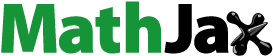
ABSTRACT
Sustainable soil nutrient management (SNM) is important for improving soil resources and food security in sub-Saharan African countries. Inherent livelihood diversity may lead to differences in household behavior in the adoption of SNM practices, thus challenging policy interventions uniformly implemented. This study aims at exploring the responsive heterogeneity in household behavior in the adoption of fertilizer use by farmers. Determinants of fertilizer uses were analyzed across different farm types. These farm types were identified from farm-household data collected in six villages in the South Western of Burkina Faso using the Sustainable Livelihood Framework. Using multilinear and binary logistic models, determinants of mineral, organic and combined mineral-organic fertilizer uses were analyzed. The results revealed that the determinants of SMN adoption include not only common determinants to whole sampled population (income, household size, access to roads and cereal areas), but also specific determinants to farm types (small ruminants, animal power, educational level and access to agro-training). This finding suggests that policy interventions will not be effective if the responsive heterogeneity in SNM adoption behavior is not taken into account. The study recommends the use of the presented framework, as an approach, to identify functional farm types to be considered in SNM policies.
1. Introduction
Hunger and undernourishment are still rife in Sub-Saharan Africa. For decades food insecurity affects many countries without any improvement (FAO, Citation2006; FAO, Citation1993; FAO et al., Citation2013; FAO et al., Citation2020) despite numerous interventions. In Burkina Faso, in particular, 47.7% of the total population was food insecure, while 19.2% were undernourished in 2017–2019 (FAO et al., Citation2020). Food insecurity fails households to ensure sustainable food production (Devereux & Maxwell, Citation2001; Devereux, Citation2009). Most cropping systems in Sub-Saharan Africa (SSA) are characterized by alarming soil nutrient depletion (Stoorvogel & Smaling, Citation1990; Bationo et al., Citation1998; LAL, Citation1995; Cobo et al., Citation2010; Van Beek et al., Citation2016). Soil degradation affects food security through low crop yields and reduced household income (LAL, Citation2009; STOCKING, Citation2003; Pimentel & Burgess, Citation2013; Grote, Citation2014; Dzanku,Jirström, & Marstorp, Citation2015).
Combatting food insecurity requires tackling soil nutrient mining issues while improving food productivity and profitability. Adoption of proven soil fertility management practices (e.g. mineral and organic fertilizers) can improve crop productivity (LAL, Citation2009; Vlek et al., Citation1997; Ingram et al., Citation2008). Indeed, mineral fertilizers are the shortest way for replenishing soil with macronutrients and avoiding widespread nutrient mining. Organic fertilizers improve soil fertility (Gaur & Singh, Citation1993) and smallholders are unable to purchase mineral fertilizers (Palm et al., Citation2001). It improves soil’s physical properties through the strengthening of organic matter (Vanlauwe & Giller, Citation2006; Palm et al., Citation1996; Ding et al., Citation2012; Körschens et al., Citation2012). Organic fertilizer provides the soil with microorganisms which are vital to biological functions, allowing nutrient cycling leading to the release of nutrients (Craswell & Lefroy, Citation2001). Beyond macronutrients, it can contain micronutrients (Cu, B, Zn, Mn, Mo) which greatly increase soil productivity (Parr & Colacicco, Citation1987). The combined use of organic and mineral fertilizers reduces nitrogen losses in sole mineral fertilizer use (Neeteson, Citation1993). It was reported to generate better yields than the sole use of mineral fertilizers (Ding et al., Citation2012; Kearney et al., Citation2012; Kismányoky & Tóth, Citation2013; Mucheru-Muna et al., Citation2007).
However, the adoption of Sustainable Land Management (SLM) practices by smallholders, including Sustainable Nutrient Management (SNM) practices, remains very low in SSA (Place et al., Citation2003; Bationo et al., Citation2006; Chianu et al., Citation2012; Chianu et al., Citation2012b; Anley et al., Citation2007). Inefficient agricultural policies (Anley et al., Citation2007) result in the low adoption of SNM. Indeed, interventions promoting SNM adoption often implement uniform policies while the farmers’ population is characterized by inherent social and ecological diversity, as highlighted by (Ojiem et al.(Citation2006). It is necessary to better understand factors affecting farmers’ adoption and based on that to inform policy leveraging farmers’ incentive to adopt.
Most studies (Makokha et al., Citation2001; Waithaka et al., Citation2007; Adesina, Citation1996; Chianu & Tsujii, Citation2005; Lambrecht et al., Citation2014; Kassie et al., Citation2013) analyzed the effects of a range of different factors on nutrient adoption/use but with a uniform affecting pattern. However, given an affecting factor, its significance, affecting direction and magnitude can be different over different types of farms/farmers. This responsive heterogeneity needs to be understood.
Therefore, the research questions of the present paper are (i) what are the common determinants of mineral and organic fertilizer uses? (ii) What are the farm/farmer type-specific determinants of mineral and organic fertilizer uses? We formulate the following hypotheses: (i) there are particular factors affecting mineral and organic fertilizer uses in the same patterns; (ii) different farm types (with distinct agricultural livelihood systems) can respond differently to the same affecting factor in different circumstances (significance level, affecting direction and magnitude).
2. Material and methods
2.1. Study area
The study was conducted in the South-Western region of Burkina Faso in 2013-2014. The region represents 6% of the country’s territory (INSD, Citation2009a) and 4.32% of its population in 2014 (772,973 inhabitants) according to projections by INSD (Citation2009b). Around 88% of the population lives in rural areas. Population density in Ioba province is the highest in the region (59.0 inhabitants/km2)(INSD, Citation2009a) and is situated above the average at the country level (51.8 inhabitants/km2). Annual population growth is estimated to be 1.8% and the population is dominated by females (52%)(INSD, Citation2009b; INSD, Citation2013). Due to land pressure, the use of fallow for replenishing soil fertility has considerably dropped (DREP/Sud-Ouest and PNGT, Citation2000). Ioba province was identified to have the most degraded lands in the south-western region (MAHRH and GTZ, Citation2004). Mineral fertilizers and compost uses remain low (MAHRH, Citation2010; Gleisberg-gerber, Citation2012). These characteristics guided the choice of the province for conducting the present study. Three communes (Dano, Koper and Ouessa) were selected () based on land use, population, land degradation information and programmes promoting sustainable land use management. Soils are generally shallow, inherently poor and vulnerable to erosion. Washed ferruginous tropical indurated soils form dominating soil types. Livelihood activities comprise mainly rain-fed agriculture, trade, handicraft and mining. The main agricultural components are cereals, cotton (the main cash crop) and animal husbandry. The study area belongs to the South-Sudanian climatic zone with savannah vegetation and mono-modal rainfall. Average annual rainfall varies between 900 and 950 mm and the province experiences erratic rainfall variability (MAHRH and GTZ, Citation2004).
2.2. Methods and data
2.2.1. Conceptual framework
The conceptual framework of this study () is based on Sustainable Livelihood framework (DFID, Citation1999; De Sherbinin et al., Citation2008; Sconnes, Citation1998). The adoption/use of sustainable land management practices by the farmer/farm is a function of available resources (Jones, Citation2002). The readiness of farmers to apply soil nutrients is not just affected by the perception of soil fertility. It is also determined by farm assets (e.g. natural, physical, financial, social and human assets). In pursuing its livelihood objectives (e.g. food security and well-being), the farmer mobilizes and allocates its assets within a livelihood strategy. This strategy needs to be accounted for when analyzing soil fertility management.
Given the level of asset endowment, farmers will have more or fewer options for building livelihood strategies (DFID, Citation1999). They will, therefore, be more or less inclined to adopt and use soil nutrients. For instance, a farm above the poverty line can still choose to use its financial resources for consumption, savings or other types of investment rather than investing in soil fertility (Reardon & Vosti, Citation1995). Poor endowment in physical assets (e.g. road and communication facilities) reduces farm exposition to SNM technologies and access to markets for mineral fertilizer acquisition. Farm tools and equipment are useful for composting and transportation of fertilizer to plots. Besides being an important source of cash (Murungweni et al., Citation2014) facilitating farm acquisition of mineral fertilizer, livestock provides draught power and manure. Also, the availability of natural assets such as land may lead to extensive farming while land constraints may compel farmers to use more fertilizer (Tittonell et al., Citation2005a). Social assets (e.g. networks) provide learning opportunities and improve farm access to fertilizer (e.g. credit system, remittances). Livelihood-based farm typology gives, therefore, more insight into soil fertility management (Bidogeza et al., Citation2009; Tittonell et al., Citation2005b; Tittonell et al., Citation2010; Oumer et al., Citation2013). Besides common determinants, there are farm-type-specific factors that influence the response of farms to soil degradation and, therefore, affect the adoption/use of soil nutrients. This specific responsiveness is inherent to the level of asset endowment and the farm livelihood strategy profile. It may change over time with the change in farm livelihood strategy and asset endowment.
2.2.2. Dependent variables
Because of limited access, farmers may apply a meaningless amount of nutrients. We assume that the applied amount of nutrients can be considered meaningful when it is more than 10% of the recommended application rate. Only farmers observing this threshold were considered users of mineral and organic fertilizers. Dependant variables and corresponding inferential statistical methods used are summarized in .
Table 1. Dependent variables and corresponding inferential statistical methods.
The use/adoption of mineral fertilizers (NPK + Urea): NPK (14-23-14) and Urea (46N) are the commonly used mineral fertilizers in the study region. Given the previously defined threshold and recommended rates of application for mineral fertilizer (CILSS, Citation2012), fertilizer users apply more than 9.7 kg N-P-K ha/year. The dependent variable Mineral fertilizer use intensity (YMinUse) expressed the amount of mineral nutrients annually applied per unit of land (kg N-P-K ha/year). It was computed by dividing the amount of nutrients applied by the total cultivated area with and without mineral fertilizer. The variable Adoption of mineral fertilizer use (YMinApt) reflected the farm’s choice to apply or not a mineral nutrient. YMinApt = 1 if the farm adopts mineral fertilizer and 0, otherwise.
Adoption of organic fertilizers (YOrgApt): the variable reflected the farm’s choice to apply or not organic fertilizer (compost and animal dung). YOrgApt = 1 if the farm adopts organic fertilizer and 0, otherwise. According to recommended application rates from the Ioba provincial directorate of Agriculture and CILSS (2012), and given the threshold defined above, organic fertilizer users apply more than 4.1 kg N-P-K ha/year and 2.7 kg N-P-K ha/year from compost and animal dung, respectively.
Mineral and organic fertilizers (YMinOrg) are adopted. This variable expressed the combined use of mineral and organic fertilizers (Mineral-organic fertilzers). YMinOrg = 1 if the farm applies both mineral and organic fertilizers and 0, otherwise.
2.2.3. Inferential statistical methods
Multiple linear regression (MLR) was used for explaining mineral fertilizer use intensity (YMinUse). MLR is a common inferential statistical method for continuous variables.
where Xi is an explanatory variable, βi (i = 1, 2, 3, … , n) their weights and α is the intercept.
Binary logit regressions were used for adoption analysis. Binary logit regression is a common method used for adoption analysis.
With y = YMinApt; YOrgApt or YMinOrg, and p(y = 1) the probability of y = 1.
In the study region, maize is the main food crop for which farmers usually apply nutrients. The number of fertilized plots of other food crops in the study sample was not high enough for conducting regression analyses. Inferential analyses were then performed only for maize plots. Three farm types out of the five farm types identified in the study area had a high enough number of fertilized maize plots for regression analyses. For each dependent variable, we estimated effects of hypothesized determinants (Xi) in the whole sample plots (n = 292 plots), and in the three main farm types (i.e. Better-off, cotton-and livestock-based farms (n = 107), Better-off, non-farm activities preference farms (n = 104) and Pro-poor, labourless-and landless farms (n = 81)).
2.2.4. Explanatory variables
Candidate explanatory variables and their hypothesized effects are presented in . They were gathered from the literature and based on Sustainable Livelihood Framework (SLF).
Table 2. Brief description and hypothesized explanatory variables for nutrient adoption and use.
Variables of financial assets: Poverty is often a factor fuelling land degradation (Scherr, Citation2000; Vu et al., Citation2014b) and constraining the use of soil nutrients by smallholder farms. The variable Cash income per capita per year (HCashCp) and the variable Remittance income per capita per year (HRemitCp) increase farm income and are expected to augment the chance for farmers to adopt mineral nutrients and increase their use intensity. Amekawa (Citation2013) highlighted that remittance can be a valuable source of income for farms. We hypothesize that these variables are common factors affecting nutrient adoption and use.
Variables of natural assets: cropped land allocation within the farm contributes to determining soil management practices. Some crops (e.g. maize and rice) are more demanding in nutrients, while others (legumes) are less and even contribute to enriching the soil through biological N fixation therefore reducing the nutrient mining effect (Enyong et al., Citation1999). An increase in the size of maize and rice plots may reduce the adoption and use of soil nutrients because of limited access. By planting more legumes, the farm has the opportunity to save soil nutrients for other crops. Also, by cultivating intervention-targeted crops, farms have more chance to adopt and use nutrients due to facilitated access to fertilizers (e.g. credit system for conventional cotton). Therefore, the area of legumes per capita (HLegCp) and the area of conventional cotton per capita (HCCotCp) are expected to augment the chance of nutrient adoption and increase their use. However, the area of maize and rice per capita (HMzeRceCp) will tend to reduce the adoption and use of nutrients. Through monetary income generation, the area of dry season irrigated land per capita (HIrrigCp) is expected to augment the chance of mineral nutrient adoption and its use intensity. Livestock is an important source of nutrients through manure production (Place et al., Citation2003; Kassie et al., Citation2013). Several small ruminants (goats and sheep) per capita (HSRumCp) are hypothesized to have a positive effect on organic fertilizer adoption and a negative effect on the adoption and use of mineral fertilizer. The expected common determinants are HMzeRceCp, HCCotCp, HLegCp and the expected farm type-specific determinants are HIrrigCp and HSRumCp.
Variables of human assets: Human assets are very important for the successful use of the other assets in sustainable nutrient management (SNM). Household size (HSize) is a source of labour necessary for applying available soil nutrients. The Age of the household head (HHAge) may reflect the accumulated experience of the farm and compel it to adopt and use sustainable nutrient management practices (Ketema & Bauer, Citation2011; Mkhabela & Materechera, Citation2003). Both variables may also have a negative effect (Freeman & Omiti, Citation2003; Chianu & Tsujii, Citation2005). In effect, the load of large household size may limit available cash for purchasing mineral fertilizer. Ageing labour may reduce available labour for composting or recycling crop residue, for instance, and render farmers more risk-averse. Their hypothesized effects on nutrient adoption and use are unclear. These two variables, however, are expected to act as common factors affecting soil nutrient use. Educated farmers may better understand the importance and benefit of sustainable nutrient management. Training, through learning and exposure to SNM practices (e.g. Field schools and demonstration plots) may render farmers more receptive to new technologies and SNM practices, in particular. Therefore, the number of education years of the household head (HHEdu) (Freeman & Omiti, Citation2003) and the number of times household members attended a training session in the last five years (HTraining) (Nkamleu, Citation2007) are expected to increase adoption and use intensity of soil nutrient. We hypothesized that they will be group-specific determinants: HHEdu is expected to be significant for farm types with more educated heads, while HTraining is expected to be significant for farm types with less educated heads.
Variables of physical assets: Animal power (HAniPowCp) plays an important role (Kassie et al., Citation2013) in smallholder farms usually not or very lowly mechanized. It allows for saving time for ploughing. It also helps transportation, to distant plots, of organic fertilizer usually applied to homestead plots (Kassie et al., Citation2013). HAniPowCp is expected to increase the farm’s chance of adopting and using more intensive soil nutrients. Farms with low access to permanent roads may also have low access to income generation opportunities and important markets. This may constrain their capabilities to purchase fertilizers or farming equipment. Therefore, the Average distance of households to the nearest paved road (HDistRoad) is expected to reduce farm’s chance of adopting and using mineral nutrients. The resulting low access to fertilizer can compel farms to turn into the adoption of organic fertilizer or not. So, the expected effect of HDistRoad on mineral nutrient adoption and use is negative but unclear for the adoption of organic nutrients.
2.2.4. Evaluation of models’ performance
The existence of multi-collinearity was checked using Variance Inflation Factor (VIF) and contingency coefficient. There will be a risk of multi-collinearity if VIF is greater than 5 and the contingency factor is less than 0.2 (Defries et al., Citation2010). The performance MLR model was evaluated using F-statistics for overall performance and adjusted R-square for goodness-of-fit. A model can be considered as having good performance for R-square values around 0.5 and 0.3 (Greene, Citation2012). For binary logistic regressions, we used the Chi-square test to evaluate the model’s overall performance. The goodness-of-fit was evaluated using the per cent of good prediction and area under the Receiver Operating Characteristic (ROC) curve (Hosmer & Lemeshow, Citation2000). For values of area under ROC of 0.60-0.70, the model performance is appreciated to be poor; 0.70-0.80, acceptable; 0.80-0.90, good and 0.90-1, excellent. The partial effect of explanatory variables was appreciated using p-values.
2.2.5. Data source
Data were collected during the dry season of 2013 from January to March. A semi-structured questionnaire guided by Sustainable Livelihood Framework (SLF) was used to gather information on households’ livelihood assets, nutrient management and production orientation. Geographical data were also collected using a Geographical Positioning System (GIS). The survey covered 360 households randomly selected in 6 villages of the Ioba province. The typology of surveyed households yielded in five main Agricultural livelihood system (ALS) types: ALS type I: Poor, cotton- and livestock-based farms (n = 105, 31% of the sample population with an income of 94 $US/person/yr); ALS type II: Medium, non-farm activities preference farms (n = 102, 31% of the sample population and an income of 155 $US/person/yr); ALS type III, very-poor, labourless-and landless farms (n = 71, 21% of the sample population and an income of only 55 $US/person/yr); ALS type IV, poor, labour-rich, marketable food crop oriented and educated farms (n = 29, 9% of the sample population and an income of 104 $US/person/yr); and ALS type V, very poor, insecure-land tenure, livestock-based farms (n = 28, 9% of the sample population and earning only 56 $US/person/yr). A total number of 349 maize plots were identified for all farms.
3. Results
3.1. Model’s performance
No multicollinearity was found between explanatory variables (VIF < 0.5 and tolerance >0.2) (See Table S1). The MLR was significant at 1% (). The prediction power of models was strong: R2 = 0.44, R2 = 0.36 and R2 = 0.41 for fam-types I, II and III respectively. But it was less strong for the whole population: R2 = 0.24. As for bi-logit models, Hosmer and Lemeshow test at 5% showed a good fit of the models to the data for mineral fertilizer adoption for the whole population, farm-types II and III (p > 0.05) but not for farm type I (p < 0.05). Values of the area under ROC showed good performance of models for the whole population, farm types I and II (0.81, 0.88 and 0.86, respectively) and excellent for ALS type III (0.91). Models for organic fertilizer adoption also had good overall performance (p > 0.05 for Hosmer and Lemeshow test). The Area under ROC varied from 0.68 to 72.6 ( and ). The results of the Hosmer and Lemeshow test () showed good performance for models of combined mineral-organic nutrient adoption too: p > 0.05. Prediction power was good (77-85%) and Area under ROC varied from 0.77 to 0.85.
Table 3. Multiple linear regression analyses for factors affecting mineral fertilizer use (kg of N-P-K ha−1 year−1).
Table 4. Binary logistic regressions for factors affecting farmer adoption of mineral fertilizer.
Table 5. Binary logistic regressions for factors affecting farmer adoption of organic fertilizer.
Table 6. Binary logistic regressions for factors affecting farmer adoption of both mineral and organic fertilizer.
Explanatory variables did not affect fertilizer use and adoption in the same way (e.g. direction and amplitude) for the whole population and different farm types. We distinguished two different types of affecting factors. (i) Common factors affecting fertilizer use and adoption by the whole population and different farm types. A common factor exhibits the same direction for the whole population and across affected farm types. (ii) Farm-type-specific factor affects a particular farm type and does not appear to be an affecting factor for the whole population. This type of factor was named type specific factor of the first order. A factor may also affect a particular farm type in a direction opposite to its affecting direction for the whole population and for other affected farm types. Or, it may affect a particular farm type and only the whole population in the same direction but with lower amplitude for the whole population. This suggests that the presence of non-affected fam types in the whole population lowers coefficient amplitude. This affecting factor was called type-specific factor of second order.
3.2. Determinants of mineral fertilizer use and adoption
3.2.1. Common affecting factors
As expected, the variable HAniPowCp had a positive effect (). The number of draught animals increases mineral fertilizer use by farmers for the whole population, farm types I and II. HDistRoad, contrary to expectations, had a positive effect on mineral fertilizer use. It was statistically significant for the whole population, fam-type I and III. Remoteness augmented the use of mineral fertilizer. The age of household members (HAge) reduced the use of mineral fertilizer. Aged household members may mobilize less financial resources for purchasing fertilizer. Also, old farmers may not fully understand the importance of sustainable soil nutrient management, on the contrary, young farmers are usually better educated and more open to new technologies. HAge was significant for the whole population only, not for individual farm types.
Common affecting factors for mineral fertilizer adoption were Cash income per capita per year (HCashCp), Household size (HSize) and Average distance of household to the nearest paved road (HDistRoad). They all had a positive effect on fertilizer adoption (). HCashCp was statistically significant at 5% for the whole population, farm types I and II. HSize and HDistRoad were significant for the whole population but not for individual farm types, at 5% and 1% respectively. Cash income per capita per year, Household size and Average distance of household to the nearest paved road increased the chance for farmers to adopt mineral fertilizer.
3.2.2. Farm-type specific affecting factors
The majority of statistically significant variables for multiple linear regression (six over nine) were farm-type-specific affecting factors (). Variables of financial assets (e.g. HCashCp and HRemitCp) had a positive effect on mineral fertilizer use for both the whole population and only one of the three farm types. They were type-specific affecting factors of second order. HIrrigCp (Natural asset), HTraining (Human asset) and HMzeRceCp were also type-specific affecting factors of second order. HIrrigCp, and HTraining had a positive effect, while HMzeRceCp had a negative effect on mineral fertilizer use intensity as expected. The amplitude of the coefficients of variables was larger for farm types than for the whole population. Only one farm-type-specific affecting factor of the first order was found: HSrumCp (financial asset). HSrumCp appeared statistically significant only for ALS type III and increased mineral fertilizer use intensity. It was not significant for the whole population.
The bi-logit regression revealed more farm-type-specific affecting factors of the first order (). HRemitCp and HTraining were significant only for ALS type I and ALS type II, respectively. They increase the chance for the farmer to adopt mineral fertilizer. HMzeRceCp was significant for both ALS type I and ALS type II but with opposite affecting direction and amplitude. It increases the chance of adopting mineral fertilizer for ALS type, I while reducing it for ALS type II. HIrrigCp, HSrumCp and HAniPowCp were all farm-type-specific affecting factors of the second order. In effect, in addition to the whole population, they increased the chance of adopting mineral fertilizer for only ALS type I and ALS type II, respectively. Their coefficients were larger for farm types than for the whole population.
3.3. Determinants of organic fertilizer adoption
3.3.1. Common affecting factors
Two common affecting factors associated with the adoption of organic nutrients in the whole population were identified. Remittance income per capita per year (HRemitCp) was positively associated with organic nutrient adoption, and the Area of maize and rice per capita (HMzeRceCp) was negatively associated with organic nutrient adoption. HRemitCp had the opposite of the expected direction and HMzeRceCp had the expected direction. These common variables were not significant for individual farm types.
3.3.2. Farm-type specific affecting factors
The number of small ruminants per capita (HSrumCp) and the Average distance of the household house to the nearest paved road (HDistRoad) were positively associated with organic nutrient adoption for Farm type I. Within this farm type, the chance for farmers to adopt organic nutrient increase with an endowment in small ruminants (goat and sheep) and distance from paved roads. In ALS type II, however, the adoption of organic nutrients was positively associated with animal power per capita (HAniPowCp). The higher the number of draught animals the farm possesses, the higher the chance of adopting organic nutrients. HAniPowCp and HSrumCp had the expected effect.
3.4. Determinants of adoption of combined mineral-organic fertilizers
3.4.1. Common affecting factors
The adoption of combined mineral-organic fertilizer was influenced by three common affecting factors (). Two of these factors were significant for the whole population and had no significant effect on individual farm types: HCashCp increases the adoption of combined mineral-organic fertilizer and HMzeRce reduces this adoption in the whole population. HDistRoad was statistically significant for farm types I, II and the whole population. The average distance of a household to sthe nearest paved road (HDistRoad) increases the chance of adopting combined mineral-organic fertilizer use.
3.4.2. Farm-type-specific affecting factors
Farm-type-specific affecting factors of first order included HSrumCp, HHEdu and HTraining. They were all likely to increase the chance for farmers to adopt combined mineral-organic fertilizer use. HSrumCp was significant for ALS type I. As for HHEdu and HTraining, they had a significant effect on ALS type III and ALS type II, respectively. Farm-type-specific affecting factors of the second order involved HIrrigCp, HSize, and HAniPowCp. An increase in the Area of dry season irrigated land per capita (HIrrigCp) significantly increases the adoption of combined mineral-organic fertilizer use for ALS type I and for the whole population. The effect on adoption had the lowest amplitude for the whole population compared to ALS type I. The chance for adopting combined mineral-organic fertilizer use augmented with household size (HSize) for ALS type III and the whole population with the highest effect amplitude for ALS type III. As for HAniPowCp, it positively affects the adoption of combined mineral-organic fertilizer use for ALS type III and Whole population, with the lowest effect amplitude for the Whole population.
4. Discussion
4.1. Contextualization of the main findings compared to other research
Identifying and understanding factors affecting the adoption and use of fertilizer in a region is crucial for improving sustainable soil nutrient management in that region. In using a set of thirteen socio-economic and ecological independent variables we found results consistent with findings of past studies. Farm financial assets play a significant role in soil nutrient management as noted by Nkamleu (Citation2007), (Citation2007), Marenya and Barrett (Citation2007), (Citation2013) and (Citation2014). Indeed, we found that farm income components Cash income (HCashCp) and Remittance received per capita (HRemitCp) augment the use intensity and adoption of mineral and organic nutrients by farms. In a study conducted in another region of Burkina Faso, Somda et al. (Citation2002) observed that farm income significantly augments the adoption of organic fertilizer. However, like in our study, they pointed out that animal power (HAniPow) and training (HTraining) have no significant effect on organic fertilizer adoption. This suggests that income generation constitutes the main entry for interventions aiming at improving organic fertilizer adoption.
The study also revealed that dry season irrigation land per capita (HIrrigCp) had a significant effect on mineral fertilizer use and adoption, and combined mineral-organic fertilizer adoption. In rural areas where off-farm activities opportunities are often limited, dry season irrigation is a valuable source of income that can be invested in purchasing mineral fertilizer. This shows that the adoption and use of mineral fertilizer can be increased by policy interventions promoting dry season irrigation as noted by Yilma and Berger (Citation2006). Not surprisingly, Training (HTraining) increases mineral fertilizer use and adoption. This is consistent with the findings of Martey et al.(Citation2014) and Grabowski and Kerr (Citation2014) who highlighted that proximity with extension services improves the adoption of mineral and organic fertilizers. Distance from paved roads (HDistRoad) increased the use and adoption of mineral and organic fertilizers. As shown by Vu et al. (Citation2014) distance to the road increases land degradation extent and thereby compels farmers to use more fertilizer. This effect of HDistRoad can also be seen as a sign of the declining importance of distribution constraints on fertilizer use through increased fertilizer retail outlets availability in rural areas (Freeman & Omiti, Citation2003). The positive effect of animal power (HAniPowerCp) on mineral nutrient use intensity and its positive influence with a small ruminant number (HSRumCp) on mineral fertilizer adoption (Kassie et al., Citation2013) demonstrate the beneficial interrelationship between livestock and cropping activities (Kristjanson et al., Citation2005; Marenya & Barrett, Citation2007). The integration of livestock agriculture needs, therefore, to be boosted for better soil nutrient management.
Studies use to consider a uniform affecting pattern when analysing the adoption and use of fertilizers. Our study, on the contrary, considered that due to differences in their socio-economic and ecological characteristics, farmers/farms are not affected in the same way by determinants of fertilizer use and adoption. Besides common affecting factors, type-specific factors were found that affected only specific farm types, with different amplitude or with different directions. In other words, parameters of independent variables (β) varied across socio-ecological farm types. It demonstrated the importance of considering farm types rather than the whole population only when analysing soil nutrient management. Considering the whole population only can be misleading and cause the failure or inefficient policies and intervention measures. To the best of our knowledge, it is hard to find a study which analysed variation of affecting patterns in sustainable soil nutrient management. The work presented in this paper can, therefore, be considered as new.
4.2. New methodological features of the present study
Although the benefits of combinational use of mineral and organic fertilizers have been recognized (e.g. reduction of N losses (Neeteson, Citation1993); organic matter (Vanlauwe & Giller, Citation2006; Palm et al., Citation1996; Ding et al., Citation2012; Körschens et al., Citation2012); nutrient cycling in the system (Mkhabela & Materechera, Citation2003), long-term soil fertility improvement (Mkhabela & Materechera, Citation2003), increase in soil productivity (Parr & Colacicco, Citation1987); generate better yields than the sole use of mineral fertilizers (Ding et al., Citation2012; Kearney et al., Citation2012; Kismányoky & Tóth, Citation2013; Mucheru-Muna et al., Citation2007)), published work analyzing factors affecting the adoption of these combinational uses are rather scarce.
Also, in this study, responsive/behaviour/preference parameters (betas) are analyzed specific to different farm/household types rather than uniform/constant to the whole population. This way of analysis allows the capture of responsive heterogeneity. The study demonstrates the relationship between structural and functional typologies and the importance of consideration of them in regional farming system studies. In addition, the results can provide an empirical framework for scaling-out studies. Responsive heterogeneity has been considered in land use choice analyses (Le, Citation2005), but in only a few mineral adoption analyses (Vu & Frossard, Citation2014). However, Vu and Frossard (Citation2014) work is in Vietnam where the background of fertilizer uses and the social and ecological conditions are very different from Burkina Faso. So far, it is hard to find a work similar to our study in SSA or West African dry land.
4.3. Implication for policies leveraging wide adoption of SLM
Burkina Faso is an agriculture-based country where more than 80% of the population relies on agriculture and related activities for their livelihood (SPCPSA, Citation2013). Despite numerous policies and intervention measures, food security remains an issue and land degradation is a growing threat. There is a necessity of implementing efficient policies for combating land degradation and food insecurity. The results of this study pave the way for new ways of intervention in the agricultural sector for sustainable land management.
In the first instance, the study contributes to knowledge of affecting factors of sustainable nutrient management. It shows that policies need to support the development of dry-season irrigation crops as an indirect way of increasing the use and adoption of Sustainable Nutrient Management (SNM). In the second instance, policies and intervention measures need henceforth, to formulate and implement well-targeted policy following the framework presented in this study. This will help to account for responsive heterogeneity and render policies and intervention measure more successful. As hinted by Rodenburg et al. (Citation2021) for the adoption of Conservation agriculture practices, land management can be greatly improved in Subs-Saharan Africa by taking into account smallholder conditions and constraints, which implies accounting for their heterogeneities.
4.4. Limitations and the way forwards
Despite interesting results, the study presents some limitations. First, the study used only MLR and bi-logit. It is important to also use a multinomial logit to explore the responsive heterogeneity and analyze the marginal effects of the variable. Second, besides inferential methods, the responsive heterogeneity could be also examined using non-parametric methods such as Participatory Rural Appraisal tools (PRA) nd decision tree analysis which can allow the capture effect of factors having less variation in sampled data (e.g. climate, prices).
5. Conclusion
Successfully improving sustainable soil nutrient management and the sustainability of agriculture in most Sub-Saharan African countries is still a challenge for governments and other rural development stakeholders. Despite the improved management practices and technologies, adoption by farmers is still low; and a better understanding of how to leverage adoption by smaller farmers is needed. The findings of this study highlight the importance of considering responsive heterogeneity in adoption interventions. It demonstrated the relationship between structural and functional typologies and the importance of considering both of them in Burkina Faso in particular and in Sub-Saharan African age ecosystems in general. The study used cross-sectional data for the analyses. Given that land management is a dynamic process, there is a need to explore the responsive heterogeneity with time series data. More biophysical data (e.g. rainfall) could then be included in the analysis. The study used only objective data. Given the influence of social settings in human-environment relations, it will be good to take second steps including perception data.
Supplemental Material
Download MS Word (13.7 KB)Acknowledgements
The authors thank the West African Science Service Center on Climate Change and Adapted Land Use (WASCAL) which funded the Ph.D. Studies of the corresponding author at Kwame Nkrumah University of Science and Technology (KNUST). They also thank CGIAR Research Programme on Dryland Systems (CRP-DS) for the study visits. QBL thanks the support from CGIAR Initiatives on Sustainable Intensification of Mixed Farming Systems and Agroecology.
Disclosure statement
No potential conflict of interest was reported by the author(s).
Additional information
Funding
Notes on contributors
Boundia Alexandre Thiombiano
Dr Boundia Alexandre Thiombiano is a Socio-economist and a Senior Lecturer at the Institute for Rural Development at University Nazi BONI in Burkina Faso. He holds a PhD in Climate Change and Adapted Land use from the Kwame N'Krumah University of Science and Technology in Ghana. He has been a graduate fellow of the West African Science Service Center on Climate Change and Adapted Land Use (WASCAL). His research interest covers mainly agricultural livelihoods resilience, farming system design, agricultural value chains, climate change, sustainable land management and agent-based modeling. He was an external partner of the CGIAR Research Program on Grain Legumes and Dry Cereals (CRP GLDC) at International Center at International Center for Agricultural Research in Dry Areas (ICARDA).
Quang Bao Le
Quang Bao Le (Ph.D., University of Bonn) has been an Agricultural Livelihood Systems Expert at International Center for Agricultural Research in Dry Areas (ICARDA) since 2015. Before joining ICARDA, Dr. Le was a Senior Researcher (2011-2015) at Swiss Federal Institute of Technology (ETH) Zurich. With a coupled human-environment system perspective, for the past 18 years his research has focused on the development and application of human-environment system methodologies to integrate multi-disciplinary scientific expertise with societal decision-making processes to foster sustainable agricultural livelihood systems. He received Breheny Prize in 2013 for the best paper in Environment and Planning B journal and the Awards of Swiss Academy of Arts and Sciences 2013 for “Distinguished Achievement” in transdisciplinary research.
Denis Ouédraogo
Dr Dénis Ouédraogo holds a Doctorate in Environmental Economics and Sustainable Development. University of Ouagadougou, Burkina Faso. He is Senior Lecturer at the Institute for Rural Development at University Nazi BONI in Burkina Faso. He has been a Graduate Fellow a Research Institute (ILRI) for three years and the Managing Director of the National Agricultural Training school of Burkina Faso for six years. His research areas span from agricultural productivity, Households Economy Analysis, food security to Environmental impact analysis.
References
- Adesina, A. (1996). Factors affecting the adoption of fertilizers by rice farmers in cote d'Ivoire. Nutrient Cycling in Agroecosystems, 46(1), 29–39. https://doi.org/10.1007/BF00210222
- Amekawa, Y. (2013). Understanding the local reality of the adoption of sustainable practices and farmer livelihoods: The case of pummelo farming in Chaiyaphum, Northeast Thailand. Food Security, 5(6), 793–805. https://doi.org/10.1007/s12571-013-0301-9
- Anley, Y., Bogale, A., & Haile-Gabriel, A. (2007). Adoption decision and use intensity of soil and water conservation measures by smallholder subsistence farmers in Dedo District, Western Ethiopia. Land Degradation & Development, 18(3), 289–302. https://doi.org/10.1002/ldr.775
- Bationo, A., Hartemink, A., Lungu, O., Naimi, M., Okoth, P., Smaling, E., & Thiombiano, L. (2006). African soils: Their productivity and profitability of fertilizer use. In A,A,H,O,L,M,N Bationo, P. Okoth, E. Smaling, & L. Thiombiano (Eds.), African ferilizer summit. 9-13 June 2006.
- Bationo, A., Lompo, F., & Koala, S. (1998). Research on nutrient flows and balances in West Africa: State-of-the-art1Paper contributes to EC INCO-DC project IC18-CT96-0092 (spatial and temporal variation of soil nutrient stocks and management in sub-Saharan African farming systems).1. Agriculture, Ecosystems & Environment, 71(1-3), 19–35. https://doi.org/10.1016/S0167-8809(98)00129-7
- Bidogeza, J. C., Berentsen, P. B. M., Graaff, j., & Oude lansink, A. G. J. M. (2009). A typology of farm households for the Umutara Province in Rwanda. Food Security, 1(3), 321–335. https://doi.org/10.1007/s12571-009-0029-8
- Chianu, J. N., Chianu, J. N., & Mairura, F. (2012b). Organic fertilizers in Sub-saharan farming systems. In E. Lichtfouse (Ed.), Organic fertilisation, soil quality and human health (pp. 31–65). Springer Netherlands.
- Chianu, J. N., Chianu, J. N., & Mairura, F. (2012). Mineral fertilizers in the farming systems of sub-Saharan Africa. A review. Agronomy for Sustainable Development, 32(2), 545–566. https://doi.org/10.1007/s13593-011-0050-0
- Chianu, J. N., & Tsujii, H. (2005). Determinants of farmers? Decision to adopt or not adopt inorganic fertilizer in the savannas of northern Nigeria. Nutrient Cycling in Agroecosystems, 70(3), 293–301. https://doi.org/10.1007/s10705-005-0715-7
- CILSS. (2012). Bonnes pratiques agro-sylvo-pastorales d’amélioration durable de la fertilité des sols au Burkina Faso Ouagadougou, Burkina Faso.
- Cobo, J. G., Dercon, g., & Cadisch, G. (2010). Nutrient balances in African land use systems across different spatial scales: A review of approaches, challenges and progress. Agriculture, Ecosystems & Environment, 136(1-2), 1–15. https://doi.org/10.1016/j.agee.2009.11.006
- Craswell, E. T., & Lefroy, R. D. B. (2001). The role and function of organic matter in tropical soils. Nutrient Cycling in Agroecosystems, 61(1/2), 7–18. https://doi.org/10.1023/A:1013656024633
- Defries, R. S., Rudel, T., Uriarte, M., & Hansen, M. (2010). Deforestation driven by urban population growth and agricultural trade in the twenty-first century. Nature Geoscience, 3(3), 178–181. https://doi.org/10.1038/ngeo756
- De Sherbinin, A., Vanwey, L. K., Mcsweeney, K., Aggarwal, R., Barbieri, A., Henry, S., Hunter, l. M., Twine, W., & Walker, R. (2008). Rural household demographics, livelihoods and the environment. Global Environmental Change, 18(1), 38–53. https://doi.org/10.1016/j.gloenvcha.2007.05.005
- Devereux, S. (2009). Why does famine persist in Africa? Food Security, 1(1), 25–35. https://doi.org/10.1007/s12571-008-0005-8
- Devereux, S., & Maxwell, S. (2001). Food security in Sub-saharan Africa London. ITDG Publishing.
- DFID. (1999). Sustainable livelihoods guidance sheets [Online]. Available: http://www.efls.ca/webresources/DFID_Sustainable_livelihoods_guidance_sheet.pdf [Accessed 15 February 2014].
- Ding, X., Han, X., Liang, Y., Qiao, Y., Li, L., & Li, N. (2012). Changes in soil organic carbon pools after 10 years of continuous manuring combined with chemical fertilizer in a Mollisol in China. Soil and Tillage Research, 122, 36–41. https://doi.org/10.1016/j.still.2012.02.002
- DREP/sud-ouest & PNGT. (2000). Monographie de la Province du Ioba. Gaoua, Burkina Faso: Direction Regionale de l'Economie et de la Planification du Sud-Ouest.
- Dzanku, F. M., Jirström, M., & Marstorp, H. (2015). Yield Gap-based poverty gaps in rural Sub-saharan Africa. World Development, 67, 336–362. https://doi.org/10.1016/j.worlddev.2014.10.030
- Enyong, L. A., Debrah, S. K., & Bationo, A. (1999). Farmers’ perceptions and attitudes towards introduced soil-fertility enhancing technologies in western Africa. Nutrient Cycling in Agroecosystems, 53(2), 177–187. https://doi.org/10.1023/A:1009745225465
- FAO. (1993). Integrated plant nutrition systems. In R. N. D. R. A. R. (Ed.), FAO fertilizer and plant nutrition bulletin.
- FAO. (2006). Food security and agricultural development in Sub-Saharan Africa: Building a case for more public support. Working paper Rome, Italy.
- FAO, IFAD, UNICEF, WFP & WHO. (2020). The state of food security and nutrition in the world 2020. Transforming food systems for affordable healthy diets. FAO, IFAD, UNICEF, WFP and WHO.
- FAO, IFAD & WFP. (2013). The state of food insecurity in the world 2013. The multiple dimensions of food security. Food and Agriculture Organization of the United Nations.
- Freeman, H. A., & Omiti, J. M. (2003). Fertilizer use in semi-arid areas of Kenya: Analysis of smallholder farmers’ adoption behavior under liberalized markets. Nutrient Cycling in Agroecosystems, 66(1), 23–31. https://doi.org/10.1023/A:1023355011400
- Gaur, C., & Singh, G. (1993). Organic and biological plant nutrient sources: Potential, methods for reducing the bulk and improving the availability of nutrients. In R. N. Roy (Ed.), Integrated plant nutrition (pp. 85–112). FAO.
- Gleisberg-gerber, K. (2012). Livelihoods and land management in the Ioba Province in south-western Burkina Faso.
- Grabowski, P. P., & Kerr, J. M. (2014). Resource constraints and partial adoption of conservation agriculture by hand-hoe farmers in Mozambique. International Journal of Agricultural Sustainability, 12(1), 37–53. https://doi.org/10.1080/14735903.2013.782703
- Greene, W. H. (2012). Econometric analysis. Prentice Hall.
- Grote, U. (2014). Can we improve global food security? A socio-economic and political perspective. Food Security, 6(2), 187–200. https://doi.org/10.1007/s12571-013-0321-5
- Hosmer, D. W., Jr., & Lemeshow, S. (2000). Applied logistic regression (2nd ed.). John Wiley and Sons, Inc. http://dx.doi.org/10.1002/0471722146
- Ingram, J. S. I., Gregory, P. J., & Izac, A. M. (2008). The role of agronomic research in climate change and food security policy. Agriculture, Ecosystems & Environment, 126(1-2), 4–12. https://doi.org/10.1016/j.agee.2008.01.009
- INSD. (2009a). Monographie de la région du Sud-ouest. In INSD (Ed.), Ougadougou (pp. 152). Burkina Faso: INSD.
- INSD. (2009b). Projections démographiques de 2007 à 2020 par région et province. In National de la statistique et de la démographie (INSD) (pp. 73). INSD.
- INSD. (2013). Annuaire statistique 2011. 2013 ed.
- Jones, S. (2002). A framework for understanding On-farm environmental degradation and constraints to the adoption of soil conservation measures: Case studies from highland Tanzania and Thailand. World Development, 30(9), 1607–1620. https://doi.org/10.1016/S0305-750X(02)00056-6
- Kassie, M., Jaleta, M., Shiferaw, B., Mmbando, F., & Mekuria, M. (2013). Adoption of interrelated sustainable agricultural practices in smallholder systems: Evidence from rural Tanzania. Technological Forecasting and Social Change, 80(3), 525–540. https://doi.org/10.1016/j.techfore.2012.08.007
- Kearney, S., Fonte, S. J., Salomon, A., Six, J., & Scow, K. M. (2012). Forty percent revenue increase by combining organic and mineral nutrient amendments in Ugandan smallholder market vegetable production. Agronomy for Sustainable Development, 32(4), 831–839. https://doi.org/10.1007/s13593-012-0097-6
- Ketema, M., & Bauer, S. (2011). Determinants of manure and fertilizer applications in eastern highlands of Ethiopia. Quarterly Journal of International Agriculture, 50, 237–252. https://doi.org/10.22004/ag.econ.155533
- Kismányoky, T., & Tóth, Z. (2013). Effect of mineral and organic fertilization on soil organic carbon content as well as on grain production of cereals in the IOSDV (ILTE) long-term field experiment, keszthely, Hungary. Archives of Agronomy and Soil Science, 59(8), 1121–1131. https://doi.org/10.1080/03650340.2012.712208
- Körschens, M., Albert, E., Armbruster, M., Barkusky, D., Baumecker, M., Behle-schalk, L., Bischoff, R., Čergan, Z., Ellmer, F., Herbst, F., Hoffmann, S., Hofmann, B., Kismanyoky, T., Kubat, J., Kunzova, E., Lopez-fando, C., Merbach, I., Merbach, W., Pardor, M. T., … Zorn, W. (2012). Effect of mineral and organic fertilization on crop yield, nitrogen uptake, carbon and nitrogen balances, as well as soil organic carbon content and dynamics: Results from 20 European long-term field experiments of the twenty-first century. Archives of Agronomy and Soil Science, 59, 1017–1040. http://dx.doi.org/10.1080/03650340.2012.704548
- Kristjanson, P., Okike, I., Tarawali, S., Singh, B. B., & Manyong, V. M. (2005). Farmers' perceptions of benefits and factors affecting the adoption of improved dual-purpose cowpea in the dry savannas of Nigeria. Agricultural Economics, 32(2), 195–210. https://doi.org/10.1111/j.0169-5150.2005.00338.x
- LAL, R. (1995). Erosion-crop productivity relationships for soils of Africa. Soil Science Society of America Journal, 59(3), 661–667. https://doi.org/10.2136/sssaj1995.03615995005900030004x
- LAL, R. (2009). Soil degradation as a reason for inadequate human nutrition. Food Security, 1(1), 45–57. https://doi.org/10.1007/s12571-009-0009-z
- Lambrecht, I., Vanlauwe, B., Merckx, R., & Maertens, M. (2014). Understanding the process of agricultural technology adoption: Mineral fertilizer in eastern DR Congo. World Development, 59, 132–146. https://doi.org/10.1016/j.worlddev.2014.01.024
- Le, Q. B. (2005). Multi-agent system for simulation of land-use and land cover change: A theoretical framework and its first implementation for an upland watershed in the Central Coast of Vietnam. In L. G. Vlek, P. Denich, M. Martius, C. Rodgers, & N. V. D. Giesen (Eds.), Bonn (pp. 283).
- MAHRH. (2010). Suivi de la campagne agricole 2010-2011 [Online]. Ouagadougou, Burkina Faso: Premier minister. Accessed 9 July 2014, from http://www.gouvernement.gov.bf/spip.php?page=impression&id_article=507
- Mahrh, & GTZ (2004). Mission d'appui à l'identification des interventions du Programme de Developement de l'Agriculture de la coopération germano-burkinabè dans la région administrative du Sud-Ouest. Ouagadougougou.
- Makokha, S., Kimani, S., Mwangi, W., Verkuijl, H., & Musembi, F. (2001). Determinants of fertilizer and manure Use for maize production in kiambu district, Kenya. KARI.
- Marenya, P. P., & Barrett, C. B. (2007). Household-level determinants of adoption of improved natural resources management practices among smallholder farmers in western Kenya. Food Policy, 32(4), 515–536. https://doi.org/10.1016/j.foodpol.2006.10.002
- Martey, E., Wiredu, A. N., Etwire, P. M., Fosu, M., Buah, S. S. J., Bidzakin, J., Ahiabor, B. D. K., & Kusi, F. (2014). Fertilizer adoption and Use intensity Among smallholder farmers in northern Ghana: A case study of the AGRA soil health project. Sustainable Agriculture Research, 3, 36.
- Mkhabela, T. S., & Materechera, S. A. (2003). Factors influencing the utilization of cattle and chicken manure for soil fertility management by emergent farmers in the moist Midlands of KwaZulu-Natal Province, South Africa. Nutrient Cycling in Agroecosystems, 65(2), 151–162. https://doi.org/10.1023/A:1022156210667
- Mucheru-Muna, M., Mugendi, D., Kung’u, J., Mugwe, J., & Bationo, A. (2007). Effects of organic and mineral fertilizer inputs on maize yield and soil chemical properties in a maize cropping system in Meru South District. Kenya, 69, 189–197. https://doi.org/10.1007/s10457-006-9027-4
- Murungweni, C., Van wijk, M. T., Giller, K. E., Andersson, J. A., & Smaling, E. M. A. (2014). Adaptive livelihood strategies employed by farmers to close the food gap in semi-arid south eastern Zimbabwe. Food Security, 6(3), 313–326. https://doi.org/10.1007/s12571-014-0348-2
- Neeteson, J. J. (1993). Combined use of inorganic and organic plant nutrients in a West European arable farming system. In R. Dudal, & R. N. Roy (Eds.), Integrated plant nutrition (pp. 391–396). FAO.
- Nkamleu, G. B. (2007). Modeling farmers’ decisions on integrated soil nutrient management in sub-Saharan Africa: A multinomial Logit analysis in Cameroon. In A. Bationo (Ed.), Advances in integrated soil fertility management in Sub-saharan Africa: Challenges and opportunities (pp. 891–903). Yaoundé: International Institute of Tropical Agriculture.
- Ojiem, J. O., De ridder, N., Vanlauwe, B., & Giller, K. E. (2006). Socio-ecological niche: A conceptual framework for integration of legumes in smallholder farming systems. International Journal of Agricultural Sustainability, 4(1), 79–93. https://doi.org/10.1080/14735903.2006.9686011
- Oumer, A. M., Hjortsø, C. N., & Neergaard, A. (2013). Understanding the relationship between livelihood strategy and soil management: Empirical insights from the central highlands of Ethiopia. Food Security, 5(2), 143–156. https://doi.org/10.1007/s12571-013-0242-3
- Palm, C. A., Gachengo, C. N., Delve, R. J., Cadisch, G., & Giller, K. E. (2001). Organic inputs for soil fertility management in tropical agroecosystems: Application of an organic resource database. Agriculture, Ecosystems & Environment, 83(1-2), 27–42. https://doi.org/10.1016/S0167-8809(00)00267-X
- Palm, C. A., Myers, R. J. K., & Nandwa, S. M. (1996). Prospects for integrated soil fertility management using organic and inorganic inputs: Evidence from smallholder African agricultural systems. In R. J. Buresh, & P. A. C. Sanchez (Eds.), 88th annual meetings of the American society of agronomy and the soil science society of America (pp. 193–218). Soil Science Society of America American Society of Agronomy.
- Parr, J. F., & Colacicco, D. (1987). Organic materials as alternative nutrient sources. In Z. R. Helsel (Ed.), Energy in plant nutrition and pest control (pp. 81–99). Elsevier Science Publishers B.V.
- Pimentel, D., & Burgess, M. (2013). Soil erosion threatens food production. Agriculture, 3(3), 443–463. https://doi.org/10.3390/agriculture3030443
- Place, F., Barrett, C. B., Freeman, H. A., Ramisch, J. J., & Vanlauwe, B. (2003). Prospects for integrated soil fertility management using organic and inorganic inputs: Evidence from smallholder African agricultural systems. Food Policy, 28(4), 365–378. https://doi.org/10.1016/j.foodpol.2003.08.009
- Reardon, T., & Vosti, S. A. (1995). Links between rural poverty and the environment in developing countries. Asset Categories and Investment Poverty World Development, 23, 1495–1506. https://doi.org/10.1016/0305-750X(95)00061-G
- Rodenburg, J., Büchi, L., Jeremy Haggar, J. (2021). Adoption by adaptation: Moving from conservation agriculture to conservation practices. International Journal of Agricultural Sustainability, 19(5-6), 437–455. https://doi.org/10.1080/14735903.2020.1785734
- Sanni, A., & Doppler, W. (2007). Socio-economic determinants of household fertilizer Use intensity for maize-based production systems in the northern Guinea savannah of Nigeria. Journal of Applied Sciences, 7(13), 1774–1779. https://doi.org/10.3923/jas.2007.1774.1779
- Scherr, S. J. (2000). A downward spiral? Research evidence on the relationship between poverty and natural resource degradation. Food Policy, 25(4), 479–498. https://doi.org/10.1016/S0306-9192(00)00022-1
- Sconnes, I. (1998). Sustainable rural livelihoods. A framework for analysis. IDS Working Paper 72.
- Somda, J., Nianogo, A. J., Nassa, S., & Sanou, S. (2002). Soil fertility management and socio-economic factors in crop-livestock systems in Burkina Faso: A case study of composting technology. Ecological Economics, 43(2-3), 175–183. https://doi.org/10.1016/S0921-8009(02)00208-2
- SPCPSA. (2013). Politique nationale de sécurité alimentaire et nutritionnelle.
- STOCKING, M. A. (2003). Tropical soils and food security: The next 50 years. Science, 302(5649), 1356–1359. https://doi.org/10.1126/science.1088579
- Stoorvogel, J., & Smaling, E. (1990). Assessment of soil nutrient depletion in Sub-Saharan Africa, 1983-2000. Wageningen Winand Staring Centre for Integrated Land, Soil and Water Research (SC-DLO).
- Tittonell, P., Muriuki, A., Shepherd, K. D., Mugendi, D., Kaizzi, K. C., Okeyo, J., Verchot, L., Coe, R., & Vanlauwe, B. (2010). The diversity of rural livelihoods and their influence on soil fertility in agricultural systems of east Africa – A typology of smallholder farms. Agricultural Systems, 103(2), 83–97. https://doi.org/10.1016/j.agsy.2009.10.001
- Tittonell, P., Vanlauwe, B., Leffelaar, P. A., Rowe, E. C., & Giller, K. E. (2005a). Exploring diversity in soil fertility management of smallholder farms in western Kenya. Agriculture, Ecosystems & Environment, 110(3-4), 149–165. https://doi.org/10.1016/j.agee.2005.04.001
- Tittonell, P., Vanlauwe, B., Leffelaar, P. A., Shepherd, K. D., & Giller, K. E. (2005b). Exploring diversity in soil fertility management of smallholder farms in western Kenya. Agriculture, Ecosystems & Environment, 110(3-4), 166–184. https://doi.org/10.1016/j.agee.2005.04.003
- Van Beek, C. L., Elias, E., Yihenew, G. S., Heesmans, H., Tsegaye, A., Feyisa, H., Tolla, M., Melmuye, M., Gebremeskel, Y., & Mengist, S. (2016). Soil nutrient balances under diverse agro-ecological settings in Ethiopia. Nutrient Cycling in Agroecosystems, 106(3), 257–274. https://doi.org/10.1007/s10705-016-9803-0
- Vanlauwe, B., & Giller, K. (2006). Popular myths around soil fertility management in sub-saharan Africa. Agriculture, Ecosystems & Environment, 116(1-2), 34–46. https://doi.org/10.1016/j.agee.2006.03.016
- Vlek, P. L. G., Kühne, R. F., & Denich, M. (1997). Nutrient resources for crop production in the tropics. Philosophical Transactions of the Royal Society of London. Series B: Biological Sciences, 352(1356), 975–985. https://doi.org/10.1098/rstb.1997.0076
- Vu, L. E. Q. B., & Frossard, E. (2014). Socio economic determinant of fertilizer use in Vietnam. Sumitted.
- Vu, Q. M., Le, Q. B., Frossard, E., & Vlek, P. L. G. (2014). Socio-economic and biophysical determinants of land degradation in Vietnam: An integrated causal analysis at the national level. Land Use Policy, 36, 605–617. https://doi.org/10.1016/j.landusepol.2013.10.012
- Waithaka, M., Thornton, P., Shepherd, K., & Ndiwa, N. (2007). Factors affecting the use of fertilizers and manure by smallholders: The case of Vihiga, western Kenya. Nutrient Cycling in Agroecosystems, 78(3), 211–224. https://doi.org/10.1007/s10705-006-9087-x
- Yilma, T., & Berger, T. (2006). Complementarity between Irrigation and Fertilizer Technologies-A justification for increased Irrigation Investment in the Less-Favored Areas of SSA International Association of Agricultural Economists Conference. Gold Coast, Australia, August 12-18, 2006.