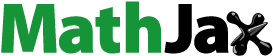
ABSTRACT
China is issuing a warning regarding the ‘unprecedented’ hurdles it faces in safeguarding its food security. The substantial conversion of farmland for non-grain cultivation is exacerbating the situation, necessitating urgent investigation into the underlying causes. Nonetheless, previous studies have revealed a dearth of village-level analysis and have been marred by a bias stemming from econometric methodologies. To remedy this, the present paper employs a sophisticated approach combining econometric modelling and machine learning techniques to unravel the intricate driving forces at the village level. The study yields three principal findings. Firstly, the availability of farmland, poverty incidence, and the age of village leaders exert a detrimental influence on non-grain use of farmland (NGUF). Secondly, a conglomerate of factors, such as the aging rural workforce, income levels, positively impact the planting of non-grain crops, with remarkably increasing effects. Finally, it is pivotal to acknowledge that the influencing factors display sensitivity to the spatial scale examined. Village-level research provides a comprehensive overview, transcending individual household decisions. Collectively, this study underscores the indispensability of incorporating the underlying principles into policy formulation. Township and county authorities should craft guidelines, policies, and regulations in accordance with the ascertained village-level drivers, rather than relying solely on household-level determinants.
1. Introduction
Global agriculture is currently facing unprecedented challenges (Huang & Tian, Citation2019; Veeck et al., Citation2020). While global food demand is projected to double by 2050 (Chen et al., Citation2014), there has been a slowdown in grain yield since the 1980s (Alston et al., Citation2009). This poses a significant concern for China, the world’s most populous country, as it faces steady population growth, a shift in consumption patterns, and increased uncertainty in the international food market. China’s food supply and demand will likely be closely balanced due to the rigid growth of total food consumption and the rising rate of grain imports. One of the major threats to China's food security is the considerable reduction in farmland and the hidden decline in grain production capacity (Wang et al., Citation2021; Ye et al., Citation2017). Reports and literature on the non-grain use of farmland have drawn attention to the shrinking grain sown area. Statistics from the China Statistical Yearbook indicate a decrease of 3,819 thousand hectares in the sown area for grain cultivation between 1978 and 2020, with the proportion of non-grain crops production increasing from 19.7% to 30.3%. This shift towards non-grain crops like fruit, tobacco, and herbs, referred to as ‘non-grain use of farmland (NGUF)’, has been spurred by increased investments in agriculture from social capital and land transfer (Ge et al., Citation2020). However, NGUF has led to various negative consequences, including heightened ecological pressures and national food insecurity, particularly in the post-pandemic era. Therefore, understanding the driving forces behind NGUF is vital for developing sustainable solutions for farmland management and addressing food insecurity.
The literature on the determinants of NGUF is extensive, attempting to uncover the factors behind this phenomenon. Various factors contribute to the preference for cash crops over grain crops. From a grain trade perspective, the increasing costs of grain production and the maintenance of low purchasing prices significantly influence farmers’ crop choices, favouring higher-profit cash crops (Kong, Citation2020; Zhu, Citation2016). Smallholding farmers are attracted to cash crops due to the low profitability of small-scale farming (Wu et al., Citation2021). Farmland size also plays a crucial role in crop selection, as grain crops require large land areas due to their land-intensive nature (Chen et al., Citation2009). Furthermore, labour-related factors such as off-farm employment and demographic aging have an impact on crop composition. Rural out-migration often reduces the likelihood of choosing labour-intensive crops (Luo & Qiu, Citation2018; Qian et al., Citation2018; Zhong et al., Citation2016). However, there is some controversy surrounding this factor. Rozelle et al. (Citation1999) investigated the relationship between rural-to-urban migration and grain production in China, finding that a 1% increase in migrant workers in rural households led to a net decrease of 14% in maize yield per unit of farmland area. Additionally, the aging farm labour force (He, Citation2010; Wang & Tian, Citation2018) and gender-related issues (Leng et al., Citation2020) are also influential. Moreover, the increasing investment of industrial and commercial capital in rural areas encourages the expansion of NGUF, driven by profit-oriented investments (Chen et al., Citation2021; Kong, Citation2020). Additionally, inadequate government support policies reduce farmers’ motivation for grain production (He & Quan, Citation2014). Studies based on survey data have also found that household characteristics, natural conditions of farmland parcels (such as landforms and soil conditions), farmland transfer, and the stability of farmland rental contracts have impacts on non-farm production (Bi et al., Citation2020; Chen et al., Citation2009; Liu & Zhang, Citation2020).
Studies on the factors influencing NGUF have examined three spatial scales. Macro-level research conducted at the national and provincial levels primarily focuses on the impacts of urbanization, industrialization, industry structure, and international and domestic grain prices on NGUF. At the middle-range spatial units, such as cities and counties, socio-economic factors like per capita GDP and per capita disposable income of urban residents (Chen et al., Citation2021; Liang et al., Citation2020; Su et al., Citation2020), resource endowments and farming conditions such as farmland acres and agricultural mechanization (Peng et al., Citation2020), demographic and social factors including population size, rural labour force, and urbanization rate (Qian et al., Citation2016; Yang et al., Citation2020), as well as policy-related factors like the area of transferred farmland and the number of agricultural cooperatives (Ma et al., Citation2015; Qiu et al., Citation2020; Yu & Huang, Citation2020), are investigated. The spatio-temporal dynamics of NGUF are analyzed using statistical data and remote sensing techniques (Sun et al., Citation2021; Xue & Zhang, Citation2017). Micro-scale studies delve into how demographic factors, farmland transfer, and farm size impact crop planting structure and farmers’ preferences using both qualitative and quantitative analyses (Luo et al., Citation2018; Zhang & Du, Citation2015). The predominant methodological approach in existing studies is econometric analysis, although a minority of research has employed qualitative case studies to investigate the impacts of difficult-to-quantify factors like social capital investment on NGUF.
There is a notable dearth of village-level studies examining the driving factors behind NGUF. In Chinese agricultural production, village collectives assume a pivotal and determinative role. Since 1955, collective ownership and unified operation of rural land have been implemented, with farmers working collectively in production teams known as ‘Shengchandui’, which serve as the minimum units responsible for land ownership. Following the reform and opening-up, these production teams evolved into village collective economic organizations (Ho, Citation2005). Although the household responsibility system granted farmers relative autonomy in land use decisions, with the ability to contract collective land and manage farm activities independently based on the contract, village collectives possess inherent advantages in resource integration and management of collective assets, strongly supported by the government (Kan, Citation2016). Village collective organizations also exert significant influence on household decisions regarding agricultural production, such as implementing strategies like the ‘one village, one product’ approach to develop a competitive advantage or introducing commercial capital for rural tourism (Yang & Zhang, Citation2021). Notably, the formation of rural agricultural industry clusters and specialized villages exemplify these dynamics. Furthermore, the geographical conditions at the village level directly impact agricultural production, which cannot be fully captured by national and provincial-level studies that lack direct information on agricultural activities. As such, village-level studies hold academic and practical significance in investigating the driving forces behind NGUF, particularly in China with its unique historical and socio-economic backgrounds.
However, it is important to note that existing studies often suffer from insufficient field survey data, leading to biased and debatable results. These studies have mainly relied on household-based or statistical-based qualitative analyses and case studies, which provide valuable insights into the driving mechanisms of NGUF. Mathematical methods and econometric models, like multiple linear regression analysis, have been frequently employed (Bi et al., Citation2020). However, the generalizability of these findings has been questioned. To overcome these limitations, it is necessary to adopt more integrated analyses and engage in comprehensive discussions. This may involve the utilization of machine learning algorithms and the incorporation of data covering a wide range of regions in China. Such an approach would contribute to a more comprehensive and holistic understanding of NGUF.
We contend that the existing literature on NGUF lacks a comprehensive elucidation of the driving mechanisms at the village scale, which has been largely overlooked. Prior research predominantly relied on aggregated macro-level data, with scant employment of machine learning methodologies, thereby disregarding the nuanced disparities and heterogeneity among villages.
To redress this research lacuna and surmount the constraints of econometric models, this paper seeks to augment our comprehension of the driving mechanisms of NGUF by honing in on the village level and harnessing the potential of a machine learning algorithm. Through a meticulous village-level analysis, we aim to bridge the gap in the insubstantial examination of NGUF within this context, while alleviating the limitations inherent in econometric models. Furthermore, this study underscores the imperative of deploying robust algorithms to discern the pivotal triggers underpinning the utilization of farmland for non-grain purposes. Our findings will furnish valuable insights into the underlying factors driving NGUF, affording consequential policy implications for the regulation of agricultural activities, encompassing imperatives of national food security and ecological preservation.
2. Data and method
2.1. Data
This study relies on data primarily sourced from the Rural Vitalization Database (RVSD), derived from surveys conducted by the Rural Development Institute, Chinese Academy of Social Sciences in 2020. The selection of sample villages employed stratified, three-stage, and PPS sampling techniques (Ma & Mu, Citation2017). These selected villages are geographically dispersed across the nation, encompassing regions such as the Northeastern Plain, the Middle-Lower Yangtze Plain, and the western mountain and plateau areas. Moreover, these villages encompass diverse climate zones, including areas with single or double annual crop ripening cycles in the north and south, as well as arid or semi-arid regions in northwest China. Consequently, our sample selection is both reasonable and statistically representative on a national scale.
To gather information, a structured questionnaire was administered to village leaders by trained enumerators proficient in the local language. The dataset encompasses 283 villages across 10 provinces and the municipality of Beijing (). These provinces include both major grain producing areas with relatively lower proportions of non-grain crops, such as Heilongjiang, Henan, Shandong, and Anhui, as well as provinces facing critical circumstances, such as Zhejiang, Guangdong, Guizhou, and Beijing, where the ratio of NGUF reaches up to 50% (see ). Information regarding various aspects of agricultural production was examined, including income levels, geographical locations, leadership characteristics, poverty levels, rural labour dynamics, off-farm employment, land utilization, infrastructure, information acquisition, natural disasters, and more. This comprehensive dataset allows us to scrutinize the driving forces behind NGUF. Variable definitions and descriptive statistics for the dataset are presented in .
Table 1. The status of NGUF in each province/municipality.
Table 2. Variable definition and descriptive statistics.
2.2. Variables
Explained variables: The preference for NGUF among farmers can be measured using various methods. One commonly used indicator is the ratio of cultivated non-grain crop area to the total cultivated area, which has been widely discussed in the literature (He & Quan, Citation2014; Wang et al., Citation2011; Zhang & Du, Citation2015). Another approach involves examining the proportion of income derived from NGUF in the total household farming revenue. In this study, we adopt the ratio of cultivated non-grain crop area to the total cultivated area as a representation of the extent of NGUF.
Explanatory variables: This study employed a total of 14 explanatory variables across 10 dimensions to analyze the factors influencing NGUF. These dimensions encompassed income level, geographical location, individuality of the village leader, poverty level, rural labour, off-farm work, land use, infrastructure, information acquisition, and natural disasters (refer to ).
Income level. To assess the influence of income on agricultural investments, per capita disposable income is selected as the representative measure of income level.
Geographical location. The geographic location of villages has been found to have an impact on their access to financial resources, technological advancements, and knowledge (Li et al., Citation2019; Wang et al., Citation2007). The spatial proximity to urban regions is particularly important in relation to agricultural production. Therefore, a dummy variable indicating whether the village is located in a suburban area is included as a predictor in this study.
Individuality of the village leader. Village secretaries or heads serve as grassroots community leaders and act as intermediaries between the government and farmers (Chen & Liu, Citation2021). Driven by the pressures of performance evaluation and the pursuit of prosperity, they possess a keen interest in implementing changes within their community, particularly in administrative arrangements and decision-making regarding agricultural production. Existing literature has highlighted the significance of village leaders’ personality traits and backgrounds, such as age, education, cognitive level, and risk preference, on village agricultural output and economic development (Gao & Bi, Citation2009; Zhao & He, Citation2016). It is hypothesized that older and less educated leaders tend to exhibit conservative decision-making tendencies. In this study, the age of the village leader is chosen as the variable to describe their individual characteristics, which also indirectly reflects their level of educational attainment.
Poverty rate. To gauge the prevalence of poverty at the village level, the poverty rate is calculated as the percentage of households with incomes below the poverty line relative to the total number of households. This measure serves as an additional indicator of the economic state within the village.
Labour. Labour-related factors can significantly influence cropping choices and farmers’ options. Research suggests that non-grain crops are generally more labour-intensive compared to grain crops (Qian et al., Citation2018). Previous studies have presented varying conclusions. Some argue for the equal productivity of working-age and elderly labour due to the extended time elderly farmers dedicate to production and the assistance of agricultural innovation (Hu & Zhong, Citation2012). Conversely, others contend that the aging labour force contributes to a decline in the agricultural industry’s structural upgrades and innovation (Fried & Tauer, Citation2016). Consequently, to investigate the relationship between NGUF and a diverse workforce, this study incorporates two variables: the proportion of labour aged 60 or older and the share of the working-age population in the total population, which serve as indicators of labour supply.
Off-farm work. The expansion of secondary and tertiary industries has a bearing on labour absorption, offering higher wages compared to those derived from agricultural production. Consequently, this prompts households to adjust their labour allocation (Ochoa et al., Citation2019; Ye et al., Citation2010). To capture the extent of labour force participation, this study employs two indicators: the proportion of rural labourers engaged in non-agricultural sectors and the proportion of perennial migrant workers.
Land use. Farmland constitutes a crucial asset in the agricultural sector, as the extent of its acreage directly influences the availability of land for food production. Additionally, by manipulating internal environmental factors, protected agriculture involves the artificial construction of facilities within agricultural areas. This practice creates favourable conditions that aim to enhance the quality of agricultural production and increase productivity (Li et al., Citation2016; Tuntiwaranuruk et al., Citation2006). To depict land use patterns, this study incorporates two variables: the percentage of farmland and the proportion of land dedicated to facility-based agriculture.
Infrastructure. Enhancing agricultural productivity necessitates the presence of robust rural infrastructure, encompassing transportation and distribution channels (Zou et al., Citation2018). The availability of such infrastructure directly impacts agricultural income and non-farm labour employment opportunities. Rural roads play a pivotal role in facilitating connectivity and access to markets. Moreover, internet connectivity is now deemed an indispensable element in modern agriculture, particularly for non-grain crops that adopt advanced agricultural technologies.
Information acquisition. Social media platforms have become instrumental in facilitating the dissemination of information, offering individuals increased access to opportunities. Within a village setting, chatting groups serve as platforms for sharing and exchanging the latest agricultural market news, information on high-quality crop varieties, and more. Such platforms have the potential to influence farmers’ production decisions, extending their impact to the village level. Additionally, previous research has revealed the vital role played by WeChat and other social media groups in fostering a sense of collective identity and promoting collective actions within villages (Wu & Guo, Citation2023; Yan & Schroeder, Citation2020). This, in turn, can lead to a phenomenon known as ‘follow the trend’ in crop choice. As a measure of information accessibility, we introduce a binary variable to indicate the presence or absence of a WeChat group among villagers.
Natural disaster. The occurrence of natural disasters poses significant challenges to agricultural production. Weather conditions, climate patterns, and water availability serve as fundamental prerequisites for successful farming, but these factors are susceptible to disruptive natural events and disasters. In light of risk aversion, farmers often make adjustments to their crop choices in response (Birthal & Hazrana, Citation2019). As an explanatory variable, we include whether the village has experienced one or more natural disasters within the past three years.
2.3. Multiple linear regression
Multiple linear regression analysis estimates the relationship between an explained variable and two or more explanatory variables, under the condition of a given value for each explanatory variable. This classic econometric model is employed to determine the significance of each factor and their individual contributions to the explained variable. The insights gained from this analysis serve as valuable references for subsequent Boosted Regression Tree (BRT) modelling.
The formula for a multiple linear regression is:
(1)
(1) Where, y represents the predicted value of the ratio of the area under cultivation of non-grain crops to total cultivated area.
is the y-intercept (value of y when all other parameters are set to 0).
indicates the regression coefficient (
) and independent variable n (
).
is model error.
In this paper, the multiple linear regression analysis was performed through a function lm and a function lm.beta, and the regression coefficient for each explanatory variable is standardized. The results can be used to complement and validate the interpretations from BRT.
2.4. Boosted regression trees
BRT is an ensemble technique that combines the strengths of decision trees and boosting, providing a powerful tool for analyzing complex responses (Ratsch et al., Citation2011). This machine learning algorithm effectively addresses nonlinearities and interactions (Qin et al., Citation2020). Decision trees, a key component of BRT, explore how a response variable changes based on a set of predictor variables through recursive binary splits (Elith et al., Citation2008). This binary split process continues iteratively until a stopping criterion is met, typically by minimizing prediction error (Yang et al., Citation2016). Decision trees are renowned for their visualization capabilities, interpretability, and flexibility (Elith et al., Citation2008). Additionally, they are resilient to outliers and can handle missing data in predictor variables using surrogates (Breiman et al., Citation1984).
Boosting is an approach aimed at enhancing model precision by combining multiple weak models into a powerful ensemble that exhibits superior prediction capabilities (Hastie et al., Citation2009; Saha et al., Citation2021). In the case of boosted regression trees, stochastic gradient boosting is employed, which minimizes the loss function (deviance) at each tree split, enabling more efficient and accurate computations (Friedman, Citation2001). Unlike other ensemble methods, boosting involves a sequential training process for classifiers (Elith et al., Citation2008), and it outperforms many traditional modelling techniques (Bauer & Kohavi, Citation1999; Hastie et al., Citation2009).
The model parameterization process utilized BRTs, as devised by Friedman et al. (Citation2000), implemented in R using the ‘gbm’ package (Elith et al., Citation2008; Ridgeway, Citation2020). The model was also constructed using the ‘dismo’ (Hijmans et al., Citation2021) and ‘gbm’ (Elith & Leathwick, Citation2013) packages. Within the ‘gbm’ package, stochasticity is controlled through the ‘bag fraction’, which determines the proportion of data selected at each iteration (Elith et al., Citation2008). To optimize computational efficiency, the bagging factor was set at 0.5, as recommended by Friedman (Citation2001), reducing computation time by approximately 50% (De’ath, Citation2007).
The calibration of the model involves configuring three key parameters: the learning rate (also known as shrinkage), the number of iterations (representing the number of trees), and the maximum number of splits (indicating tree complexity) for fitting each regression tree (Hastie et al., Citation2009).
During the iterative process of adding trees to the model, the contribution of each tree is compressed by the learning rate (lr). Opting for a slower learning rate necessitates a greater number of iterations, resulting in increased computing time (Elith et al., Citation2008). It is important to strike a balance when setting the learning rate, considering both computational efficiency and predictive performance.
The number of trees (nt) in the model represents its complexity. Excessive iterations can lead to overfitting and poor generalization (Müller et al., Citation2013). To strike a balance between prediction variance in highly complex models and significant biases in overly simplistic models, it becomes crucial to address the ‘bias-variance tradeoff’ when selecting an appropriate number of trees (De’ath, Citation2007). To achieve this, employing an independent test set or cross-validation with deviance reduction as the evaluation metric is considered the optimal approach for determining the ideal nt and lr (Elith et al., Citation2008).
The concept of ‘tree complexity’ (tc) refers to the number of nodes within a tree, determining its depth and constraining the interactions between predictors and the response variable. Increasing tc allows for the capture of more intricate levels of interactions (Cheong et al., Citation2014). When aiming to fit complex trees, fewer trees are required, particularly when considering a fixed learning rate (lr). As a result, if a sufficient number of trees are fitted, reducing the lr becomes necessary as tc increases.
In our study, we utilized 10-fold cross-validation and employed the predictive deviance measure to fit the model, utilizing a ‘Laplace’ distribution. To identify the best fit, we explored various combinations of lr (0.001, 0.005, 0.01) and tc (4, 5, 6) parameters, selecting the model with the lowest deviation ().
BRT modelling provides the ability to create plots showcasing partial reliance and the relative impact of predictor variables. By analyzing the frequency of variable selection for splitting, we gain insights into the relationship between factors and the response, ultimately determining the relative importance of predictor variables (Friedman & Meulman, Citation2003). To capture the marginal impact of an independent variable on the response, while considering the average impacts of other factors, partial dependence functions are utilized (Carslaw & Taylor, Citation2009; Friedman et al., Citation2000; Friedman & Meulman, Citation2003) to generate partial dependence plots. To enhance interpretation and depict the range of predictor variables and the distribution of the response variable, smoothing splines are applied to the partial dependence plots.
Table 3. Parameter selection of BRT models with different Tree complexity and Learning rates.
3. Results and analysis
3.1. Multiple linear regression analysis
presents the outcomes of multiple regression analysis with OLS estimation. All of the variable coefficients are displayed in the third column.
Table 4. The results of OLS estimation.
Several associations have been uncovered regarding NGUF. Income level exhibits a positive correlation, while geographical location demonstrates a significant positive relationship with non-grain utilization of farmland. Interestingly, a notably negative correlation is observed between the age of the leader and NGUF. Conversely, the poverty level variable does not present a significant coefficient. In terms of rural labour, both the aging of rural labour and the number of rural labour force exhibit positive relationships with NGUF. Off-farm employment is also found to be related to the explained variable. Regarding land use variables, both variables display highly significant associations with NGUF, with coefficients of −0.204 and 0.117 respectively. However, the two variables concerning infrastructure do not play a significant positive role in facilitating the level of NGUF. Additionally, the use of WeChat and the likelihood of a disaster occurring show no connections with the explained variables.
The standardized coefficients of the explanatory factors significantly correlated with the explained variable are as follows: farmland (−0.204), age of the leader (−0.148), location (0.135), income (0.120), land used for protected agriculture (0.117), situation of rural labour aging (0.108), off-farm employment (0.074), and number of rural labour force (0.051). In general, the top five factors and the last one exhibit the expected links with the NGUF. However, the situation of rural labour aging and off-farm employment demonstrate positive impacts on NGUF, which contradicts our initial expectations and existing research on non-grain crop demand in relation to additional labour force. These factors will be given special attention in further BRT analysis and discussion.
3.2. Boosted regression tree analysis
Through correlation analysis, no significant rank correlations (rho = 0.76 > 0.70) were observed among the predictor variables. Therefore, we retained the model that incorporates all of the aforementioned variables. The final BRT model was fitted with a lr of 0.001, tc of 6, and a total of 4150 decision trees (±one standard error of 0.009). It exhibited the lowest cross-validation deviance of 0.232 (), indicating that the BRT model can reliably provide insights into the determinants of NGUF. In this study, we further identified the relative influence and marginal effects of the predictors in the BRT model.
presents the significance of predictor factors. The chosen model revealed that 10 out of 14 indicators were significantly significant, surpassing the randomness threshold of 6.1%. The farmland area exhibited the highest influence among the indicators, with a relative influence of 15.5%. The poverty rate also made a meaningful contribution to the models, with a relative relevance of 14.6%. Next in line, the situation of rural labour aging and income level emerged as the third and fourth significant predictors of NGUF, with relative importance values of 10.8% and 10.3% respectively. Additionally, variables such as the number of rural labour force (9.6%), internet access (8.5%), age of the leader (7.7%), off-farm employment (7.6%), land used for protected agriculture (6.9%), and perennial out-migration (6.1%) also made considerable contributions to the model.
Figure 3. Relative importance of explanatory variables.
Note: Relative importance of variables in percentage.

The partial dependence plots () provide insights into the marginal effects and the influence of dominant factors on non-grain output at specific stages. The marginal effect curve of farmland reveals a non-linear relationship with NGUF, characterized by reductions in two phases. The prevalence of poverty demonstrates a favourable impact on NGUF, initially experiencing a sharp decline, followed by noticeable fluctuations, and eventually stabilizing at a constant state. Income, positively associated with NGUF, exhibits a sharp increase in the curve. On the other hand, the trajectories of off-farm employment and the number of perennial out-migration show relatively smooth non-linear increases. The number of rural labour force and perennial out-migration display steady patterns with minor fluctuations around 0. In terms of the age of the leader, a non-linear negative relationship with NGUF is observed, with the curve generally decreasing despite a few small fluctuations.
4. Discussion
4.1. Contributing factors of NGUF in China
4.1.1. Partial dependence analysis
This work uses complementary methods to identify the factors that influence the non-grain production, including the multiple regression model and boosted regression trees (BRT), while the findings are partially inconsistent, but not contradictory, in terms of the explanatory variables that present no significant connections with non-grain production. Besides, it has been demonstrated that the relative contribution of each variable in different models is different. The proportion of farmland proves to be a crucial factor ranking top one in contribution both for two methods. Consistent results are drawn in terms of income, off-farm employment and the situation of rural labour aging. While the contribution of the age of the leader, location, land used for protected agriculture is underestimated in BRT compared to the multiple regression model, reversed situation happens to the number of rural labour force. These differences can be attributed to the different principles of these two methodologies. Furthermore, in BRT, the partial dependence plots () provide insights into the marginal effects and the influence of dominant factors on non-grain output at specific stages. The marginal effect curve of farmland reveals a non-linear relationship with NGUF, characterized by reductions in two phases. The prevalence of poverty demonstrates a favourable impact on NGUF, initially experiencing a sharp decline, followed by noticeable fluctuations, and eventually stabilizing at a constant state. Income, positively associated with NGUF, exhibits a sharp increase in the curve. On the other hand, the trajectories of off-farm employment and the number of perennial out-migration show relatively smooth non-linear increases. The number of rural labour force and perennial out-migration display steady patterns with minor fluctuations around 0. In terms of the age of the leader, a non-linear negative relationship with NGUF is observed, with the curve generally decreasing despite a few small fluctuations.
4.1.2. Multifaceted driving forces
Farmland acreage emerged as a significant determinant of NGUF in China, exhibiting a predominantly negative influence. This suggests that NGUF is relatively susceptible to fluctuations in farmland holdings. However, our findings diverge from Zhang and Du's (Citation2015) study, which suggests an inverted ‘U’ shaped relationship between farm size and NGUF. One possible explanation for this inconsistency is that the farm size of our samples mostly exceeds the inflection point of the curve. On the contrary, our results align with Chen and Kong's (Citation2016) interpretation, indicating a matter of considerable controversy in different regions. Further research is needed, encompassing diverse scales of farmland in both major grain-producing areas and other regions. The non-grain use of farmland exhibits a non-linear association with poverty incidence, characterized by an initial decline, followed by a rise, then a subsequent decline, and finally stabilizing. Generally, high poverty incidence constrains financial input and hampers the adoption of agricultural innovations, which hinders the implementation of technology-intensive and capital-intensive agricultural production modes. Conflicting findings have been reported regarding the status of aging rural labour (Yi & Chen, Citation2010). Our study demonstrates a positive correlation between the proportion of rural labourers older than 60 and NGUF. This could be attributed to the physical capabilities of farmers to perform demanding tasks and the limited competitiveness of aging labourers in seeking off-farm jobs, especially considering the influence of the ongoing pandemic. When sufficient labour is available, households tend to opt for non-grain crops with high profitability. The marginal effect curve of income reveals a slight increase for incomes below approximately 10,000, followed by a decrease, and then a subsequent growth trend. This can potentially be explained by the tendency of farmers with lower incomes to actively engage in agricultural production, investing more in NGUF to enhance their agricultural gains. However, as income rises, farmers may prioritize off-farm employment over agricultural production. Higher-welfare farmers, on the other hand, may invest more in NGUF for the purpose of investment. Furthermore, it is noteworthy that the age of village leaders exhibited a negative correlation with NGUF (Qian et al., Citation2018). As individuals age, they tend to adopt a more economically conservative approach, which could explain this negative correlation.
4.2. Policy recommendations: national food security vs household livelihood
Ensuring food security in rural areas of China remains a daunting task, as farmers increasingly prioritize the cultivation of profitable ‘cash crops’, potentially compromising regional or national food safety. Given the conflicts between individual and collective (national/regional) goals, this emerging trend calls for careful attention. To address this issue, policy implications should focus on striking a balance between these seemingly conflicting functions of NGUF, taking into account the underlying driving forces.
The draft of the 14th Five-Year Plan (2021–2025) by the Chinese central government marks the first mention of grain security as a priority. The plan sets a target for China to produce over 650 million tons of grain annually. Our findings, along with previous studies, have indicated that expanding cultivated areas has a positive impact on grain production. However, it has also been recognized that there exists an inverted ‘U’ shaped relationship between farm size and NGUF. This suggests that the proportion of non-grain land use increases and then decreases as farm size grows larger (Zhang & Du, Citation2015). Hence, it is crucial to identify the ‘inflection point’ to inform policymakers when formulating strategies to encourage land transfer and promote grain production. In addressing the issue of scaling up, two main approaches have been discussed in the academic literature. The normative approach views scaling up through agricultural industrialization and proposes that a market-based rental system can facilitate the lawful transfer of land to more productive operators (Ye, Citation2015; Zhang & Donaldson, Citation2008). Conversely, another perspective argues that allocating land to agribusinesses, large family farms, and farmer cooperatives, which receive government support in the form of subsidies, encourages non-grain land use (Rogers et al., Citation2021). However, other research suggests that small farms remain economically viable, and the rental market is not limited by the long-term investment required for cash crops (Rogers et al., Citation2021). Thus, agricultural production in different regions may fall in between the aforementioned turning point and large-scale production. Our findings indicate that household engagement in off-farm activities is positively associated with non-grain crop production, which contrasts with household-level results. One possible explanation is that villages are influential decision-makers and do not simply reflect the aggregation of households. Literature confirms that off-farm employment promotes land transfer, especially for those with higher off-farm income, to profit-oriented agribusinesses and large family farms(Xu et al., Citation2022; Zhou et al., Citation2017, Citation2020). To encourage participation in grain production, the promotion of agricultural and farming services, such as equipment rental, shareholding, and product distribution, should be prioritized. In recent years, agricultural trusteeship services, which involve outsourcing agricultural activities such as cultivation, planting, and harvesting to agricultural organizations or individuals for a fee, have gained popularity in rural areas. This approach allows villagers to engage in secondary and tertiary industries without leaving their farmland abandoned. With the guidance of relevant policies, this service presents a potential solution to address the non-grain phenomenon in areas with a shortage of labour force.
The shift towards diversifying livelihood activities reduces farmers’ dependence on traditional grain cultivation, as off-farm employment and profitable non-grain crops become more appealing. With urbanization accelerating, rural out-migration has become an inevitable trend in population mobility, making it crucial to provide farming services that assist the elderly and address labour shortages. The adoption of protected cultivation facilities has been proven to increase farm profits by 68–73% (Liao et al., Citation2020). China aims to improve infrastructure and mechanization to enhance output and farmers’ income through the promotion of facility-based agricultural cultivation. To safeguard farmers’ livelihoods, it is essential to encourage the mechanized production of staple crops, protected flowers, fruit trees, and vegetables. Simultaneously, ensuring food security is considered a paramount objective for the government. Technological innovations in agriculture, including advancements in seeds, irrigation, and fertilizers, are encouraged to enhance agricultural productivity (Hua et al., Citation2023). Consequently, the agricultural sector should strengthen its financial support for the adoption of new technologies in grain production and farming services. By prioritizing these measures, sustainable livelihoods can be promoted alongside efforts to ensure food security.
Our findings highlight the significance of scale when analyzing the driving forces behind NGUF (). Therefore, a range of diversified measures is required to address the non-grain utilization of farmland. Identifying the primary drivers at the village level is essential for effective planning and overall coordination by township or county governments, enabling the identification of key regions. Within the village level, households serve as the decision-makers for the choice of planting structure. Hence, household-level determinants serve as important references for village collectives. In conclusion, it is imperative to establish a hierarchical management system that considers drivers at different spatial scales. At the county level, territorial space planning systems should be optimized, taking into account factors such as cultivated land conditions, conservation red lines, local economic conditions, advantageous crops, and cultivation practices. At the township level, local governments should provide guidance to each administrative village on their development strategies, while also imposing restrictions on land transfer and increasing spending on subsidies and public services. Within the villages, village collectives should closely consider household planting decisions and introduce socialized services, particularly for off-farm households.
4.3. Contributions and limitations
This paper makes two significant contributions to the existing literature. Firstly, our quantitative approach yields intriguing findings that enhance our understanding of the driving forces behind NGUF. It expands our knowledge of the relative impact and marginal effects of various influencing factors. Our results reveal a non-linear relationship between poverty incidence and income, and offer different insights into the links between rural labour aging and off-farm employment at the village level, which diverge from some household-level studies. These findings contribute to our understanding of the patterns of NGUF across different spatial scales and hold important implications for village-level agricultural policy development.
Furthermore, our research innovatively combines econometric modelling with machine learning methodology to uncover the diverse driving forces of NGUF. This approach represents a pioneering quantitative exploration in this field. By leveraging machine learning techniques, we effectively handle variables with non-linear interactions with dependent variables, overcoming the limitations of traditional econometric models and ensuring the robustness of our findings. Additionally, with a vast sample size spanning across China, the application of machine learning and other data mining technologies becomes more practical, ensuring the validity and generalizability of our results.
While the suggested approach demonstrates substantial benefits, further advancements can still be made in the future. Firstly, village-level datasets currently lack information on the overall land transfer situations within households, which hinders our ability to understand how land reallocation affects NGUF. Future studies should strive to incorporate this crucial aspect into their analyses. Secondly, NGUF encompasses diverse forms including flower or nursery cultivation, poultry farming, and agro-tourism (Su et al., Citation2019). However, our current study measures NGUF solely by the ratio of cultivated non-grain crop area to total cultivated area, thus neglecting non-agricultural forms of NGUF. To provide a comprehensive view, future research should explore representative metrics that capture the entire spectrum of NGUF.
5. Conclusions
By employing a blended approach of multiple regression analysis and boosted regression trees model, this study discerns the pivotal determinants of Non-Grain Use of Farmland (NGUF), along with their relative contributions and marginal effects. Findings demonstrate that the size of farmland, poverty incidence, and the age of village leaders exert negative influences on NGUF. In contrast, factors such as rural labour aging, income, rural labour force size, off-farm employment opportunities, internet access, and land specifically designated for protected agriculture exhibit positive effects on non-grain utilization, exhibiting amplifying marginal effects. Of particular interest is the presence of divergent associations between certain factors, such as the proportion of labourers aged 60 or older and off-farm employment, at both the village and household levels. This underscores the significance of considering the scale at which these drivers operate when comprehending the dynamics of NGUF. Village-level data provides a comprehensive overview of resource endowment and labour factors, transcending individual household decision-making. The threshold values of marginal effects associated with these factors offer empirical insights to inform policy formulation and refinement aimed at regulating NGUF while concurrently balancing national food security with household livelihoods. Notably, non-linear interconnections are observed among poverty incidence, income, and NGUF. The central government's commitment to curbing the non-grain utilization of arable land and stabilizing grain production to safeguard food security emphasizes the imperative of enhancing grain production through future land utilization policies. Consequently, judicious planning and arrangements undertaken by government entities at the county and township levels, predicated on the driving forces identified in this study, gain heightened significance. Furthermore, village collectives assume a guiding and decisive role in the realm of Chinese agricultural production, warranting their inclusion in policy deliberations.
Disclosure statement
No potential conflict of interest was reported by the author(s).
Additional information
Funding
References
- Alston, J. M., Beddow, J. M., & Pardey, P. G. (2009). Agriculture. Agricultural research, productivity, and food prices in the long run. Science, 325(5945), 1209–1210. https://doi.org/10.1126/science.1170451
- Bauer, E., & Kohavi, R. (1999). An empirical comparison of voting classification algorithms: Bagging, boosting, and variants machine. Machine Learning, 36(1–2), 105–139. https://doi.org/10.1023/A:1007515423169
- Bi, X., Zhou, J., & Zou, W. (2020). The effect of operation scale on farmers’ planting structure selection under the constraints of family labor. China Land Science, 34, 68–77 (In Chinese). https://doi.org/10.11994/zgtdkx.20201126.180431
- Birthal, P. S., & Hazrana, J. (2019). Crop diversification and resilience of agriculture to climatic shocks: Evidence from India. Agricultural Systems, 173, 345–354. https://doi.org/10.1016/j.agsy.2019.03.005
- Breiman, L., Friedman, J. H., Stone, C. J., & Olshen, R. A. (1984). Classification and regression trees. Chapman and Hall/CRC. http://refhub.elsevier.com/S0197-3975(20)30926-7/sref7
- Carslaw, D. C., & Taylor, P. J. (2009). Analysis of air pollution data at a mixed source location using boosted regression trees. Atmospheric Environment, 43(22–23), 3563–3570. https://doi.org/10.1016/j.atmosenv.2009.04.001
- Chen, F., Liu, J., Chang, Y., Zhang, Q., Yu, H., & Zhang, A. (2021). Spatial pattern differentiation of non-grain cultivated land and its driving factors in China. China Land Science, 35, 33–43 (In Chinese). https://doi.org/10.11994/zgtdkx.20210903.093613
- Chen, J., & Kong, X. (2016). Impact analysis of farm size on grain production: Based on rural household survey in China’s 13 major grain producing areas. Hebei Academic Journal, 36(03), 122–128.
- Chen, J., Liu, R., & Zhang, J. (2009). Report on big farmers in Anhui Province: Based on survey of Huaining County and Zongyang County. China Rural Survey, (4), 2–12.
- Chen, X., & Liu, J. (2021). Village leaders, dual brokerage and political order in rural China. The China Quarterly, 247, 662–680. https://doi.org/10.1017/S0305741020000752
- Chen, X. W., Yan, X., Yang, J., Deng, X., Gao, Q., Zhang, Q., Guo, S., Ren, J., Li, S., Ye, Y., Wang, Z., Huang, J., Tang, Q., Sun, Y., Peng, X., Zhang, J., He, M., Zhu, Y., Xue, J., … Zhang, F. (2014). Producing more grain with lower environmental costs. Nature, 514(7523), 486–489. https://doi.org/10.1038/nature13609
- Cheong, Y., Leitão, P. J., & Lakes, T. (2014). Assessment of land use factors associated with dengue cases in Malaysia using boosted regression trees. Spatial and Spatio-temporal Epidemiology, 10, 75–84. https://doi.org/10.1016/j.sste.2014.05.002
- De’ath, G. (2007). Boosted trees for ecological modeling and prediction. Ecology, 88(1), 243–251. https://doi.org/10.1890/0012-9658(2007)88[243:BTFEMA]2.0.CO;2
- Elith, J., & Leathwick, J. (2013). Boosted regression trees for ecological modeling. Retrieved February 20, 2022, from http://refhub.elsevier.com/S0197-3975(20)30926-7/sref14/
- Elith, J., Leathwick, J. R., & Hastie, T. (2008). A working guide to boosted regression trees. Journal of Animal Ecology, 77(4), 802–813. https://doi.org/10.1111/j.1365-2656.2008.01390.x
- Fried, H. O., & Tauer, L. W. (2016). The aging U.S. farmer: Should we worry? In J. Aparicio, C. Lovell, & J. Pastor (Eds.), Advancesin efficiency and productivity (pp. 391–407). Springer.
- Friedman, J. H. (2001). Greedy function approximation: A gradient boosting machine. The Annals of Statistics, 29(5), 1189–1232. https://doi.org/10.1214/aos/1013203451
- Friedman, J. H., Hastie, T., & Tibshirani, R. (2000). Additive logistic regression: A statistical view of boosting. The Annals of Statistics, 28(2), 337–407. https://doi.org/10.1214/aos/1016218223
- Friedman, J. H., & Meulman, J. J. (2003). Multiple additive regression trees with application in epidemiology. Statistics in Medicine, 22(9), 1365–1381. https://doi.org/10.1002/sim.1501
- Gao, M., & Bi, L. (2009). The impact of intellectualization and rejuvenation of village leaders on household income: An empirical analysis based on micro panel data. Journal of Management World, 190, 77–84 + 92 (In Chinese). https://doi.org/10.19744/j.cnki.11-1235/f.2009.07.009
- Ge, D., Long, H., Qiao, W., Wang, Z., Sun, D., & Yang, R. (2020). Effects of rural-urban migration on agricultural transformation: A case of Yucheng City, China. Journal of Rural Studies, 76, 85–95. https://doi.org/10.1016/j.jrurstud.2020.04.010
- Hastie, T., Tibshirani, R., & Friedman, J. H. (2009). The elements of statistical learning: Data mining, inference, and prediction. Springer. https://doi.org/10.1007/978-0-387-84858-7
- He, F. (2010). The impact of rural labor force aging on Chinese food security. Seeker, 11, 74–76 (In Chinese). https://doi.org/10.16059/j.cnki.cn43-1008/c.2010.11.068
- He, P., & Quan, L. (2014). Analysis of the phenomenon of non-grain use of farmland in current China: Based on a food security perspective. Journal of Yangtze University (Natural Science Edition), 11, 73–75 (In Chinese). https://doi.org/10.16772/j.cnki.1673-1409.2014.11.019
- Hijmans, R. J., Phillips, S., Leathwick, J., & Elith, J. (2021). Dismo: Species distribution modeling, version 1.3-5. Retrieved February 20, 2022, from https://CRAN.R-project.org/package=dismo/
- Ho, P. (2005). Institutions in transition: Land ownership, property rights, and social conflict in China. Oxford University Press.
- Hu, X., & Zhong, F. (2012). The impact of rural population aging on food production: An analysis based on data from fixed rural observation sites. Chinese Rural Economy, 331(07), 29–39.
- Hua, J., Wang, H., Kang, M., Wang, X., Guo, S., Chang, F., & Wang, F. (2023). The design and implementation of a distributed agricultural service system for smallholder farmers in China. International Journal of Agricultural Sustainability, 21(1), 2221108. https://doi.org/10.1080/14735903.2023.2221108
- Huang, Y., & Tian, X. (2019). Food accessibility, diversity of agricultural production and dietary pattern in rural China. Food Policy, 84, 92–102. https://doi.org/10.1016/j.foodpol.2019.03.002
- Kan, K. (2016). The transformation of the village collective in uranising China: A historical institutional analysis. Journal of Rural Studies, 47, 588–600. https://doi.org/10.1016/j.jrurstud.2016.07.016
- Kong, X. (2020). Problems, causes and countermeasures of non-grain conversion of cultivated land. China Land, 11, 17–19 (In Chinese). https://doi.org/10.13816/j.cnki.ISSN1002-9729.2020.11.05
- Leng, Z., Xing, Y., & Qian, L. (2020). Impact of gender structure of agricultural labor force on grain production: An empirical analysis based on CFPS data. Finance and Trade Research, 31, 36–48 (In Chinese). https://doi.org/10.19337/j.cnki.34-1093/f.2020.12.003
- Li, H., He, J., Bharucha, Z., Lal, R., & Pretty, J. (2016). Improving China’s food and environmental security with conservation agriculture. International Journal of Agricultural Sustainability, 14(4), 377–391. https://doi.org/10.1080/14735903.2016.1170330
- Li, Y., Fan, P., & Liu, Y. (2019). What makes better village development in traditional agricultural areas of China? Evidence from long-term observation of typical villages. Habitat International, 83, 111–124. https://doi.org/10.1016/j.habitatint.2018.11.006
- Liang, X., Li, Y., Ran, C., Li, M., & Zhang, H. (2020). Study on the transformed farmland landscape in rural areas of southwest China: A case study of Chongqing. Journal of Rural Studies, 76, 272–285. https://doi.org/10.1016/j.jrurstud.2020.04.017
- Liao, P., Liu, J., Sun, L., & Chang, H. (2020). Can the adoption of protected cultivation facilities affect farm sustainability. Sustainability, 12(23), 9970. https://doi.org/10.3390/su12239970
- Liu, H., & Zhang, L. (2020). Will agricultural land transfer lead to non-grain use of farmland: An empirical analysis at the parcel level. Rural Economy, (11), 45–53.
- Luo, B., Jiang, X., Li, S., & Qiu, T. (2018). Will agricultural land transfer lead to non-grain planting structure. Jianghai Academic Journal (Bimonthly), (02), 94–101.
- Luo, B., & Qiu, T. (2018). The adjustment of China's agricultural planting structure: “non-grain trend” or “grain trend”. Social Science Front, (02), 39–51.
- Ma, S., & Mu, R. (2017). Forced off farm? Labor allocation response to land requisition in rural China. IZA Working Paper, 10640. https://doi.org/10.2139/ssrn.2940630
- Ma, X., Heerink, N., Feng, S., & Shi, X. (2015). Farmland tenure in China: Comparing legal, actual and perceived security. Land Use Policy, 42, 293–306. https://doi.org/10.1016/j.landusepol.2014.07.020
- Müller, D., Leitão, P. J., & Sikor, T. (2013). Comparing the determinants of cropland abandonment in Albania and Romania using boosted regression trees. Agricultural Systems, 117, 66–77. https://doi.org/10.1016/j.agsy.2012.12.010
- Ochoa, M. W. S., Härtl, F. H., Paul, C., & Knoke, T. (2019). Cropping systems are homogenized by off-farm income: Empirical evidence from small-scale farming systems in dry forests of southern Ecuador. Land Use Policy, 82, 204–219. https://doi.org/10.1016/j.landusepol.2018.11.025
- Peng, L., Zhou, X., Tan, W., Liu, J., & Wang, Y. (2020). Analysis of dispersed farmers’willingness to grow grain and main influential factors based on the structural equation model. Journal of Rural Studies, https://doi.org/10.1016/j.jrurstud.2020.01.001
- Qian, L., Yuan, H., Liu, J., & Cao, B. (2018). The impacts of off-farm employment and farmland circulation on farmers’ grain planting structure adjustment: A microcosmic study of selected fixed national rural observation points. Journal Research of Agricultural Modernization, 39(5), 789–797 (In Chinese). https://doi.org/10.13872/j.1000-0275.2018.0070
- Qian, W., Wang, D., & Zheng, L. (2016). The impact of migration on agricultural restructuring: Evidence from Jiangxi Province in China. Journal of Rural Studies, 47, 542–551. https://doi.org/10.1016/j.jrurstud.2016.07.024
- Qin, X., Li, Y., Lu, Z., & Pan, W. (2020). What makes better village economic development in traditional agricultural areas of China? Evidence from 338 villages. Habitat International, 106, 102286. https://doi.org/10.1016/j.habitatint.2020.102286
- Qiu, T., Boris Choy, S. T., Li, S., He, Q., & Luo, B. (2020). Does land renting-in reduce grain production? Evidence from rural China. Land Use Policy, 90, 104311. https://doi.org/10.1016/j.landusepol.2019.104311
- Ratsch, G., Onoda, T., & Muller, K. (2011). Soft margins for AdaBoost. Machine Learning, 42(3), 287–320. https://doi.org/10.1023/A:1007618119488
- Ridgeway, G. (2020). Generalized boosted models: A guide to the gbm package, version 2.1.8. Retrieved February 20, 2022, from https://cran.r-project.org/web/packages/gbm/vignettes/gbm.pdf/
- Rogers, S., Wilmsen, B., Han, X., Wang, Z. J., Duan, Y., He, J., Li, J., Lin, W., & Wong, C. (2021). Scaling up agriculture? The dynamics of land transfer in inland China. World Development, 146, 105563. https://doi.org/10.1016/j.worlddev.2021.105563
- Rozelle, S., Taylor, J. E., & DeBrauw, A. (1999). Migration, remittances, and agricultural productivity in China. American Economic Review, 89(2), 287–291. https://doi.org/10.1257/aer.89.2.287
- Saha, S., Arabameri, A., Saha, A., Blaschke, T., Ngo, P. T. T., Nhu, V. H., & Band, S. S. (2021). Prediction of landslide susceptibility in Rudraprayag, India using novel ensemble of conditional probability and boosted regression tree-based on cross-validation method. Science of The Total Environment, 764, 142928. https://doi.org/10.1016/j.scitotenv.2020.142928
- Su, Y., Li, C., Wang, K., Deng, J., Shahtahmassebi, A. R., Zhang, L., Ao, W., Guan, T., Pan, Y., & Gan, M. (2019). Quantifying the spatiotemporal dynamics and multi-aspect performance of non-grain production during 2000–2015 at a fine scale. Ecological Indicators, 101, 410–419. https://doi.org/10.1016/j.ecolind.2019.01.026
- Su, Y., Qian, K., Lin, L., Wang, K., Guan, T., & Gan, M. (2020). Identifying the driving forces of non-grain production expansion in rural China and its implications for policies on cultivated land protection. Land Use Policy, 92, 104435. https://doi.org/10.1016/j.landusepol.2019.104435
- Sun, W., Chen, Y., Guo, Y., Chen, Z., Guo, L., Yang, X., & Lin, J. (2021). Research on the spatial characteristics and benefits of “non-grained” cultivated land at the county scale: Take Longnan city, Jiangxi as an example. Journal of Gannan Normal University, 42, 97–102 (In Chinese). https://doi.org/10.13698/j.cnki.cn36-1346/c.2021.06.020
- Tuntiwaranuruk, U., Thepa, S., Tia, S., & Bhumiratana, S. (2006). Modeling of soil temperature and moisture with and without rice husks in an agriculture greenhouse. Renewable Energy, 31(12), 1934–1949. https://doi.org/10.1016/j.renene.2005.09.017
- Veeck, G., Veeck, A., & Yu, H. (2020). Challenges of agriculture and food systems issues in China and the United States. Geography and Sustainability, 1(2), 109–117. https://doi.org/10.1016/j.geosus.2020.05.002
- Wang, H., Wei, J., & Wu, G. (2007). Impacts of socio-economic policies on agriculture in the mountainous upland of the Dalinghe river watershed, Northeast China. International Journal of Agricultural Sustainability, 5(4), 331–342. https://doi.org/10.1080/14735903.2007.9684832
- Wang, S., Bai, X., Zhang, X., Reis, S., Chen, D., Xu, J., & Gu, B. (2021). Urbanization can benefit agricultural production with large-scale farming in China. Nature Food, 2(3), 183–191. https://doi.org/10.1038/s43016-021-00228-6
- Wang, S., & Tian, X. (2018). The influence of rural labor force aging on agricultural production: An empirical analysis of cultivated land terrain. Journal of Agrotechnical Economics, 4, 15–26 (In Chinese). https://doi.org/10.13246/j.cnki.jae.2018.04.002
- Wang, Y., Chen, Y., Yi, X., & Xiao, B. (2011). The non-grain problem in the process of land transfer and the countermeasures. Chinese Journal of Agricultural Resources and Regional Planning, 32(04), 13–16.
- Wu, Y., Zhang, P., Yu, Y., & Xie, R. (2021). Research progress and prospect of “NGUF” of cultivated land in China from the perspective of food security. China Land Science, 35, 116–124 (In Chinese). https://doi.org/10.11994/zgtdkx.20210909.102404
- Wu, Z., & Guo, C. (2023). From tweeters to whispering WeChat groups: Rural publicness reproduction and social governance transformation: A field survey based on a village in North China. China Rural Observation, 170, 34–52 (In Chinese). https://doi.org/10.20074/j.cnki.11-3586/f.2023.02.002
- Xu, C., Wang, Q., Fahad, S., Kagatsume, M., & Yu, J. (2022). Impact of off-farm employment on farmland transfer: Insight on the mediating role of agricultural production service outsourcing. Agriculture, 12(10), 1617. https://doi.org/10.3390/agriculture12101617
- Xue, X., & Zhang, Y. (2017). Current situation, causes and countermeasures regarding non-grain growing of farmland in major grain-producing counties. Academic Journal of Zhongzhou, (08), 40–45.
- Yan, P., & Schroeder, R. (2020). Variations in the adoption and use of mobile social apps in everyday lives in urban and rural China. Mobile Media & Communication, 8(3), 318–341. https://doi.org/10.1177/2050157919884718
- Yang, J., Wan, Q., & Bi, W. (2020). Off-farm employment and grain production change: New evidence from China. China Economic Review, 63, 101519. https://doi.org/10.1016/j.chieco.2020.101519
- Yang, Q., & Zhang, D. (2021). The influence of agricultural industrial policy on non-grain production of cultivated land: A case study of the “one village, one product” strategy implemented in Guanzhong Plain of China. Land Use Policy, 108, 105579. https://doi.org/10.1016/j.landusepol.2021.105579
- Yang, R. M., Zhang, G. L., Liu, F., Lu, Y. Y., Yang, F. F., Yang, F., Yang, M., Zhao, Y. G., & Li, D. C. (2016). Comparison of boosted regression tree and random forest models for mapping topsoil organic carbon concentration in an alpine ecosystem. Ecological Indicators, 60, 870–878. https://doi.org/10.1016/j.ecolind.2015.08.036
- Ye, C., Xu, X., & Hu, Z. (2010). Analysis on the effect to the income structure of farmers by the process of urbanization. Urban Development Studies, 17(10), 26–30.
- Ye, J. (2015). Land transfer and the pursuit of agricultural modernization in China. Journal of Agrarian Change, 15(3), 314–337. https://doi.org/10.1111/joac.12117
- Ye, Y., Qi, Q., Jiang, L., & Li, X. (2017). Spatial-temporal changes in grain input-output and the driving mechanism in China since 1985. International Journal of Agricultural Sustainability, 15(4), 445–456. https://doi.org/10.1080/14735903.2017.1335571
- Yi, X., & Chen, Y. (2010). Analysis of farmers’ behavior and scale of non-grain use of farmland: Based on the survey data of farmers in Zhejiang and Hebei provinces. China Rural Observation, (06), 2–10.
- Yu, L., & Huang, W. (2020). Non-economic societal impact or economic revenue? A performance and efficiency analysis of farmer cooperatives in China. Journal of Rural Studies, 80, 123–134. https://doi.org/10.1016/j.jrurstud.2020.08.010
- Zhang, Q. F., & Donaldson, J. A. (2008). The rise of agrarian capitalism with Chinese characteristics: Agricultural modernization, agribusiness and collective land rights. The China Journal, 60, 25–47. https://doi.org/10.1086/tcj.60.20647987
- Zhang, Z., & Du, Z. (2015). Will land transfer necessarily lead to non-grain use of farmland: Empirical analysis of monitoring data from 1740 family farms in China. Economic Perspectives, (09), 63–69.
- Zhao, R., & He, A. (2016). Village cadres quality, village democracy and farmers’ income: Empirical analysis based on CHIPS data. Nankai Economic Studies, 188, 129–152 (In Chinese). https://doi.org/10.14116/j.nkes.2016.02.008
- Zhong, F., Lu, W., & Xu, Z. (2016). Is the rural labor force working outside not conducive to grain production: Analysis of substitution of peasant household elements, adjustment behavior of planting structure and constraint conditions. Chinese Rural Economy, (07), 36–47.
- Zhou, L. Y., Rao, F. P., & Ma, X. L. (2017). The effects of off-farm employment type on arable land transfer in hilly areas of Jiangxi. Resources Science, 39, 209–219. https://doi.org/10.18402/resci.2017.02.04
- Zhou, X., Ma, W., Renwickb, A., & Li, G. (2020). Off-farm work decisions of farm couples and land transfer choices in rural China. Applied Economics, 52(57), 6229–6247. https://doi.org/10.1080/00036846.2020.1788709
- Zhu, H. (2016). Review on the non-grain phenomenon in farmland transfer and its influence on food security. People's Tribune, 17, 82–84 (In Chinese). https://doi.org/10.16619/j.cnki.rmlt.2016.17.027
- Zou, B., Mishra, A. K., & Luo, B. (2018). Aging population, farm succession, and farmland usage: Evidence from rural China. Land Use Policy, 77, 437–445. https://doi.org/10.1016/j.landusepol.2018.06.001