypothesis have not been replicated. Specifically, according to this hypothesis, the severity of language or reading impairment and the severity of auditory impairment should be highly correlated. Tallal (Citation1980) reported an extremely high Spearman correlation of rs = .81 between auditory processing skill and score on a phonics test. Later studies didn’t find such strong relationships (Witton et al., Citation1998), and within groups with a disorder, the relationship was essentially non-existent (Rosen, Citation2003).
Alternative hypotheses to try to explain the relationship between auditory processing deficits and reading deficits have been proposed. The imprecise temporal sampling (Goswami, Citation2011) and neural noise (Hancock et al., Citation2017) hypotheses discuss the role of neural oscillations and neuronal timing in affecting both sensory/perceptual and language systems. Other hypotheses implicate decision-making and executive functions as part of a multifactorial model of RD, both of which may impact psychophysical task performance (O’Brien & Yeatman, Citation2020). These non-causal hypotheses generally share the idea that shared neural architecture between reading and auditory processing skills explains why both may be impaired in RD.
Although the mechanism of auditory processing deficit hypotheses for RD is not clear, evidence points to auditory processing deficits in RD, on average (i.e., when comparing groups with versus without RD). Many qualitative reviews exist regarding auditory processing deficits in individuals with RD, but even the best qualitative reviews, such as the one completed by Hämäläinen et al. (Citation2013), fall short of identifying deficits in RD for various categories of auditory processing (e.g., duration perception, intensity perception). In order to draw conclusions about whether a meaningful effect was present, they relied on the number of significant results in a category. For example, intensity discrimination had mostly non-significant results (only 2 of 16 studies were significant at p = .05), and their conclusion was that intensity discrimination is not impaired in RD. The study reported that a mean weighted effect size (weighted by sample size) for that analysis as d = .5, which is a medium effect. Many of the original results were near p = .05, and the lower bounds of confidence intervals were often extremely close to 0. These facts are not factored into the interpretation, in favor of focus on the proportion of significant p-values. Because that review did not perform a true meta-analysis, the true effect and its confidence interval remain unknown. As a result, researchers could draw incorrect conclusions from this review, and a formal meta-analysis may reveal that this effect size is significant. Clinicians and school professionals are also left without clear guidelines on the relationship between auditory processing and RD which could inform decisions such as when to refer children with APD or RD for comprehensive evaluation.
The only extant meta-analysis related to this topic focused only on frequency discrimination impairment in RD, and potential moderators of this relationship such as reading impairment severity and psychophysical task design (Witton et al., Citation2020). This meta-analysis describes a large frequency discrimination impairment (d = 0.76; p < .001) in RD. Moderator analyses revealed that psychophysical task design and performance on phoneme deletion, a phonological awareness task, moderate the relationship between RD and frequency discrimination. This study is an important first step toward understanding the complex mechanisms shared by reading and pitch perception, but its narrow scope does not contextualize the findings alongside other auditory tasks. The present study advances knowledge by expanding scope to a variety of auditory processing measures and implementing needed improvements to the methodology, which will be described below.
The primary goal of the present study was to estimate the mean auditory processing impairments in RD as compared to typical reader groups, across multiple auditory domains. We accomplished this by updating and extending the studies by Hämäläinen et al. (Citation2013) and Witton et al. (Citation2020). The importance of following gold-standard meta-analysis techniques cannot be understated; as a result, we made five key methodological and statistical improvements that are required in order to produce high-quality evidence. First, we used snowball searching to identify related articles using references and citations, which neither study did. Snowball searching is essential in order to identify all relevant effect sizes in the literature that should be included in the analysis (Greenhalgh & Peacock, Citation2005). As an example, in a recent snowball search for a meta-analysis in our lab related to reading (McWeeny et al., Citation2022), we initially screened 4,000 titles identified during database searching and found 91 relevant articles to include in the analysis; we found additional 39 relevant articles from the snowball search. Second, we used robust variance estimation (RVE) models to estimate effect sizes rather than sample-size-based effect size weighting (Hedges et al., Citation2010). RVE models allow for multiple effect sizes from the same sample to be included in each analysis, so we will not have to take the mean of effect sizes within a given study, as was done in Witton et al. (Citation2020). We also assessed the degree to which each analysis is powered, which is essential for meta-analytic moderator analyses (Hedges & Pigott, Citation2004; Schmidt, Citation2017). Fourth, we described and analyzed measures of study quality and risk of bias, which allows for both descriptive data about the health of the literature and allows inference testing on whether study quality affects effect sizes. For example, we can test whether lower quality studies tend to find larger effect sizes. Each of these design considerations were made in concordance with the Preferred Reporting Items for Systematic Reviews and Meta-Analyses (PRISMA; Moher et al., Citation2009; Page et al., Citation2021) and represent the gold-standard in meta-analysis research. These considerations improve the quality of meta-analysis in our field at all steps of the process, from literature searching through analysis. Finally, as we took the same approach for frequency discrimination, duration discrimination, intensity discrimination, and gap detection, we are able to contextualize summary effect sizes across domains, which provides key advances over the existing single-domain meta-analysis and cross-domain qualitative analysis. This allowed us to address key theoretical questions; for example, many studies suggest that intensity discrimination can serve as a control for psychophysical task demands (e.g., Goswami et al., Citation2010); comparing these summary effect sizes for each domain will allow us to directly test the size of a frequency discrimination deficit as compared to an intensity discrimination deficit.
A secondary aim of this meta-analysis was to test the hypothesis that auditory processing impairments in RD are in part due to differing demands across psychophysical tasks used to measure auditory processing. This hypothesis predicts that different task designs (e.g., same-different vs. two-alternative forced choice; 2AFC) will yield different estimates for the average auditory processing impairment in RD. A meta-analysis of frequency discrimination in RD (Witton et al., Citation2020) found evidence of task design moderating effect sizes; specifically, same-different tasks yielded smaller effect sizes (d = 0.40, SD = 0.27, n = 4) than either AXB (d = 1.10, SD = 0.79, n = 11) or 2AFC tasks (d = 0.92, SD = 0.39, n = 9). We predicted that this moderation effect would apply to any auditory domain in which discrimination is measured, as tasks that have greater executive function (EF) demands are likely to result in lower scores in RD samples, due to the high co-occurrence of EF difficulties and ADHD with RD (Germanò et al., Citation2010; Lonergan et al., Citation2019). This analysis should provide additional information related to the mechanism by which RD is associated with poorer auditory processing.
Methods
Overview
We examined the relationship between reading impairment and auditory processing; as a result, we encountered designs that analyzed reading from a disorder vs. typical development perspective (categorial) and ones that measure reading ability as a continuum within typical readers or across varied reading ability (correlational). Based on the literature review from Hämäläinen et al. (Citation2013) and pilot searches, most studies treated reading categorically by comparing groups; as a result, the primary focus of the analyses is categorical. The heterogeneity present in correlational analyses, such as whether the whole sample is analyzed together, or subgroups are analyzed separately, as well as the variety within each sample’s composition (e.g., typical sample, language disorder, or APD sample), precludes meta-analyzing these correlations.
Study inclusion criteria
Studies included in the quantitative analysis had four characteristics: 1) a group of individuals with RD (see below for definition), 2) a group of control/typically developing individuals,Footnote3 3) a relevant behavioral auditory processing task (see details in Auditory Processing Tasks, below), and 4) calculable standardized mean difference. No neural data is included in this meta-analysis (for a meta-analysis of the mismatch negativity (MMN), see Gu and Bi (Citation2020). No study was excluded because its effect size was an outlier, as was done in Hämäläinen et al. (Citation2013); unless the study was excluded for poor study quality, as using these effect sizes most accurately represents the true effect size in the population. In order to assess whether extreme outliers exerted effects on our main questions of interests, models with extreme outliers (≥2 SD) both included and excluded are presented.
For RD definition, we included studies that used alternate terms such as dyslexia or poor readers, given the heterogeneity of definitions used in both research and practice that use different types of reading measures and scores for inclusion and for formal diagnosis. We did not exclude a study if the sample had comorbidities such as ADHD, or other language impairment, but we did exclude the study if the sample was designed to characterize autism spectrum disorder (ASD), schizophrenia, or chromosomal disorder (e.g., Fragile X, Down Syndrome). If the study did not list their participant exclusion criteria, they may thus include individuals with ASD incidentally; we included these studies. As mentioned above, we chose to use the broader term reading disability, as the term dyslexia is sometimes understood as a deficit only at the single word level with no other co-occurring deficits and typical IQ. Participants’ reading skills needed not be reported in the paper; however, study quality measures (see Appendix 1) served to mark studies that only used participant report or purported diagnosis for reading ability distinctions as lower quality. We also required that children had begun formal reading instruction and thus would be able to be diagnosed with RD, which diverges from the Hämäläinen et al. (Citation2013) study approach. Inclusion of pre-readers dilutes the analysis of the relationship between auditory processing and RD, as approximately 50% children who are identified as at-risk for reading problems based on family history of reading problems will not go on to develop RD (Puolakanaho et al., Citation2007).
Auditory processing tasks
Auditory processing tasks that were analyzed here comprise four primary domains: frequency discrimination, duration discrimination, intensity discrimination, and gap detection. We chose these domains because they represent basic acoustic features (i.e., frequency, duration, and intensity), with gap detection being an additional way to test duration processing. We also restricted our tasks only to thresholds in the relevant domains, as was done in Witton et al. (Citation2020). We also further restricted our stimuli to only tones/tone pips or noise.
Auditory processing task descriptions and analytic considerations
Definitions used to guide inclusion by domain are described below.
Frequency
Any task that assesses an individual’s ability to tell the difference between two frequencies and for which a threshold (in Hz) was considered a frequency discrimination task. We included all frequency discrimination thresholds in the main analysis, regardless of the frequency measured. This decision is motivated by comparison to previous literature (Witton et al., Citation2020), despite pitch being encoded by primarily different mechanisms (e.g., cochlear place, timing) above and below ~4 kHz. Thresholds must be reported in Hz; some authors refer to auditory repetition tasks with varying inter-stimulus intervals (ISIs; e.g., Tallal, Citation1980) as frequency discrimination, but thresholds from these tasks are reported in ms.
Intensity
Any task that assesses an individual’s ability to tell the difference between two intensities and for which a threshold (in dB) is derived was considered an intensity discrimination task.
Duration
Any task that assesses an individual’s ability to tell the difference between two durations and for which a threshold (in ms) is derived was considered a duration discrimination task.
Gap detection
Any task in which a participant is asked whether they hear a period of silence (i.e., a gap) in a period of noise or a tone and a threshold (in ms) is derived from it, was considered a gap detection task.
Task designs
There are several possible methods that can be used to estimate behavioral discrimination thresholds. Most use adaptive thresholds to estimate a percent detectable change. We categorized each task according to the guidelines used by Witton et al. (Citation2020). Two-alternative forced choice (2AFC) tasks require participants to choose between two stimuli on the domain of interest (e.g., which tone was higher/lower, longer/shorter, or louder/quieter). Same-different tasks also use two stimuli, but only require the participant to respond whether the stimuli were the same or different (similar to the yes-no method). Three-alternative forced choice (3AFC) tasks require participants to select the “odd one out” from a group of three sounds. AXB tasks also use three sounds, but the middle sound is a fixed reference, and the listener chooses between the first and last sound on the domain of interest. ABABA/AAAAA tasks use 10 stimuli over two intervals and participants are asked which interval had two different sounds. A similar paradigm might play four sounds in two groups and participants will be asked which interval (1 or 2) had different tones; authors differ in their description of this task, which we called two interval, two-alternative forced choice (2I-2AFC). Gap detection tasks have an additional design, in which the participant is asked if they heard two sounds or one. These “fusion” tasks only exist for gap detection thresholds. Finally, oddball paradigms with fixed “levels” are occasionally used to estimate a threshold. These tasks were included as long as they had a sufficient description of their methodology used to estimate the threshold.
Procedure
Data collection
To begin, we conducted a snowball search (i.e., a backward and forward search and subsequent searches from results) using references and citations of the 61 included studies from the Hämäläinen et al. (Citation2013) study in September 2020. To conduct this snowball search, we used Microsoft Academic Graph (Wang et al., Citation2019), which is a database that tracks connections between publications (peer-reviewed papers and other scientific products such as dissertations and theses), such that every backward reference is also a forward citation. Microsoft Academic has higher citation counts than Scopus or Web of Science, with a high number of unique items (Harzing & Alakangas, Citation2017). This allowed us to efficiently identify studies that have been published since the search for their review was completed in 2010. Newly identified articles were also snowball searched in February 2022.
Abstract and title screening
Titles of papers from the search were reviewed individually by the first author, who has expertise in the relevant constructs and meta-analysis methods; titles that were deemed to be clearly irrelevant were screened out. Potentially relevant abstracts were then each reviewed by two different screeners using a checklist of inclusion criteria; consensus was reached in all cases of conflict. Articles with relevant abstracts were then full text screened by two independent coders. Agreement for full-text inclusion was 86.9%; consensus was reached in all cases of conflict. A PRISMA flow diagram describing the search and screening procedure is presented in .
Data extraction
For each of the studies meeting the inclusion criteria listed above, two independent coders extracted information from each paper in four domains: 1) sample characteristics, 2) reading measures or groups, 3) auditory processing tasks, and 4) study quality. Sample characteristics included age, demographics (i.e., SES, race, language, etc.), and how the RD category was defined (e.g., were they all individuals with a formal diagnosis, or were they tested and scored below a cutoff?). We included studies of all languages and demographics. The specific reading measure(s) used were extracted and categorized as single word or connected text reading that was rate-based, accuracy-based, both rate and accuracy-based, or a task of reading comprehension, as these constructs provide good coverage of the types of reading measures used in the literature across countries/orthographies. Information relating to the auditory processing tasks included what auditory domain was being tested (frequency, duration, and intensity discrimination, as well as gap detection) and the stimuli characteristics. Every article was double-coded, with consensus being reached in cases of conflict; across all extracted fields, agreement was 90.2%.
Study quality was assessed for each study using the NIH Quality Assessment Tool for Observational Cohort and Cross-Sectional Studies.Footnote4 Although many meta-analyses use a sum score of different quality metrics, these types of scores are not recommended for meta-regression (Shamliyan et al., Citation2010; Whiting et al., Citation2005). Instead, each study was categorized as good, fair, or poor according to the NIH tool independently by two coders, and consensus was met in all cases of conflict. These categories were then used as categorical variables in meta-regression.
For extracting effect sizes, we coded standardized mean difference (SMD) measures, whether they were reported as separate sample means and SDs or if they were reported as an effect size, typically Cohen’s d. For a study to be included, it needed to have either a reported SMD and corresponding variance, or group means and SDs.
Analytic plan
Main analyses included descriptive statistics (e.g., demographics, stimuli characteristics), main effects, and within- and between-study bias analyses for each of the four categories of auditory processing skills. Only select moderator analyses (i.e., subgroup analyses and meta-regression) with large anticipated effect sizes were included in the main planned analyses, as moderator analyses have much lower power than main meta-analyses because they depend on the number of studies in each category (Hedges & Pigott, Citation2004; Schmidt, Citation2017). Questions such as developmental trajectories and differences across languages have strong theoretical implications but cannot be tested with adequate power given the size of the extant literature.
All standardized mean difference (SMD) effect sizes were transformed to Hedges’ g, which corrects for small sample sizes. All statistical analyses were conducted in R, using robumeta for statistical modeling and metafor for effect size calculation and auxiliary functions (e.g., generating funnel plots) (Fisher et al., Citation2017; Hedges et al., Citation2010; R Core Team, Citation2013). Robumeta uses robust variance estimation (RVE) models, which allow for correlated effects within a study, maximizing data retention. Intercept-only models were generated for each of the auditory domain categories listed above.
To test for study quality bias, we used the NIH Quality Assessment Tool for Observational Cohort and Cross-Sectional Studies as described above. This allowed us to test whether high-quality studies had systematically smaller or larger effect sizes. We tested this across all studies rather than within each auditory category, due to low power within each category. To test for funnel plot asymmetry, which is indicative of publication or reporting bias, we used a technique that allows for multiple effect sizes per category. Traditional methods for examining funnel plot asymmetry, such as Egger’s Regression or trim-and-fill analyses, only accommodate one effect size per study or category. Recently, these traditional methods have been expanded to correlated effects models with “sandwich” estimators (Rodgers & Pustejovsky, Citation2020). We therefore used an “Egger’s Sandwich Regression” to test for funnel plot asymmetry.
Power analyses
Using the SMD effect size estimates reported by Hämäläinen et al. (Citation2013), we calculated the power at α = .05 for each of the categories they analyzed. This provided us with a strong estimate of the likely effect size, making our power analyses more accurate. With a mean n = 22 per group across all studies, assuming moderate heterogeneity in a random effects model, we calculated power for effect sizes, listed in , using the method described in Valentine et al. (Citation2010). Power for all analyses was extremely high (≥.99), given the medium and large effect sizes present and the number of studies found during pilot searching.
Table 1. A Priori Power Estimates from Known Extant Literature for Categorical Studies.
At present, there is no clear consensus on calculating power for moderator analyses for RVE models. To calculate the power for task design as a moderator, we used the metapower package in R (Griffin, Citation2020) assuming moderate heterogeneity (I2 = 50%), a minimum k = 20 per discrimination category, a mean n = 22 per group, and the effect sizes present in Witton et al. (Citation2020; d1 = .4, d2 = 1.1). A priori power for this analysis was .94; however, the 20 studies do not fit into just two categories, as there are many possible task designs (e.g., 2AFC, AXB, 3AFC, same-different, etc.). Actual power may decrease as a result of the 20 studies not being evenly distributed between two task design categories. We approached this in two ways; we only included a task design category in the moderator analyses if it had at least N = 5 samples, and we report the a priori power calculated at Stage 1 alongside the analysis in the final (Stage 2) manuscript, as has been done in other meta-analyses (e.g., Araújo et al., Citation2015).
For bias analyses, we pooled studies across all categories, as the primary question is about bias rather than auditory processing. Assuming a moderate effect of study quality (d = ±.35; that is, .35 between poor and average quality and .35 between average and high quality) and moderate heterogeneity (I2 = 50%) in a random-effects model, we were a priori powered at ≥ .99Footnote5 assuming we had k = 77 studies, as estimated from pilot searching. This was recalculated during Stage 2 acceptance using the k = 63 studies, and was still ≥ .99. For Egger’s Regression, we used a standard regression power calculation. There would need to be 70 studies included to be adequately (.90) powered to find a moderate effect (f2 = .15). We believed a priori that this was highly feasible given the snowball search technique, but at stage 2, had 63 samples and thus an estimated power of .87. If any analysis is not powered at .9 or greater, we report this in the results, below.
Results
Main registered analyses
Descriptive statistics
The analytic sample (n = 3,545; k = 135) was drawn from 63 independent samples across 65 reports that assessed at least one of the four auditory processing task categories. A majority of the participants (n = 2,206) were children under 12 years old, whereas adults (>18 years old; n = 1,045), adolescents (12 ≤ x ≤18 years; n = 253), and combined child/adolescent samples (n = 82) comprised the remainder of the sample.Footnote6 A majority of participants (n = 2,003) spoke English, although there were large samples of Hebrew (n = 558), Chinese (n = 230), German (n = 182), Greek (n = 181), and Dutch (n = 166) participants; other languages included Portuguese, Spanish, Finnish, and French (combined n = 225).
Frequency, duration, and intensity discrimination, and gap detection deficits in RD
To estimate the average impairment in each of the four auditory processing task categories measured, we ran intercept-only RVE models. There were at least 22 effect sizes analyzed per task category. A significant deficit was found in each auditory category at an alpha level of p = .01, indicating that in each case, the RD participants had significantly poorer auditory processing, and all effect sizes were medium or large (Hedges’ g of 0.60–0.80). The forest plots presenting effect sizes for each auditory processing task category are presented in . Full model results are given in . Heterogeneity was moderate to high for each category (all I2 ≥ 0.48.) with and without outliers excluded. Model results for frequency discrimination (g = 0.79), duration discrimination (g = 0.80), and intensity discrimination (g = 0.60) changed minimally with the exclusion of outliers. However, the model for gap detection with a high-end outlier excluded yielded a smaller effect size estimate (g = 0.80 as opposed to g = 1.05), less heterogeneity (I2 = 75.20 and τ2 =0 .35 as opposed to I2 = 89.13 and τ2 = 0.97), and a larger t-value despite a smaller effect size estimate (t = 4.89 as opposed to t = 3.65). Because we consider the data excluding the outlier to be a more accurate representation of the relationship between gap detection and reading ability, we will primarily use the model with outliers excluded in the interpretation of our gap detection analysis. Models with and without outliers are presented in Supplemental Table S1.
Figure 5. Forest Plot of Gap Detection Effect Sizes. Note. The 4I-2AFC used 4 stimuli and participants were told either stimulus 2 or 3 was different from the other 3.

Table 2. Magnitude of Auditory Processing Impairments in RD: Intercept-only Models.
Task design as a moderator of effect size
We next tested whether specific task designs (e.g., 2AFC, AXB) yielded larger effects than others by running RVE meta-regression models for each auditory processing task category. In order to be analyzed, a task design needed to be present in 5 or more samples. The number of task designs analyzed thus varied for the different auditory processing task categories; 3 task designs were included in the frequency discrimination analysis, 2 task designs were included in each of the duration discrimination, intensity discrimination, and gap detection analyses. No significant moderating effect of task design was found for any task. A priori power as registered at Stage 1 was .94 for each analysis; however, many of our assumptions turned out to be incorrect, and thus these power values were inflated. Specifically, one of the key conditions, same-different designs, did not reach the threshold of N = 5 studies to be included and calculating power with its corresponding effect size of g = 0.4 would inflate power greatly. To address this concern in Stage 2 analyses, we created an adjusted power calculation that used the actual N, n present in our data (n = 29 per group for frequency and duration discrimination, n = 26 per group for intensity discrimination, and n = 20 per group for gap detection), and I2 values (rounded to the nearest quartile to reflect the benchmarks present in metapower), with the effect sizes from Witton et al. (Citation2020). For example, for duration discrimination, we calculated adjusted power using the corresponding effect sizes from Witton et al. (Citation2020) for the two designs that met the N = 5 studies threshold (g2AFC = 0.9, gAXB = 1.1). As gap detection designs (i.e., fusion and the gaps-in-noise test) were not present in the Witton et al. meta-analysis, we chose a moderate difference (Δg = 0.3) between the two conditions to match the intensity discrimination power calculation (gABABA = 0.6 and g2AFC = 0.9). Full model results showing no significant differences across task design for any auditory processing task category are presented in .
Table 3. Effects of Task Design: Meta-regression Models.
Bias analyses
Does study quality bias effect sizes?
Of the 63 included studies, each was assigned a study quality rating based on the criteria specified; 4 were rated good quality, 43 were fair quality, and 16 were poor quality. The criteria that prevented most fair quality studies from being coded as good was the lack of reported reliability for both auditory processing and reading tasks, and the lack of reported power analyses. To test whether study quality systematically biased effect sizes, we collapsed across auditory domains to increase power. No significant effect of study quality was found, though good quality studies had marginally smaller effect sizes (Δg = −0.48; p = .07) than fair quality studies. Poor studies had slightly larger effect sizes than fair studies, but the effect was not significant (Δg = 0.28; p = .17). Full model results are presented in .
Table 4. Between- and Within-study Bias Analyses.
Is there publication bias?
To test whether publication bias exists in the literature, we performed a one-tailed Egger’s sandwich regression across all auditory processing task categories (Rodgers & Pustejovsky, Citation2020). This analysis revealed a significant effect of sampling bias on effect sizes, indicating that studies with larger sampling bias (i.e., standard error) had larger effect sizes, which is characteristic of publication bias. The degrees of freedom for the regressor, which is sampling variance, was notably low (df < 4) due to a few high-end outliers (i.e., large sampling variance due to small sample size). We suggest caution in the interpretation of significant results in the presence of this df < 4, as type I error is inflated in the t-distribution at low degrees of freedom. Full model results are presented in .
Discussion
This systematic review and meta-analysis examined non-linguistic auditory processing deficits in RD. Our results reveal a significant impairment for individuals with RD as compared with typical readers in auditory frequency (g = 0.79), duration (g = 0.80), and intensity discrimination (g = 0.60), as well as gap detection (g = 0.80). These results are broadly consistent with previous reviews that documented deficits in frequency discrimination (Hämäläinen et al., Citation2013; Witton et al., Citation2020), as well as duration discrimination and gap detection (Hämäläinen et al., Citation2013) in RD. However, in contrast to past reviews, we also found a significant impairment in intensity discrimination, similar in magnitude to the other domains analyzed, despite this being considered a control or neutral baseline in many studies (e.g., Goswami et al., Citation2010).
The effect sizes presented here can be reasonably compared to the weighted effect sizes from Hämäläinen et al. (Citation2013) and Witton et al. (Citation2020). Despite the increased specificity in our inclusion criteria, namely the restriction to behavioral thresholds from non-linguistic stimuli in only four auditory processing task categories, our effect sizes for each auditory processing task category were highly similar. Effect sizes were within g = ± .1 for frequency, duration, and intensity discrimination, and within g = ± .2 for gap detection. The present analysis has a distinct advantage in also reporting confidence intervals for each category, which ranged considerably in size among the auditory processing task categories ([0.44 0.76] for intensity discrimination and [0.45 1.15] for gap detection). In sum, these results suggest that individuals with RD are on average, poorer than age-matched typical-reader peers in multiple domains of non-linguistic auditory processing, reflecting even broader deficits than previous reviews have suggested.
We found considerable heterogeneity and effect size variance in the literature as demonstrated by large I2 values and a large range of effect sizes. Given the range of ages, task designs, and stimulus characteristics included in this meta-analysis, this heterogeneity is somewhat expected. To illustrate some potential sources of this heterogeneity, we present an example of how much effect sizes can vary within the same study and sample. In this example, Thomson et al. (Citation2013) tested intensity discrimination in the first year of a longitudinal study when children were age 9;8 (years;months). In that assessment, the RD children (n = 33) performed extremely poorly (g = 1.88) relative to controls (n = 11) in a 2AFC task (29.25 dB standard). Thomson and Goswami (Citation2008) used a subset of these participants’ data from one year later (age 10;8) to test intensity discrimination in an AAAAA/ABABA task. There, the RD group (n = 25) had no intensity discrimination deficit (75 dB standard) and outperformed the controls (n = 23; g = −.14). There are a number of possible reasons for this large effect size change between the two studies: differences in task design, stimulus properties, developmental changes, selection and subsetting bias, selective reporting, low reliability of intensity discrimination thresholds in children, or any combination of these.
In an attempt to explain some of this heterogeneity across studies, we tested whether specific task designs yielded larger effects across domains, as was found for frequency discrimination in Witton et al. (Citation2020). We found no differences for any of the task designs (e.g., whether task designs that required different processing, such as higher working memory demands, led to greater group differences) in any of the auditory processing task categories. As described in the results section, neither the “same-different” design nor 2I-2AFC were not included in any of our moderator analyses. The adjusted power calculations presented in , which we believe to be the most accurate representation of the analyses’ power, are considerably lower than the a priori power calculations. Accordingly, the interpretation of these findings is limited in that the null effects described are unsurprising given the adjusted power.
Risk of bias within and between studies: Study quality and publication bias
Study quality is a key consideration in assessing a literature’s risk of bias, with more standardized, transparent, and replicable methods decreasing the risk of bias within each study. Only 6% of studies qualified as “good” (n = 4), 68% as “fair” (n = 43) and 25% as “poor” (n = 16Footnote7). The primary study quality dimensions on which studies differed were the clarity and strength of the RD diagnostic inclusion criteria, whether any framework was given for handling co-occurring diagnoses such as ADHD or DLD/SLI, and the description of the relevant auditory processing tasks. Though there were varying strengths and weakness in these papers, no study reported a priori power analyses, and only three studies (Georgiou et al., Citation2010; Heath et al., Citation2006; Papadopoulos et al., Citation2012) measured or reported reliability for non-standardized behavioral tasks, reducing the overall variability of study quality. No systematic bias for low quality or high-quality articles was found, though the lack of variability likely contributed to finding no effect of study quality.
Finally, we found an effect of publication bias in the included studies, contrary to the findings of Hämäläinen et al. (Citation2013). Comparing the publication bias analyses between our reviews is difficult given the differences in inclusion criteria described above. We do offer some caution in the interpretation of our publication bias analysis, as the degrees of freedom were below 4, at which point the t-distribution inflates type I error. Going forward, studies of auditory processing and RD should report a priori power and pre-register analysis plans, to promote replicability (Ansari & Gervain, Citation2018).
Effect size comparison among auditory processing and reading-related deficits
A primary goal of this study was to use shared methodology among our four meta-analyses in order to compare these effect sizes to each other and discuss the context of each described deficit. The magnitude of the deficits among the four auditory processing task categories was strikingly similar, and quite large. The deficits measured here are also similar, though smaller in magnitude, to several meta-analyses looking at deficits for RD in skills more directly relating to reading, including phonological awareness (g = 1.37; Melby-Lervåg et al., Citation2012), RAN (g = 1.19; Araújo & Faísca, Citation2019), and orthographic knowledge (g = 1.17; Georgiou et al., Citation2021). The similarity in magnitude does not necessarily suggest that auditory processing plays similarly important roles in reading development as phonological awareness and RAN, but rather that the role of auditory processing in reading development needs further testing so that it can properly be factored into theoretical models or potentially included in universal screening.
Implications for theoretical models
Regarding theoretical models, the overall result of large, cross-domain auditory processing deficits do not necessarily falsify existing hypotheses linking RD and auditory processing, such as the temporal sampling framework (Goswami, Citation2011) and neural noise hypothesis (Hancock et al., Citation2017). However, it does necessitate either a broadening of the current models or more precisely specifying the causal paths (as suggested by Protopapas, Citation2014). Both of these models implicate poor phase-locking in auditory cortical networks as the link between perceptual deficits (e.g., in rise-time discrimination or beat detection) and reading deficits. As we do not believe that the frequency, duration, and intensity discrimination deficits derived from mostly pure-tone stimuli arise from purely subcortical processes (e.g., phase-locking at the auditory nerve), nor as a result of oscillatory dynamics, there must be an additional explanation to account for the auditory processing deficits described here. Below, we offer an approach for understanding the relationship among the four auditory processing task domains measured here.
Though deficits in frequency discrimination, duration discrimination, and gap detection have been described before, our review is the first to conclude that there is a significant weakness in intensity processing in RD. Though intensity discrimination is often included as a “control task” to ensure that other effects of interest are not due to task demands (e.g., Goswami et al., Citation2010), its magnitude was highly similar to frequency and duration discrimination as well as gap detection. The reason that many individual studies and a major systematic review (Hämäläinen et al., Citation2013) described no intensity discrimination deficits in RD is unclear. However, a potential explanation is that low power in individual studies and the comparison of their respective p-values across studies led researchers to believe that there was no intensity discrimination deficit.
Our cross-domain analyses offer two primary interpretations for the intensity discrimination deficit. One is that intensity discrimination is truly impaired in RD and should be considered alongside spectral and temporal processing deficits as a core auditory processing impairment. Another possible explanation is that there is no true effect of intensity discrimination, and that as a control task, the magnitude of its effect should be subtracted from the other auditory processing task categories to quantify their respective effects. This latter interpretation would be in line with an executive functioning deficit (e.g., Bruder & Schulte-Korne, Citation2010; Snowling et al., Citation2018), in which auditory processing deficits should all be impaired a similar amount for a given individual. This account necessitates that the magnitude of the intensity discrimination deficit correlates with the magnitude of frequency or duration discrimination deficit. Only Tong et al. (Citation2018) reported correlations between intensity thresholds and other psychoacoustic tasks, with intensity discrimination correlating with a one-rise task at r = .29 and with a rise rove task at r = .37. Few studies have even published the correlations between the tasks described in this meta-analysis with other psychoacoustic tasks more broadly (Gibson et al., Citation2006; Halliday & Bishop, Citation2006). For example, Gibson et al. (Citation2006) found low to moderate correlations (r = .29 and r = .39 for the control and RD groups, respectively) for the relationship between thresholds of frequency discrimination and frequency modulation detection. However, low reliability of auditory processing measures (e.g., Snowling et al., Citation2018) attenuate correlations, and the published relationships among auditory processing measures may be lower than true relationships among them in the following manner (Spearman, Citation1904).
Thus, although we find it unlikely that the intensity discrimination deficit is highly correlated with a frequency or duration discrimination deficit, further research is needed to understand the relationship among auditory processing measures.
In sum, these results broaden our understanding of auditory processing deficits in RD. This broadening was necessary, given the previous interpretation of intensity discrimination as a control task. However, we are not left with any clearer picture for how these deficits fit with the prevailing multifactorial models of RD (Compton, Citation2020), as evidence for a cascading causal path remains weak. Models such as the neural noise hypothesis (Hancock et al., Citation2017) remain viable under these results, but need updating to reflect the relative lack of time-specific requirements in cortex for intensity processing.
Underlying distributions of auditory processing and reading abilities
Some papers have described bimodal distributions for auditory processing ability, such that some individuals with RD have no auditory processing impairment, whereas others have a large impairment (Banai & Ahissar, Citation2004, Citation2005). If this is indeed the case, it raises the question of what utility describing a “mean impairment” for a given task serves when it is possible that no individual child falls at the mean of the auditory processing ability distribution for that task. The mean impairments described here still have utility for creating clinical benchmarks for children diagnosed with co-occurring APD and RD, such that children performing worse than the estimated effect sizes for a given auditory processing task category likely fall into the impaired group of a bimodal distribution, regardless of how many children fall at the mean.
Our data partly support the argument that individuals with RD have larger between-subject variability in auditory processing task performance, which has been noted by a number of studies (e.g., Banai & Ahissar, Citation2005; King et al., Citation2003). Using a rule of thumb for comparing variance ratios (s2max/s2min <3; Dean & Voss, Citation1999), 58 of the 135 effect sizes had unequal variance (43%), with RD having the larger variance in 55 of these 58 effect sizes (95%). However, in studies with multiple effect sizes, it was common (N = 17) for a study with multiple effect sizes to have unequal variances for one effect size (e.g., frequency discrimination), but equal variance for others (e.g., a different frequency discrimination task). Only six studies with multiple effect sizes had unilaterally unequal variances. Moreover, variance ratios with small samples are more likely to yield unequal variance between groups. In sum, though there is some evidence that individuals with RD are more variable in their performance on auditory processing tasks, the effects are not unilateral, nor are they particularly damaging to the interpretation of mean impairments.
Limitations
The primary limitation of meta-analyses is that pooling data across studies, samples, stimuli, and task designs may average out theoretically motivated and potentially meaningful variability between effect sizes. For example, Ahissar et al. (Citation2006) tested the hypothesis that individuals with RD are particularly poor performers in the presence of a perceptual anchor or reference tone, and they find starkly different effect sizes in a “no-reference” condition (g = 1.78) versus a “reference” condition (g = −0.07); yet the inclusion criteria we chose in the Stage 1 manuscript necessitate pooling these effect sizes and thus wash away potentially meaningful variability. There are many such critiques to be levied against data pooling, ranging from concern over pooling frequency perception data for stimuli above and below ~4 kHz, to including adults alongside children for skills that we know change over the course of development, and to concerns about including RD samples who may have co-occurring ADHD. We recognize the validity of these critiques and invite researchers to use materials from this study’s Open Science Framework page to test specific hypotheses from extant data if there is sufficient power to do so. If there is not, this meta-analysis should be used as justification for funding/support to generate high-quality data in future studies.
Another methodological limitation specific to our meta-analysis was our decision to not conduct our own full/new database search. Replicable database searches are highly recommended by PRISMA (Page et al., Citation2021), and our decision to forgo one needs strong justification. Primarily, our decision was motivated by the highly overlapping topic area and inclusion criteria in Hämäläinen et al. (Citation2013). We identified and screened a large number of potential articles from our snowball search, in line with the size of typical database search results, including numerous additional samples totaling hundreds of participants that were not included in the original systematic review (e.g., Farmer & Klein, Citation1993). Nonetheless, we cannot definitively rule out the possibility that a database search may have identified additional sources and support updating of our materials on our Open Science Framework page if new sources are identified.
In addition, our sample, like most studies on RD, overrepresents English-speaking and reading participants (Share, Citation2021). Though we were sensitive to the differing diagnostic cutoff criteria across orthographies, such as using non-word or fluency reading measures in transparent languages where reading accuracy plateaus after approximately first grade (Papadopoulos et al., Citation2021), we are unable to remove the English-speaking bias from our meta-analyses.
Conclusions and future directions
The meta-analytic methods described here could reasonably be applied to other psychoacoustic tasks, such as others presented in Hämäläinen et al. (Citation2013), as well as to stimuli from non-linguistic to include linguistic stimuli. Further specifying these impairments is paramount to being able to formulate detailed hypotheses surrounding why these deficits exist in RD. We strongly encourage these lines of research.
Despite the seemingly elusive mechanisms that link auditory processing and RD, an independent effort toward translation to clinical impact should continue. Namely, the size of these behavioral impairment warrants further investigation as potential screening measures for RD. Comparatively few prospective longitudinal studies (e.g., Law et al., Citation2017; Snowling et al., Citation2018) include measures of auditory processing compared to more traditional measures of letter and letter-sound knowledge, PA, and RAN, as the evidence base surrounding auditory processing in RD is comparatively weaker (for a longitudinal meta-analysis on RAN, PA, and reading, see McWeeny et al., Citation2022). We hope that this meta-analysis can be used as justification for creating standardized auditory processing measures with published reliability, which, if satisfactory, can be followed by their inclusion in prospective longitudinal studies.
Taken together, the findings of this systematic review and meta-analysis advance the field forward in multiple ways. The addition of an intensity discrimination deficit to meta-analytically documented deficits in frequency discrimination (Witton et al., Citation2020), suggests that auditory impairments in RD are broader, and perhaps larger, than previously thought, precluding the use of domain-specific causal hypotheses (Protopapas, Citation2014). Study quality measures reveal the need to make major updates to reliability reporting and a priori power calculations. Improving reliability and registering adequately powered analysis plans may help reduce the extant publication bias. Finally, we hope that the present study can be used as motivation for increased research activity in the area of auditory processing and RD, with the goal of improving our understanding of why these deficits exist, and whether we can leverage them in identifying RD earlier.
Data sharing and accessibility
We have shared all raw data, digital materials, and analysis code, as well as registered our approved protocol on the study’s Open Science Framework page (https://osf.io/nwctx).
Ethical approval
As this study is a systematic review and meta-analysis, ethical approval is not required.
Supplemental Material
Download MS Word (21.5 KB)Supplemental Material
Download MS Word (15.9 KB)Acknowledgments
We thank Rick Qian, Gabriella Leibowitz, and Tessneem Shahbandar for their assistance with data collection and extraction. We also thank the many authors who sent us their data to be included in this meta-analysis. We thank Beth Tipton for essential statistical guidance. We thank Sumit Dhar and Megan Roberts for input and comments on earlier versions of this manuscript. The authors declare that they have no conflict of interest.
Disclosure statement
No potential conflict of interest was reported by the authors.
Supplementary material
Supplemental data for this article can be accessed online at https://doi.org/10.1080/10888438.2023.2252118.
Additional information
Funding
Notes
1. Here, we use the term RD to describe a primary deficit in reading accuracy, speed, or comprehension; this is a slightly broader term than developmental dyslexia, which is typically defined by deficits primarily at the word level, though the terms are sometimes used interchangeably (Peterson & Pennington, Citation2015).
2. There is considerable debate in the field about the criteria for APD (see Chermak et al., Citation2018; Vermiglio, Citation2018). This is orthogonal to the current analyses because isolated, continuous auditory task scores are used, thus this debate will not be discussed here.
3. The Stage 1 submission of this manuscript contained the wording “children with RD” rather than individuals with RD in the methods, when the intention was to include all ages. This oversight was presented plainly to the editors and reviewers.
4. The study quality tool was adapted from its original form in order to better fit the study designs and constructs present in the literature. The original tool and the modified tool are presented in Supplemental Materials.
5. During final code review before publishing, authors found that power estimate calculation should have been ≥.99 rather than the value of .96 reported in the Stage 1 Registered Report.
6. The sample sizes (n)s of each age group do not add to the full sample because Goswami et al. (Citation2010) and Thomson and Goswami (Citation2008) are part of the same longitudinal study, in which the subjects were children at the initial timepoint and are adolescents by the final reported timepoint. These participants are counted twice only in descriptive statistics and are considered correlated effects in the meta-analyses.
7. Due to differences in the format of a dissertation vs. a published journal article with the same data (Zaidan, Citation2009; Zaidan & Baran, Citation2013, respectively), one study had ratings as both “poor” and “fair.” The “fair” study quality rating is used here.
References
- Ahissar, M., Lubin, Y., Putter-Katz, H., & Banai, K. (2006). Dyslexia and the failure to form a perceptual anchor. Nature Neuroscience, 9(12), 1558–1564. https://doi.org/10.1038/nn1800
- Amitay, S., Ben‐Yehudah, G., Banai, K., & Ahissar, M. (2002). Disabled readers suffer from visual and auditory impairments but not from a specific magnocellular deficit. Brain A Journal of Neurology, 125(10), 2272–2285 https://doi.org/10.1093/brain/awf231
- Ansari, D., & Gervain, J. (2018). Registered reports: Introducing a new article format in developmental science. Developmental Science, 21(1), e12650. https://doi.org/10.1111/desc.12650
- Araújo, S., & Faísca, L. (2019). A meta-analytic review of naming-speed deficits in developmental dyslexia. Scientific Studies of Reading, 23(5), 349–368. https://doi.org/10.1080/10888438.2019.1572758
- Araújo, S., Reis, A., Petersson, K. M., & Faísca, L. (2015). Rapid automatized naming and reading performance: A meta-analysis. Journal of Educational Psychology, 107(3), 868–883. https://doi.org/10.1037/edu0000006
- Banai, K., & Ahissar, M. (2004). Poor frequency discrimination probes dyslexics with particularly impaired working memory. Audiology and Neurotology, 9(6), 328–340. https://doi.org/10.1159/000081282
- Banai, K., & Ahissar, M. (2005). Psychoacoustics and working memory in dyslexia. In J. Syka & M. M. Merzenich (Eds.), Plasticity and signal representation in the auditory system (pp. 233–242). Springer Publishing Co. 2005-03154-021. https://doi.org/10.1007/0-387-23181-1_21.
- Chermak, G. D., Vivian, I., Doris-Eva, B., & E, M. F. (2018). Letter to the editor: Response to vermiglio, 2018, “The gold standard and auditory processing disorder” Perspectives of the ASHA Special Interest Groups, 3(6), 77–82. https://doi.org/10.1044/persp3.SIG6.77
- Compton, D. L. (2020). Focusing our view of dyslexia through a multifactorial lens: A commentary. Learning Disability Quarterly, 44(3), 225–230. https://doi.org/10.1177/0731948720939009
- Dean, A., & Voss, D. (Eds.), (1999). Checking model assumptions. In Design and analysis of experiments (pp. 103–134). Springer. https://doi.org/10.1007/978-3-319-52250-0_5
- Farmer, M. E., & Klein, R. (1993). Auditory and visual temporal processing in dyslexic and normal readers. Annals of the New York Academy of Sciences, 682(1), 339–341.
- Fisher, Z., Tipton, E., & Zhipeng, H. (2017). Robumeta: An R-package for robust variance estimation in meta-analysis. https://cran.r-project.org/web/packages/robumeta/vignettes/robumetaVignette.pdf
- Georgiou, G. K., Martinez, D., Vieira, A. P. A., & Guo, K. (2021). Is orthographic knowledge a strength or a weakness in individuals with dyslexia? Evidence from a meta-analysis. Annals of Dyslexia, 71(1), 5–27. https://doi.org/10.1007/s11881-021-00220-6
- Georgiou, G. K., Protopapas, A., Papadopoulos, T. C., Skaloumbakas, C., & Parrila, R. (2010). Auditory temporal processing and dyslexia in an orthographically consistent language. Cortex, 46(10), 1330–1344. https://doi.org/10.1016/j.cortex.2010.06.006
- Germanò, E., Gagliano, A., & Curatolo, P. (2010). Comorbidity of ADHD and dyslexia. Developmental Neuropsychology, 35(5), 475–493. https://doi.org/10.1080/87565641.2010.494748
- Gibson, L. Y., Hogben, J. H., & Fletcher, J. (2006). Visual and auditory processing and component reading skills in developmental dyslexia. Cognitive Neuropsychology, 23(4), 621–642. https://doi.org/10.1080/02643290500412545
- Goswami, U. (2011). A temporal sampling framework for developmental dyslexia. Trends in Cognitive Sciences, 15(1), 3–10. https://doi.org/10.1016/j.tics.2010.10.001
- Goswami, U., Gerson, D., & Astruc, L. (2010). Amplitude envelope perception, phonology and prosodic sensitivity in children with developmental dyslexia. Reading and Writing, 23(8), 995–1019. https://doi.org/10.1007/s11145-009-9186-6
- Goswami, U., Thomson, J., Richardson, U., Stainthorp, R., Hughes, D., Rosen, S., & Scott, S. K. (2002). Amplitude envelope onsets and developmental dyslexia: A new hypothesis. Proceedings of the National Academy of Sciences, 99(16), 10911–10916. https://doi.org/10.1073/pnas.122368599
- Greenhalgh, T., & Peacock, R. (2005). Effectiveness and efficiency of search methods in systematic reviews of complex evidence: Audit of primary sources. BMJ: British Medical Journal, 331(7524), 1064–1065. https://doi.org/10.1136/bmj.38636.593461.68
- Griffin, J. W. (2020). Metapower: Power analysis for meta-analysis (0.2.1). https://CRAN.R-project.org/package=metapower
- Gu, C., & Bi, H.-Y. (2020). Auditory processing deficit in individuals with dyslexia: A meta-analysis of mismatch negativity. Neuroscience & Biobehavioral Reviews, 116, 396–405. https://doi.org/10.1016/j.neubiorev.2020.06.032
- Halliday, L. F., & Bishop, D. V. M. (2006). Is poor frequency modulation detection linked to literacy problems? A comparison of specific reading disability and mild to moderate sensorineural hearing loss. Brain and Language, 97(2), 200–213. https://doi.org/10.1016/j.bandl.2005.10.007
- Hämäläinen, J. A., Salminen, H. K., & Leppänen, P. H. T. (2013). Basic auditory processing deficits in dyslexia: Systematic review of the behavioral and event-related potential/field evidence. Journal of Learning Disabilities, 46(5), 413–427. https://doi.org/10.1177/0022219411436213
- Hancock, R., Pugh, K. R., & Hoeft, F. (2017). Neural noise hypothesis of developmental dyslexia. Trends in Cognitive Sciences, 21(6), 434–448. https://doi.org/10.1016/j.tics.2017.03.008
- Harzing, A.-W., & Alakangas, S. (2017). Microsoft academic is one year old: The phoenix is ready to leave the nest. Scientometrics, 112(3), 1887–1894. https://doi.org/10.1007/s11192-017-2454-3
- Heath, S. M., Bishop, D. V. M., Hogben, J. H., & Roach, N. W. (2006). Psychophysical indices of perceptual functioning in dyslexia: A psychometric analysis. Cognitive Neuropsychology, 23(6), 905–929. https://doi.org/10.1080/02643290500538398
- Heath, S. M., & Hogben, J. H. (2004). The reliability and validity of tasks measuring perception of rapid sequences in children with dyslexia. Journal of Child Psychology and Psychiatry, 45(7), 1275–1287. https://doi.org/10.1111/j.1469-7610.2004.00313.x
- Hedges, L. V., & Pigott, T. D. (2004). The power of statistical tests for moderators in meta-analysis. Psychological Methods, 9(4), 426–445. https://doi.org/10.1037/1082-989X.9.4.426
- Hedges, L. V., Tipton, E., & Johnson, M. C. (2010). Robust variance estimation in meta-regression with dependent effect size estimates. Research Synthesis Methods, 1(1), 39–65. https://doi.org/10.1002/jrsm.5
- Helenius, P., Uutela, K., & Hari, R. (1999). Auditory stream segregation in dyslexic adults. Brain: A Journal of Neurology, 122(5), 907–913. https://doi.org/10.1093/brain/122.5.907
- King, W. M., Lombardino, L. J., Crandell, C. C., & Leonard, C. M. (2003). Comorbid auditory processing disorder in developmental dyslexia. Ear and Hearing, 24(5), 448–456. https://doi.org/10.1097/01.AUD.0000090437.10978.1A
- Law, J. M., Vandermosten, M., Ghesquière, P., & Wouters, J. (2017). Predicting future reading problems based on pre-reading auditory measures: A longitudinal study of children with a familial risk of dyslexia. Frontiers in Psychology, 8, 8. https://doi.org/10.3389/fpsyg.2017.00124
- Lonergan, A., Doyle, C., Cassidy, C., MacSweeney Mahon, S., Roche, R. A. P., Boran, L., & Bramham, J. (2019). A meta-analysis of executive functioning in dyslexia with consideration of the impact of comorbid ADHD. Journal of Cognitive Psychology, 31(7), 725–749. https://doi.org/10.1080/20445911.2019.1669609
- Marshall, C. M., Snowling, M. J., & Bailey, P. J. (2001). Rapid auditory processing and phonological ability in normal readers and readers with dyslexia. Journal of Speech, Language, & Hearing Research, 44(4), 925–940. https://doi.org/10.1044/1092-4388(2001/073)
- McWeeny, S., Choi, S., Choe, J., LaTourrette, A., Roberts, M. Y., & Norton, E. S. (2022). Rapid automatized naming (RAN) as a kindergarten predictor of future reading in English: A systematic review and meta‐analysis. Reading Research Quarterly, 57(4), 1187–1211. https://doi.org/10.1002/rrq.467
- Melby-Lervåg, M., Lyster, S.-A. H., & Hulme, C. (2012). Phonological skills and their role in learning to read: A meta-analytic review. Psychological Bulletin, 138(2), 322–352. https://doi.org/10.1037/a0026744
- Mody, M., Studdert-Kennedy, M., & Brady, S. (1997). Speech perception deficits in poor readers: Auditory processing or phonological coding? Journal of Experimental Child Psychology, 64(2), 199–231. https://doi.org/10.1006/jecp.1996.2343
- Moher, D., Liberati, A., Tetzlaff, J., Altman, D. G., & The PRISMA Group, (2009). Preferred reporting Items for systematic reviews and meta-analyses: The PRISMA statement. PLOS Medicine, 6(7), e1000097. https://doi.org/10.1371/journal.pmed.1000097
- Nittrouer, S., Krieg, L. M., & Lowenstein, J. H. (2018). Speech recognition in noise by children with and without dyslexia: How is it related to reading? Research in Developmental Disabilities, 77, 98–113. https://doi.org/10.1016/j.ridd.2018.04.014
- O’Brien, G., & Yeatman, J. (2020). Bridging sensory and language theories of dyslexia: Towards a multifactorial model. Developmental Science, e13039. https://doi.org/10.1111/desc.13039
- Page, M. J., McKenzie, J. E., Bossuyt, P. M., Boutron, I., Hoffmann, T. C., Mulrow, C. D., Shamseer, L., Tetzlaff, J. M., Akl, E. A., Brennan, S. E., Chou, R., Glanville, J., Grimshaw, J. M., Hróbjartsson, A., Lalu, M. M., Li, T., Loder, E. W., Mayo-Wilson, E., McDonald, S. … Moher, D. (2021). The PRISMA 2020 statement: An updated guideline for reporting systematic reviews. BMJ, 372, n71. https://doi.org/10.1136/bmj.n71
- Papadopoulos, T. C., Csépe, V., Aro, M., Caravolas, M., Diakidoy, I.-A., & Olive, T. (2021). Methodological issues in literacy research across languages: Evidence from alphabetic orthographies. Reading Research Quarterly, 56(S1), S351–S370. https://doi.org/10.1002/rrq.407
- Papadopoulos, T. C., Georgiou, G. K., & Parrila, R. K. (2012). Low-level deficits in beat perception: Neither necessary nor sufficient for explaining developmental dyslexia in a consistent orthography. Research in Developmental Disabilities, 33(6), 1841–1856. https://doi.org/10.1016/j.ridd.2012.04.009
- Peterson, R. L., & Pennington, B. F. (2015). Developmental dyslexia. Annual Review of Clinical Psychology, 11(1), 283–307. https://doi.org/10.1146/annurev-clinpsy-032814-112842
- Protopapas, A. (2014). From temporal processing to developmental language disorders: Mind the gap. Philosophical Transactions of the Royal Society B: Biological Sciences, 369(1634), 20130090. https://doi.org/10.1098/rstb.2013.0090
- Puolakanaho, A., Ahonen, T., Aro, M., Eklund, K., Leppänen, P. H. T., Poikkeus, A.-M., Tolvanen, A., Torppa, M., & Lyytinen, H. (2007). Very early phonological and language skills: Estimating individual risk of reading disability. Journal of Child Psychology and Psychiatry, 48(9), 923–931. https://doi.org/10.1111/j.1469-7610.2007.01763.x
- Ramus, F., Rosen, S., Dakin, S. C., Day, B. L., Castellote, J. M., White, S., & Frith, U. (2003). Theories of developmental dyslexia: Insights from a multiple case study of dyslexic adults. Brain A Journal of Neurology, 126(4), 841–865. https://doi.org/10.1093/brain/awg076
- R Core Team. (2013). R: A language and environment for statistical computing. R Foundation for Statistical Computing. http://www.R-project.org/
- Reed, M. A. (1989). Speech perception and the discrimination of brief auditory cues in reading disabled children. Journal of Experimental Child Psychology, 48(2), 270–292. https://doi.org/10.1016/0022-0965(89)90006-4
- Rodgers, M., & Pustejovsky, J. (2020). Evaluating meta-analytic methods to detect selective reporting in the presence of dependent effect sizes. MetaArXiv, 1–25. https://doi.org/10.31222/osf.io/vqp8u
- Rosen, S. (2003). Auditory processing in dyslexia and specific language impairment: Is there a deficit? What is its nature? Does it explain anything? Journal of Phonetics, 31(3), 509–527. https://doi.org/10.1016/S0095-4470(03)00046-9
- Schmidt, F. L. (2017). Statistical and measurement pitfalls in the use of meta-regression in meta-analysis. Career Development International, 22(5), 469–476. https://doi.org/10.1108/CDI-08-2017-0136
- Schulte-Körne, G., & Bruder, J. (2010). Clinical neurophysiology of visual and auditory processing in dyslexia: a review. Clinical Neurophysiology, 121(11), 1794–1809.
- Shamliyan, T., Kane, R. L., & Dickinson, S. (2010). A systematic review of tools used to assess the quality of observational studies that examine incidence or prevalence and risk factors for diseases. Journal of Clinical Epidemiology, 63(10), 1061–1070. https://doi.org/10.1016/j.jclinepi.2010.04.014
- Share, D. L. (2021). Is the science of reading just the science of reading English? Reading Research Quarterly, 56(S1), S391–S402. https://doi.org/10.1002/rrq.401
- Sharma, M., Purdy, S. C., & Kelly, A. S. (2009). Comorbidity of auditory processing, language, and reading disorders. Journal of Speech, Language, & Hearing Research, 52(3), 706–722. https://doi.org/10.1044/1092-4388(2008/07-0226)
- Snowling, M. J., Gooch, D., McArthur, G., & Hulme, C. (2018). Language skills, but not frequency discrimination, predict reading skills in children at risk of dyslexia. Psychological Science, 29(8), 1270–1282. https://doi.org/10.1177/0956797618763090
- Spearman, C. (1904). The proof and measurement of association between two things. The American journal of psychology, 15(1), 72–101.
- Strong, G. K., Torgerson, C. J., Torgerson, D., & Hulme, C. (2011). A systematic meta-analytic review of evidence for the effectiveness of the ‘Fast ForWord’ language intervention program. Journal of Child Psychology and Psychiatry, 52(3), 224–235. https://doi.org/10.1111/j.1469-7610.2010.02329.x
- Tallal, P. (1980). Auditory temporal perception, phonics, and reading disabilities in children. Brain and Language, 9(2), 182–198. https://doi.org/10.1016/0093-934X(80)90139-X
- Tallal, P., Merzenich, M. M., Miller, S., & Jenkins, W. (1998). Language learning impairments: Integrating basic science, technology, and remediation. Experimental Brain Research, 123(1), 210–219. https://doi.org/10.1007/s002210050563
- Thomson, J. M., & Goswami, U. (2008). Rhythmic processing in children with developmental dyslexia: Auditory and motor rhythms link to reading and spelling. Journal of Physiology-Paris, 102(1), 120–129. https://doi.org/10.1016/j.jphysparis.2008.03.007
- Thomson, J. M., Leong, V., & Goswami, U. (2013). Auditory processing interventions and developmental dyslexia: A comparison of phonemic and rhythmic approaches. Reading and Writing, 26(2), 139–161. https://doi.org/10.1007/s11145-012-9359-6
- Tong, X., Tong, X., & King Yiu, F. (2018). Beyond auditory sensory processing deficits: Lexical tone perception deficits in Chinese children with developmental dyslexia. Journal of Learning Disabilities, 51(3), 293–301. https://doi.org/10.1177/0022219417712018
- Valentine, J. C., Pigott, T. D., & Rothstein, H. R. (2010). How many studies do you need? A primer on statistical power for meta-analysis. Journal of Educational and Behavioral Statistics, 35(2), 215–247.
- Vermiglio, A. J. (2018). The gold standard and auditory processing disorder. Perspectives of the ASHA Special Interest Groups, 3(6), 6–17. https://doi.org/10.1044/persp3.SIG6.6
- Wang, K., Shen, Z., Huang, C., Wu, C.-H., Eide, D., Dong, Y., Qian, J., Kanakia, A., Chen, A., & Rogahn, R. (2019). A review of microsoft academic services for science of science studies. Frontiers in Big Data, 2, 2. https://doi.org/10.3389/fdata.2019.00045
- Whiting, P., Harbord, R., & Kleijnen, J. (2005). No role for quality scores in systematic reviews of diagnostic accuracy studies. BMC Medical Research Methodology, 5(1), 19. https://doi.org/10.1186/1471-2288-5-19
- Witton, C., Swoboda, K., Shapiro, L. R., & Talcott, J. B. (2020). Auditory frequency discrimination in developmental dyslexia: A meta‐analysis. Dyslexia: An International Journal of Research and Practice, 26(1), 36–51. https://doi.org/10.1002/dys.1645
- Witton, C., Talcott, J. B., Hansen, P. C., Richardson, A. J., Griffiths, T. D., Rees, A., Stein, J. F., & Green, G. G. R. (1998). Sensitivity to dynamic auditory and visual stimuli predicts nonword reading ability in both dyslexic and normal readers. Current Biology, 8(14), 791–797. https://doi.org/10.1016/S0960-9822(98)70320-3
- Wright, C. M., & Conlon, E. G. (2009). Auditory and visual processing in children with dyslexia. Developmental Neuropsychology, 34(3), 330–355. https://doi.org/10.1080/87565640902801882
- Zaidan, E. (2009). An investigation of temporal resolution abilities in school-aged children with and without dyslexia. Thesis. University of Massachusetts Amherst.
- Zaidan, E., & Baran, J. A. (2013). Gaps-in-noise (GIN©) test results in children with and without reading disabilities and phonological processing deficits. International Journal of Audiology, 52(2), 113–123. https://doi.org/10.3109/14992027.2012.733421