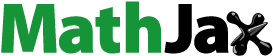
ABSTRACT
Balanced and coordinated allocation of production factors is crucial for promoting the apple industry. We constructed a cost-ecological 21-indicator system for apple production in China based on labour cost, opportunity cost, fertilizer machinery input, natural resource, meteorological factor, ecological pressure and topography. The Hurst Index was used to analyse spatial changes in apple production in 617 counties of six provinces from 1999 to 2019. Grey correlation and TOPSIS models were adopted for optimal region selection and efficiency improvement. The areas supporting significant and sustained growth in apple production were concentrated in the eastern edge of Gansu, the northern, central and southern parts of Shaanxi, the western and central parts of in Henan, the northern, central and southern parts of Shanxi, the northern, central and southern parts of Hebei and the western, central and eastern parts of Shandong, covering 197 counties. Fertilizer machinery input was the key factor to improving apple production efficiency in Hebei, Shandong, Shanxi and Henan. The optimal regions were distributed in northern and southern Hebei, eastern and western Shandong, central and southern Shanxi, western Henan, central and northern Shaanxi and northeastern and southern Gansu. Compared with Shandong, the optimal factor allocation in Shaanxi is particularly favourable for optimal region selection, and the development momentum is remarkable despite a small county scale. Apple production has expanded westwards and northwards both between and within provinces over the past 21 years.
Highlights
Constructing a cost-ecological indicator system can achieve balanced and coordinated distribution of factors
We measured the optimal area for efficiency improvement of apple production.
Fertilizer machinery input is a prerequisite for apple production layout
Apple production has expanded westwards and northwards both between and within provinces.
1. Introduction
Food is essential for human survival, and food security based on quality is a prerequisite. Apples are one of the most popular fruits produced worldwide. The global apple industry is largely dependent on apple cultivation and consumption in China. Both the planting area and output of apples in China account for more than 40% of the global total. From 1978 to 2019, 21.86% of the increase in China’s fruit production was attributed to apples (China Rural Statistical Yearbook, Citation1979–Citation2020). Apple production has made substantial contributions to promoting the transformation of agricultural structure, upgrading the apple industry, and increasing farmers’ income. With increasing living stands of urban and rural residents, the consumption structure of apples has changed from quantitative consumption to quality consumption.
Due to factors such as climate change, technological advances, business scale and management levels, there is a growing contradiction between ‘small farmers’ and ‘big markets’ for apple production. Additionally, the increase in apple planting area and output exacerbates the imbalance between supply and demand, making selling difficult. Currently, there are still significant challenges in increasing apple production while ensuring apple quality and security. For example, intensive exploitation of non-renewable resources may lead to land degradation, resulting in reduced soil productivity (Cláudia et al., Citation2022). Increased extreme climate events (e.g. droughts, floods) and frequent pest and disease outbreaks associated with climate change are likely to cause crop failure or destruction (Silva et al., Citation2023; Congedo & Macchi, Citation2015). Population growth is expected to increase nutritional insecurity (Hall et al., Citation2017; United Nations, Citation2021). Variation in apple production patterns can increase the demand for land and water, exhausting both resources and increasing uncertainty regarding apple quality and security (Krupnik et al., Citation2021).
Growing apple trees is an effective strategy for maintaining and increasing apple harvests. In recent years, China’s central government has paid increasing attention to adjusting the apple industrial development plan and policy orientation. Following the ‘Apple Advantage Regional Development Plan (Citation2003–Citation2007)’, the Ministry of Agriculture formulated the ‘Apple Advantage Regional Layout Plan (Citation2008–2015)’ to promote apple cultivation in advantageous regions. Accordingly, Bohai Rim and the Loess Plateau are identified as two major apple production areas. The idea of ‘stabilising areas, improving quality, expanding exports, and increasing benefits’ is put forward to establish a modern apple cultivation technology system, improving standardized production levels and increasing the national apple production. Increased competitiveness of the industrial market may increase farmers’ income and economic development in advantageous areas. The regional layout plan proposes to speed up implementation of the apple strategic project, enhance the establishment of advantageous and suitable areas for apples, and accelerate implementation of the apple production capacity construction plan to meet the output target. Based on adapting to changes in circumstances, resource endowments, productivity levels and industrial development advantages, it is imperative to strengthen implementation of the regional layout plan for increased apple production.
Previous research on the distribution of apple production areas in China has focused on the evolution of apple production layout and its driving factors. The research methods are mainly based on econometric models, including conventional and dynamic panel data, as well as spatial Doberman, spatial lag panel and spatial error panel models (Zhang et al., Citation2018; Yuan et al., Citation2017; Wang & Shi, Citation2017; Bai et al., Citation2015; Liu & Fan, Citation2012). The motives behind the evolution of apple production layout are demonstrated, such as natural conditions, economic factors, comparative benefits, technical conditions, market factors, production costs and national policies. Despite outstanding research achievements for agricultural planting layout, some areas still need to be further explored for apple production layout, given the unbalanced distribution and development of apple-planting areas.
Apple trees are dryland crop plants. The ecological environment in arid and semi-arid areas is fragile and sensitive, with low potential for recovery. There are distinct resources and habitats, uneven distribution of water, and zonal distribution of soil and vegetation, so the production and consumption factors do not match in these areas (Ni et al., Citation2021; Zhang et al., Citation2021b; Sandile et al., Citation2020). With the intensification of climate change and human activities, the arid and semi-arid areas may encounter environmental challenges, such as desertification, rising temperatures, water scarcity, ecological degradation, pollution, and natural disasters (Tautges et al., Citation2022; Malmir et al., Citation2021; Kharanagh et al., Citation2020). Furthermore, these challenges may have socio-economic and ecological impacts on the man–land coupling system. The sustained stability of apple production is a basic precondition for rapid economic development, an important driver for social stability and harmony, and a strategic foundation for national security. However, the areas suitable for apple production show imbalances in input-output distribution due to regional differences in resource endowments, geographical location, economic foundation, technological progress, agricultural infrastructure and government behaviour and policies (Souza & Gomes, Citation2023). Therefore, a balanced and coordinated distribution of cost-effective factors is crucial for promoting apple production.
Optimizing region selection and improving apple quality and production efficiency must occur to allow the apple industry to expand in China. Stabilizing apple production needs to be a long-term strategic goal. Based on the ecological background in the post-pandemic period, we construct a cost-ecological indicator system to evaluate the region selection and efficiency improvement of apple production. The findings provide reference data that could be utilized by the government to formulate regional development policies. In the post-pandemic era, the layout of apple production should be more ecological to ensure sustainable development of the apple industry in China.
In this paper, we divide different areas in 31 provinces (municipalities and autonomous regions, hereafter referred to as provinces) of mainland China ((a)) based on their suitability for apple production (Wang & Liu, Citation2022): advantageous, suitable and unsuitable areas ((a)). Taking into account method selection and data availability, coherence and calibre consistency, we chose China's north–south and east–west orientations as sequential criteria and divide 617 counties (prefecture-level cities, county-level cities and districts, hereafter referred to as counties) into six provinces: Hebei, Shandong, Shanxi, Henan, Shaanxi and Gansu ((c)). These six provinces, as well as the Bohai Rim and Loess Plateau, are divided into the four major production areas ((b)) following the apple zoning plan of China's modern apple industry system (Zhang et al., Citation2018; Liu & Fan, Citation2012). The modern apple industry system divides apple production areas into the ‘Key Counties and Cities of Apple's Advantage Regional Layout 2017 − 2025’ using seven optimal ecological indicators: average annual temperature, average annual precipitation, average mid-January temperature, annual extreme low temperature, average summer temperature, number of >35°C days, and average summer minimum temperature. The plan involves 143 counties in seven provinces: Liaoning, Hebei, Shandong, Shanxi, Henan, Shaanxi and Gansu ((c)). Because it is difficult to obtain data from Liaoning, this study further confirms the superiority of 126 counties in six provinces based on the adaptability theory.
2. Theoretical analysis and research hypothesis
2.1. Identifying countermeasures for increasing apple output
Regional economic development is mainly driven by primary, secondary and tertiary industries. In a large agricultural country like China, apples are a major agricultural product, providing income for farmers at regional scales. Factor allocation refers to the allocation and combination of agricultural production factors such as capital, labour, land, technology, fertilizer and machinery in the process of production by apple growers as rational economists. Since reasonable factor allocation will greatly improve apple production efficiency, farmers’ income and output, the feasibility of region selection by apple growers in the post-pandemic context must be investigated from a factor allocation perspective. Regional governments should be guided to reasonably formulate and use factors of production, thereby avoiding inefficiencies caused by insufficient inputs of factors and excessive production costs due to excessive inputs of factors. Reasonable and effective factor allocation can enable efficient production of apples.
The strategic response to increasing apple output includes two ‘changes’: change in production practices and change in productive financial management. The first change involves diversifying crop cultivars, altering agricultural production time, increasing fertilizer and pesticide inputs, adopting new technologies and modifying irrigation methods; the second change involves diversifying sources of income and purchasing agricultural insurance (Chemeris et al., Citation2022). Unlike annual grain crops, apples are a perennial fruit crop with high-value agricultural products. Apples have a high degree of commercialization and marketization; their production and operation processes are characterized by marketization, specialization and strong asset specificity. As a consequence, some of the strategies, such as diversifying crop cultivars, changing agricultural production time and purchasing agricultural insurance, are no longer applicable. Compared with other crops, apples show essential differences in terms of input-output processes, technical nature, sensitivity to external conditions and responses to climate change.
Based on the above theoretical analysis and judgment, research hypothesis 1 was proposed: optimal factor allocation supports highly efficient regional apple production.
2.2. Analysing impact of apple production cost
Total production cost includes accounting costs (explicit costs) and economic costs (the sum of explicit and implicit costs – the comprehensive embodiment of accounting costs and implicit costs). Apple production cost comprises direct cash expenditure, operator self-employment costs and the combined cost of own machinery and equipment (Mousavi-Avval et al., Citation2011). Presently, the most authoritative cost-benefit accounting data for agricultural products in China come from the National Compilation of Cost-benefit Data of Agricultural Products (issued by the National Development and Reform Commission, Citation2023). The data encompass cost-benefit structure information such as material and service costs, indirect costs, labour costs, land costs, outputs and output values. According to neoclassical economics, apple growers’ decision-making is the result of behavioural selection in pursuit of maximizing expected utility under uncertain conditions. Natural conditions, including climate, topography, natural resources and ecological pressure are the main external risks faced in regional agricultural production (Estrada-Carmona et al., Citation2020), which can be defined as eco-environmental vulnerability. Region selection based on the optimal factor set can maximize the expected utility under natural conditions and help adjust factors of production under both natural and anthropogenic constraints.
While natural conditions vary across regions, the main impact path of eco-environmental vulnerability on apple production is consistent with regard to production inputs, mainly input factors. For example, high temperature accelerates the release and utilization of soil fertilizers in orchards, resulting in a shortened nutrient release cycle (Lv et al., Citation2019). Apple growers increase the amount and frequency of fertilization inputs to improve fertilizer use efficiency and increase apple production. In this way, an increase in production inputs is likely to increase the cost input of apple production. The impact on production cost is characterized by periodic differences because apples grow in six key stages – germination (March), flowering (April), early fruit (May), bud differentiation (June), fruit expansion (July-August) and fruit colouring (September) – in spring, summer and autumn. Based on cost structure classification by the Compilation of Cost-benefit Information for Agricultural Products in China, we took into account apple production and regional characteristics to divide the production cost of apples as follows: material and service cost, labour cost, and opportunity cost ().
Table 1. Construction of an indicator system for selection of apple production areas in China.
Based on the above theoretical analysis and judgment, research hypothesis 2 was proposed: eco-environmental vulnerability plays a significant role in regulating the impact of cost input on regional apple production.
Hypothesis 2a: resource endowment positively regulates the impact of cost input on regional apple production.
Hypothesis 2b: extreme climate, ecological pressures and topography negatively regulate the impact of cost input on regional apple production.
3. Research and design
3.1. Data sources and indicator system construction
3.1.1. Data sources and processing
The data on labour cost, opportunity cost, input of chemical fertilizer machinery, resource endowment and ecological pressure (A15 and A17) of apple production were obtained from the China Rural Statistical Yearbook (2001–2020), China County Statistical Yearbook (2001–2020) and Municipal Statistical Yearbook (2001–2020). Meteorological data, which covered 699 basic meteorological stations, were derived from the daily dataset of China's surface climate data (1999–2019) provided by the China Meteorological Data Network (https://data.cma.cn). Mean value replacement was adopted to interpolate the missing daily meteorological data of individual stations in each year and calculate the monthly datasets of various meteorological factors at 699 stations. Since January–February and October are respectively the dormant and fruit ripening stages, these three months have minimal impact on apple growth. To accurately reflect factor contribution to apple growth, we selected meteorological data (A10–A14) from March to September for mathematical calculations.
The inverse distance weight interpolation module in ArcGIS v10.4 (Environment System Research Institute Inc., Redlands, CA, USA) was used to obtain meteorological raster image data in China. A total of 617 county-level meteorological datasets were obtained by splitting the administrative boundaries of each county in six provinces. The standardized precipitation evapotranspiration index (SPEI) was calculated using the monthly datasets of precipitation and temperature (Wan & Wang, Citation2018). ASTER DEM V3 was obtained from a 30 m × 30 m classification product released by the Geographical Information Monitoring Cloud Platform (https://www.dsac.cn), and was embedded after gridding. The topographic data (A19–A21) of 617 counties were obtained and their administrative boundaries in six provinces were divided. In addition to topographic and geomorphologic datasets (617 counties), vector data (including China’s administrative boundaries and first-level rivers – Yellow River and Yangtze River) were collected from the Resource and Environmental Science Data Centre, Chinese Academy of Sciences (https://www.resdc.cn). The administrative boundary datasets included administrative divisions of provinces, cities, and counties in 2019.
3.1.2. Indicator system construction
From the Bohai Rim to the Loess Plateau ((b)), adoption of adaptive behaviour and average productive income of fruit farmers are decreasing (Feng et al., Citation2016). Thus, farmers use various adaptation strategies such as irrigation and fertilization, and their income shows uneven spatial distribution with remarkable inter-provincial differences. Moreover, there is a spatial autocorrelation between the adaptive behaviour and income of fruit farmers in these two advantageous areas. We must reinforce regional economic development in the apple industry in a timely manner by performing empirical analyses of natural and anthropogenic factors at the spatiotemporal scale. The eco-environmental adaptation of regional economic development is particularly important based on the international background in the post-pandemic era. The 617 counties within the six provinces around the Bohai Rim and the Loess Plateau are located in the arid and semi-arid climate zone, where the fragile eco-environment potentially limits the development of apple cultivation.
To construct the indicator system based on cost and eco-environmental vulnerability (), the cost of apple production depends on three factors: water and fertilizer, plant protection, and labour costs. However, apple production is a labour-intensive industry, and the motivation of farmers to choose apple planting is to obtain more actual profits on limited arable land. In economics, the resource is used to obtain a certain income while giving up another income – opportunity cost. Accordingly, we divided the cost of apple production into the following three second-level indicators: labour cost, opportunity cost, and fertilizer machinery input (including water, fertilizer and plant protection). In the three-level indicator system, labour cost comprises all the costs incurred by the input of labour factors in apple production and operation, as reflected in wage remuneration and welfare supply (A1, A2). Opportunity cost is another income that farmers have to give up after adopting apple cultivation on limited cultivated land (A3, A4). Fertilizer machinery input refers the technological input of water, fertilizer and plant protection in apple production (A5–A7).
When evaluating eco-environmental vulnerability, many studies mainly looked at the impact of both natural and human factors (He et al., Citation2018; Zhang et al., Citation2018), and other studies analysed the causes and consequences of ecological fragility (Hu, Ma, Huang, & Guo, Citation2021; Mafi-Gholami, Pirasteh, Ellison, & Jaafari, Citation2021). Based on these two research perspectives, we selected natural resources and meteorological factors as natural factors for the second-level indicators of eco-environmental vulnerability. Ecological pressure and topography were used to represent human factors and the causes and consequences of ecological vulnerability. Specifically, land and water resources are the prerequisites for regional apple production, that is, resource endowment (A8, A9) (Sun et al., Citation2022; Gao et al., Citation2021; Liao et al., Citation2021; Huo et al., Citation2021; Zhang et al., Citation2021a). Meteorological factors include precipitation, temperature, relative humidity, wind speed and sunshine duration (A10–A14). Drought – usually manifested in land desertification (A16) – indicates the combined effects of all climatic factors in a habitat and is the main factor limiting apple production. Drought is often described using SPEI, which detects a higher drought frequency by difference between the normalized precipitation and evapotranspiration (Wan & Wang, Citation2018). Among the available drought index methods, SPEI has a better applicability for the time span and regional heterogeneity (Wan & Wang, Citation2018; Ma et al., Citation2020; Li et al., Citation2021). Moreover, high technical and capital investment improves agricultural production efficiency and farmers’ income during rapid urbanization (A18). However, urbanization has also introduced serious problems for agricultural production, especially in the conversion of cultivated land (A17) due to urban expansion driven by population concentration to urban area (A15) (Li & Li, Citation2019). Topographic factors (A19–A21) that affect apple production were selected based on the natural geographical attributes of China.
When calculating the three-level indicators, the data are not available readily for individual indicators. In the case of A3, we adopted similar indicator substitution using the data from two major apple production areas – Shandong and Shaanxi. The total agricultural output value of each county, rather than farmers’ income from arable land, was assessed. For A18, the concept of urbanization was used: first, the proportion of rural population was calculated from the population of each county in the two major production areas with better data; then, the urbanization rate was calculated as one minus the proportion of rural population.
3.2. Model setting
3.2.1. Hurst index
The optimal selection of apple production areas necessitates capturing the evolutionary trends of apple production in time and space. The Hurst index is a widely used non-parametric method, which was proposed by Hurst in 1951 to evaluate the reservoirs along the Nile River in Egypt (Hurst, Citation1951). Among several methods for calculating the Hurst index (Granero et al., Citation2008; Kendziorski et al., Citation1999; Tong et al., Citation2018), rescaled (R/S) interval analysis can provide the variation point and continuity of apple production timing. The calculation steps of the R/S principle are as follows.
Mean:
(1)
(1) Cumulative deviation:
(2)
(2) Range:
(3)
(3) Standard deviation:
(4)
(4) As
increases, we can get different rescale ranges of
, which should satisfy the following expressions:
(5)
(5) where H is the Hurst index (range: 0–1). H = 0.5 means that apple production will change randomly in the future. 0.5 < H < 1 indicates a positive continuous change in apple production; that is, the future increase in apple production will be consistent with the past trend. The closer the H value is to 1, the stronger the positive continuity. 1 < H < 0.5 indicates discontinuous changes in future apple production; that is, the future development trend of apple production will be opposite to the past. The closer the H value is to 0, the stronger the reverse sustainability of apple production in the future.
3.2.2. Grey correlation model
The grey correlation model evaluates the grey correlation between sequences based on the similarity between the reference sequence and multiple comparison sequences (Wang et al., 2020; He et al., Citation2016). Using the grey correlation model, we calculated the impact degree for each indicator of cost and eco-environment vulnerability on apple production, which allowed to determine the optimal factor allocation for regional apple production. The higher the grey correlation degree between an individual indicator and other indicators, the greater the weight of that indicator in the entire system (Liu et al., Citation2019).
Based on the cost-ecological indicator system constructed in this study, the grey correlation model was used to calculate the weights of third-level and second-level indicators. The top three major factors in terms of weight were extracted. The specific steps are as follows.
Standardize data. In the indicator system, the dimensions of each indicator are different. To eliminate the influence of the indicator dimensions, the maximum – minimum method is used to standardize the data as follows.
(6)
(6) where
is the number of samples and
is the number of indicators.
Calculate grey correlation degree. Taking
as a reference sequence, calculate the grey correlation degree
any
and
, and calculate the grey correlation degree
between
and other index vectors. The calculation formula is as follows:
(7)
(7)
(8)
(8) where
,
and
are the
indicator vector and
indicator vector, respectively,
is the resolution coefficient, and
generally takes the value (0, 1). When
, the resolution is the best, usually
(Wang et al., 2020).
Calculate indicator weights.
(9)
(9)
(10)
(10) where
is the weight of the
-th three-level indicator,
is the weight of the
-th second-level indicator, and
is the number of second-level indicators.
According to their weights, the top three second-level indicators were selected as the major factors and the proportion of all indicators in the selected factors were calculated. Among the three factors (,
is taken as an example to calculate the weights of all three-level indicators. The grey incidence matrix of
is calculated according to the grey correlation model as
, and the weights of three-level indicators in
are calculated according to the grey incidence matrix
as follows.
(11)
(11)
(12)
(12)
(13)
(13) where
is the number of three-level indicators in
,
is the number of all three-level indicators,
is the weight of the
indicator in the major factor
, and
is the correlation coefficient vector between
and
.
3.2.3 TOPSIS model
The TOPSIS method (Shi et al., Citation2017; Zhou et al., Citation2016) is a sequential optimization technique for the similarity of ideal targets. It uses a normalized data matrix to determine the relationship between the target and the ideal solution as well as the anti-ideal solution. The closeness of the target to the ideal solution is calculated, which is then used as the standard to evaluate the target's pros and cons. Therefore, based on TOPSIS closeness, apple production areas can be prioritized under optimal factor allocation. The steps for region selection based on TOPSIS closeness are as follows.
Standardize data. The calculation is given by formula (6).
Construct weight matrix. Indicator weights are calculated using the grey correlation model to construct the weight matrix
.
(14)
(14)
Determine the ideal solution and the anti-ideal solution.
is the positive ideal solution, and
is the negative ideal solution.
(15)
(15)
Determine the Euclidean distance.
is the Euclidean distance between the
area and the positive ideal solution, and
is the Euclidean distance between the
area and the negative ideal solution.
(16)
(16)
Determine the closeness
. The comprehensive closeness is calculated, according to which the priority area for apple production under optimal factor allocation is selected.
(17)
(17)
4. Results
4.1. Optimal allocation of regional factors
4.1.1. Spatial evolution of apple production based on the Hurst index
Depicting the spatiotemporal evolution of apple production is essential for interpreting the selection of apple production areas. Since the reform and opening of China, apple production has followed a continuous upward trend, with output and planting areas increasing by 7.4% and 2.6%, respectively (Wang & Liu, Citation2022). From a regional perspective, upward trends in apple output have been observed for Shandong, Shaanxi, Henan, Hebei, Shanxi and Gansu among 31 provinces over the past 42 years, with growth rates of 6.059%, 12.257%, 8.273%, 6.428%, 9.905% and 11.258%, respectively. The corresponding trends in planting areas show a transition from strong to weak for the six provinces, with growth rates of 0.726%, 6.135%, 0.665%, 1.97%, 3.362% and 5.932%, respectively (China Rural Statistical Yearbook, Citation1979–Citation2020). Regardless of the time or regional scale, the increase in apple output was substantially larger than that in apple planting area. Therefore, we selected the six provinces as the research area and used apple output to evaluate apple production.
Under conditions of equal classification, the counties with high apple outputs in 1999–2019 were concentrated in the central (Shaanxi) and eastern (Shandong) areas of the six provinces ((a)), with 17 counties having an output ≥30 × 107 kg. By province, there were more high-output counties in Shandong and Shaanxi, which are major apple production areas in China (Wang & Liu, Citation2022). Among the 123 counties in Shandong, only 17 had an apple output ≥10 × 107 kg, which was markedly less than 24 counties in Shaanxi. Especially among counties with an apple output ≥ 50 × 107 kg, only Luochuan (Yan’an City), Liquan and Chunhua (Hsienyang City) in Shaanxi achieved high quality development. By contrast, counties meeting the conditions also converged for Lingbao County and Sanmenxia City in Henan, as well as Linyi County and Yuncheng City in Shanxi. Overall, 558 of 617 counties in six provinces had an apple output <10 × 107 kg, including 106 counties in Shandong and 52 counties in Shaanxi which accounted for 86.179% and 68.421% of the total pixel area, respectively. Following reform and opening up, apple output in Shaanxi changed suddenly in 2008; its annual growth rate before 2008 was lower than that of Shandong, but has exceeded that of Shandong since then. Therefore, apple production in Shaanxi has the potential to catch up with that of Shandong year-on-year. Apple output in Hebei, Shanxi, Henan and Gansu was mostly <10 × 107 kg.
Figure 2. Spatial evolution of apple production (unit: 107 kg) and the Hurst index for each county in six provinces over three periods (1999–2019, 1999–2009, 2010–2019).

To explore the spatial evolution of apple production at the county level (1999–2019) and predict its future trends in the six provinces, we performed separate calculations by R/S analysis while ensuring the continuity and representativeness of data acquisition for 617 counties. The Hurst index for apple production in the six provinces was determined, with H = 0.5 being the cut-off for sustainability of growth in apple production. Since the smallest unit of the research area was county, we reclassified the H values into five categories ((a)) as described by Tong et al. (Citation2018). Overall, the six provinces continue to grow in apple production throughout the whole region, with the pixel area accounting for up to 93.8%. The current growth trend will not last in the future, and the areas predicted to decline in apple production are concentrated in southern Binzhou City, eastern Jinan City, southern Zibo City, eastern Tai'an City and northern Linyi in Shandong, as well as northern Yulin City, eastern Yan'an City, northwestern Weinan City in Shaanxi.
From west to east, a total of 197 counties with a significant and sustained growth in future apple production are concentrated in Gansu (7 in Qingyang City, 1 in Pingliang City, 2 in Longnan City), Shaanxi (2 in Yulin City, 6 in Yan'an City, 9 in Weinan City, 3 in Tongchuan City, 4 in Xi'an City, 3 in Hsienyang City, 6 in Baoji City, 6 in Shangluo City), Henan (3 in Sanmenxia City, Jiyuan City, 3 in Luoyang City, 8 in Nanyang City, 2 in Zhumadian City, 6 in Pingdingshan City, 1 in Luohe City, 3 in Xuchang City, 4 in Zhengzhou City and 2 in Zhoukou City), Shanxi (7 in Xinzhou City, 1 in Datong City, 3 in Shuozhou City, 3 in Lvliang City, 1 in Yangquan City, 3 in Jinzhong City, 7 in Yuncheng City, 9 in Linfen City, 3 in Jincheng City), Hebei (2 in Chengde City, 1 in Qinhuangdao City, 5 in Tangshan City, 3 in Baoding City, 7 in Shijiazhuang City, 9 in Xingtai City, 3 in Handan City, 6 in Hengshui City, 6 in Cangzhou City) and Shandong (2 in Dezhou City, 9 in Binzhou City, 3 in Jining City, 3 in Zaozhuang City, 1 in Zibo City, 7 in Weifang City, 6 in Linyi City, 4 in Rizhao City, 1 in Qingdao City).
To verify the accuracy of the predicted future trends in apple production, we divided the time scale into 1999–2009 and 2010–2019. Then, the 2010–2019 data were used to verify the 1999–2009 data, and the results confirmed the reliability of the analysis based on the Hurst index ((b,c)). Under the same level, it was more conducive to the visual analysis of apple output and H value with regard to spatial distribution. Therefore, the analysis results of both segmented datasets were consistent with those based on the 1999–2019 dataset ((a)). In 1999–2009, the apple output of six counties was ≥30 × 107 kg in Shandong (1 in Yantai City), Shaanxi (1 in Yuncheng City), Henan (1 in Sanmenxia City) and Shaanxi (3 in Hsienyang City; (b)). However, in 2010–2019, 24 counties had an apple output ≥30 × 107 kg in Shandong (1 in Weihai City, 1 in Zibo City, 5 in Jinan City), Shanxi (3 in Yuncheng City), Henan (1 in Shangqiu City, 2 in Sanmenxia City), Shaanxi (3 in Yan’an City, 2 in Weinan City, 5 in Hsienyang City) and Gansu (1 in Pingliang City; (c)). Compared with data for the two periods, 18 new counties were added.
In contrast with that in 1999–2019 ((a)), the spatial evolutionary trend of apple production in 1999–2009 was scattered, whereas the trend in 2010–2019 was concentrated ((b)). In 1999–2009, the number of counties with continuous growth in apple production in six provinces was slightly less than that in 1999–2019, with the pixel area accounting for 91.9%. The spatial evolutionary trend of apple production in the whole region was clear, with the addition of 75 counties in Gansu (2 in Jiuquan City, Jiayuguan City, 4 in Zhangye City, 3 in Wuwei City, 3 in Baiyin City, 4 in Lanzhou City, 1 in Linxia Hui Autonomous Prefecture, 5 in Dingxi City, 3 in Gannan Tibetan Autonomous Prefecture, 6 in Longnan City, 1 in Qingyang City, 6 in Tianshui City, 5 in Pingliang City), Shaanxi (3 in Hsienyang City, 1 in Shangluo City), Henan (2 in Luoyang City), Shanxi (3 in Changzhi City, 1 in Linfen City, 1 in Yuncheng City) and Shandong (2 in Weihai City, 10 in Yantai City, 4 in Qingdao City, 2 in Weifang City, 1 in Dongying City).
Compared with that in 1999–2009, the areas where apple production continued to grow in 2010–2019 showed ‘westward and northward migration’ between the six provinces or within provinces. Their spatial distribution was highly agglomerated, with a pixel area accounting for 67.5%. Based on of the data of 1999–2019 and 1999–2009, 86 counties were added in Shaanxi (7 in Yulin City, 1 in Yan’an City), Henan (2 in Sanmenxia City, 1 in Luoyang City, 2 in Nanyang City, 1 in Jiaozuo City, 5 in Shangqiu City, 6 in Zhoukou City, 1 in Kaifeng City, 1 in Xinxiang City), Shanxi (2 in Xinzhou City, 1 in Datong City, 2 in Lvliang City, 3 in Yuncheng City, 2 in Linfen City, 1 in Changzhi City), Hebei (3 in Chengde City, 5 in Tangshan City, 11 in Zhangjiakou City, 7 in Baoding City, 5 in Shijiazhuang City, 1 in Xingtai City, 1 in Hengshui City), and Shandong (2 in Weihai, 4 in Jining City, 9 in Heze City).
Based on the comprehensive third-phase data and the segmented data of two phases in the major apple production areas, the prediction results of apple production in the six provinces were highly consistent with the conclusions of the R/S principle. Combined with the Apple Division Plan of Key Counties and Cities with Apple's Advantageous Regional Layout 2017–2025 ((c)), all 126 counties in six provinces met the seven optimal ecological indicators, suggesting advantageous apple production. However, taking into account the evolutionary trends and changes of apple production revealed by our data analysis, the advantages of some counties with optimal ecological indicators were not fully captured. This means that the factors of apple production are allocated unevenly and the factor input and output are mismatched in China. It is crucial to solve the problem of optimal factor allocation in the optimal ecological areas. Therefore, we explored the input allocation of the optimal factors selected in apple production areas based on the adaptation conditions of apple production.
4.1.2. Selection of optimal factors for regional apple production
The weights of third-level indicators () and second-level indicators () in the past 21 years were calculated using the grey correlation model. The annual indicator correlation coefficient was >0.95, which shows that it is appropriate and feasible to select regions and improve the efficiency of apple production in China based on a cost-ecological perspective. Among the 21 third-level indicators, the weight fluctuations of A6, A9 and A7 were most prominent, followed by A5, all of which showed rapid changes with 2006 as the variation point. There were three peak changes: first rising in 1999–2001, 2002–2004 and 2005–2006, and then declining after 2001–2002, 2004–2005 and 2006–2007. The opposite fluctuation peaks were observed for A21, A19 and A2, followed by A18, which ranged from strong to weak. The weights of the other 13 third-level indicators were relatively steady and showed a slightly rising trend from 1999 to 2019.
Figure 3. Weights of third-level indicators for each year in 617 counties within six provinces from 1999–2019.

Figure 4. Weights of second-level indicators for each year in 617 counties within six provinces from 1999–2019.

Throughout the six provinces, A6 had a significant promoting effect on apple production in Hebei, Shanxi, Shaanxi and Gansu, whereas A7 mainly contributed to apple production in Shandong and Henan. Apple growth starts from germination to fruit colouring (March to September), and fertilization is a key human factor in the early growth of branch buds (March). Considering the geographical advantages of Hebei, Shanxi, Shaanxi and Gansu, all possessing natural conditions are amenable for apple cultivation, and the economic development of the regional apple industry is relatively mature. As a result, the amount of fertilizer application has become the main factor promoting regional apple production in these four provinces.
In Henan, the apple industry fully embraces the production potential of existing technologies, and producers grasp the performance of existing apple cultivars and cultivation technologies (Gu & Wang, Citation2007). However, the population density of Henan has reached 6.827 persons/hm2, the highest among the six provinces. Population is a key factor limiting the increase in apple output of Henan, as A18 and A15 respectively ranked 18th and 19th in terms of weight among the 21 third-level indicators. Therefore, agricultural mechanization could alleviate the inhibition effect of population pressure on apple production in Henan. On the contrary, Gansu is a typical vast and sparsely populated province with a population density of only 1.884 persons/hm2, which is a major contributor to the development of the regional apple industry. Sufficient cultivated land area also provides a factor input advantage for apple production in Gansu, based on the larger weights of A8 and A17. Due to the disadvantages of natural resource endowments such as climate and topography, lack of cultivated land area (A17) is the principal factor restricting the development of the regional apple industry in Hebei, Shandong and Shanxi.
Based on second-level indicators (), apple production has been promoted mainly by fertilizer machinery input and then by natural resources over the past 21 years. Especially before 2007, fertilizer machinery input was the decisive factor in improving apple production. Overall, the contributions of the seven second-level indicators to apple production can be divided into two stages: 1999–2007 and 2007–2019, with each indicator having a greater fluctuation range in the former stage that in the latter stage. The contribution of opportunity cost to apple production was relatively stable across the two stages. Topography and labour cost were the prerequisites that held back apple production throughout the six provinces. Specifically, fertilizer machinery input and natural resources were the decisive factors to enhance apple production in the six provinces and each province. Topography was the main factor limiting apple production in the six provinces, as well as in Hebei and Henan, labour cost was the primary limiting in Shanxi, Shaanxi and Gansu, and ecological pressure was the key limiting factor in Shandong.
Apple cultivation in Shandong, Shanxi, Shaanxi and Gansu has reached large-scale operations, making a substantial contribution to China's apple market. During apple picking, extreme weather can lead to limited human investment, whereas mechanized procurement can effectively reduce pressure on apple picking and acquisition. In Hebei and Henan, economic development of the apple industry mainly depends on technological progress, but these two provinces have not made full use of technologies. In particular, Hebei has a scale efficiency close to 1, with small space for expansion and limited natural resources for large-scale operations. As such, apple growers can obtain less economic surplus without increasing or adding factor inputs. Income and storage amounts are inconsistent with the expectations of apple growers to increase household income by growing apples, which strongly weakens the economic development of the apple industry in Hebei. Improving the efficiency of apple production and fully embracing the potential of technological production is the core driving force for regional economic growth in Hebei (Shi et al., Citation2011). Therefore, in the apple production layout, fertilizer machinery input should be considered at first, and natural resources are another major factor to be taken into account.
The comprehensive weights and priorities of third-level indicators () and second-level indicators () in 617 counties during 1999–2019 were calculated based on the annual indicator weights. The third-level indicators that had the largest contribution to apple production (top 20%) were A7, A9, A6 and A5. Based on the concept of eco-environmental fragility, apple production has become a pillar industry in arid areas, among wealthy people and well-developed counties. The six provinces have geographical advantages in terms of natural conditions for apple planting, which plays a significant role in rural revitalization. Under these conditions, modernization of agricultural resources is essential for improving apple production. Therefore, these four indicators have become the primary factors affecting regional apple production. Additionally, cultivated land is a basic resource for agricultural development. During economic development based on the ecological concept, the available arable land area in China should be adequate for cultivating not only the four major food crops but also for apples. Accordingly, A3 has become the main contributor for large-scale apple production. In summary, these five third-level indicators are extremely important in the third-indicator system and are assigned high weights. Among the second-level indicators, fertilizer machinery input, natural resources, and opportunity cost were the key factors affecting regional apple production, and they particularly played a decisive role in Hebei, Shanxi, Henan, Shaanxi and Gansu. In Shandong, natural resources were the most important factor for apple production.
Table 2. Comprehensive weights and priorities of third-level indicators in 617 counties during 1999–2019.
Table 3. Comprehensive weights and priorities of second-level indicators in 617 counties during 1999–2019.
4.1.3. Impact of cost input on apple production regulated by eco-environmental vulnerability
Since the key factors influencing apple production in the six provinces were fertilizer machinery input, natural resources and opportunity cost, the impact of cost input on regional apple production could be positively regulated by natural resources. However, spatial heterogeneity occurs due to the impact of second-level indicators on regional apple production. To address this issue in greater depth, we analysed the data for each province separately. Using the grey correlation model, the comprehensive weights and priorities of provincial second-level indicators were calculated from annual indicator weights in 1999–2019. The first, second and third influencing factors of apple production in Shandong were fertilizer machinery input (0.1438), natural resources (0.1432) and topography (0.1430), which partly differed from those in the six provinces. The top three influencing factors in Hebei, Shanxi, Henan, Shaanxi and Gansu were the same as those in the six provinces, namely, fertilizer machinery input (0.1460, 0.1455, 0.1478, 0.1522, 0.1485), natural resources (0.1443, 0.1445, 0.1446, 0.1452, 0.1458) and opportunity cost (0.1436, 0.1424, 0.1417, 0.1415, 0.1418). This shows that eco-environmental vulnerability in the six provinces exhibits varied effects in regulating the impact of cost input on regional apple production. The regulatory effect of eco-environmental vulnerability is significant for the impact of cost input on apple production efficiency in the six provinces and each province, especially in Shandong. In particular, natural resources have a prominent positive regulatory effect, with fertilizer machinery input being the key factor to improve apple production efficiency.
The top three influencing factors were taken as the main factors affecting apple production in each province, and the weights of all third-level indicators in each county were calculated based on these factors and grey incidence matrix. Assuming that a higher TOPSIS closeness is correlated with a more optimal region, we took into account the combination of TOPSIS closeness and indicator weights for the six provinces and each province, as well as the regional division of China's apple production under different schemes ((c)). As such, the third-level indicators with a weight ranking ≥0.051 were extracted as the optimal factor input of regional apple production. The optimal third-level indicators of the top three influencing factors were analysed by taking the county domain with a TOPSIS closeness ranking ≥0.20 as the optimal region input under the optimal factor. The 617 counties in six provinces were divided into five categories by TOPSIS closeness: < 0.1, 0.1 (including 0.1) − 0.2, 0.2 (including 0.2) − 0.3, 0.3 (including 0.3) − 0.4 and ≥0.4, which was used to characterize the optimal regions for apple production ().
Figure 5. Proportion of optimal indicators for the top three factors influencing of counties in the optimal region selection of six provinces in 1999–2019 (%).

There was a high heterogeneity in the optimal region selection for the six provinces and each province, as well as in the top third-level indicator weight ranking area of the three main influencing factors (). Among the first, second and third influencing factors, 14 (a0)), 15 (b0)) and 14 (c0)) counties in the six provinces had the highest weighting ratio of third-level indicators, respectively. Nine indicators contributed significantly to apple production, among which A20, A11, A14, A13 and A1 were the greatest contributors. The best counties from east to west were mainly distributed in Shanxi (Yuncheng City and Linfen City), Shandong (Heze City), Henan (Lingbao City), Shaanxi (Weinan City and Yan'an City) and Gansu (Jiuquan City). For all three influencing factors, A15, A17, A6, A9, A3, A7, A1 and A14 showed the optimal factor allocation for apple production in each province. Additionally, A6 was the determinant of apple production for the first influencing factor (a1–a6)), A3 and A1 were the determinants of apple production for the second influencing factor (b1–b6)), A1 and A16 were the determinants of apple production for the third influencing factor (c1–c6)). Overall, A11, A16, A12, A20 and A13 also achieved optimal factor allocation to facilitate regional apple production (). The results indicate that meteorological factors, ecological pressure and topography play a significant positive role in regulating the impact of cost input on regional apple production.
4.1.4. Region selection under optimal factor allocation for apple production
Based on TOPSIS closeness, the optimal region selection for apple production in Hebei was distributed in Chengde and Zhangjiakou in the north, Tangshan in the east, Baoding in the centre, and Shijiazhuang and Hengshui in the south. The optimal region selection of Hebei under the first, second and third influencing factors comprised 27, 19 and 16 counties, respectively (). Shandong had the largest number of counties with TOPSIS closeness ranking ≥0.20 among the six provinces. Its optimal region selection was distributed in Yantai, Weifang, Zibo in the east, Binzhou in the north and Heze in the west. There were 35, 32 and 29 optimal counties under the first, second and third influencing factors, respectively, which cumulatively accounted for 28.46% of the total counties in Shandong. That is, nearly a quarter of the counties in Shandong are optimal for apple production, which provides sufficient space for large-scale operations of apple production. The optimal region selection of Shanxi was distributed in Taiyuan, Jinzhong and Lvliang in the centre, in addition to Yuncheng in the south, and there were 7, 6 and 4 counties under the first, second and third influencing factors, respectively.
The optimal region selection of Henan involved Anyang in the north and Shangqiu in the east, as well as Luoyang and Sanmenxia in the west, with 19, 18 and 18 counties under the first, second and third influencing factors, respectively. The optimal region selection of Shaanxi was distributed in Yulin and Yan’an in the north, in addition to Xi’an, Weinan and Hsienyang in the centre, with 28, 27 and 26 counties under the first, second and third influencing factors, respectively. The optimal region selection of Gansu was distributed in Qingyang, Pingliang and Tianshui in the northeast, Jiuquan in the northwest, Lanzhou in the centre and Longnan and Gannan in the south, with 23, 18 and 19 counties under the first, second and third main factors, respectively. All selected counties () were optimal regions for apple production regardless of the influencing factors. Accordingly, apple production in nearly a quarter of Shandong and Shaanxi, as well as in 27 counties of Hebei, seven counties of Shanxi, 19 counties of Henan and 23 counties of Gansu have achieved optimal factor allocation, which allowed for optimal region selection.
Based on the distribution patterns of the three main influencing factors, the regions with TOPSIS closeness ≥0.1 in the six provinces shifted from Shandong, Shanxi, Shaanxi and Gansu under the first influencing factor to Shanxi, Shaanxi and Gansu under the second influencing factor. The optimal regions under the third influencing factor showed spatial spillover from the optimal regions under the first and second influencing factors in Shandong, Shaanxi and Gansu (). Compared with variation within the six provinces, two patterns were highlighted: first, since 1999, the apple production area has expanded year-by-year, with prominent large-scale operation; second, either between or within the six provinces, there is remarkable variation in apple production. Therefore, based on the top three factors influencing region selection and efficiency improvement, the following regions should be prioritized for apple production: northeastern and southern Gansu, central and northern Shaanxi, central and southern Shanxi, western Henan, eastern and western Shandong and northern and southern Hebei.
4.2. Determination of optimal regions based on closeness
We ranked the optimal regions for apple production based on TOPSIS closeness, and used a threshold score ≥0.20 for 617 counties at the first level and <0.20 at the second level (). Data from five periods (1999, 2005, 2010, 2015 and 2019) were used to analyse the changes in optimal region selection. The first-level selection changed dynamically and steadily from 1999 to 2015, with 45, 30, 25 and 15 optimal counties in the four periods, respectively; the number of optimal counties showed a decreasing trend year by year (). By 2015, the second-level selection covered almost six provinces, and the first-level optimal counties were scattered from west to east in western Gansu (Jiuquan), central Shaanxi (Hsienyang), western Henan (Sanmenxia), western Shanxi (Yuncheng), western (Heze) and eastern (Yantai and Qingdao) Shandong and southern Hebei (Hengshui). Presently, the first-level optimal region selection involves six provinces, and the number of first-level optimal counties is up to 51 counties, an increase of 5.835% compared with the spatial layout in 2015. Dynamically altered regions selected at the provincial level were significantly heterogeneous (). For Hebei, Shandong, Henan and Shaanxi, the ‘westward and northward migration’ of optimal regions in 1999–2019 was particularly evident.
Specifically, changes in the number of first-level optimal counties in Hebei and Shandong were consistent with that of the six provinces. There were segmental decreases from 1999 to 2015 followed by a rebound in 2019. In particular, a ‘northward expansion’ occurred in Hebei. The number of first-level optimal regions increased by 17.692% and 42.277% in Hebei and Shandong, respectively. The first-level optimal regions were mainly distributed in counties located in Tangshan (eastern Hebei), Chengde and Zhangjiakou (northern Hebei). From 2005 to 2015, the first-level optimal regions in Shandong were steady, mainly distributed in Yantai, Qingdao, Weifang, Zibo and Heze. However, in 2019, the number of first-level optimal counties in Shandong reached 85 (69.106%), which was much higher than that in 2015 (26.829%). The ‘westward and northward migration’ was the most prominent in Henan and Shaanxi. The number of first-level optimal counties in Henan increased and decreased steadily from 2005 to 2019. By 2019, a total of 45 first-level optimal counties were distributed mainly in Zhoukou, Xinxiang, Zhengzhou, Luoyang and Sanmenxia, accounting for 40.179% of the total counties in Henan. From 1999, the number of first-level optimal counties in Shaanxi increased year by year, reaching 72.368% of the total counties in 2019. Moreover, the first-level optimal counties showed remarkable spatial distribution and clustering throughout Shaanxi.
Generally, the first-level optimal region selection showed an increasing trend from 1999 to 2019 in each province. The advantageous apple production areas – Shandong and Shaanxi – have outstanding advantages in the spatial layout of first-level optimal regions, and changes in their optimal region selection are mainly driven by policies. Shandong, relative to Shaanxi, issued plans or policies for apple production for the first time on March 30, 2012 (Implementation Opinions of the People's Government of Shandong Province on Accelerating the Transformation and Upgrading of Agricultural Mechanisation and Agricultural Machinery Equipment Industry, Citation2013). Later plans or policies were released on May 6, 2012 (2015), February 5, 2013 (2017–2019), 2015 (2020–2022), March 20, 2017 (2017–2020) and October 13, 2017 (2016–2020). However, the policy-driven role in apple production is relatively weak in Shandong Province. The government of Shaanxi issued and implemented the ‘Notice on Printing and Distributing the Apple Industrialization Development Plan (Citation1998–2010)’ on May 20, 1998 (hereafter referred to as the ‘Plan’). Four years later, the ‘Plan (2001–2010)’ was released on June 23, 2002, and several plans or policy measures related to apple production were promulgated by December 30, 2019. All these plans and policies have contributed to the development of apple production in Shaanxi. Therefore, the first-level optimal region selection of Shaanxi was characterized by a county-level agglomeration distribution from 1999 to 2019, and the number of optimal counties for apple production increased over time.
Comparing the spatial distribution of optimal regions in each province () revealed that Shanxi had the largest number of optimal counties that could be further expanded (84%). Hebei and Henan ranked second (66.154%) and third (59.821%), respectively. Therefore, Shanxi needs to explore the advantages of the apple industry, improve the competitiveness of apple production, encourage farmers to plant apples and increase their income, and enhance regional agricultural development. Hebei and Henan is expected to increase technical investment and consolidate large-scale operation for apple production. As far as the two advantageous production areas – Shandong and Shaanxi are concerned, Yantai City (the apple capital of China and having rich experiences in apple production planning or policy formulation) has always been the major apple production area. However, in terms of the first-level optimal region selection in the five periods, Heze had consistently ranked first for the TOPSIS closeness in Shandong by 2019. Additionally, Juancheng, Juye County, Shanxian, Yuncheng County and Mudan District had shown the optimal factor allocation, providing additional ‘capital’ areas for apple production in Shandong. In the case of Shaanxi, Luochuan County and Yan'an City are landmark advantageous areas for apple production. In 2019, Chengcheng County in Weinan City had the optimal factor allocation and entered the first-level optimal selection in Shaanxi. Additionally, optimal factor allocation was achieved in Qianxian of Hsienyang City, Yanchuan County of Yan'an City, Liquan County of Hsienyang City, Fu County of Yan'an City and Xunyi County of Hsienyang City.
5. Discussion
5.1. Spatial evolution of apple production
Based on the Hurst index, we predicted the spatial evolution of apple production in 617 counties of six provinces within the major apple production areas of China over the past 21 years. The prediction results for 1999–2009 and 2010–2019 in the six provinces were highly compatible with the conclusions of the R/S principle. Compared with that in 1999–2009, the areas with continuous growth in apple production showed a strong spatial agglomeration and remarkable expansion in 2010–2019. This may be a significant manifestation of regional large-scale operations and the spatial spillover of advantageous areas for apple production. Additionally, these areas (H > 0.5) tended to migrate westwards and northwards between the six provinces or within provinces. In total, 197 counties have achieved a continuous increase in apple production over the past 21 years, mainly in the eastern edge of Gansu, northern, central and southern parts of Shaanxi, western and central parts of Henan, northern, central and southern parts of Shanxi, northern, central and southern parts of Hebei, and western, central and eastern parts of Shandong. From 2010 to 2019, 86 new counties were added, which indicates the uneven allocation of production factors and the mismatch of factor input and output in apple production areas. This underscores the priority of optimizing factor allocation in the optimal ecological areas for apple production in China.
In the advantageous apple production areas such as Shandong, decreased apple production occurred in counties that have achieved rapid economic development in recent years. Industrial diversification and advantageous industries quickly replace apple production with weaker development momentum. In addition to the original apple cultivars (red Fuji and Guoguang) in Shandong, both cultivar and quality are brought to the fore by other advantageous areas in the context of cultivar optimization. For example, the emergence of dominant cultivars (Ruiyang and Ruixue) in Shaanxi contributes to the apple market, and such strong market stock has caused the apple output in Shandong to exceed market demand, resulting in oversupply and a subsequent decline in production. Shaanxi, which also has a unique endowment of apple production resources, has implemented policies in recent years to improve orchard ecology, advance apple breeding systems, and integrate technologies for prevention and control of diseases, pests and weeds that restrict the development of the apple industry in arid areas. With varying levels of adjustment and optimization, apple production has become the main fruit cash crop driving the agricultural product market. Sales of local apples are increasing annually and market demand shows insufficient supply, so more recently, apple production has steadily increased in Shaanxi.
5.2. Efficiency improvement of regional apple production under optimal factor allocation
In the cost-ecological indicator system we constructed, five third-level indicators – A6, A9, A7, A5 and A3 – accounted for an extremely high proportion of optimal county weights in the six provinces. From a habitat perspective, all six provinces are located in arid and semi-arid areas with geographical advantages such as long sunshine hours, large diurnal temperature difference and strong solar radiation, which are major areas for growing high-quality apples. The pillar industries of enriching people and strengthening the county play an essential role in the fight against poverty. Under favourable external natural conditions, formulating and implementing rational cultivation and management measures, increasing apple outputs, reducing production costs, and ensuring quality standardization are key technical issues for the regional apple industry. Therefore, fertilizer machinery input and natural resources have become the main factors affecting apple production in the six provinces. Additionally, arable land area is a basic condition for agricultural development and it can be used for apple orchards in China based on guaranteeing food production (including the four major food crops – rice, wheat, corn and soybean). Thus, available arable land area has become the main limiting factor for increasing the scale of regional apple production, with A17 being the main ecological pressure for regional apple production. Thus, fertilizer machinery input and natural resources play a significant role in improving the efficiency of regional apple production, whereas ecological pressure inhibits the efficiency of cost input.
5.3. Rationality of optimal region selection
According to the theory of regional development, regional economic development mainly relies on the first, second and tertiary industries under the jurisdiction of prefecture-level city residences, municipal districts and prefecture-level central cities. In the areas with a relatively high urbanization rate, agriculture is not the main factor driving regional economic development, so these areas were excluded in this study. Based on the completeness of the yearbook data and the actual regional apple cultivation, 617 counties were selected and a 21-indicator system considering cost-eco-environmental vulnerability was constructed to comprehensively evaluate the optimal region selection and efficiency improvement of apple production. Using the TOPSIS model, the counties where the TOPSIS closeness ranking was ≥0.20 (corresponding to the third-level indicator of weight ranking ≥0.051) was selected as the optimal regions where the optimal factor input of apple production was obtained. To verify the authenticity of the optimal region selection, we further divided three major habitats according to the theory of adaptability based on the division schemes of four major apple production areas. We confirmed the excellent results for 126 counties in the six provinces and counties obtained by the Apple's zoning plan Key County and Cities of Apple's Advantageous Regional Layout 2017–2025. The optimal region selection results obtained from our study led us to the following conclusions:
First, based on extensive research and economic development models in the post-pandemic era, it is necessary to take into account the regulatory role of eco-environmental vulnerability factors in cost input to facilitate the sustainable development of apple industry in China. This will greatly help local governments to formulate regional factor matching for apple production while enhancing regional economic coordination and comprehensive development.
Second, apple cultivation – a high value-added and labour-intensive agricultural endeavour – has become a key channel for farmers to increase their income and wealth in the Loess Plateau, Bohai Rim and other major apple production areas. In the advantageous area of Shandong, the number of county-level cities and districts among the 123 research units (28 and 53, respectively) is the highest among the six provinces. Regarding report notification (State Council Guofa, Citation1993, No. 38) on county-level cities and counties, population is a measure of how these areas can meet the criteria for the conversion of counties into cities, and counties into districts. Therefore, compared with Shandong, a higher level of A15 in Shaanxi is a principal factor to increase the labour force advantage in regional apple production. However, Shandong has a better ecological environment than Shaanxi in terms of regional natural resources, topography and meteorological factors. As such, the increase of A1 in Shandong can stimulate farmers’ willingness to plant apples and increase the cost advantage of regional apple production, leading to a significant positive effect on the allocation of production factors.
Additionally, apples are dryland crops and our research area is located in arid and semi-arid areas. Therefore, the 617 counties are suitable regions for apple cultivation, meeting the habitat conditions for growing apples from a climate perspective. Taking into account the economic cost of apple production, we optimized region selection for apple production in China from a comprehensive cost-ecological perspective.
6. Conclusions and policy enlightenments
With respect to the spatial evolution of apple production, areas with continuous growth in 1999–2019 were spread all over the six provinces, with the proportion of pixel area as high as 93.8%. These areas showed a dispersed evolutionary trend in 1999–2009, with the regional pixel area accounting for 91.9%, but their spatial distribution was highly agglomerated in 2010–2019, with the regional pixel area accounting for 67.5%. Some counties with optimal ecological indicators have failed to capitalize on their advantages. The uneven allocation of regional factors and the mismatch between factor input and output are key issues that need to be urgently addressed to improve the efficiency of region apple production.
Based on the optimal indicators of the top three influencing factors for apple production, Yuncheng and Linfen in Shanxi, Heze in Shandong, Lingbao in Henan, Weinan and Yan'an in Shaanxi and Jiuquan in Gansu are the best candidates for the development of apple production areas. Fertilizer machinery input, natural resources, and opportunity costs are the main factors affecting apple production in Hebei, Shanxi, Henan, Shaanxi and Gansu, indicating that natural resources play a significant positive role in regulating the impact of cost input. The amount of agricultural fertilizer application converted to scalar amount of fertilizer machinery input is a key factor for improving apple production efficiency in Hebei, Shanxi, Shaanxi and Gansu. The total power of agricultural machinery mainly contributes to apple production efficiency in Shandong and Henan. Additionally, the amount of agricultural plastic film used is a major contributor to apple production in Shaanxi and Gansu, whereas effective irrigation area exhibits a strong positive effect in regulating the impact of cost input in Hebei, Shandong, Shanxi and Henan.
The results of the TOPSIS model indicate noticeable changes in the first-level optimal region selection from 1999 to 2019 in Hebei, Shandong, Henan and Shaanxi, which exemplified by a ‘northward expansion’ in Hebei and a ‘westward migration’ in Henan. Shanxi has the largest number of counties for further expansion (84%), followed by Hebei (66.154%) and Henan (59.821%). Accordingly, Shanxi needs to explore the advantages and disadvantages of the apple industry to promote the development of regional agricultural economy, whereas Hebei and Henan are expected to increase technology investment and consolidate large-scale operation.
Based on our conclusions, we propose the following policy enlightenments:
At the regional level, the governments should pay full attention to the differences in natural resources and further integrate the actual situation of regional apple industry structure with natural resource conditions and the concentration of technological innovation factors, as well as adjust measures to local conditions in order to strengthen the economic development model with regional apple production characteristics. Additionally, it is crucial to correctly match and develop the modern agricultural land uses – non-agricultural use of arable land and non-grain use of grain crop fields, which is practical and feasible to ensure global food security. In particular, it is necessary to accelerate the development planning of suitable areas, effectively promote these areas to play the latecomer advantage driven by technological innovation, and provide a strong backup force for the large-scale operation of apple production in advantageous areas during the supply-side structural reform.
With regard to production factors, the governments ought to firmly reinforce the reform of land property right system, considerably improve the value-added policy system of management rights based on stable contract rights, and vigorously strengthened the restructuring, integration and orderly transfer of rural land and other production factors based on market-oriented mechanisms, which can fully stimulate and release the potential productivity of rural apple planting. Further, it is crucial to strengthen the innovation of the two-way flow system of manpower and logistics between urban and rural areas, thereby providing the necessary environment of external mechanisms for economic development that supports the continuous improvement of apple production efficiency in the current global context.
Acknowledgment
The authors would like to thank the anonymous reviewers for their helpful comments on the previous version of this paper.
Data availability statement
The data used in this study are available upon reasonable request from the corresponding author.
Disclosure statement
No potential conflict of interest was reported by the author(s).
Additional information
Funding
Notes on contributors
Jing Wang
Jing Wang is a PhD candidate at Northwest A&F University. The major is agriculture and forestry economic management, engaged in spatial layout and optimization research of apple production area. During my doctoral study I published research results on the spatio—temporal evolution and driving mechanism of apple production layout in China in the Journal of Food and Energy Security. The previous study focused on the research of ecological restoration. A number of papers related to this research have been published in journals such as the Transaction of the Chinese Society of Agricultural Engineering, Acta Ecologica Sinica, Arid Zone Research and Resources and Environment in the Yangtze River Basin. At present, there are three SSCI articles on Apple production layout under submission.
Tianjun Liu
Tianjun Liu is a professor and doctoral supervisor at the School of Economics and Management at Northwest A&F University. He is currently the minister (director) of the Talent Work Department (High—level Talent Office) of the Party Committee of Northwest A&F University, the director of the West Development Research Institute, a new think tank for colleges and universities in Shaanxi Province and the deputy director of the Rural Revitalization Strategy Research Institute of Northwest A&F University. His research interests include agricultural economic theory and policy, regional economy and industrial development, farmer entrepreneurship, cultivation and opening of agricultural markets, etc. It has made remarkable achievements in the field of Apple's industrial system. He has presided over more than 20 provincial and ministerial level projects, including key projects of the National Natural Science Foundation, general projects, humanities and social science projects of the Ministry of Education and national soft science projects. In recent years, he has published nearly 16 representative academic papers.
References
- Apple Advantage Area Layout Plan. (2008–2015). Ministry of Agriculture and Rural Affairs of the People’s Republic of China. 2008. https://www.moa.gov.cn.
- Apple Advantage Regional Development Plan. (2003–2007). Ministry of Agriculture and Rural Affairs of the People’s Republic of China. 2003. https://www.moa.gov.cn.
- Bai, X. G., Li, J. S., & Huo, X. X. (2015). Empirical studies on the climate change and variation of regional apple production structure in China. Economic Geography, 35(6), 130–137. https://doi.org/10.15957/j.cnki.jjdl.2015.06.018
- Chemeris, A., Liu, Y., & Ker, A. (2022). Insurance subsidies, climate change, and innovation: Implications for crop yield resiliency. Food Policy, 108, 102232. https://doi.org/10.1016/j.foodpol.2022.102232
- China Rural Statistical Yearbook. (1979–2020). China Economic and Social Big Data Research Platform. https://data.cnki.net/.
- Cláudia, M. V., Dulce, F., Patrícia, A., Jorge, R., & Paulo, P. (2022). Agricultural land systems importance for supporting food security and sustainable development goals: A systematic review. Science of the Total Environment, 806(3), 150718. https://doi.org/10.1016/j.scitotenv.2021.150718
- Congedo, L., & Macchi, S. (2015). The demographic dimension of climate change vulnerability: Exploring the relation between population growth and urban sprawl in Dar es Salaam. Current Opinion in Environmental Sustainability, 13, 1–10. https://doi.org/10.1016/j.cosust.2014.12.002
- Estrada-Carmona, N., Attwood, S., Cole, S. M., Remans, R., & DeClerck, F. (2020). A gendered ecosystem services approach to identify novel and locally-relevant strategies for jointly improving food security, nutrition, and conservation in the Barotse Floodplain. International Journal of Agricultural Sustainability, 18(4), 351–375. https://doi.org/10.1080/14735903.2020.1787618
- Feng, X. L., Liu, M. Y., & Huo, X. X. (2016). Influence of adaptive behavior to climate change and its spatial effects on farmers’ income: Based on the survey data of apple farmers from 4 provinces. Journal of Agro-Forestry Economics and Management, 15(5), 570–578. https://xuebao.jxau.edu.cn.
- Gao, X. D., Zhao, X. N., Wu, P. T., Yang, M., Ye, M. T., Tian, L., Zou, Y. F., Wu, Y., Zhang, F. S., & Siddique, K. H. M. (2021). The economic–environmental trade-off of growing apple trees in the drylands of China: A conceptual framework for sustainable intensification. Journal of Cleaner Production, 296, 126497. https://doi.org/10.1016/j.jclepro.2021.126497
- Granero, M. A. S., Segovia, J. E. T., & Pérez, J. G. (2008). Some comments on Hurst exponent and the long memory processes on capital markets. Physica A: Statistical Mechanics and its Applications, 387(22), 5543–5551. https://doi.org/10.1016/j.physa.2008.05.053
- Gu, H., & Wang, A. M. (2007). Evaluation of apple production efficiency in Henan based on Malmquist index. Journal of Agrotechnical Economics, 02, 99–104. https://kns.cnki.net/kcms/detail/detail.aspx?FileName=NYJS200702017&DbName=CJFQ2007.
- Hall, C., Dawson, T. P., Macdiarmid, J. I., Mattews, R. B., & Smith, P. (2017). The impact of population growth and climate change on food security in Africa: Looking ahead to 2050. International Journal of Agricultural Sustainability, 15(2), 124–135. https://doi.org/10.1080/14735903.2017.1293929
- He, L., Shen, J., & Zhang, Y. (2018). Ecological vulnerability assessment for ecological conservation and environmental management. Journal of Environmental Management, 206(1), 1115–1125. https://doi.org/10.1016/j.jenvman.2017.11.059
- He, X., Lin, Z. S., Liu, H. Y., & Qi, X. Z. (2016). Analysis of the driving factors of PM2.5 in Jiangsu province based on grey correlation model. Acta Geographica Sinica, 71(7), 1119–1129. https://doi.org/10.11821/dlxb201607003
- Hu, X. J., Ma, C. M., Huang, P., & Guo, X. (2021). Ecological vulnerability assessment based on AHP-PSR method and analysis of its single parameter sensitivity and spatial autocorrelation for ecological protection – A case of Weifang city, China. Ecological Indicators, 125, 107464. https://doi.org/10.1016/j.ecolind.2021.107464
- Huo, G. P., Gosme, M., Gao, X. D., Dupraz, C., Yang, J. L., & Zhao, X. N. (2021). Dynamics of interspecific water relationship in vertical and horizontal dimensions under a dryland apple–Brassica intercropping system: Quantifying by experiments and the 3D Hi–sAFe model. Agricultural and Forest Meteorology, 310, 108620. https://doi.org/10.1016/j.agrformet.2021.108620
- Hurst, H. E. (1951). Long term storage capacity of reservoirs. Transactions of the American Society of Civil Engineers, 116(12), 776–808.
- Implementation Opinions of the People's Government of Shandong Province on Accelerating the Transformation and Upgrading of Agricultural Mechanization and Agricultural Machinery Equipment Industry. (2013). Shaanxi Provincial People's Government. [2012–03–30]. https://nync.shandong.gov.cn/zwgk/tfwj/njhglc/201912/t20191223_2492311.html.
- Kendziorski, C. M., Bassingthwaighte, J. B., & Tonellato, P. J. (1999). Evaluating maximum likelihood estimation methods to determine the Hurst coefficient. Physica A: Statistical Mechanics and its Applications, 273(3–4), 439–451. https://doi.org/10.1016/S0378-4371(99)00268-X
- Kharanagh, S. G., Banihabib, M. E., & Javadi, S. (2020). An MCDM–based social network analysis of water governance to determine actors’ power in water–food–energy nexus. Journal of Hydrology, 581, 124382. https://doi.org/10.1016/j.jhydrol.2019.124382
- Krupnik, T. J., Timsina, J., Devkota, K. P., Tripathi, B. P., Karki, T. B., Urfels, A., Gaihre, Y. K., Choudhary, D., Beshir, A. R., Pandey, V. P., Brown, B., Gartaula, H., Shahrin, S., & Ghimire, Y. N. (2021). Chapter four-agronomic, socio-economic, and environmental challenges and opportunities in Nepal’s cereal-based farming systems. Advances in Agronomy, 170, 155–287. https://doi.org/10.1016/bs.agron.2021.06.004
- Li, J. T., & Li, Y. X. (2019). Influence measurement of rapid urbanization on agricultural production factors based on provincial panel data. Socio-Economic Planning Sciences, 67, 69–77. https://doi.org/10.1016/j.seps.2018.09.004
- Li, Y., Xiong, S., Tang, X. Y., Wu, H., Han, C., Yi, M., & Wang, Y. (2021). Loose nanofiltration membrane with highly-branched SPEI/PEI assembly for dye/salt textile wastewater treatment. Journal of Environmental Chemical Engineering, 9(6), 106371. https://doi.org/10.1016/j.jece.2021.106371
- Liao, Y., Cao, H. X., Liu, X., Li, H. T., Hu, Q. Y., & Xue, W. K. (2021). By increasing infiltration and reducing evaporation, mulching can improve the soil water environment and apple yield of orchards in semiarid areas. Agricultural Water Management, 253, 106936. https://doi.org/10.1016/j.agwat.2021.106936
- Liu, D., Qi, X. C., Fu, Q., Li, M., Zhu, W. F., Zhang, L. L., Faiz, M. A., Khan, M. I., Li, T. X., & Cui, S. (2019). A resilience evaluation method for a combined regional agricultural water and soil resource system based on Weighted Mahalanobis distance and a Gray–TOPSIS model. Journal of Cleaner Production, 229, 667–679. https://doi.org/10.1016/j.jclepro.2019.04.406
- Liu, T. J., & Fan, Y. (2012). Analysis on the changes and influencing factors of the production layout of China's apple main production areas. Issues in Agricultural Economy, 33(10), 36–42, 111. https://doi.org/10.13246/j.cnki.iae.2012.10.007
- Lv, X. D., Wang, T., Ma, Z. M., Zhao, C. Y., Siddique Kadambot, H. M., & Ju, X. T. (2019). Enhanced efficiency nitrogen fertilizers maintain yields and mitigate global warming potential in an intensified spring wheat system. Field Crops Research, 244, 107624. https://doi.org/10.1016/j.fcr.2019.107624
- Ma, B., Zhang, B., Jia, L. G., & Huang, H. (2020). Conditional distribution selection for SPEI-daily and its revealed meteorological drought characteristics in China from 1961 to 2017. Atmospheric Research, 246, 105108. https://doi.org/10.1016/j.atmosres.2020.105108
- Mafi-Gholami, D., Pirasteh, S., Ellison, J. C., & Jaafari, A. (2021). Fuzzy–based vulnerability assessment of coupled social–ecological systems to multiple environmental hazards and climate change. Journal of Environmental Management, 299, 113573. https://doi.org/10.1016/j.jenvman.2021.113573
- Malmir, M., Javadi, S., Moridi, A., Neshat, A., & Razdar, B. (2021). A new combined framework for sustainable development using the DPSIR approach and numerical modeling. Geoscience Frontiers, 12(4), 101169. https://doi.org/10.1016/j.gsf.2021.101169
- Mousavi-Avval, S. H., Rafiee, S., & Mohammadi, A. (2011). Optimization of energy consumption and input costs for apple production in Iran using data envelopment analysis. Energy, 36(2), 909–916. https://doi.org/10.1016/j.energy.2010.12.020
- National Development and Reform Commission. 2023. China Economic and Social Big Data Research Platform. https://data.cnki.net/.
- Ni, N., Li, X. N., Yao, S., Shi, R. Y., Kong, D. Y., Bian, Y. R., Jiang, X., & Song, Y. (2021). Biochar applications combined with paddy-upland rotation cropping systems benefit the safe use of PAH–contaminated soils: From risk assessment to microbial ecology. Journal of Hazardous Materials, 404, 124123. https://doi.org/10.1016/j.jhazmat.2020.124123
- Notice on Printing and Distributing Apple Industrialization Development Plan. (1998–2010). Shandong Modern Agriculture and Rural Development Research Center. [1998–05–20]. https://www.shaanxi.gov.cn/gk/zfwj/47884.htm.
- Sandile, T., Hadebe, T.M., & Albert, T.M. (2020). Sorghum best practice management recommendations based on AquaCrop modeling scenario analysis in various agro–ecologies of KwaZulu Natal, South Africa. Physics and Chemistry of the Earth, Parts A/B/C, 117, 102866. https://doi.org/10.1016/j.pce.2020.102866
- Shi, C. N., Gao, J., Su, B., & Yao, S. B. (2017). Region selection of returning farmland to forest based on cost-benefit analysis: A case study on the loess plateau. Issues of Forestry Economics, 37((04|4)), 18–22+99. https://doi.org/10.16832/j.cnki.1005–9709.2017.04.004
- Shi, H. J., Wang, J. Q., & Wang, Y. D. (2011). Empirical research on the production efficiency of the apple industry in Hebei province based on DEA. Journal of Agrotechnical Economics, 10, 86–91. https://doi.org/10.13246/j.cnki.jae.2011.10.011
- Silva, D. S., Arima, E. Y., dos Reis, T. N. P., & Rattis, L. (2023). Temperature effect on Brazilian soybean yields, and farmers’ responses. International Journal of Agricultural Sustainability, 21(1), 2173370. https://doi.org/10.1080/14735903.2023.2173370
- Souza, G. d. S., & Gomes, E. G. (2023). Assessing the influence of external factors on agricultural production in Brazil. Socio-Economic Planning Sciences, (in press). 101440. https://doi.org/10.1016/j.seps.2022.101440
- State Council Guofa. (1993). No. 38 Notice of the State Council Approving and Transmitting the Ministry of Civil Affairs Report on Adjusting the Standards for CityEstablishment. https://www.gov.cn/xxgk/pub/govpublic/mrlm/201208/t20120820_65479.html.
- Sun, M., Gao, X. R., Zhang, Y. L., Song, X. L., & Zhao, X. N. (2022). A new solution of high–efficiency rainwater irrigation mode for water management in apple plantation: Design and application. Agricultural Water Management, 259, 107243. https://doi.org/10.1016/j.agwat.2021.107243
- Tautges, N., Borrelli, K., Goldberger, J., Machado, S., Fuerst, E. P., Roberts, D., & Burke, I. (2022). Chapter three-growing small grains organically in the semiarid west: A review of markets and management practices to optimize productivity and sustainability. Advances in Agronomy, 171, 111–141. https://doi.org/10.1016/bs.agron.2021.10.005
- Tong, S. Q., Lai, Q., Zhang, J. Q., Bao, Y. H., Lusi, A., Ma, Q. Y., Li, X. Q., & Zhang, F. (2018). Spatiotemporal drought variability on the Mongolian plateau from 1980–2014 based on the SPEI-PM, intensity analysis and hurst exponent. Science of The Total Environment, 615, 1557–1565. https://doi.org/10.1016/j.scitotenv.2017.09.121
- United Nations (Department of Economic and Social Affairs). (2021). Population, food security, nutrition and sustainable development. Apr. https://digitallibrary.un.org/record/3924543.
- Wan, H. L., & Wang, J. (2018). Study of dynamic pattern evolution of drought and its correlation with vegetation cover in Baoji area on multi–scale. Acta Ecologica Sinica, 38(19), 6941–6952. https://doi.org/10.5846/stxb201708201496
- Wang, C. F., & Shi, J. M. (2017). Study of spatio-temporal evolution characteristics of apple planting area in Shandong province. Chinese Journal of Agricultural Resources and Regional Planning, 38(12), 170–177. https://doi.org/10.7621/cjarrp.1005-9121.20171225
- Wang, J., & Liu, T. J. (2022). Spatiotemporal evolution and suitability of apple production in China from climate change and land use transfer perspectives. Food and Energy Security, 11, 15. https://doi.org/10.1002/fes3.386
- Yuan, B., Zhang, Y. Y., & Chen, C. (2017). Spatial pattern evolution and its driving factors for apple industry in China. Journal of Arid Land Resources and Environment, 6, 32–37. https://doi.org/10.13448/j.cnki.jalre.2017.175
- Zhang, C. Y., Chang, Q., & Huo, X. X. (2018). Analysis on the layout of China’s apple production transition—Based on the application of GIS technology and barycenter theory. Economic Geography, 38(8), 141–151. https://doi.org/10.15957/j.cnki.jjdl.2018.08.018
- Zhang, D. W., Wu, L. L., Huang, S. Q., Zhang, Z. Y., Ahmad, F., Zhang, G. L., Shi, N., & Xu, H. (2021a). Ecology and environment of the Belt and Road under global climate change: A systematic review of spatial patterns, cost efficiency, and ecological footprints. Ecological Indicators, 131, 108237. https://doi.org/10.1016/j.ecolind.2021.108237
- Zhang, Y. H., Wang, R., Peng, X. X., Zhang, Y. J., Ning, F., Xu, Z. G., Wang, Q., Dong, Z. Y., Jia, G. C., Wei, L., & Li, J. (2021b). Changes in soil organic carbon and total nitrogen in apple orchards in different climate regions on the loess plateau. Catena, 197, 104989. https://doi.org/10.1016/j.catena.2020.104989
- Zhou, Y. X., Li, Y. X., Sun, Y. Y., & Jiang, H. L. (2016). Evaluation of land eco–security based on different evaluation model: A case in shandong peninsula blue economic zone. China Population, Resources and Environment, 26(S2), 207–210. https://kns.cnki.net/kcms2/article/abstract?v=Qe77F90WpyEwp_BWeyXyiqYHZ1onadB60dy5sirCGf0Usd-DOdNLv7vlKX6IgXD7g0sPQqplFWCbi4UPYntkdRRYBMIUv_0KvtaWRDgQKC43dTRQXzMvd5KQmHqch0pKCmtgOiEPvM=&uniplatform=NZKPT&language=CHS.