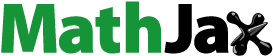
ABSTRACT
Weather events have threatened the realization of food security in Ethiopia. Weather-index crop insurance (WICI) is a risk-transferring strategy recently introduced to farmers in Ethiopia. The study aims to assess the impact of WICI on smallholder farmers' multidimensional food security. To realize its objective, relevant indicators, and the Foster-Greer-Thorbecke index were applied to capture the multidimensional realities of food security and the propensity score matching technique to examine the impact. The results show that WICI has a significant positive impact on smallholder farmers' availability, access, utilization and food stability. Besides, the insured farmers have lower incidence, depth, and severity of food insecurity by 9.44%, 3.24% and 1.06% than non-insured farmers. Thus, to realize progress in food security, we advocate improving smallholder farmers' access to affordable WICI products: the existing pilot crop insurance projects shall be used as a milestone to establish an all-inclusive crop insurance program in Ethiopia. The study urges due attention and integrated efforts to build a reliable weather database system and solve smallholder farmers' meager willingness to pay. It contributes to the debate on how WICI impacts farmers' multidimensional food security, arouses further studies on the issue and policy action on building shock-resilient food security.
1. Introduction
Extreme weather events increasingly threatened Ethiopia (Alemu & Mengistu, Citation2019; Ayinu et al., Citation2022; Tofu et al., Citation2022). The potential adversative effects of climate change on crop production are major concerns (Belay et al., Citation2017). Erratic rainfall patterns, hail, floods, and extreme temperatures are becoming more profound issues causing severe challenges to smallholders’ food production in the country (Gebrtetsadik, Citation2022; Mesfin et al., Citation2022). During the El Niño drought of 2015/2016, more than 27 million Ethiopians faced a food insecurity crisis (Hill & Porter, Citation2017). Historic meteorological data witnessed northern Ethiopia has been recurrently affected by drought hazards (Eze et al., Citation2020). Drought is one of the top causes of food insecurity in the study area (Alemayehu & Bewket, Citation2016; Mohamed, Citation2017).
In Ethiopia, the crop subsector constitutes the largest share (60%) of the agricultural gross domestic product, followed by the livestock sub-sector (27%) (Asresie & Zemedu, Citation2015; Debela & Mohammed, Citation2020). The livelihood of farmers in Ethiopia mainly depends on highly frangible rain-fed agriculture (Tadese et al., Citation2022). Subsistence smallholder farmers dominate crop production: 12 million smallholders account for an estimated 95% of crop production and 85% of employment opportunities in the country (Belay et al., Citation2017; Mesfin et al., Citation2022). It is naturally dependent-lacks technological innovation (Ayinu et al., Citation2022; Eze et al., Citation2020; Orr et al., Citation2021; Tofu et al., Citation2022) and is highly vulnerable to several covariate and idiosyncratic risks (Daryanto et al., Citation2016; Lesk et al., Citation2016; Mbugua et al., Citation2019; Senapati, Citation2020). Various policy-driven strategies such as soil and water conservation, crop varieties improvement, cropping calendar adjustment, tree plantation, and chemical fertilizer application have been promoted to boost smallholders’ crop productivity in Ethiopia. However, the adoption capacity remains limited as their vulnerability to weather-induced risks persists (Belay et al., Citation2017; Tofu et al., Citation2022).
There is a strong bond between adopting improved agricultural inputs and weather risks in Ethiopia (Dercon & Christiaensen, Citation2011; McIntosh et al., Citation2013). Recurrent drought, hail, pests, and erratic rainfall create uncertainty in the farmers’ adoption and investment in agronomic technologies and inputs (Adimassu, Citation2019; Ceballos et al., Citation2019). Weather index crop insurance (WICI) schemes may help to avoid/reduce uncertainties, compensate for weather-induced damage, and cope with adverse effects of weather risks (Anghileri et al., Citation2022; Tadesse et al., Citation2015). The smallholder farmers’ high-risk aversion behaviour hampers the adoption of improved agronomy technologies (Kathage et al., Citation2016; Sibiko & Qaim, Citation2020). From this, we can imagine that WICI coverage can increase smallholder farmers’ input use and food crop yield thus, improve their food security.
Studies advocated crop insurance is an effective mechanism of transferring weather induced-risks from the crop production chain to the insure(s) (Abugri et al., Citation2017; Haile et al., Citation2020; Jin et al., Citation2016) and building smallholders’ resilience against the vagaries of nature (Isaboke et al., Citation2016). A WICI scheme alleviates smallholders’ high-risk aversion behaviour-embarks their confidence: and augments crop yield expectations(Haile et al., Citation2020). Thus, farmers invest more in agronomy technologies, switch to more adaptive high-yield crop varieties, and ultimately bring them stable higher incomes (Abugri et al., Citation2017; Fu et al., Citation2018; Raucci et al., Citation2019). Abugri et al. (Citation2017) and Gebrehiwot (Citation2015) indicated crop insurance raises the confidence of micro-financial institutions in lending farm inputs to farmers.
From the theoretical point of view, (Carter et al., Citation2016) shed light on how index insurance can boost the adoption of improved agronomic technologies. They argued that technology adoption without crop insurance coverage is low: risk-rationed farmers fear taking credit for agronomic technologies and choose to use traditional technology. Besides, studies conducted by (Carter et al., Citation2016; Farrin & Miranda, Citation2015; Gebrehiwot, Citation2015; Sibiko & Qaim, Citation2020) indicate weather-index insurance coverage for smallholder farmers associated with increased use of artificial fertilizers and improved seeds. Moreover, (Shi & Jiang, Citation2016; Sibiko & Qaim, Citation2020) revealed crop insurance increases crop yields. However, it may be a surprise to state that there is a shortage of literature on the impacts of weather-index crop insurance on smallholder farmers’ multidimensional food security. In Ethiopia, crop insurance products are at the pilot project stage that did not widely disseminate due to a lack of institutional arrangement, inadequate research outcomes, and farmers’ meager willingness to pay (Castellani et al., Citation2014; Eze et al., Citation2020). To address these presumable challenges, policymakers, donors, and other stakeholders are urging research and development institutions to make their research on climatic shocks mitigating strategies and disaster risk management schemes to ensure smallholder farmers’ food security (Ayinu et al., Citation2022; Lesk et al., Citation2016; Mishra et al., Citation2021).
Studies have criticized traditional indemnity-based crop insurance products as plagued by moral hazards, adverse selection problems, and high administrative costs (Carter et al., Citation2016; Castellani et al., Citation2014; Maize Index, Citation2011). Crop insurance products based on the weather index are promoted and rapidly expanded to alleviate the drawbacks of traditional crop insurance (Adiku et al., Citation2017). WICI is one of the index crop insurance products applicable in drought-prone areas. It protects vulnerable farmers against specified weather-induced shocks (Eze et al., Citation2020; Tadesse et al., Citation2015). It is interconnected with meteorological rainfall records of the insured area triggered at different growth stages of crops (Adiku et al., Citation2017; Jin et al., Citation2016). It uses remote sensing to retrieve rainfall data from a satellite to substitute/supplement data collected from rainfall stations. It is cost-effective and has freely available high-quality data: an autonomous and tamper-proof database. Thus, it avoids moral hazards and adverse selection problems. It makes payouts when the actual rainfall index outstrips/drops below the pre-specified threshold (Adiku et al., Citation2017; Stutley, Citation2010; Vroege et al., Citation2019).
The study conducted in Ethiopia: Tigray region as WICI based on a rural resilience initiative has been implemented by the Horn of Africa Risk Transfer and Adaptation Project (HARITA) since 2009. It is a pilot project supported by Oxfam America (OA), local and international organizations such as the International Climate Research Institute (IRI) at Columbia University, Swiss Re, and Nyala Insurance Company (Eze et al., Citation2020; Tadesse et al., Citation2015). The project integrates four risk management strategies: risk reduction, risk transfer, risk-taking, and risk reserves (Madajewicz et al., Citation2017; Spiegel & Satterthwaite, Citation2013). We conducted the study to answer the research questions: is there any significant discrepancy between the insured and non-insured smallholder farmers’ food security? Did weather-index crop insurance products impact the smallholder farmers’ multidimensional food security? To address these, we employ an impact evaluation model that includes suite indicators for each food security dimension, the Foster-Greer-Thorbecke index for analyzing food security indices, and the propensity matching technique with the logit model to examine the impact. The study offers a prototypical diagnostic tool for further studies and urges eradicating smallholders’ chronic food insecurity requires devoted action on alleviating their uncertainties, risk aversion behaviour, and improving access to weather-induced risks management and transfering schemes, agricultural inputs/technologies, and other facilities.
2. Research methodology
2.1. Data type, source, and methods of data collection
The study employs a multistage random sampling method to select study districts and respondent households. We deliberately select the study region and districts because they are highly susceptible to drought and land degradation with a high incidence of food insecurity (Asfaw et al., Citation2017; Mohamed, Citation2017). To avoid bias, we randomly select three districts from the 18 districts of the zone. To effectively assess the impact, we used the list of farm-households living in the sampled districts as the sampling frame by stratifying into two (i) treated groups: farmers who got WICI insurance coverage at least for five successive years and (ii) control groups: those who had never got insurance coverage. Following (Adam, Citation2020; Israel, Citation1992), we used Yamane’s (Citation1967) sampling formulae to calculate the representative sample size of 353. Eventually, we used a systematic random sampling technique proportionate to the size of treated and control groups to select 120 treated (30 female-headed) and 233 control (38 female-headed) households. For maximum variability, we assume a 95% confidence level and a p-value of 0.05. Cross-sectional data were collected by a household survey using a structured questionnaire administrated by 10 (3 female) trained data enumerators. Moreover, we retrieved data from secondary sources like project documents, relevant published articles, and books.
2.2. Econometric model specification
2.2.1. Modelling multidimensional food security analysis
Despite the multifaceted concepts and different definitions of food security, scholars seem to agree on the 1996 World Food Summit. We adopt the following in this study: ‘Food security exists when all people, at all times, have physical, social and economic access to sufficient, safe and nutritious food which meets their dietary needs and food preferences for an active and healthy life’ (Skoet & Stamoulis, Citation2006). It has four dimensions: availability, access, utilization, and stability (Skoet & Stamoulis, Citation2006; Magrini & Vigani, Citation2016). The measurement of food security is difficult for many reasons. Thus, there is no single, internationally accepted rule on what data to collect and how to analyze that data (Carletto et al., Citation2013; Lele et al., Citation2016). Different authors and organizations have suggested systematically examining it using suit indicators to capture its multidimensional realities (see Leroy et al., Citation2015; Moroda et al., Citation2018). Following these suggestions, we used the most recently recommended indicators of multidimensional food security:
Analysis of food availability: food availability is the first dimension of food security that refers to the physical existence of food in an adequate amount and appropriate quality for consumption. It may come from production, purchases, or food aid (Carletto et al., Citation2013; FAO, Citation1996). It is an essential but not satisfactory condition to realize households’ food security. We focus on the FAO methodology of examining food availability through the household’s per capita dietary energy supply per adult equivalent (HDES/day/AE) (Carletto et al., Citation2013). Indeed, we used food-crop yield as the farm households’ food mainly comes from their production suggested by (Jones et al., Citation2013).
Analysis of food access: food access is the capability to obtain sufficient food of guaranteed quality to meet the nutritional requirements of all household members. Smallholder farmers’ access to food depends on their purchasing power and nonmarket entitlements: rights to cultivable land, remittance, and food aid. Farm-households’ yearly total food expenditure per adult equivalent (HFEAE) assessed their capability to obtain sufficient food. However, the HFEAE does not investigate the households’ hidden hunger problem or micronutrient(s) deficiency (Lele et al., Citation2016). To overcome this problem, we follow (Hussein et al., Citation2018; Jones et al., Citation2013) while incorporating the households’ dietary diversity score (HDDS) as the proxy measure of households’ access to a balanced diet or nutritional security.
HDDS is a count of food groups a particular respondent household has consumed over the past 24 h of the assessment time (Carletto et al., Citation2013; Hussein et al., Citation2018). Although there is no universal consensus on the number and food types to include in the HDDS (FAO, Citation2010; Kennedy et al., Citation2010), the FAO guideline adopted in this study with the following 12 food groups: cereals, root and tubers; vegetables; leafy vegetables; fruits; meat, poultry, offal; eggs; fish; pulses/legumes/nuts; milk and milk products; oil/fats; and sugar/honey (FAO, Citation2010; Moroda et al., Citation2018). If a food group was part of the household’s dish, it scored 1, otherwise 0. The HDDS value of given household ranges from 0 to 12; the lowest value indicates chronic food insecurity and vice versa.
iii. Analysis of food utilization: food utilization refers to the households’ ability to prepare and consume healthful meals(Calloway et al., Citation2023); ingestion and digestion of food that determines nutritional well-being and food conversion efficiency by metabolic process (Carletto et al., Citation2013). Following (Berry et al., Citation2015), we used proxy measures: the households’ score on access and utilization of sanitary facilities like access to safe drinking water; private toilets; hand washing facilities; solid and liquid waste disposal, and separation of households’ residence room from their animal yard.
iv. Analysis of food stability: food stability is the ability to sustainably meet dietary needs over time without risks of losing access to food due to climatic hazards (Wineman, Citation2016). Different authors used different indicators such as the variability of per capita food supply (see Berry et al., Citation2015; Lele et al., Citation2016), households’ vulnerability to food insecurity (see (Magrini & Vigani, Citation2016)), and coping strategy index (see Carletto et al., Citation2013). Following (Van der Geest & Warner, Citation2015), we used the methodology presented in (Annex) to measure the household’s vulnerability to food insecurity. This method has ten indicators, each with five category thresholds. To facilitate interpretations of results, the functional form of the vulnerability score changed into a logarithmic form. Moreover, we adopted the variability in per capita food supply as an indicator of food stability measured by the households’ food deficit periods over the last 12 months of the assessment.
v. Analyzing indices of food security: as described in the equations from 1 to 4 below, the study uses the FGT index to measure the incidence, depth, and severity of food insecurity of smallholder farmers.
(1)
(1)
To precisely analyze the food security of respondents, the study applied the expenditure approach based on the cost of basic needs (CBN) to establish the food poverty line in the study area. This method enables estimating the amount of money per adult equivalent could buy a basket of food items to meet the minimum nutritional threshold for a healthy and active life. The food security line in Ethiopia is 2200 kcal/adult equivalent/day required for an active and healthy life.
2.2.2. Modeling the impact of WICI on food security
This study is a non-experimental research design: thus, we used propensity matching (PSM) techniques to analyze the impact. In this type of research design, using the PSM has many advantages (see details in Magrini & Vigani, Citation2016). As defined in Equation 5, numerous explanatory variables affected the farmers’ decision to uptake WICI. Respondents were self-selected to participate in WICI: no random assignment was done to treated and control groups by this study. Thus, impact evaluation requires a selection bias correcting method (Cobb-Clark & Crossley, Citation2003). A method of correcting bias and isolating the treatment effect is comparing the matched treated and control groups’ outcomes (Becker & Ichino, Citation2002; Hirko et al., Citation2018). We define the treatment (T) as a binary variable equal to 1 if the farmer is insured in WICI and otherwise zero. Y (1) and Y (0) denote the outcome variables of the insured and non-insured farmers. These outcome variables are the suit indicators of multidimensional food security reported in . Further, as described in EquationEquation 6(6)
(6) below, we apply the standard methodology proposed by (Rosenbaum & Rubin, Citation1983) while specifying the propensity score matching (PSM) model to analyze the impact by using the average treatment effect of WICI on the Treated (ATT).
(5)
(5)
(6)
(6) ATT is the difference between the expected outcomes of the treated and control groups (Osarfo et al., Citation2016; Zhang et al., Citation2019). Here, it is a difference in the food security indicators with and without WICI for insured farmers. We can observe the covariates (Xi) influencing the decision of farmers to uptake WICI and the outcomes of the insured/treated farmers: (E[Y (1) |T = 1]). But unobservable variables may simultaneously affect both the decision of farmers to uptake WICI and the outcomes of those insured had they not insured: (E[Y (0) |T = 1]). Thus, ‘the hidden bias’ that reduces the robustness of ATT outcomes might arise. As suggested (Becker & Caliendo, Citation2007; Rosenbaum, Citation2002a), we address the problems by satisfying the conditional independence assumption (CIA): fulfillment of the balancing hypothesis before estimating ATT and bounding method after ATT estimation. The study has continuous outcome variables. Hence, we used Rosenbaum bounds (rbounds) to check hidden bias.
Empirical literature applied different matching algorithms to ensure the ‘similarity’ between treatment and control groups. We used the two-step PSM method because it reduces the conditional dimensionality problem by matching households with an identical probability to participate in WICI without controlling covariate variables in a vector Xi (Mendola, Citation2007). First, we estimate the conditional probability of each household participating in WICI by logistic regression model: P (Xi) = Pr(T = 1│Xi) = E(T|Xi). Secondly, we computed ATT as:
(7)
(7) From the different matching techniques, we focused on the nearest-neighbor matching with three matches: (ATT-NNM (3)), 0.25 caliper, and imposing common support while estimating the ATT. Next, we used the radius matching at 0.25 calipers (ATT-RM) to guarantee the quality of ATT-NNM outcomes. To maintain good matching quality and satisfy the CIA assumption, we examined the fulfillment of the balancing hypotheses based on the standardized bias approach suggested by (Rosenbaum & Rubin, Citation1985). It includes ex-ante and ex-post matching inspections on the differences between the covariates of insured/treated and non-insured/control farmers. Moreover, as indicated by (Magrini & Vigani, Citation2016), we re-estimated the propensity score on the matched sample to confirm if the pseudo-R2 after matching is very low and test the joint significance of all explanatory variables by a likelihood ratio test.
3. Results and discussion
3.1. Descriptive analysis
The study results in indicate 77.9% and 22.1% of farm households in the study area are male-headed and female-headed. Although both male and female couples have equal rights to access and control the household’s assets, a cultural tradition in the study area empowers males as the household leader and manages their main assets. They reap 66.86% of their income from subsistence agriculture. To cope with the drought hazards, 32.29% of households have diversified their income sources to non-farm activities because non-farm activities are less susceptible to idiosyncratic and covariate shocks than farming activities (Asfaw et al., Citation2017). In the study area, the mean land holding of smallholder farmers is 0.52 hectares per household. Crop production is low-input: uses an average of 56.64 kgs/ha artificial fertilizer and 13.24 kgs/ha improved seed, and low-output with a mean crop yield of 11.23 quintals/hectare. Consequently, agricultural production is inadequate: this exposed 40.79% of households in the study area to an average of 2.6 months of cyclical food deficit per annum.
Table 1. Statistical difference tests of treated and control groups.
Generally, we found significant differences in covariates between insured and non-insured farmers. This condition might influence the participation of farmers in WICI. The continuous explanatory variables that show statistically significant differences include the size of cultivated land owned, livestock assets, dependency rate, household size, and household head’s education. It implies that there could be a possibility of bias if ATT had computed without correcting the bias. Furthermore, reports significant differences in tested outcome variables like the quantity of inorganic fertilizer utilized, the amount of improved seed used, average crop yield, and food expenditure per adult equivalent. The average amount of inorganic fertilizer utilization is 56.79kgs/ha, far below the region’s standard of 200kgs/ha, and the mean amount of improved seed used in the study area is 13.24kgs/ha. The result shows a significant difference in the amount of fertilizer and improved seeds used between the insured and non-insured farmers.
3.2. Correlation analysis between WICI participation and food gap months
The existence of a strong correlation between food deficit and smallholder farmers’ participation in WICI is a necessary condition for cause and effect or impact analysis on food security. As illustrated in above, the percentage of non-insured smallholders increased from 1.288% to 13.305% as annual food deficit months increased from 1 to 4. Simultaneously, the insured smallholder farmers decreased from 12.5% to 0% as the number of food deficit months per annum increased from 1 to 5. It implies smallholder farmers’ participation in WICI and food security have a statistically significant (Pr (χ2) = 0.000) strong positive correlation and vice versa.
3.3. Econometric analysis
3.3.1. Estimating propensity scores and balancing property
reports the logistic regression results for propensity scores of smallholder farmers’ participation in WICI. All except the household head’s age and size of cultivable land owned are statistically significant explanatory variables influencing the uptake of WICI in the study area. The probability of participation in WICI increases with the household head’s education, livestock asset, household size, and access to relevant information by radio. Conversely, the probability reduces with female-headed households, the dependency rate, and the number of income sources. We used the propensity scores to match insured/treated and non-insured/control households. Firstly, we guaranteed sufficient overlaps in the propensity score distributions between insured and non-insured farmers. Hence, the common support area is [0.0552, 0.9273], with 24 observations left outside. Further, we verified the balancing property to eliminate pre-treatment differences in the covariates between insured and non-insured farmers. It indicates the satisfaction of the CIA given the propensity scores that there exists a set of Xi covariates such that controlling these variables result in the ATT outcomes that are autonomous from the treatment status (Becker & Ichino, Citation2002; Hirko et al., Citation2018).
Table 2. Logit estimates of propensity scores.
3.3.2. Estimating the impact of WICI on multidimensional food security
indicates the removal of significant differences between the covariates in the unmatched sample following the matching technique except for the household head’s gender and age. As a result, the mean absolute bias decreased from 32.4% to 9.3%, while ‘absolute’ bias reductions ranged [from 63.9% to 84%]. The entire standardized bias of each covariate is below the 20% threshold implies an acceptable balance for impact analysis. Ultimately, the joint significance of the covariates based on the pseudo-R2 test and the likelihood ratio test approved after matching that there are no systematic differences between the insured and non-insured farmers. The value of the pseudo-R2 varies from 0.203 to 0.018. After matching, the p-value of the likelihood ratio test fails to reject the null hypothesis: all the coefficients of covariates are equal to zero.
Table 3. Balancing property of covariates.
For better justification of results, first, we estimate the impact of WICI on adopting the agricultural inputs for crop production: the quantity of inorganic fertilizer and improved seed. As discussed in the background section, crop insurance has to solve the farmers’ high-risk aversion to invest and adopt improved inputs affecting food crop production. According to the results reported in (Annex), WICI has a statistically significant positive impact on the amount of inorganic fertilizer and improved seed uptake. It is associated with a 29% increase in the quantity of inorganic fertilizer utilization, a 24% increase in the adoption of improved seeds, and a 19% increase in food-crop yield. These results are consistent with the findings (Sibiko & Qaim, Citation2020) and (Gebrehiwot, Citation2015).
indicates the impacts of WICI on multidimensional food security using different matching techniques. Specifically, we focus on ATT-NNM (3) at 0.25calipers and ATT-RM at 0.25 calipers, and we impose the common support in both methods to obtain better quality results. Indicators of each pillar show WICI has a positive and significant impact on food security. From the food availability dimension point of view, uptake of WICI increases food-crop yield ranging [15.54%, 18.90%] and [216, 238 kcal/adult] more per capita daily dietary energy supply. The study’s findings are consistent with (Sibiko & Qaim, Citation2020): uptake of crop insurance is associated with 62% higher maize yields. However, we focused on the impacts on staple food crops of the study area rather than on a single crop.
Table 4. Treatment effects and sensitivity analysis with matching techniques.
The access dimension of food security was also positively affected by WICI. The uptake of WICI increases the farm households’ HFEA in the range of [707.34, 775.36 ETB]. Furthermore, the HDDS reports that uptake of WICI guarantees an 8.9% more diversified and balanced nutrient intake. Respondent farmers asserted that crop insurance coverage makes them less vulnerable to weather-induced hazards that increase their propensity to spend on a more diversified diet. also indicates that insured smallholder farmers have better access and utilization of sanitary facilities like a private toilet, hand washing, solid waste disposal, liquid waste disposal, and separation of household’s residence room(s) from their livestock barn. The result shows uptake of WICI improves households’ access and utilization of health care and sanitary facilities in the range of [30%, 35%] is a positive impact [at 1%, t = 7.1]. Indirectly, it implies the uptake of WICI boosts households’ investment in health care and basic sanitary facilities that improve food utilization. Simplifying the interpretation of the percentage differences in the households’ vulnerability index, the functional form of its score changed into a logarithmic form. reports uptake of WICI reduces a household’s vulnerability to food insecurity by 10%, implying that insured farmers have a lower likelihood of future food deficits and better capability to withstand moderate shocks.
Moreover, shows WICI has a statistically significant positive impact on food stability [at 1%, t = −2.41]: reducing their cyclical food deficit by two months. In , we also report the significance level of hidden bias (γ), which shows the effect of unabsorbed variables on the conditional probability of participating in WICI and the validity of ATT results. The hidden bias of all food security indicators used is zero: (γ = 0.00). It ensures the study results are free of hidden bias showing the observable variables [Xi] included in the model are the sole determinants of farmers’ participation in WICI(Becker & Caliendo, Citation2007; Rosenbaum, Citation2002b).
To obtain more robust results, we further analyzed the differences in the food security indices of insured and non-insured farmers. The local food security line of 10263.28 Birr/adult equivalent/year was established using the purchasing cost of the daily nutritional threshold of 2200 kcal/adult necessary for an active and healthy life in Ethiopia (Kibret, Citation2020; Mohamed, Citation2017). reports that 37.77% of the non-insured farmers, 28.33% of the insured farmers, and 34.56% of the study population fall below the food-security line and are food insecure. Comparatively, insured farmers show lower incidence: [by 9.44%], depth: [by 3.24%], and severity: [by 1.06%] of food insecurity than non-insured. It implies, on average, each adult person in non-insured households is $US 20.52 more below the food-security line than the insured, and the inequality among the non-insured food-insecure households is more severe.
Table 5. Food insecurity indices of insured and non-insured farmers.
4. Conclusion and policy implications
Under unpredictable rainfall conditions: drought, hail, and flood risks curtail smallholder farmers’ investment in improved agronomy technologies and expose them to food insecurity (Mesfin et al., Citation2022; Tofu et al., Citation2022). Without alleviating the high-risk aversion behaviours, smallholder farmers are not producing enough food even during good rainfall seasons because these hamper the uptake of agronomic technologies and inputs which endangered crop production (Kathage et al., Citation2016; Sibiko & Qaim, Citation2020), and are prone to food insecurity. Thus, we advocate extending WICI coverage as a strategy for transferring such potential risks from the smallholder farmers to insure(s).
Given that smallholder crop production is highly vulnerable to weather risks, WICI directly alleviates risk aversion behaviours and boosts crop yield expectations(Haile et al., Citation2020). These outcomes enhance the use of artificial fertilizer, improved seeds, and other technologies adoption that increase crop yield (Haile et al., Citation2020; Raucci et al., Citation2019). The more risk-averse the farmer, the greater the probability of taking WICI products. Ceteris paribus, the differences in the adoption of WICI result in differences in the uptake of agronomy technologies, crop yield, and multidimensional food security indicators. Generally, results indicate WICI has significant positive impacts on smallholder farmers’ availability, access, utilization, and food stability. It is associated with a 29% increased use of artificial fertilizer, a 24% increase in improved seed adoption, and a 19% increase in food-crop yield. Furthermore, it increases the daily per caput dietary energy supply insured farmers ranging [216, 238 kcal]; adult equivalent’s food expenditure in the range [707.34, 775.36 Birr]; nutritional diversity by [8.9%, 10%]; improves proper health care and food utilization ranged [30%, 35%] and it reduces vulnerability to food insecurity by 10%.
Crop insurance is in its infant stage in Ethiopia and is a pilot project in the study area(Eze et al., Citation2020; Tadesse et al., Citation2015). Therefore, to realize considerable progress in smallholder farmers’ food security, the WICI programme needs to be sufficiently expanded and tailored to the priorities and preferences of smallholder farmers. The existing donor-driven pilot crop insurance projects should be used as a milestone to establish a comprehensive national agricultural insurance programme. Besides, the Ethiopian government, planners, practitioners, and stakeholders shall play a pivotal role in creating a credible weather database system, capacitating domestic reinsurers, and enhancing farmers’ awareness as part of the agricultural extension service. Weather-induced risks like drought, hail, and flood are systemic risks that can simultaneously affect millions of farmers, prone to enormous liability beyond the financial capacity of the currently operating private reinsurers, and might knock down their financial solvency. Therefore, it is more advisable for the government, NGOs, and other stakeholders actively engage in the system.
Supplemental Material
Download MS Word (30.8 KB)Acknowledgments
We are grateful to Adigrat University for its financial support while conducting the study. Indeed, the respondents, editors, reviewers who devoted their insights and knowledge, and all other secondary data sources used are also duly acknowledged.
Disclosure statement
No potential conflict of interest was reported by the author(s).
Additional information
Funding
Notes on contributors

Yohannes Halefom Gebretsadik
Yohannes Halefom Gebretsadik is a Lecturer and Researcher at Adigrat University, Department of Agricultural Economics, Ethiopia. He obtained Bachelor's in Economics from Alpha University College and MSc in Development Economics from Aksum University. He teaches undergraduate courses including macroeconomics, international trade, food security & agricultural policy, project planning & management, and farm management. Yohannes has authored two types of research: the impact of nonfarm activities on farmers' income poverty and rural livelihood diversification and poverty. Since 2005, he has worked in Ethiopia in a wide range of fields: agricultural extension, he was M&E specialist in various development programs-household asset building programs, rural livelihood improvement programs and manager of food and nutritional security program.

Kahsay Gebru Tesfay
Kahsay Gebru Tesfay is a graduate assistant at Adigrat University Department of Agricultural Economics, Tigray Region, Ethiopia. He was born in Ethiopia, and obtained his Bachelor's in Agricultural Economics from Aksum University. He teaches undergraduate courses: Natural Resource and Environmental Economics, Entrepreneurship and Small Scale Business Management and Farming System and Livelihood Analysis.
References
- Abugri, S. A., Amikuzuno, J., & Daadi, E. B. (2017). Looking out for a better mitigation strategy: Smallholder farmers’ willingness to pay for drought-index crop insurance premium in the Northern Region of Ghana. Agriculture & Food Security, 6(1), 71. https://doi.org/10.1186/s40066-017-0152-2
- Adam, A. M. (2020). Sample size determination in survey research. Journal of Scientific Research and Reports, 26(5), 90–97. https://doi.org/10.9734/jsrr/2020/v26i530263
- Adiku, S. G., Debrah-Afanyede, E., Greatrex, H., Zougmoré, R. B., & MacCarthy, D. S. (2017). Weather-index based crop insurance as a social adaptation to climate change and variability in the Upper West Region of Ghana: developing a participatory approach. Retrieved from Copenhagen, Denmark. www.ccafs.cgiar.org.
- Adimassu, Z. (2019). Constraining the constraints: Factors affecting farmers’ investment in climate-smart land management. In K. M. B. Hadgu, M. Iiyama, E. Birhane, A. Negussie, C. M. Davis, & B. Bernart (Eds.), Climate-Smart agriculture centre (pp. 183–193). World Agroforestry (ICRAF).
- Alemayehu, A., & Bewket, W. (2016, July). Local climate variability and crop production in the central highlands of Ethiopia. Environmental Development, 19, 36–48. https://doi.org/10.1016/j.envdev.2016.06.002
- Alemu, T., & Mengistu, A. (2019). Impacts of climate change on food security in Ethiopia: Adaptation and mitigation options: A review. In Climate change-resilient agriculture and agroforestry: Ecosystem services and sustainability (pp. 397–412). First Online: January 25, 2019. https://doi.org/10.1007/978-3-319-75004-0_23
- Anghileri, D., Bozzini, V., Molnar, P., Jamali, A. A., & Sheffield, J. (2022). Comparison of hydrological and vegetation remote sensing datasets as proxies for rainfed maize yield in Malawi. Agricultural Water Management, 262, 107375. https://doi.org/10.1016/j.agwat.2021.107375
- Asfaw, A., Simane, B., Hassen, A., & Bantider, A. (2017). Determinants of non-farm livelihood diversification: Evidence from rainfed-dependent smallholder farmers in northcentral Ethiopia (Woleka sub-basin). Development Studies Research, 4(1), 22–36. https://doi.org/10.1080/21665095.2017.1413411
- Asresie, A., & Zemedu, L. (2015). The contribution of the livestock sector to the Ethiopian economy. A Review. Advances in Life Science and Technology, 29(online), 79–90.
- Ayinu, Y. T., Ayal, D. Y., Zeleke, T. T., & Beketie, K. T. (2022, August). Impact of climate variability on household food security in Godere District, Gambella Region, Ethiopia. Climate Services, 27, 100307. https://doi.org/10.1016/j.cliser.2022.100307
- Becker, S. O., & Caliendo, M. (2007). Sensitivity analysis for average treatment effects. The Stata Journal: Promoting Communications on Statistics and Stata, 7(1), 71–83. https://doi.org/10.1177/1536867X0700700104
- Becker, S. O., & Ichino, A. (2002). Estimation of average treatment effects based on propensity scores. The Stata Journal: Promoting Communications on Statistics and Stata, 2(4), 358–377. https://doi.org/10.1177/1536867X0200200403
- Belay, A., Recha, J. W., Woldeamanuel, T., & Morton, J. F. (2017). Smallholder farmers’ adaptation to climate change and determinants of their adaptation decisions in the Central Rift Valley of Ethiopia. Agriculture & Food Security, 6(1), 1–13. https://doi.org/10.1186/s40066-017-0100-1
- Berry, E. M., Dernini, S., Burlingame, B., Meybeck, A., & Conforti, P. (2015). Food security and sustainability: Can one exist without the other? Public Health Nutrition, 18(13), 2293-2302. https://doi.org/10.1017/S136898001500021X
- Calloway, E. E., Carpenter, L. R., Gargano, T., Sharp, J. L., & Yaroch, A. L. (2023). New measures to assess the “Other” three pillars of food security–availability, utilization, and stability. International Journal of Behavioral Nutrition and Physical Activity, 20(1), 51. https://doi.org/10.1186/s12966-023-01451-z
- Carletto, C., Zezza, A., & Banerjee, R. (2013). Towards the better measurement of household food security: Harmonizing indicators and the role of household surveys. Global Food Security, 2(1), 30–40. https://doi.org/10.1016/j.gfs.2012.11.006
- Carter, M. R., Cheng, L., & Sarris, A. (2016, January). Where and how index insurance can boost the adoption of improved agricultural technologies. Journal of Development Economics, 118, 59–71. https://doi.org/10.1016/j.jdeveco.2015.08.008
- Castellani, D., Vigano, L., & Tamre, B. (2014). A discrete choice analysis of smallholder farmers’ preferences and willingness to pay for weather derivatives: Evidence from Ethiopia. Journal of Applied Business Research (JABR), 30(6), 1671–1692. https://doi.org/10.19030/jabr.v30i6.8882
- Ceballos, F., Kramer, B., & Robles, M. (2019). The feasibility of picture-based insurance (PBI): Smartphone pictures for affordable crop insurance. Development Engineering, 4, 100042. https://doi.org/10.1016/j.deveng.2019.100042
- Cobb-Clark, D. A., & Crossley, T. (2003). Econometrics for evaluations: An introduction to recent developments. Economic Record, 79(247), 491–511. https://doi.org/10.1111/j.1475-4932.2003.00148.x
- Daryanto, S., Wang, L., & Jacinthe, P.-A. (2016). Global synthesis of drought effects on maize and wheat production. PloS One, 11(5), e0156362. https://doi.org/10.1371/journal.pone.0156362
- Debela, M. F., & Mohammed, A. F. (2020). The role of urban agriculture in improving the livelihood of the urban poor and the challenges: The case of hawassa city administration, SNNPRS, Ethiopia. Journal of Economics and Sustainable Development, 11(1), 1–12. https://doi.org/10.7176/JESD/11-1-01
- Dercon, S., & Christiaensen, L. (2011). Consumption risk, technology adoption, and poverty traps: Evidence from Ethiopia. Journal of Development Economics, 96(2), 159–173. https://doi.org/10.1016/j.jdeveco.2010.08.003
- Eze, E., Girma, A., Zenebe, A. A., & Zenebe, G. (2020). Feasible crop insurance indexes for drought risk management in Northern Ethiopia. International Journal of Disaster Risk Reduction, 47, 101544. https://doi.org/10.1016/j.ijdrr.2020.101544
- FAO. (1996, 13-17 November). Rome declaration on world food security and world food summit plan of action. Paper presented at the World Food Summit, Rome, Italy.
- FAO. (2010). Guidelines for measuring household and individual dietary diversity. FAO.
- Farrin, K., & Miranda, M. J. (2015). A heterogeneous agent model of credit-linked index insurance and farm technology adoption. Journal of Development Economics, 116(2015), 199–211. https://doi.org/10.1016/j.jdeveco.2015.05.001
- Fu, H., Li, J., Li, Y., Huang, S., & Sun, X. (2018). Risk transfer mechanism for agricultural products supply chain based on weather index insurance. Complexity, 2018(Article ID 2369423), 7. https://doi.org/10.1155/2018/2369423
- Gebrehiwot, D. B. (2015). Impact of weather index insurance on household demand for fertilizer in Tigray region. Ethiopian Journal of Business and Economics (The), 5(1). https://doi.org/10.4314/ejbe.v5i1.4
- Gebrtetsadik, Y. H. (2022). Impact of rural, non-farm activities on smallholder farmers’ income-poverty in Eastern zone of Tigray Region, Ethiopia. African Journal of Science, Technology, Innovation and Development, 14(2), 504–511. https://doi.org/10.1080/20421338.2020.1857543
- Haile, K. K., Nillesen, E., & Tirivayi, N. (2020, June). Impact of formal climate risk transfer mechanisms on risk-aversion: Empirical evidence from rural Ethiopia. World Development, 130, 104930. https://doi.org/10.1016/j.worlddev.2020.104930
- Hill, R. V., & Porter, C. (2017, August). Vulnerability to drought and food price shocks. Evidence from Ethiopia, 96, 65–77. https://doi.org/10.1016/j.worlddev.2017.02.025
- Hirko, T., Ketema, M., & Beyene, F. (2018). Evaluating the impact of small-scale irrigation practice on household income in abay chomen district of oromia national regional state, Ethiopia. Journal of Development and Agricultural Economics, 10(12), 384–393. https://doi.org/10.5897/JDAE2018.0992
- Hussein, F. M., Ahmed, A. Y., & Muhammed, O. S. (2018). Household food insecurity access scale and dietary diversity score as a proxy indicator of nutritional status among people living with HIV/AIDS, Bahir Dar, Ethiopia, 2017. PloS One, 13(6), e0199511. https://doi.org/10.1371/journal.pone.0199511
- Isaboke, H. N., Zhang, Q., & Nyarindo, W. N. (2016). The effect of weather index-based micro-insurance on the food security status of smallholders. Agricultural and Resource Economics: International Scientific E-Journal, 2(3), 5–21. https://doi.org/10.51599/are.2016.02.03.01
- Israel, G. D. (1992). Sampling the evidence of extension program impact. University of Florida.
- Jin, J., Wang, W., & Wang, X. (2016). Farmers’ risk preferences and agricultural weather index insurance uptake in rural China. International Journal of Disaster Risk Science, 7(4), 366–373. https://doi.org/10.1007/s13753-016-0108-3
- Jones, A. D., Ngure, F. M., Pelto, G., & Young, S. L. (2013). What are we assessing when we measure food security? A compendium and review of current metrics. Advances in Nutrition, 4(5), 481–505. https://doi.org/10.3945/an.113.004119
- Kathage, J., Kassie, M., Shiferaw, B., & Qaim, M. (2016). Big constraints or small returns? Explaining non-adoption of hybrid maize in Tanzania. Applied Economic Perspectives and Policy, 38(1), 113–131. https://doi.org/10.1093/aepp/ppv009
- Kennedy, G., Berardo, A., Papavero, C., Horjus, P., Ballard, T., Dop, M., Delbaere, J., & Brouwer, I. D. (2010). Proxy measures of household food consumption for food security assessment and surveillance: Comparison of the household dietary diversity and food consumption scores. Public Health Nutrition, 13(12), 2010–2018. https://doi.org/10.1017/S136898001000145X
- Kibret, B. G. (2020). Measuring rural poverty among rural households in Gedeo Zone, SNNP Region, Ethiopia. Journal of Development and Agricultural Economics, 12(1), 9–16. https://doi.org/10.5897/JDAE2019.1134
- Lele, U., Masters, W. A., Kinabo, J., Meenakshi, J., Ramaswami, B., Tagwireyi, J., & Goswami, S. (2016). Measuring food and nutrition security: An independent technical assessment and user's guide for existing indicators. Measuring food and nutrition security technical working group. Rome: Food Security Information Network. Retrieved from http://www.fsincop.net/topics/fnsmeasurement.
- Leroy, J. L., Ruel, M., Frongillo, E. A., Harris, J., & Ballard, T. J. (2015). Measuring the food access dimension of food security: A critical review and mapping of indicators. Food and Nutrition Bulletin, 36(2), 167–195. https://doi.org/10.1177/0379572115587274
- Lesk, C., Rowhani, P., & Ramankutty, N. (2016). Influence of extreme weather disasters on global crop production. Nature, 529(7584), 84–87. https://doi.org/10.1038/nature16467
- Madajewicz, M., Tsegay, A., & Lee, R. (2017). Managing risks in smallholder agriculture: The impacts of R4 on livelihoods in Tigray, Ethiopia from 2012 to 2016. Retrieved from http://p4arm.org/app/uploads/2018/07/R4_Ethiopia_Impact_Evaluation_Report_2012-2016_2yOZSeM.pdf
- Magrini, E., & Vigani, M. (2016). Technology adoption and the multiple dimensions of food security: The case of maize in Tanzania. Food Security, 8(4), 707–726. https://doi.org/10.1007/s12571-016-0593-7
- Maize Index, M. (2011). Weather index insurance for agriculture: Guidance for development practitioners. World Bank.
- Mbugua, M., Nzuma, J., & Muange, E. (2019). Social networks and ex-post risk management among smallholder farmers in Kenya. Development Studies Research, 6(1), 30–39. https://doi.org/10.1080/21665095.2019.1573149
- McIntosh, C., Sarris, A., & Papadopoulos, F. (2013). Productivity, credit, risk, and the demand for weather index insurance in smallholder agriculture in Ethiopia. Agricultural Economics, 44(4–5), 399–417. https://doi.org/10.1111/agec.12024
- Mendola, M. (2007). Agricultural technology adoption and poverty reduction: A propensity-score matching analysis for rural Bangladesh. Food Policy, 32(3), 372–393. https://doi.org/10.1016/j.foodpol.2006.07.003
- Mesfin, A. H., Gebremedhin, K. G., Birhan, Y. T., Yohannes, T., Weldeyesus, H., Tefera, T., Diro, M., Vonk, R., Confidence, D., Fikadu, D., & Teferi, N. (2022). Weather information and shocks: Policy implications to ensure food security in Tigray Region of Ethiopia. Journal of Arid Environments, 205, 104769. https://doi.org/10.1016/j.jaridenv.2022.104769
- Mishra, A., Ketelaar, J. W., Uphoff, N., & Whitten, M. (2021). Food security and climate-smart agriculture in the lower Mekong basin of Southeast Asia: Evaluating impacts of system of rice intensification with special reference to rainfed agriculture. International Journal of Agricultural Sustainability, 19(2), 152–174. https://doi.org/10.1080/14735903.2020.1866852
- Mohamed, A. A. (2017). The food security situation in Ethiopia: A review study. International Journal of Health Economics and Policy, 2(3), 86–96. https://doi.org/10.11648/j.hep.20170203.11
- Moroda, G. T., Tolossa, D., & Semie, N. (2018). Food insecurity of rural households in Boset district of Ethiopia: A suite of indicators analysis. Agriculture & Food Security, 7(1), 65. https://doi.org/10.1186/s40066-018-0217-x
- Orr, A., Tiba, Z., Congrave, J., Porázik, P., Dejen, A., & Hassen, S. (2021). Smallholder commercialization and climate change: A simulation game for teff in South Wollo, Ethiopia. International Journal of Agricultural Sustainability, 19(5–6), 595–608. https://doi.org/10.1080/14735903.2020.1792735
- Osarfo, D., Senadza, B., & Nketiah-Amponsah, E. (2016). The impact of nonfarm activities on Rural farm household income and food security in the Upper East and Upper West Regions of Ghana. Theoretical Economics Letters, 6(online), 388–400. https://doi.org/10.4236/tel.2016.63043
- Raucci, G. L., Lanna, R., da Silveira, F., & Capitani, D. H. D. (2019). Development of weather derivatives: Evidence from the Brazilian soybean market. Italian Review of Agricultural Economics, 74(2), 17–28. https://doi.org/10.13128/rea-10850
- Rosenbaum, P. R. (2002a). Overt bias in observational studies. New York, NY: Springer.
- Rosenbaum, P. R. (2002b). Sensitivity to hidden bias. In P. R. Rosenbaum (Ed.), Observational studies (2nd ed., pp. 105–170). New York: Springer.
- Rosenbaum, P. R., & Rubin, D. B. (1983). The central role of the propensity score in observational studies for causal effects. Biometrika, 70(1), 41–55. https://doi.org/10.1093/biomet/70.1.41
- Rosenbaum, P. R., & Rubin, D. B. (1985). Constructing a control group using multivariate matched sampling methods that incorporate the propensity score. The American Statistician, 39(1), 33–38. https://doi.org/10.1080/00031305.1985.10479383
- Senapati, A. K. (2020). Assessing the vulnerability of agricultural households to covariate and idiosyncratic shocks: A case study in Odisha, India. Climate and Development, 12(2), 183–197. https://doi.org/10.1080/17565529.2019.1611532
- Shi, H., & Jiang, Z. (2016). The efficiency of composite weather index insurance in hedging rice yield risk: Evidence from China. Agricultural Economics, 47(3), 319–328. https://doi.org/10.1111/agec.12232
- Sibiko, K. W., & Qaim, M. (2020). Weather index insurance, agricultural input use, and crop productivity in Kenya. Food Security, 12(1), 151–167. https://doi.org/10.1007/s12571-019-00987-y
- Skoet, J., & Stamoulis, K. G. (2006). The state of food insecurity in the world 2006: Eradicating world hunger-taking stock ten years after the world food summit. Rome, Italy: Food & Agriculture Org.
- Spiegel, A., & Satterthwaite, D. (2013). The R4 rural resilience initiative—Protecting livelihoods in a changing climate. The Geneva Association.
- Stutley, O. M. C. J. (2010). Government support to agricultural insurance: Challenges and options for developing countries. The World Bank.
- Tadese, M. T., Kumar, L., Koech, R., & Kogo, B. K. (2022). Perception of the impacts of climate and environmental variability on water availability, irrigation, and farming systems: A study in rural households of Awash River Basin, Ethiopia. International Journal of Agricultural Sustainability, 20(2), 231–246. https://doi.org/10.1080/14735903.2021.1930738
- Tadesse, M. A., Shiferaw, B. A., & Erenstein, O. (2015). Weather index insurance for managing drought risk in smallholder agriculture: Lessons and policy implications for sub-Saharan Africa. Agricultural and Food Economics, 3(1), 1–21. https://doi.org/10.1186/s40100-015-0044-3
- Tofu, D. A., Woldeamanuel, T., & Haile, F. (2022, June). Smallholder farmers’ vulnerability and adaptation to climate change induced shocks: The case of Northern Ethiopia highlands. Journal of Agriculture and Food Research, 8, 100312. https://doi.org/10.1016/j.jafr.2022.100312
- Van der Geest, K., & Warner, K. (2015). Vulnerability, coping, and loss and damage from climate events. In A. E. Collins, J. Samantha, B. Manyena, & J. Jayawickrama (Eds.), Hazards, risks, and disasters in society (pp. 121–144). Bonn, Germany: Elsevier.
- Vroege, W., Dalhaus, T., & Finger, R. (2019). Index insurances for grasslands – A review for Europe and North-America. Agricultural Systems, 168, 101–111. https://doi.org/10.1016/j.agsy.2018.10.009
- Wineman, A. (2016). Multidimensional household food security measurement in rural Zambia. Agrekon, 55(3), 278–301. https://doi.org/10.1080/03031853.2016.1211019
- Yamane, T. (1967). Elementary sampling theory (1st ed.). Englewoods Cliffs, NJ: Prentice Hall Inc.
- Zhang, Z., Kim, H. J., Lonjon, G., & Zhu, Y. (2019). Balance diagnostics after propensity score matching. Annals of Translational Medicine, 7(1), 16. doi:10.21037/atm.2018.12.10
Annex
Table A1. Household vulnerability to food insecurity suit indicators used.
2. Dependency ratio category threshold
3. Size of land owned category
4. Livestock asset-owned category
Livestock owned in tropical livestock units, conversion factors include-ox,1; cow, 1, calf, 0.25; heifer/bull, 0.75; sheep/goat, 0.13; lamb/kid, 0.06; camel, 1.25; horse 1.1; mule, 1.1; donkey, 0.7; young donkey, 0.35; and poultry, 0.0.1.
5. Annual Income generated from the non-farm income category
Exchange rate of US$1 = 33 ETB (deflated to the base year of 2020)
6. Livelihood diversification
Number of livelihood sources from the following list: rain-fed crop production, irrigation, livestock rearing, trees, farm labour, nonfarm income, remittances, pension, and rent.
7. Total income
Total cash income over the past 12 months, expressed in US$ at the exchange rate of US$1 = 33 ETB. We add income reaped from all sources mentioned under livelihood diversity to totalize income.
We apply an exchange rate of US$1 = 33 (deflated to the base year of 2020)
8. House Quality
9. Sanitary facilities
It depends on the household’s access to clean drinking water and toilet (private toilet with hand washing) facilities.
10. Food gap months category
It is based on the number of food shortage months in the last 12 months
Table A2. Impact of WICI on inorganic fertilizer, improved seeds utilization, and crop yield
The quantity of inorganic fertilizer used in quintal/hectare, improved seeds used in quintal/hectare, and average crop yield harvested in quintal/hectare are mean outcomes taken from the secondary data of 2016/2017to 2019/2021 cropping calendars and are changed to their logarithmic form to facilitate interpretation of impacts as a percentage of change.